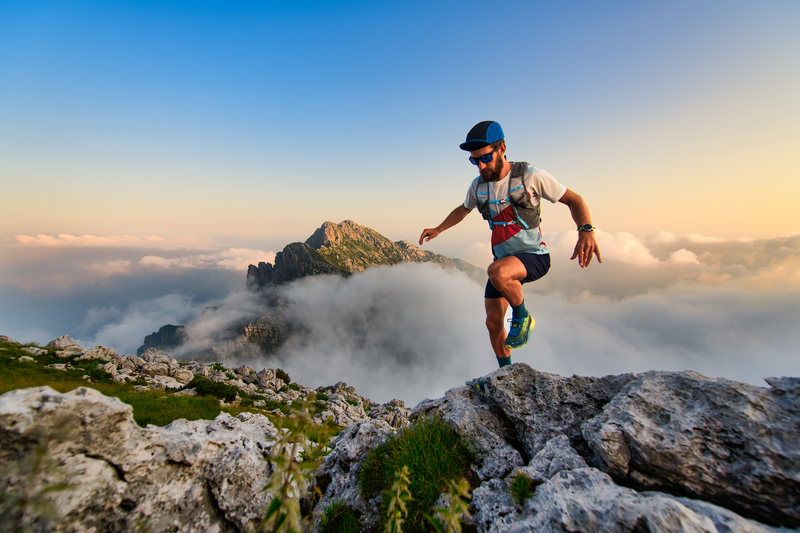
95% of researchers rate our articles as excellent or good
Learn more about the work of our research integrity team to safeguard the quality of each article we publish.
Find out more
REVIEW article
Front. Immunol.
Sec. Cancer Immunity and Immunotherapy
Volume 16 - 2025 | doi: 10.3389/fimmu.2025.1526518
This article is part of the Research Topic Artificial Intelligence for Cancer Immunotherapy View all 6 articles
The final, formatted version of the article will be published soon.
You have multiple emails registered with Frontiers:
Please enter your email address:
If you already have an account, please login
You don't have a Frontiers account ? You can register here
Radiation therapy remains a cornerstone in the treatment of cancer, with the delineation of Organs at Risk (OARs), tumors, and malignant lymph nodes playing a critical role in the planning process. However, the manual segmentation of these anatomical structures is both time-consuming and costly, with inter-observer and intra-observer variability often leading to delineation errors. In recent years, deep learning-based automatic segmentation has gained increasing attention, leading to a proliferation of scholarly works on OAR and tumor segmentation algorithms utilizing deep learning techniques. Nevertheless, similar comprehensive reviews focusing solely on malignant lymph nodes are scarce. This paper provides an in-depth review of the advancements in deep learning for malignant lymph node segmentation and detection. After a brief overview of deep learning methodologies, the review examines specific models and their outcomes for malignant lymph node segmentation and detection across five clinical sites: head and neck, upper extremity, chest, abdomen, and pelvis.The discussion section extensively covers the current challenges and future trends in this field, analyzing how they might impact clinical applications. This review aims to bridge the gap in literature by providing a focused overview on deep learning applications in the context of malignant lymph node challenges, offering insights into their potential to enhance the precision and efficiency of cancer treatment planning.
Keywords: deep learning, Lymph Node, segmentation, detection, Delineation
Received: 11 Nov 2024; Accepted: 17 Mar 2025.
Copyright: © 2025 Wu, Laville, Deutsch and Sun. This is an open-access article distributed under the terms of the Creative Commons Attribution License (CC BY). The use, distribution or reproduction in other forums is permitted, provided the original author(s) or licensor are credited and that the original publication in this journal is cited, in accordance with accepted academic practice. No use, distribution or reproduction is permitted which does not comply with these terms.
* Correspondence:
Roger Sun, Institut Gustave Roussy, Villejuif, France
Disclaimer: All claims expressed in this article are solely those of the authors and do not necessarily represent those of their affiliated organizations, or those of the publisher, the editors and the reviewers. Any product that may be evaluated in this article or claim that may be made by its manufacturer is not guaranteed or endorsed by the publisher.
Research integrity at Frontiers
Learn more about the work of our research integrity team to safeguard the quality of each article we publish.