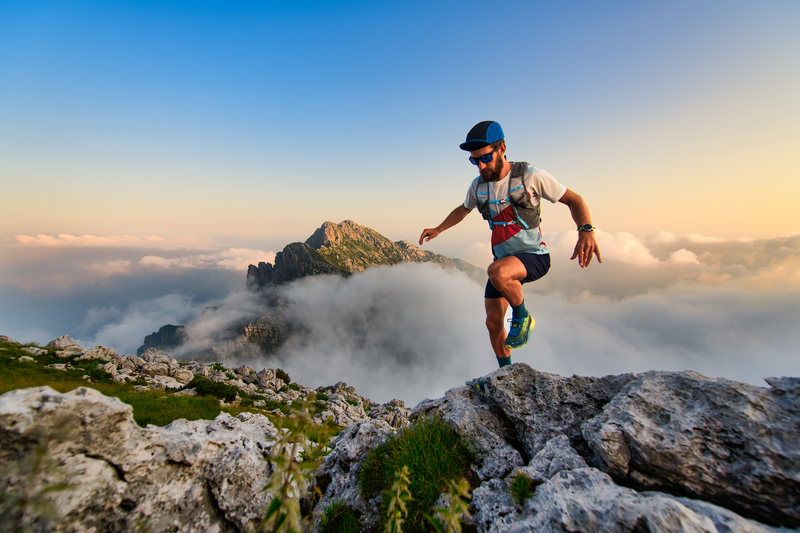
94% of researchers rate our articles as excellent or good
Learn more about the work of our research integrity team to safeguard the quality of each article we publish.
Find out more
ORIGINAL RESEARCH article
Front. Immunol.
Sec. Systems Immunology
Volume 16 - 2025 | doi: 10.3389/fimmu.2025.1530214
The final, formatted version of the article will be published soon.
You have multiple emails registered with Frontiers:
Please enter your email address:
If you already have an account, please login
You don't have a Frontiers account ? You can register here
Background: Domestication of wild boars into local and intensive pig breeds has driven adaptive genomic changes, resulting in significant phenotypic differences in intestinal immune function. The intestine relies on diverse immune cells, but their evolutionary changes during domestication remain poorly understood at single-cell resolution. Methods: We performed single-cell RNA sequencing (scRNA-seq) and marker gene analysis on jejunal tissues from wild boars, a Chinese local breed (Jinhua), and an intensive breed (Duroc). Then, we developed an immune cell evaluation system that includes immune scoring, gene identification, and cell communication analysis. Additionally, we mapped domestication-related clustering relationships, highlighting changes in gene expression and immune function.We generated a single-cell atlas of jejunal tissues, analyzing 26,246 cells and identifying 11 distinct cell lineages, including epithelial and plasma cells, and discovered shared and unique patterns in intestinal nutrition and immunity across breeds. Immune cell evaluation analysis confirmed the conservation and heterogeneity of immune cells, manifested by highly conserved functions of immune cell subgroups, but wild boars possess stronger immune capabilities than domesticated breeds. We also discovered four patterns of domestication-related breed-specific genes related to metabolism, immune surveillance, and cytotoxic functions. Lastly, we identified a unique population of plasma cells with distinctive antibody production in Jinhua pig population. Conclusions: Our findings provide valuable single-cell insights into the cellular heterogeneity and immune function evolution in the jejunum during pig at various domestication stages. The singlecell atlas also serves as a resource for comparative studies and supports breeding programs aimed at enhancing immune traits in pigs.
Keywords: single-cell RNA sequencing, Jejunum, immune cells, Domestication, Plasma Cells
Received: 18 Nov 2024; Accepted: 11 Feb 2025.
Copyright: © 2025 Fu, Xie, Yu, Liu, Xu, Ye, Zhao, Wang, Pan, Zhang and Wang. This is an open-access article distributed under the terms of the Creative Commons Attribution License (CC BY). The use, distribution or reproduction in other forums is permitted, provided the original author(s) or licensor are credited and that the original publication in this journal is cited, in accordance with accepted academic practice. No use, distribution or reproduction is permitted which does not comply with these terms.
* Correspondence:
Zhe Zhang, Zhejiang University, Hangzhou, China
Zhen Wang, Zhejiang University, Hangzhou, China
Disclaimer: All claims expressed in this article are solely those of the authors and do not necessarily represent those of their affiliated organizations, or those of the publisher, the editors and the reviewers. Any product that may be evaluated in this article or claim that may be made by its manufacturer is not guaranteed or endorsed by the publisher.
Research integrity at Frontiers
Learn more about the work of our research integrity team to safeguard the quality of each article we publish.