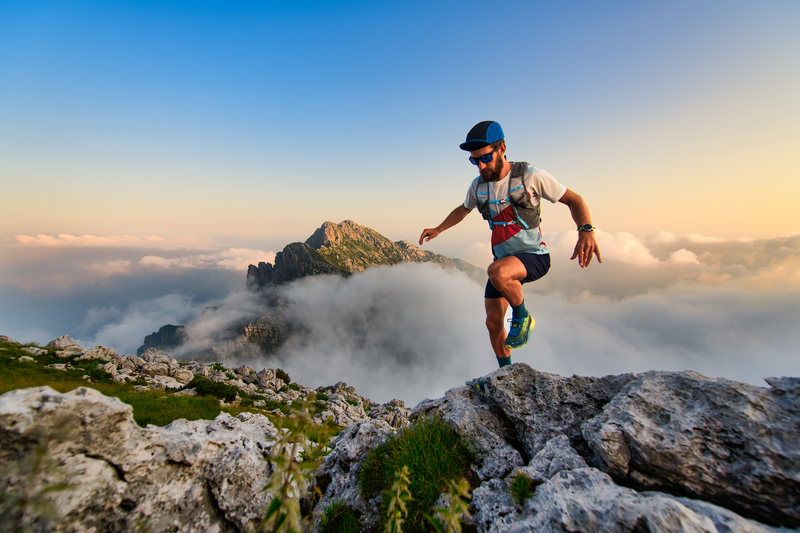
94% of researchers rate our articles as excellent or good
Learn more about the work of our research integrity team to safeguard the quality of each article we publish.
Find out more
ORIGINAL RESEARCH article
Front. Immunol.
Sec. Cancer Immunity and Immunotherapy
Volume 16 - 2025 | doi: 10.3389/fimmu.2025.1530789
This article is part of the Research Topic Immune Predictive and Prognostic Biomarkers in Immuno-Oncology: Refining the Immunological Landscape of Cancer View all 10 articles
The final, formatted version of the article will be published soon.
You have multiple emails registered with Frontiers:
Please enter your email address:
If you already have an account, please login
You don't have a Frontiers account ? You can register here
Background: Pancreatic adenocarcinoma (PAAD) ranks among the most lethal malignancies worldwide. Current treatment options have limited efficacy, underscoring the need for new therapeutic targets. Methods: This study employed a multi-omics analytical framework to delve into the expression profiles and prognostic implications of ADGRG6 within the pan-cancer dataset of The Cancer Genome Atlas (TCGA) database, highlighting the prognostic value and potential carcinogenic role of ADGRG6 in PAAD, which was further validated using data from multiple PAAD cohorts in the Gene Expression Omnibus (GEO) database. To assess the role of ADGRG6 in the tumor microenvironment of PAAD, we evaluated immune infiltration using CIBERSORT, ssGSEA, xCell and Tracking Tumor Immunophenotype (TIP), and utilized single-cell sequencing data to explore cell-cell interactions. Further cellular and animal experiments, such as colony formation assay, transwell assay, western blot, real-time PCR, and tumor xenograft experiments, were used to investigate the effect of ADGRG6 on the proliferation, metastatic potential and immune marker expression of PAAD and the underlying mechanisms.Results: ADGRG6 emerged as a potential prognostic biomarker and a therapeutic target for PAAD, which was further corroborated by data extracted from multiple PAAD cohorts archived in the GEO database. Single-cell sequencing and immune infiltration analyses predicted the positive correlation of ADGRG6 with the infiltration of immune cells and with the interaction between malignant cells and fibroblasts/macrophages within the PAAD microenvironment. In vitro cell assays demonstrated that ADGRG6 promoted the proliferation, metastatic potential and immune marker expression of PAAD cells by increasing protein level of mutated p53 (mutp53), which activated a spectrum of gain-of-functions to promote cancer progression via the EGFR, AMPK and NF-κB signaling cascades. Furthermore, subcutaneous xenograft experiments in mice demonstrated that ADGRG6 knockdown substantially suppressed the growth of engrafted PAAD tumors.Conclusions: ADGRG6 may serve as a novel prognostic marker and a therapeutic targets for PAAD, playing a crucial role in the proliferation, metastasis, and immune marker regulation of PAAD through elevating protein level of mutated p53.
Keywords: Pancreatic Cancer, ADGRG6, Mutated p53, prognosis marker, membrane receptors
Received: 19 Nov 2024; Accepted: 10 Mar 2025.
Copyright: © 2025 Wu, Shang, Bao, Liu, Zhang, Xiao, Li, Huang, Cheng, Ma, Zhang, Wang, Zhang and Zhan. This is an open-access article distributed under the terms of the Creative Commons Attribution License (CC BY). The use, distribution or reproduction in other forums is permitted, provided the original author(s) or licensor are credited and that the original publication in this journal is cited, in accordance with accepted academic practice. No use, distribution or reproduction is permitted which does not comply with these terms.
* Correspondence:
Daxuan Wang, Provincial College of Clinical Medicine, Fujian Provincial Hospital, Fuzhou, Fujian Province, China
Mingqing Zhang, Department of Gastroenterology, The 909th Hospital, School of Medicine, Zhangzhou, Fujian Province, China
Yan-yan Zhan, Cancer Research Center, School of Medicine, Xiamen University, Xiamen, Fujian Province, China
Disclaimer: All claims expressed in this article are solely those of the authors and do not necessarily represent those of their affiliated organizations, or those of the publisher, the editors and the reviewers. Any product that may be evaluated in this article or claim that may be made by its manufacturer is not guaranteed or endorsed by the publisher.
Research integrity at Frontiers
Learn more about the work of our research integrity team to safeguard the quality of each article we publish.