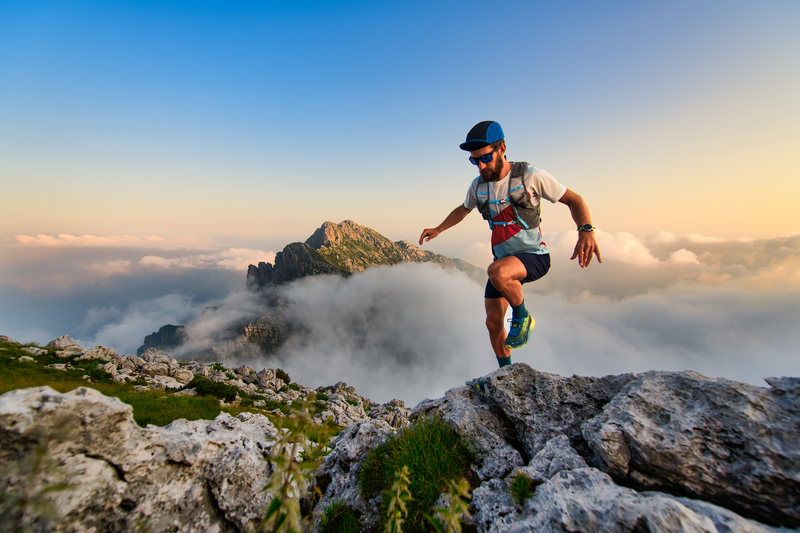
94% of researchers rate our articles as excellent or good
Learn more about the work of our research integrity team to safeguard the quality of each article we publish.
Find out more
REVIEW article
Front. Immunol.
Sec. Cancer Immunity and Immunotherapy
Volume 16 - 2025 | doi: 10.3389/fimmu.2025.1530305
The final, formatted version of the article will be published soon.
You have multiple emails registered with Frontiers:
Please enter your email address:
If you already have an account, please login
You don't have a Frontiers account ? You can register here
Glioblastomas are the most prevalent primary brain tumors and are associated with a dramatically poor prognosis. Despite an intensive treatment approach, including maximal surgical tumor removal followed by radio-and chemotherapy, the median survival for glioblastoma patients has remained around 18 months for decades. Glioblastoma is distinguished by its highly complex mechanisms of immune evasion and pronounced heterogeneity. This variability is apparent both within the tumor itself, which can exhibit multiple phenotypes simultaneously, and in its surrounding microenvironment. Another key feature of glioblastoma is its "cold" microenvironment, characterized by robust immunosuppression. Recent advances in single-cell RNA sequencing have uncovered new promising insights, revealing previously unrecognized aspects of this tumor. In this review, we consolidate current knowledge on glioblastoma cells and its microenvironment, with an emphasis on their biological properties and unique patterns of molecular communication through signaling pathways. The evidence underscores the critical need for personalized poly-immunotherapy and other approaches to overcome the plasticity of glioblastoma stem cells. Analyzing the tumor microenvironment of individual patients using single-cell transcriptomics and implementing a customized immunotherapeutic strategy could potentially improve survival outcomes for those facing this formidable disease.
Keywords: Glioblastoma, Glioma, ligand-receptor interaction, Signaling Pathways, Tumor Microenvironment, Glioblastoma stem cells, cancer-associated fibroblasts, Immunotherapy
Received: 18 Nov 2024; Accepted: 07 Mar 2025.
Copyright: © 2025 Ismailov, Spallone, Belogurov, Herbert and Poptsova. This is an open-access article distributed under the terms of the Creative Commons Attribution License (CC BY). The use, distribution or reproduction in other forums is permitted, provided the original author(s) or licensor are credited and that the original publication in this journal is cited, in accordance with accepted academic practice. No use, distribution or reproduction is permitted which does not comply with these terms.
* Correspondence:
Maria Poptsova, National Research University Higher School of Economics, Moscow, Russia
Disclaimer: All claims expressed in this article are solely those of the authors and do not necessarily represent those of their affiliated organizations, or those of the publisher, the editors and the reviewers. Any product that may be evaluated in this article or claim that may be made by its manufacturer is not guaranteed or endorsed by the publisher.
Research integrity at Frontiers
Learn more about the work of our research integrity team to safeguard the quality of each article we publish.