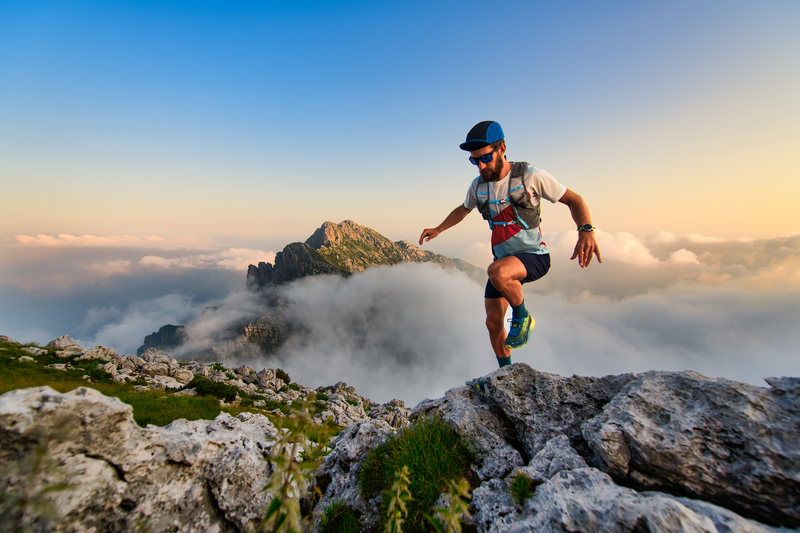
94% of researchers rate our articles as excellent or good
Learn more about the work of our research integrity team to safeguard the quality of each article we publish.
Find out more
ORIGINAL RESEARCH article
Front. Immunol.
Sec. Cancer Immunity and Immunotherapy
Volume 16 - 2025 | doi: 10.3389/fimmu.2025.1529707
This article is part of the Research Topic Current Insights in Melanoma Immunology, Immune Escape and Immunotherapy Advances View all 4 articles
The final, formatted version of the article will be published soon.
You have multiple emails registered with Frontiers:
Please enter your email address:
If you already have an account, please login
You don't have a Frontiers account ? You can register here
Background and Purpose: Promoting adaptive immunity with ICIs has drastically improved melanoma prognosis, but not for all patients. Some cases relapse in the first few months, while others keep durable benefit, even after immunotherapy discontinuation. To identify cellular/molecular signatures in peripheral blood that could differentiate advanced from metastatic melanoma and predict dynamics for primary/secondary immune escape, we examined 100 consecutive patients with stage III/IV melanoma scheduled to start ICIs . Materials and Methods: At melanoma diagnosis, a multiparameter flow cytometric analysis and purification scheme using standard conjugated antibodies were performed for all individuals prior to ICI initiation. In each stage (III/IV) according to their RFS/PFS, we retrospectively selected the cases with the clearest clinical outcomes and focused our analysis on the extreme responders (n=7) and non-responders (n=7) to characterize the transcriptomes of circulating CD4+T-cells by bulk RNA-seq, Differential Expression Analysis(DEA)and Gene Ontology (GO) enrichment analysis. Based on our selected patient cohort, we examined for differentially expressed genes (DEGs) and key-pathways that appear preferentially activated in stage III vs. IV melanoma, and in long vs. short immunotherapy responders.Results: Although circulating immune-cells did not numerically differ in both sets of analysis(staging and ICI responsiveness), DEA and GO data showed that patients could be clustered separately, identifying 189vs.92 DEGs in stage IV/III and 101vs.47 DEGs in early progressors/long responders. These DEGs were functionally implicated in distinct pathways. For metastatic cases: inflammatory response (log p-value=-9.2: ADGRE5/2,CYBA,GRN,HMOX1,IRF5,ITGAM), adaptive immunity(log p-value=-7.7: CD1C,CD74,CYBB,NCF2,CTSA,S100A8/9,BCL3,FCER1G), T-cell activation(log p-value=-6.3: BCL3,CD1C,CD74,FCER1G,FGL2)and lipid metabolism/catabolism(log p-value=-2.5/-2.6: ARF3,GPX1,MVD,OCRL,PCCB,CTSA,PNPLA2,NAGLU,GBA2,ABHD4); while in early-progressors to ICIs: immune effector processing(log p-value=-13.7: BCL6,FGR,HLA-DQA1/DQB1,HLA-DRA,HLA-DRB1/DRB5,NKG7,SLC11A1,TYROBP,SPON2,HAVCR2),PD-1(log p-value=-10.2: HLA-DQA1/DQB1,HLA-DRA,HLA-DRB1/DRB5)and IFN signaling(logp-value=-8.5: HLA-DQA1/DQB1,HLA-DRA,HLA-DRB1/DRB5,NCAM1,IFITM3),positive regulation of T-cell activation(log p-value=-7.7: BCL6,HLA-DQA1/DQB1,HLA-DRA,HLA-DRB1/DRB5,SASH3,HAVCR2)and CD28 co-stimulation(log p-value=-10.3: HLA-DQA1/DQB1,HLA-DRA,HLA-DRB1/DRB5), supporting an immune-mediated behavior. Conclusions: Specific pathways and marker genes in the peripheral CD4+T-cells may predetermine melanoma staging and immunotherapy resistance.
Keywords: For metastatic cases: inflammatory response(logp-value=-9.2:ADGRE5/2, cybA, GRN, HMOX1, IRF5, ITGAM), adaptive immunity(logp-value=-7.7:CD1C, CD74
Received: 17 Nov 2024; Accepted: 10 Mar 2025.
Copyright: © 2025 Palli, Lavigne, Verginis, Alissafi, Anastasopoulou, Lyrarakis, Kirkwood, Gogas and Ziogas. This is an open-access article distributed under the terms of the Creative Commons Attribution License (CC BY). The use, distribution or reproduction in other forums is permitted, provided the original author(s) or licensor are credited and that the original publication in this journal is cited, in accordance with accepted academic practice. No use, distribution or reproduction is permitted which does not comply with these terms.
* Correspondence:
Dimitrios C. Ziogas, National and Kapodistrian University of Athens, Athens, Greece
Disclaimer: All claims expressed in this article are solely those of the authors and do not necessarily represent those of their affiliated organizations, or those of the publisher, the editors and the reviewers. Any product that may be evaluated in this article or claim that may be made by its manufacturer is not guaranteed or endorsed by the publisher.
Research integrity at Frontiers
Learn more about the work of our research integrity team to safeguard the quality of each article we publish.