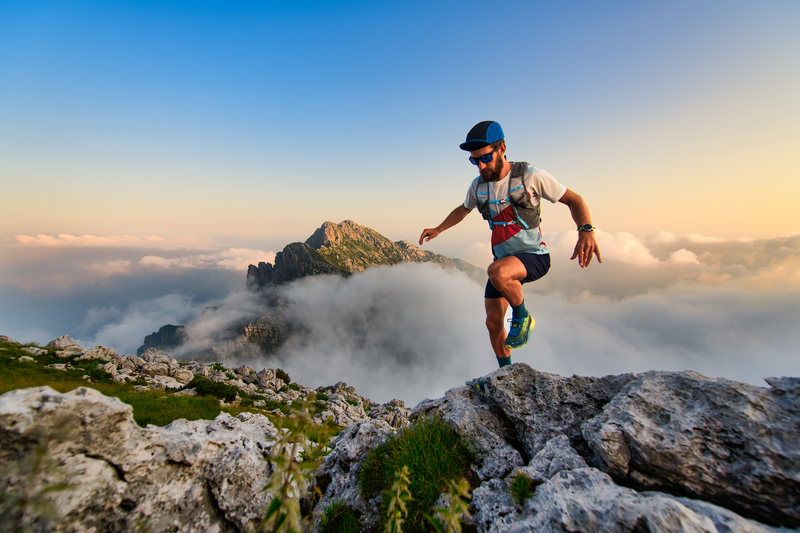
94% of researchers rate our articles as excellent or good
Learn more about the work of our research integrity team to safeguard the quality of each article we publish.
Find out more
ORIGINAL RESEARCH article
Front. Immunol.
Sec. Cancer Immunity and Immunotherapy
Volume 16 - 2025 | doi: 10.3389/fimmu.2025.1521965
This article is part of the Research Topic Integrating Molecular Mechanisms, Immunotherapy, and Drug Sensitivity in Cancer Immunology and Oncology View all 20 articles
The final, formatted version of the article will be published soon.
You have multiple emails registered with Frontiers:
Please enter your email address:
If you already have an account, please login
You don't have a Frontiers account ? You can register here
Background: The SUSD3 protein, marked by the Sushi domain, plays a key role in cancer progression, with its expression linked to tumor advancement and patient prognosis. Altered SUSD3 levels could serve as a predictive biomarker for cancer progression. Recognized as a novel susceptibility marker, SUSD3 presents a promising target for antibody-based therapies, offering a potential approach for the prevention, diagnosis, and treatment of breast cancer.Methods: Using the HPA and GeneMANIA platforms, the distribution of SUSD3 protein across tissues was analyzed, while expression levels in tumor and healthy tissues were compared using The Cancer Genome Atlas data. The TISCH and STOmics DB databases facilitated the mapping of SUSD3 expression in different cell types and its spatial relationship with cancer markers. Univariate Cox regression assessed the prognostic significance of SUSD3 expression in various cancers.Genomic alterations of SUSD3 were explored through the cBioPortal database. The potential of SUSD3 as a predictor of immunotherapy response was investigated using TIMER2.0, and GSEA/GSVA identified related biological pathways. Drugs targeting SUSD3 were identified through CellMiner, CTRP, and GDSC databases, complemented by molecular docking studies. In vitro experiments demonstrated that SUSD3 knockdown in breast cancer cell lines significantly reduced proliferation and migration. Results: SUSD3 expression variations in pan-cancer cohorts are closely linked to the prognosis of various malignancies. In the tumor microenvironment (TME), SUSD3 is predominantly expressed in monocytes/macrophages and CD4+ T cells. Research indicates a strong correlation between SUSD3 expression and key cancer immunotherapy biomarkers, immune cell infiltration, and immunomodulatory factors. To explore its immune regulatory role, StromalScore, ImmuneScore, ESTIMATE, and Immune Infiltration metrics were employed. Molecular docking studies revealed that selumetinib inhibits tumor cell proliferation. Finally, SUSD3 knockdown reduced cancer cell proliferation and migration. Conclusion: These findings provide valuable insights and establish a foundation for further exploration of SUSD3's role in pan-carcinomas. Additionally, they offer novel perspectives and potential targets for the development of innovative therapeutic strategies in cancer treatment.
Keywords: SUSD3, Pan-cancer, prognostic biomarker, Immune-related factors, Immunotherapy
Received: 03 Nov 2024; Accepted: 17 Feb 2025.
Copyright: © 2025 Zhong, Mao, Peng, Li, Xie, Xia, Chen, Zhang, Sun and Wang. This is an open-access article distributed under the terms of the Creative Commons Attribution License (CC BY). The use, distribution or reproduction in other forums is permitted, provided the original author(s) or licensor are credited and that the original publication in this journal is cited, in accordance with accepted academic practice. No use, distribution or reproduction is permitted which does not comply with these terms.
* Correspondence:
Shasha Zhang, Key Laboratory of Systems Biomedicine, Ministry of Education, Shanghai Center for Systems Biomedicine, Shanghai Jiao Tong University, Shanghai, 200240, China
Aijun Sun, Department of Laboratory Medicine, Affiliated Hospital of Xuzhou Medical University, Xuzhou, Jiangsu Province, China
Shiyan Wang, Huaiyin Institute of Technology, Huaiyin, China
Disclaimer: All claims expressed in this article are solely those of the authors and do not necessarily represent those of their affiliated organizations, or those of the publisher, the editors and the reviewers. Any product that may be evaluated in this article or claim that may be made by its manufacturer is not guaranteed or endorsed by the publisher.
Research integrity at Frontiers
Learn more about the work of our research integrity team to safeguard the quality of each article we publish.