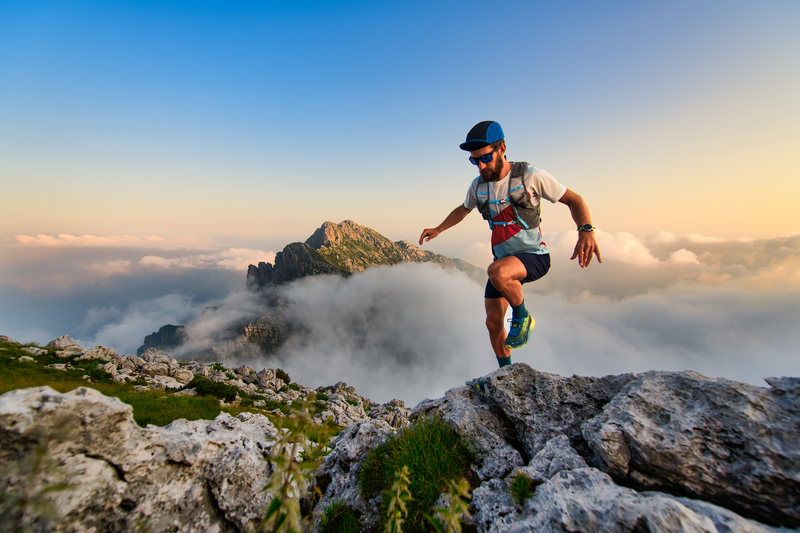
94% of researchers rate our articles as excellent or good
Learn more about the work of our research integrity team to safeguard the quality of each article we publish.
Find out more
ORIGINAL RESEARCH article
Front. Immunol.
Sec. Systems Immunology
Volume 16 - 2025 | doi: 10.3389/fimmu.2025.1524120
This article is part of the Research Topic Mathematical Modeling in Discovery and Analysis of Immune Responses View all 5 articles
The final, formatted version of the article will be published soon.
You have multiple emails registered with Frontiers:
Please enter your email address:
If you already have an account, please login
You don't have a Frontiers account ? You can register here
Tumor-infiltrating B lymphocytes (TILBs) play a pivotal role in molding the immune microenvironment of tumors (TIME) and in the course of cancer development within lung adenocarcinoma (LUAD). Despite their recognized importance, comprehensive and methodical delineations of TIL-Bs within LUAD are scarce, and the precise molecular pathways through which TILBs modulate the efficacy of immunotherapy remain to be elucidated. The research employed single-cell RNA sequencing from the GSE117570 dataset to pinpoint markers linked to TILBs. A comprehensive machine learning method, utilizing ten distinct algorithms, facilitated the creation of a TILBs-related index (BRI) encompassing the TCGA, GSE31210, and GSE72094 datasets. BRI independently served as a risk determinant in LUAD, evidencing strong and dependable capability in forecasting total survival rates. In the datasets containing outcomes of immunotherapy patients (GSE91061 and GSE126044), we found that patients with lower BRI were more likely to respond to immunotherapy. Furthermore, lower BRI were linked to reduced IC50 values in standard chemotherapy and targeted therapy medications, in contrast to higher BRI. Additionally, the BRI-based survival prediction nomogram demonstrated significant promise for clinical application in predicting the 1-, 3-, and 5-year total survival rates among LUAD patients.
Keywords: lung cancer, Immunotherapy, machine learning, TCGA, B-cell, Tumor Microenvironment
Received: 07 Nov 2024; Accepted: 03 Mar 2025.
Copyright: © 2025 Fang, Yu, Wang, Liu, Lv, Jin, Han, Zhou and Wang. This is an open-access article distributed under the terms of the Creative Commons Attribution License (CC BY). The use, distribution or reproduction in other forums is permitted, provided the original author(s) or licensor are credited and that the original publication in this journal is cited, in accordance with accepted academic practice. No use, distribution or reproduction is permitted which does not comply with these terms.
* Correspondence:
Jiale Fang, Southern University of Science and Technology, Shenzhen, China
Yukun Wang, Southern University of Science and Technology, Shenzhen, China
Disclaimer: All claims expressed in this article are solely those of the authors and do not necessarily represent those of their affiliated organizations, or those of the publisher, the editors and the reviewers. Any product that may be evaluated in this article or claim that may be made by its manufacturer is not guaranteed or endorsed by the publisher.
Research integrity at Frontiers
Learn more about the work of our research integrity team to safeguard the quality of each article we publish.