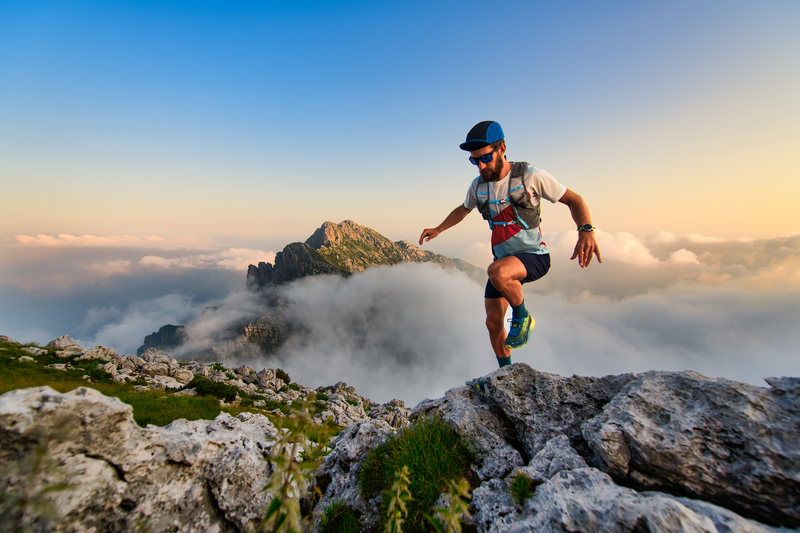
94% of researchers rate our articles as excellent or good
Learn more about the work of our research integrity team to safeguard the quality of each article we publish.
Find out more
ORIGINAL RESEARCH article
Front. Immunol.
Sec. Cancer Immunity and Immunotherapy
Volume 16 - 2025 | doi: 10.3389/fimmu.2025.1519766
This article is part of the Research Topic Clinical Implementation of Precision Oncology Data to Direct Individualized and Immunotherapy-Based Treatment Strategies View all 16 articles
The final, formatted version of the article will be published soon.
You have multiple emails registered with Frontiers:
Please enter your email address:
If you already have an account, please login
You don't have a Frontiers account ? You can register here
Purpose: This study aimed to create a nomogram model to predict the spread through air spaces (STAS) in patients diagnosed with stage IA lung adenocarcinoma, utilizing a substantial sample size alongside a blend of clinical and imaging features. This model serves as a valuable reference for the preoperative planning process in these patients.Materials and methods: A total of 1244 individuals were included in the study. Individuals who received surgical intervention between January 2022 and May 2023 were categorized into a training cohort (n=950), whereas those treated from June 2023 to October 2023 were placed in a validation cohort (n=294). Data from clinical assessments and CT imaging were gathered from all participants. In the training cohort, analyses employing both multivariate and univariate logistic regression were performed to discern significant clinical and CT characteristics. The identified features were subsequently employed to develop a nomogram prediction model. The evaluation of the model's discrimination, calibration, and clinical utility was conducted in both cohorts.Results: In the training cohort, multivariate logistic regression analysis revealed several independent risk factors associated with invasive adenocarcinoma: maximum diameter (OR=2.459, 95%CI: 1.833-3.298), nodule type (OR=4.024, 95%CI: 2.909-5.567), pleura traction sign (OR=2.031, 95%CI: 1.394-2.961), vascular convergence sign (OR=3.700, 95%CI: 1.668-8.210), and CEA (OR=1.942, 95%CI: 1.302-2.899). A nomogram model was constructed utilizing these factors to forecast the occurrence of STAS in stage IA lung adenocarcinoma. The Area Under the Curve (AUC) measured 0.835 (95% CI: 0.808–0.862) in the training cohort and 0.830 (95% CI: 0.782–0.878) in the validation cohort. The internal validation conducted through the bootstrap method yielded an AUC of 0.846 (95% CI: 0.818-0.881), demonstrating a robust capacity for discrimination. The Hosmer–Lemeshow goodness-of-fit test confirmed a satisfactory model fit in both groups (P > 0.05). Additionally, the calibration curve and decision analysis curve demonstrated high calibration and clinical applicability of the model in both cohorts.Conclusion: By integrating clinical and CT imaging characteristics, a nomogram model was developed to predict the occurrence of STAS, demonstrating robust predictive performance and providing valuable support for decision-making in patients with stage IA lung adenocarcinoma.
Keywords: Clinical feature, radiologic characteristic, Lung Adenocarcinoma, STAS, nomogram
Received: 30 Oct 2024; Accepted: 12 Mar 2025.
Copyright: © 2025 Yang, Hu, Li, Zhou, Qiubo, ZHAO, Duan, Li, Luo, Jiang, Yang, Zhao, Huang and Ye. This is an open-access article distributed under the terms of the Creative Commons Attribution License (CC BY). The use, distribution or reproduction in other forums is permitted, provided the original author(s) or licensor are credited and that the original publication in this journal is cited, in accordance with accepted academic practice. No use, distribution or reproduction is permitted which does not comply with these terms.
* Correspondence:
Yantao Yang, Yunnan Cancer Hospital, Kunming, China
Lianhua Ye, Yunnan Cancer Hospital, Kunming, China
Disclaimer: All claims expressed in this article are solely those of the authors and do not necessarily represent those of their affiliated organizations, or those of the publisher, the editors and the reviewers. Any product that may be evaluated in this article or claim that may be made by its manufacturer is not guaranteed or endorsed by the publisher.
Research integrity at Frontiers
Learn more about the work of our research integrity team to safeguard the quality of each article we publish.