- 1Laboratory of Regenerative Medicine, Medical University of Warsaw, Warsaw, Poland
- 2Stem Cell Institute at James Graham Brown Cancer Center, University of Louisville, Louisville, KY, United States
Background: The complement system expressed intracellularly and known as complosome has been indicated as a trigger in the regulation of lymphocyte functioning. The expression of its genes was confirmed also in several types of human bone marrow-derived stem cells: mononuclear cells (MNCs), very small embryonic-like stem cells (VSELs), hematopoietic stem/progenitor cells (HSPCs), endothelial progenitors (EPCs) and mesenchymal stem cells (MSCs). In our previous studies, we demonstrated the expression of complosome proteins including C3, C5, C3aR, and cathepsin L in purified HSPCs. However, there is still a lack of results showing the expression of complosome system elements and other immunity-related proteins in human HSPCs at the level of single cell resolution.
Methods: We employed scRNA-seq to investigate comprehensively the expression of genes connected with immunity, in two populations of human HSPCs: CD34+Lin-CD45+ and CD133+Lin-CD45+, with the division to subpopulations. We focused on genes coding complosome elements, selected cytokines, and genes related to antigen presentation as well as related to immune regulation.
Results: We observed the differences in the expression of several genes e.g. C3AR1 and C5AR1 between two populations of HSPCs: CD34+LinCD45+ and CD133+Lin-CD45+ resulting from their heterogeneous nature. However, in both kinds of HSPCs, we observed similar cell subpopulations expressing genes (e.g. NLRP3 and IL-1β) at the same level, which suggests the presence of cells performing similar functions connected with the activation of inflammatory processes contributing to the body's defense against infections.
Discussion: To our best knowledge, it is the first time that expression of complosome elements was studied in HSPCs at the single cell resolution with the use of single cell sequencing. Thus, our data sheds new light on complosome as a novel regulator of hematopoiesis that involves intracrine activation of the C5a-C5aR-Nlrp3 inflammasome axis.
1 Introduction
Hematopoiesis and the immune system originate from a common hematopoietic/lymphopoietic stem cell. This close developmental relationship explains that cells belonging to both lineages often share the same receptors and respond to similar factors (1–7). Gene expression analysis performed on bulk RNA supported this developmental origin, explained by the common goal of both lineages being involved in tissue homeostasis, fighting invading pathogens, and promoting tissue repair (8–12). The complement, a crucial innate and adaptive immunity element, is a complex multiprotein system responsible for inflammatory processes and other biological effects (13–16). Initially, it was considered a serum-effective system in which proteins were synthesized in the liver and released into circulation (17, 18). Recent data demonstrated the presence of complement elements expressed intracellularly, which is crucial for the normal functioning of lymphocytes (19–21). Furthermore, the expression of its components was also detected in several types of human bone marrow-derived stem cells, including mononuclear cells (MNCs), very small embryonic-like stem cells (VSELs), hematopoietic stem/progenitor cells (HSPCs), endothelial progenitors (EPCs) and mesenchymal stem cells (MSCs) (22). Our previous studies demonstrated the expression of functional complosome proteins, including C3, C5, C3aR, and cathepsin L in purified human and murine HSPCs (18, 22). It has also been reported that HSPCs express several receptors that protect them from potential damage by circulating in peripheral blood (PB) activated complement proteins (23), some members of the toll-like receptor family (TLRs) (24, 25), and intracellular pattern recognition receptors (PRRs) (26, 27). Pattern recognition receptors (PRRs) are germline-encoded sensors that detect pathogen-associated (PAMPs) or damage-associated molecular patterns (DAMPs). They are highly expressed in innate immune cells, such as dendritic cells, macrophages, monocytes, and epithelial cells Some elements of innate immunity, such as intracellular PRR, have been implicated, for example, in the specification of HSPCs in mammals during embryogenesis (28) and being involved in the early stages of hematopoiesis in the zebrafish (29, 30). Furthermore, bone marrow (BM) cells from TLR4−/−, TLR9−/−, and MyD88−/− mice show a repopulating advantage over wild-type cells in lethally irradiated recipients (28), suggesting that TLR signaling supports HSPC maintenance.
To our best knowledge, there is still a lack of information about the expression and functioning of the complosome elements, obtained from single-cell sequencing experiments. This method (scRNA-seq) is becoming an increasingly widely used tool in biological and biomedical research and has advanced the understanding of a range of biological processes and molecular mechanisms occurring in cells. It potentially may have a clinical application and may be valuable in new diagnostic and therapeutic strategies (31). In the case of hematopoietic stem cells, scRNA-seq allowed to decode both human (32) and mice (33) hematopoiesis. Furthermore, the first hematopoietic stem cells in human embryo were traced by scRNA-seq (34). In our previous scRNA-seq experiment (35), we identified several HSPCs subpopulations (14 clusters for both CD34+Lin-CD45+ and CD133+Lin-CD45+, with their basic division into 2 main cell groups. One, with confirmed expression of expression of two stem cell markers: CD34 and PROM1 (CD133). We call them primitive or quiescent clusters. And another one, with confirmed expression of lymphocyte (T and B), monocytes, macrophages, NK cells and granulocytes markers (e. g. CD2, CD3, CD19, CD14, FCGR3A, CD68). We call them ‘fate decision’ clusters (35). In the current study, we employed scRNA-seq and untargeted proteomic analysis using mass spectrometry to investigate the expression of genes immunity in two populations of purified human umbilical cord blood (UCB) HSPCs: CD34+Lin-CD45+ and CD133+Lin-CD45+, paying special attention to track the differences between primitive and ‘fate decision’ clusters. We analyzed our mRNA expression data sets employing the Uniform Manifold Approximation and Projection for Dimension Reduction (UMAP) technique, which allows us to visualize separate cell clusters (36, 37). We focused on genes encoding proteins involved in immune responses, encoding complosome elements, and selecting cytokines. To our knowledge, it is the first time that the expression of all these genes was studied in HSPCs at the single-cell resolution using single-cell sequencing. We observed some similarities and differences in gene expression between CD34+Lin-CD45+ and CD133+Lin-CD45+ cells. Moreover, scRNA-seq results revealed significant differences from previously published data using bulk mRNA analyses. Finally, the expression of some of these genes was subsequently evaluated by proteomic analysis.
2 Materials and methods
2.1 Isolation of human CD34+ and CD133+ HSPCs
Human umbilical cord blood (hUCB) was obtained from a healthy newborn delivered at the Department of Obstetrics and Gynecology, Medical University of Warsaw (Warsaw Bioethics Committee permission number KB/50/2022). Blood was diluted with phosphate-buffered saline (PBS) and carefully layered over Ficoll-Paque (GE Healthcare, Chicago, IL, USA). Next, it was centrifuged for 30 min at 400x g at 4°C. The mononuclear cells (MNCs) were collected, washed, and stained with the following antibodies: hematopoietic lineage markers (Lin) cocktail of antibodies, each FITC-conjugated: CD235a (clone GA-R2 [HIR2]), anti-CD2 (clone RPA-2.10), anti-CD3 (clone UCHT1), anti-CD14 (clone M5E2), anti-CD16 (clone 3G8), anti-CD19 (clone HIB19), anti-CD24 (clone ML5), anti-CD56 (clone NCAM16.2) and anti-CD66b (clone G10F5) (all BD Biosciences, San Jose, CA, USA); PE-Cy7-conjugated anti-CD45 (clone HI30, BioLegend, San Diego, CA, USA), PE-conjugated anti-CD34 (clone 581, BioLegend, San Diego, CA, USA) and APC-conjugated anti-CD133 (clone CD133, MiltenyiBiotec, Gladbach, Germany). Cells were then stained in the dark at 4°C for 30 min. After incubation, they were centrifugated and resuspended in RPMI-1640 medium containing 2% fetal bovine serum (FBS, Corning Inc, Corning, NY, USA). Finally, cells were sorted according to the strategy described in numerous reports (38–40). Briefly, small events (2–15 μm in size) were included in the “lymphocyte-like” gate (P1) and then further analyzed for the expression of the Lin marker. Lin negative events were gated and subsequently analyzed for the expression of CD45 and CD34 or CD133 antigens. Populations of CD34+ HSPCs (CD34+Lin−CD45+) and CD133+ HSPCs (CD133+Lin−CD45+) were obtained on the MoFlo Astrios EQ cell sorter (Beckman Coulter, Brea, CA, USA).
2.2 Single-cell sequencing
After sorting, cells were directly proceeded using Chromium X Controller (10X Genomics, USA) and Chromium Next GEM Chip G Single Cell Kit (10X Genomics, USA). Chromium Next GEM Single Cell 3’ GEM, Library & Gel Bead Kit v3.1, and Single Index Kit T Set A (10X Genomics, USA) were used for library preparation according to manufacturer’s guidelines. Libraries were then pooled and run on Illumina NextSeq 1000/2000 (Illumina, San Diego, CA, USA) in P2 flow cell chemistry (200 cycles) with paired-end sequencing mode (read 1–28 bp, read 2–90 bp, index 1 – 10 cycles, index 2 – 10 cycles), assuming 25,000 reads per single cell.
2.3 Bioinformatic analysis
Downstream analysis was performed using Seurat (version 5.0.1), preceded by the 10X Genomics Cell Ranger pipelines (CellRanger version 7.2.0, 10x Genomics, USA) (41, 42). Raw sequencing files (BCL files) were demultiplexed and converted to fastq files using the bcl2fastq (version v2.20.0.422) within the 10X Genomics Cell Ranger mkfastq pipeline (43, 44). Then, the Cell Ranger count pipeline was used. Cells with less than 200 and more than 2500 transcripts and those with more than 5% of mitochondria-related transcripts were excluded from the analysis. Sequencing reads were mapped to a human genome GRCh38 (version 2020-A) acquired from the 10×Genomics website (https://www.10xgenomics.com/support/software/cell-ranger/downloads#reference-downloads). Gene expression measurements for each cell were normalized, and the normalized values were log-transformed (“LogNormalize” method) and then reduced to the first 2000 most highly variable genes. Non-linear dimensional reduction to visualize clusters was performed using uniform manifold approximation and d projection (UMAP) implemented in Seurat (version 5.0.1.). Cell clusters were recognized based on differentially expressed genes, both positive (up-regulated genes) and negative (down-regulated genes) markers, using an adjusted p-value <0.05 and a log2FC >1. However, for this publication, we focused only on positive ones.
2.4 Data visualization
Data visualization was done using R (R Core Team) with the ggplot2 R package (version 3.4.4) (45). We selected hematopoietic lineage differentiation cell markers (CD2, CD3, CD4, CD11b (ITGAM), CD14, CD16 (FCGR3A), CD19, CD34, CD41 (ITGA2B), CD45 (PTPRC), CD66b (CEACAM8), CD68, GYPA, PROM1 (CD133), CD117 (c-KIT) to determine their expression in CD34+lin-CD45+ and CD133+lin-CD45+ and confirm the hematopoietic character of the studied cells. Furthermore, we selected several genes related to the complosome system and immunity. They were as follows: TLR1, TLR2, TLR3, TLR4, TLR5, TLR6, TLR7, TLR8, TLR9, TLR10, NOD1, NOD2, NOD3, NOD4, NOD5, NLRC4, CIITA, NAIP, NLRP1, NLRP2, NLRP3, NLRP4, NLRP5, NLRP6, NLRP7, NLRP8, NLRP9, NLRP10, NLRP11, NLRP12, NLRP13, NLRP14, C1QA, C1QB, C1QC, C1R, C1S, C2, C3, C4, C5, C6, C7, C8, C9, CFB, CFP, C3AR1, C5AR1, C5AR2, CTSL, CFH, CFI, CD46, CD55, CD59, C1NH, CFHR1, CFHR2, CFHR3, CFHR4, CFHR5, CASP1, IL1B, IL18, PYCARD, MEFV, AIM2, MDAS, DDX58.
2.5 Data availability
The data sets supporting this article’s results are available in the Sequence Read Archive (SRA) repository (https://www.ncbi.nlm.nih.gov/sra) and assigned a unique persistent identifier: PRJNA1128409 (https://www.ncbi.nlm.nih.gov/sra/PRJNA1128409).
2.6 Mass spectrometry
MoFlow-isolated CD133+lin-CD45+ and CD34+lin-CD45+ HSPCs were lysed in 50 μL of RIPA buffer. Samples were incubated for 60 min at 96°C and then sonicated for 90 min in a water bath. Samples were precipitated with the use of ice cold (−20°C) Acetonitrile (ACN, Merck, Darmstadt, Germany) in a volume 1:4 ratio and incubated for 120 min in (−20°C). After precipitation, samples were centrifuged (30 min, −9°C, 18000 × g), and the supernatant was removed. The protein pellet was dissolved in 40 mM ammonium bicarbonate (Merck, Darmstadt, Germany). Reduction and alkylation were performed using 500 mM DTT (in a final concentration of 20 mM) and 1 M iodoacetamide (IAA, in a final concentration of 40 mM). Proteins were in-solution digested for 16 h at 37°C using Trypsin Gold (Promega, Madison, WI, United States).
Liquid chromatography-mass spectrometry (LC-MS) analysis was carried out with the use of nano-UHPLC (nanoElute, Bruker, Billerica, MA, United States) coupled by Captive Spray (Bruker, Billerica, MA, United States) to ESI-Q-TOF mass spectrometer (Compact, Bruker, Billerica, MA, United States). A two-column separation method was used, that is, pre-column (300 μm × 5 mm, C18 PepMap 100, 5 μm, 100 Å, Thermo Scientific, Waltham, MA, United States) and Bruker fifteen separation column (75 μm × 150 mm, C18 1.6 μm) in gradient 2% B to 55% B in 45 min with the 300 nL/min flow rate.
2.7 Proteomic data analysis
The collected spectra were calibrated (lock mass calibrant) and analyzed using Data Analysis software (Bruker). They were then identified in ProteinScape (Bruker) using the MASCOT server. Proteins were identified using the online SwissProt database, and their annotation and biological significance were determined using UniProt.org and KEGG. All analyses were performed in the R version [4.3.3]. The following R packages were employed for data processing, statistical analysis, and visualization: tidyverse (46) for data manipulation and wrangling, readxl (47) for importing Excel files, dplyr (48) and purrr (49) for functional programming and data cleaning, and writexl (50) for exporting processed results to Excel.
3 Results
3.1 Cell subpopulations within CD34+Lin-CD45+ and CD133+Lin-CD45+
We identified several cell types and cell subpopulations in CD34+Lin-CD45+ (Figure 1A) and CD133+Lin-CD45+ (Figure 1B) cells, as it was previously published (35). Cluster 0 and 1 of CD34+Lin-CD45+ cells and clusters 1 and 4 of CD133+Lin-CD45+ cells were identified to express transcripts of CD34, CD133 (PROM1), and c-kit genes, corresponding to the most primitive cells evaluated in our study. Additionally, we also identified clusters 6 and 7 of CD34+Lin-CD45+ (Figure 1A) as cells expressing CD34, CD133dim, and c-kitdim and cluster 11 of CD133+Lin-CD45+ (Figure 1B) as primitive cells expressing CD34, CD133 and c-kitdim. Furthermore, both HSCPs populations (Figures 1A, B) contain cell clusters with confirmed expression of myeloid (CD14, CD68) and lymphoid (CD2, CD4) progenitors. We call them “fate decision” clusters.
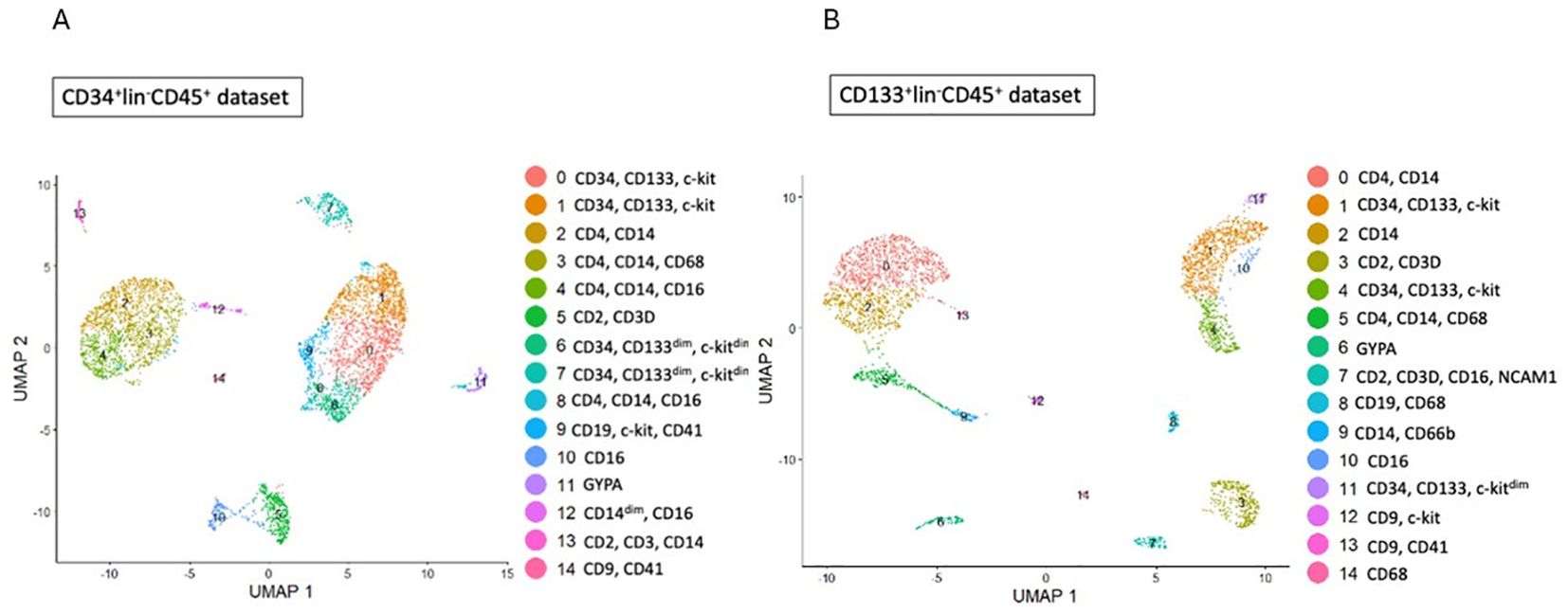
Figure 1. Identification of subpopulations in studied samples of HSCs. (A, B) - CD34+lin-CD45+ (A), CD133+lin-CD45+ (B) visualized by UMAP method. The datasets are first analysed without integration. The resulting clusters are defined both by cell type Uniform manifold approximation and projection (UMAP) plot.
3.2 Expression of toll-like receptors
Our scRNA-seq data (Figure 2) revealed the expression of transcripts of TLR1 and TLR 2 receptors in primitive clusters (0 and 1 of CD34+lin-CD45+ and 1 of CD133+lin-CD45+), as well as fate decision clusters (2, 3 and 4 of CD34+lin-CD45+; and 0, 2 and 5 of CD133+lin-CD45+) Additionally, TLR4, TLR5, TLR6, and TLR8, were also expressed on cells in clusters enriched of CD2, CD4, CD14 and CD68 mRNA in both datasets (Figures 2A, B).
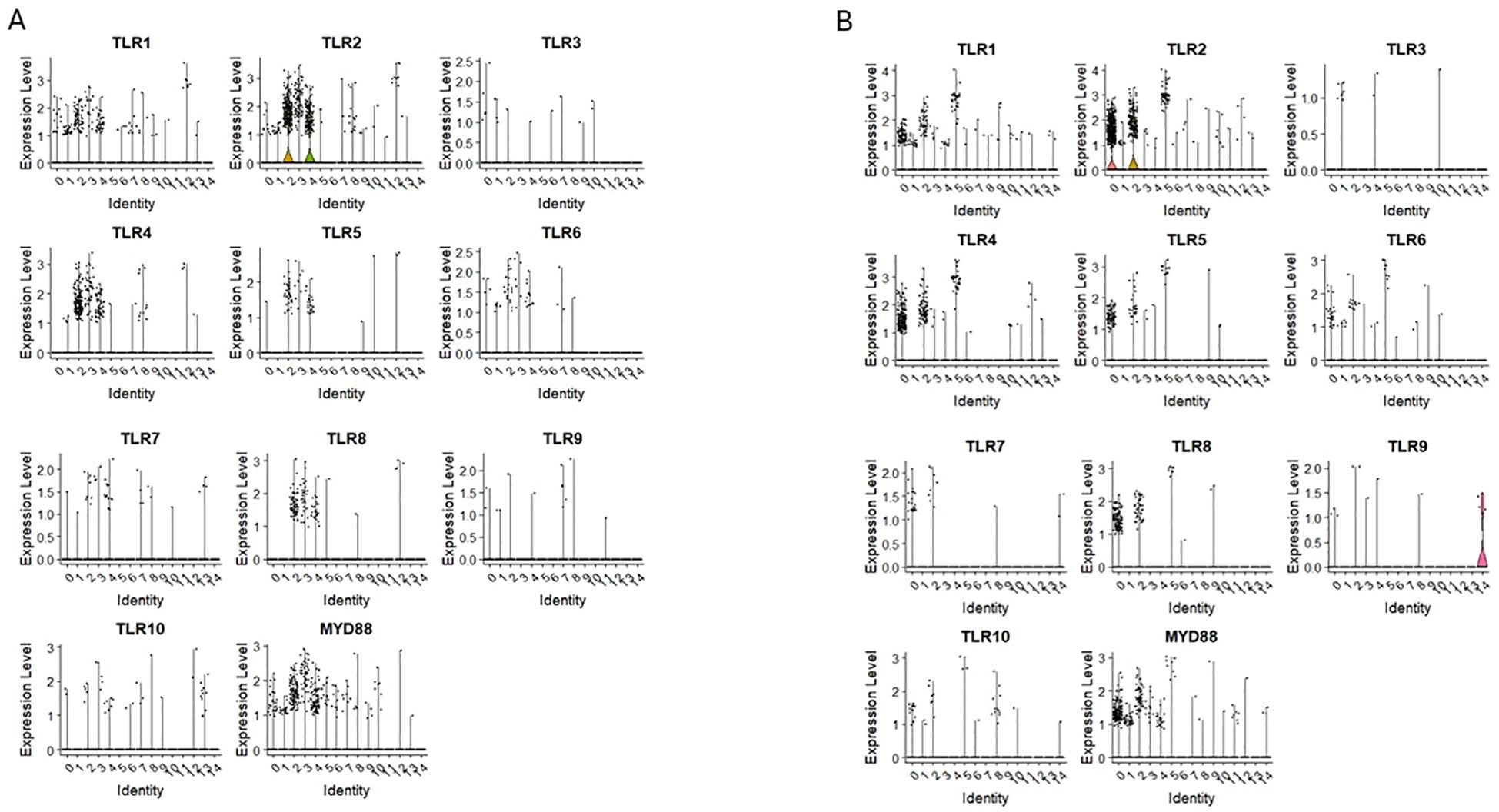
Figure 2. The expression of Toll-like receptor (TLRs) genes in CD34+lin-CD45+ (A) and CD133+lin-CD45+ (B) cells with the division to clusters. Violin plots shows differential expression of TLR1, TLR2, TLR3, TLR4, TLR5, TLR6, TLR7, TLR8, TLR9, TLR10 and MYD88 in different subpopulations of both datasets.
3.3 Expression of NOD-like receptors
Next, we evaluated mRNA expression for NOD-like receptors in our scRNA-seq data sets:
-CARD domain – containing NODs/NLRCs
The nucleotide-binding oligomerization domain-like receptors, or NOD-like receptors (NLRs), are intracellular sensors known as pattern recognition receptors (51, 52). We detected transcripts for NOD1/NLRC1, NOD3/NLRC3, NOD4/NLRC5, NOD5/NLRZ1, CIITA/NLRA and NAIP in clusters 0 and 1 for CD34+Lin-CD45+ (Figure 3A) and clusters 1 and 4 for CD133+Lin-CD45+ (Figure 3B) enriched for mRNA for CD34, CD133, and c-kit (primitive clusters). The mRNA for these receptors was also expressed in other cell clusters with confirmed expression of mRNA for lymphocytic precursors.
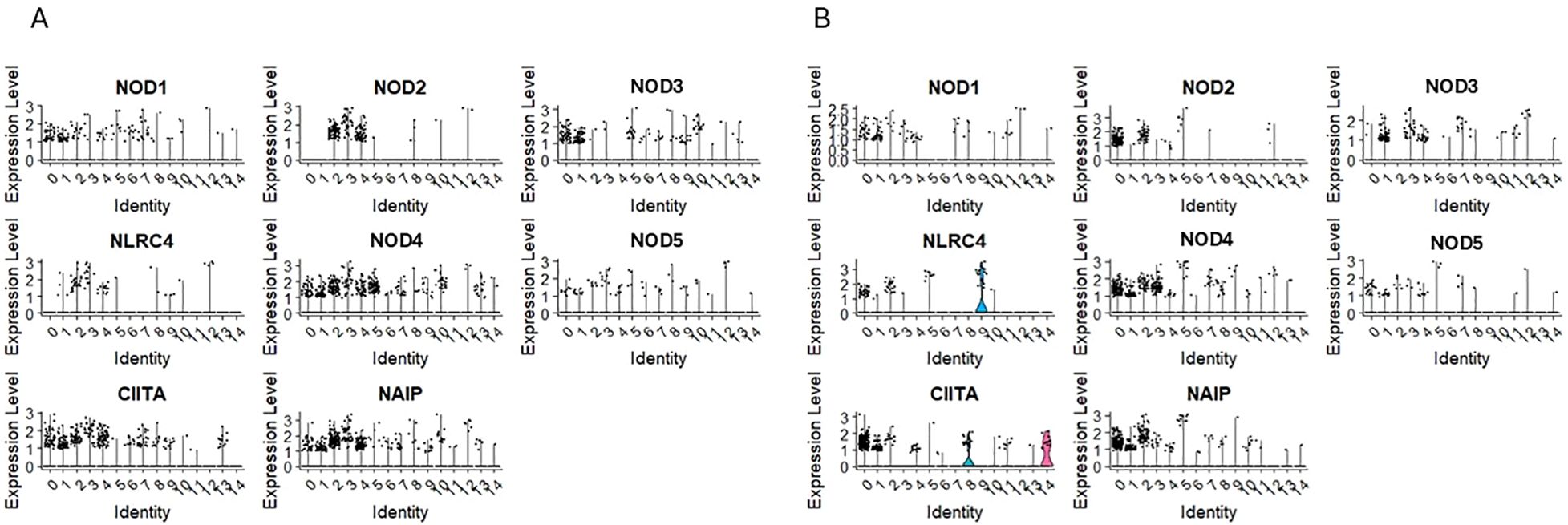
Figure 3. The expression of CARD domain–containing NOD-like receptor (NOD) genes in CD34+lin-CD45+ (A) and CD133+lin-CD45+ (B) cells with the division to clusters. Violin plots show differential expression of NOD1, NOD2, NOD3, NLRC4, NOD4, NOD5, CIITA, and NAIP in different subpopulations of both datasets.
-Pyrin domain – containing NODs/NLRPs
The expression of NLRP1, NLRP2, NLRP3, NLRP4/PAN2 was observed in clusters 0 and 1 of CD34+Lin-CD45+ (Figure 4A) and clusters 1 and 4 of CD133+Lin-CD45+ (Figure 4B), which are, as it was mentioned above the most primitive cell subpopulations of HPSCs (expressing CD34, CD133, and c-kit transcripts). Additionally, their expression was observed in “fate decision” subpopulations, with confirmed expression of CD4 and CD14 in both HSPCs populations. The same was found for NLRP12, which was also expressed in cell clusters expressing mRNA for lymphocytic precursors (CD4, CD14, CD68). Expression of NLRP5, NLRP10, NLRP11, and NLRP14 was not observed in both scRNA-seq datasets.
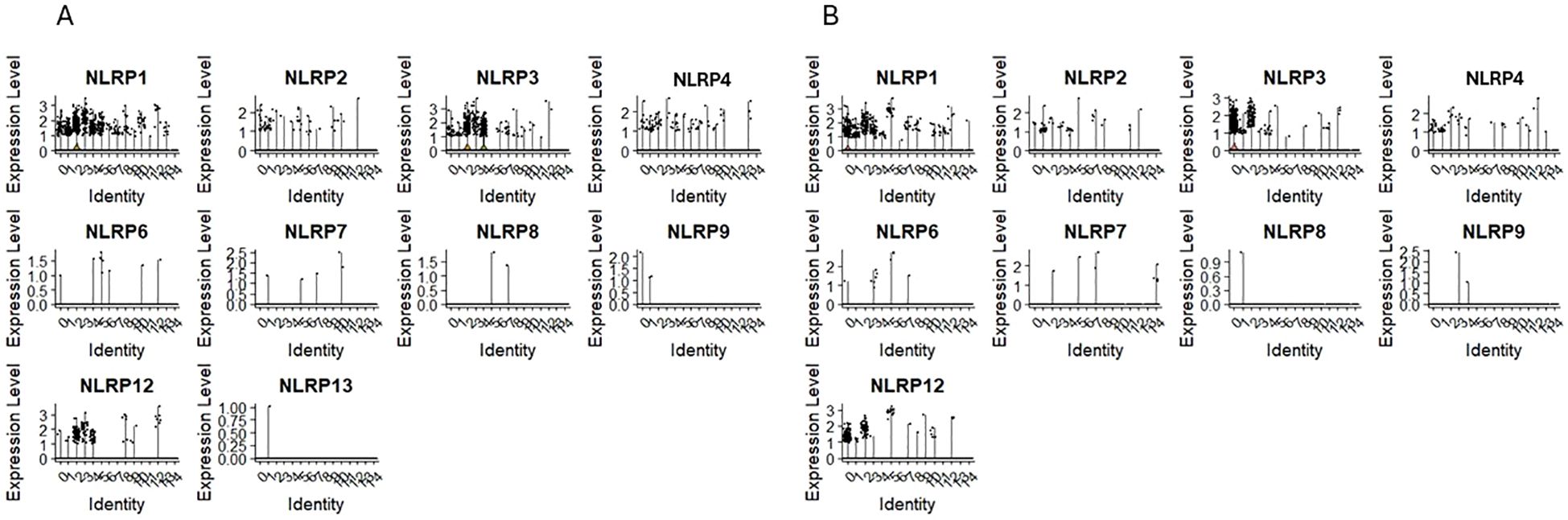
Figure 4. The expression of Pyrin domain–containing NOD-like receptor (NOD) genes in CD34+lin-CD45+ (A) and CD133+lin-CD45+ (B) cells with the division to clusters. Violin plots shows differential expression of NLRP1, NLRP2, NLRP3, NLRP4, NLRP6, NLRP7, NLRP8, NLRP9, NLRP12 and NLRP13 in different subpopulations of both datasets.
3.4 Expression of mRNA for RIG-1-like receptors and AIM2
We found the expression of RIG-1-like receptors MDAS (IFIHI) and DDX58 in clusters 0 and 1 of CD34+Lin-CD45+ (Figure 5A) and clusters 1 and 4 of CD133+Lin-CD45+ (Figure 5B).
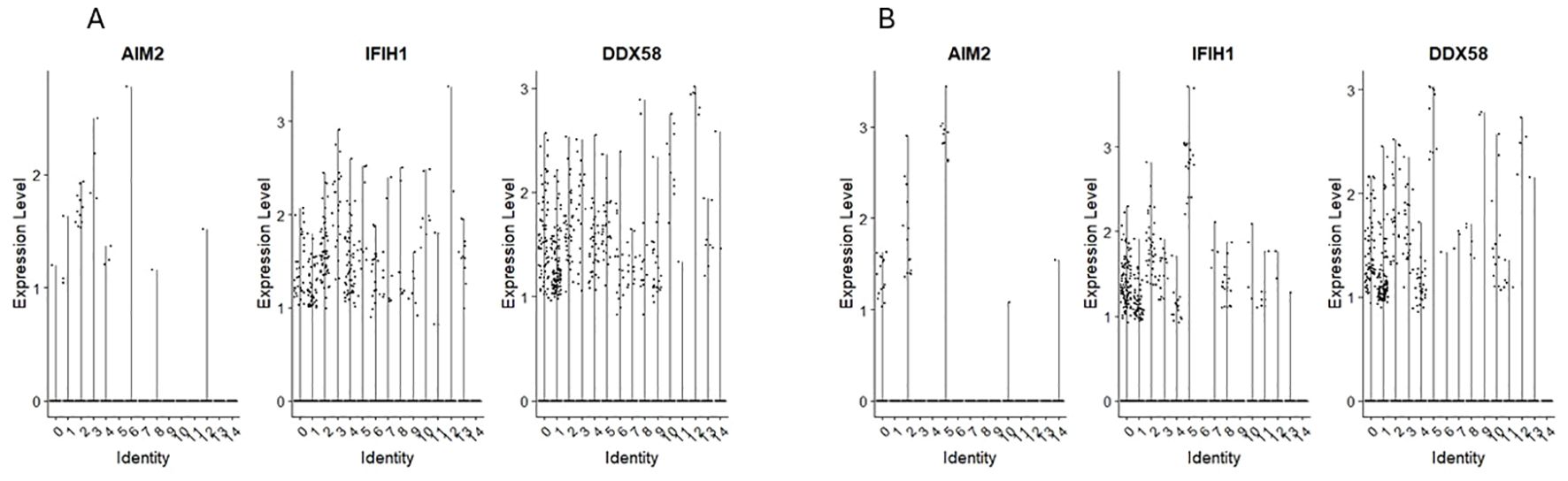
Figure 5. The expression of RIG-like receptors genes in CD34+lin-CD45+ (A) and CD133+lin-CD45+ (B) cells with the division to clusters. Violin plots show differential expression of AIM2, IFIH1, and RIG-1 in different subpopulations of both datasets.
AIM2 expression was found however, its level was very slight and observed only in single cells of both HSPCs populations.
3.5 Expression of mRNA for complement cascade/complosome
We found the expression of C3 and C5 in both HSPCs populations: CD34+lin-CD45+ (Figure 6A) and CD133+lin-CD45+ (Figure 6B) however it was on the slight level with just a few cells expressing mRNA of these genes. Interestingly, the expression of C3AR1, C5AR1, and C5AR2 (Figures 6A, B) was observed not in all cells of both HSPCs populations but in the subpopulations with confirmed expression of CD4 and CD14 markers, corresponding to ‘fate decision’ cells, where the mechanisms of immunological actions start to become active. Because the expression of receptor genes is observed only in this group of cells, we can assume that their later immunological potential comes from there. We do not see the expression of C3, C5, C3AR1, C5AR1, and C5AR2 (Figures 6A, B) in the quiescent subpopulation of HSPCs with a confirmed expression of CD34, CD144, and c-KIT. Interestingly, in both HSPCs populations: CD34+lin-CD45+ and CD133+lin-CD45+.
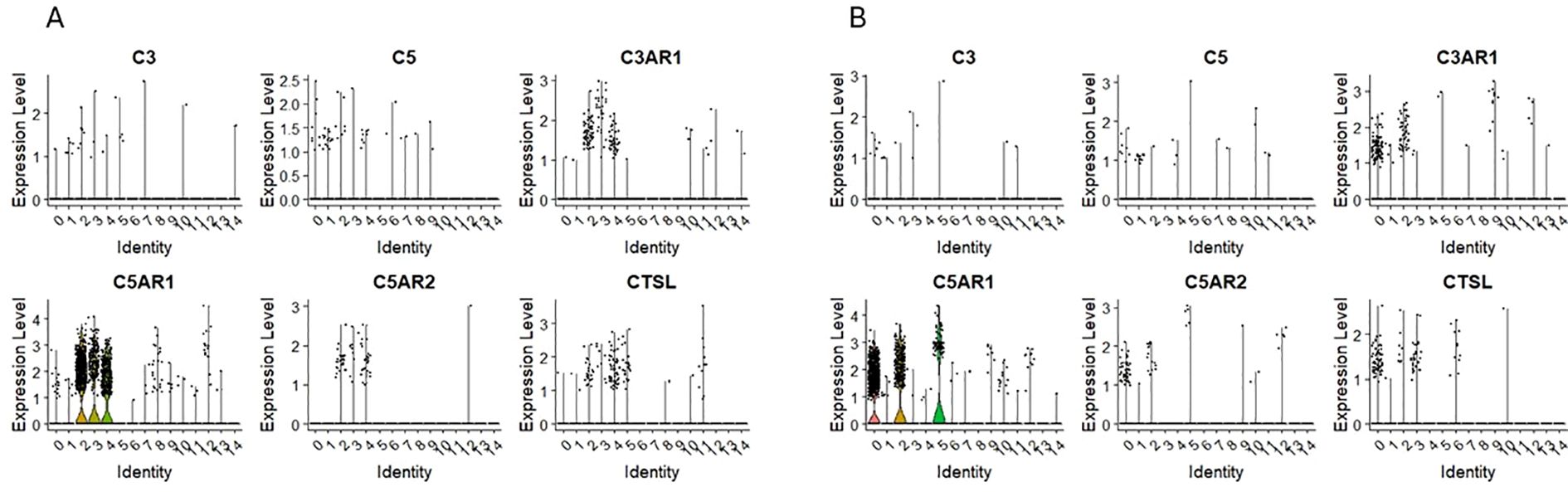
Figure 6. The expression of complement system genes in CD34+lin-CD45+ (A) and CD133+lin-CD45+ (B) cells with the division to clusters. Violin plots show differential expression of C3, C5, C3AR1, C5AR1, C5AR2, and CTSL in different subpopulations of both datasets.
3.6 Expression of mRNA for NLRP3 inflammasome components
Our scRNA-seq datasets revealed that mRNA for Nlrp3 inflammasome is expressed in clusters 0 and 1 for CD34+Lin-CD45+ and UMAP clusters 1 and 4 for CD133+Lin-CD45+ enriched for mRNA of HSPCs (Figure 4). In addition, Figures 7A, B show mRNA expression for crucial components of this pattern recognition receptor, including mRNA for IL1β, IL18, PYCARD, and CASP1 in the same clusters. The NOD-like receptor protein 3 (NLRP3) inflammasome is a protein complex that regulates innate immune responses by activating caspase-1 and the inflammatory cytokines interleukin (IL)-1β and IL-18 (53–55). We and others have demonstrated that Nlrp3 inflammasome is expressed in HSPCs and regulates the trafficking and metabolism of these cells (56–59).
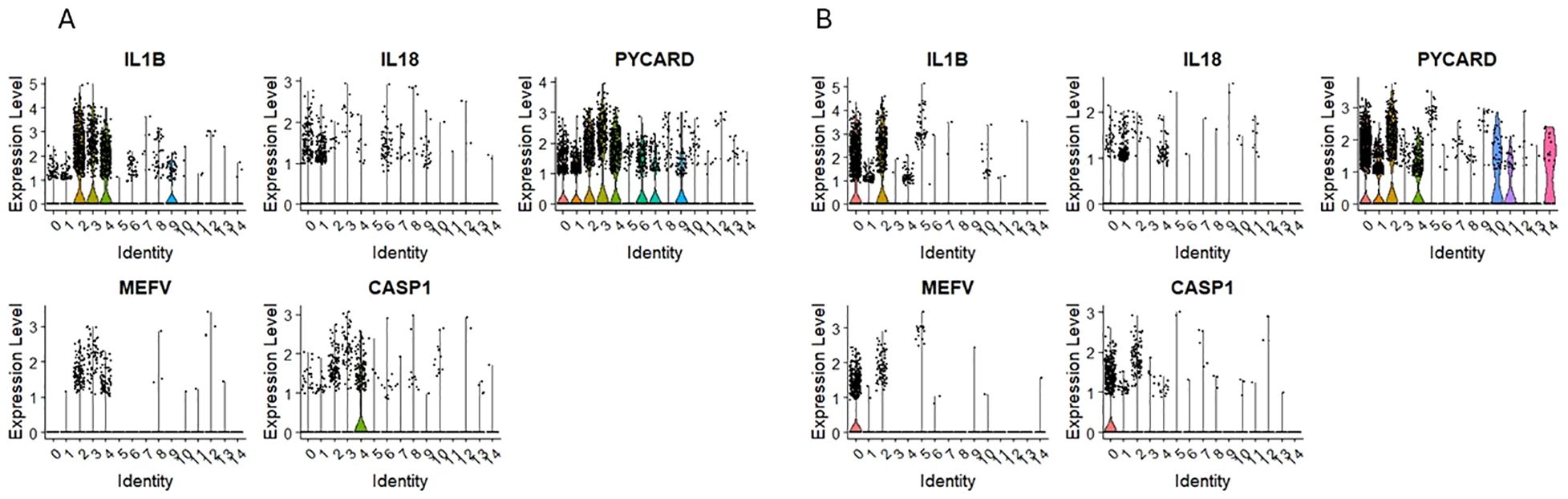
Figure 7. The expression of innate immunity genes in CD34+lin-CD45+ (A) and CD133+lin-CD45+ (B) cells with the division to clusters. Violin plots show differential expression of IL1B, IL18, PYCARD, MEFV, and CASP1 in different subpopulations of both datasets.
3.7 Expression of mRNA for complement regulatory proteins
Opposite to complement system genes, which expression was mostly observed in ‘fate decision’ HSPCs subpopulations; the expression of complosome regulatory elements was found in the primitive, quiescent subpopulations in both HSPCs datasets. Complement factor H (CFH) expression was found only in cells expressing CD34, CD133, and c-KIT in CD34+lin-CD45+ and CD133+lin-CD45+ cells (Figures 8A, B). Additionally, the expression of CD59 was found primarily on primitive subpopulations (Figures 8A, B); however, in the case of CD34+lin-CD45+, it was also found in cells expressing GYPA (Figure 8B).
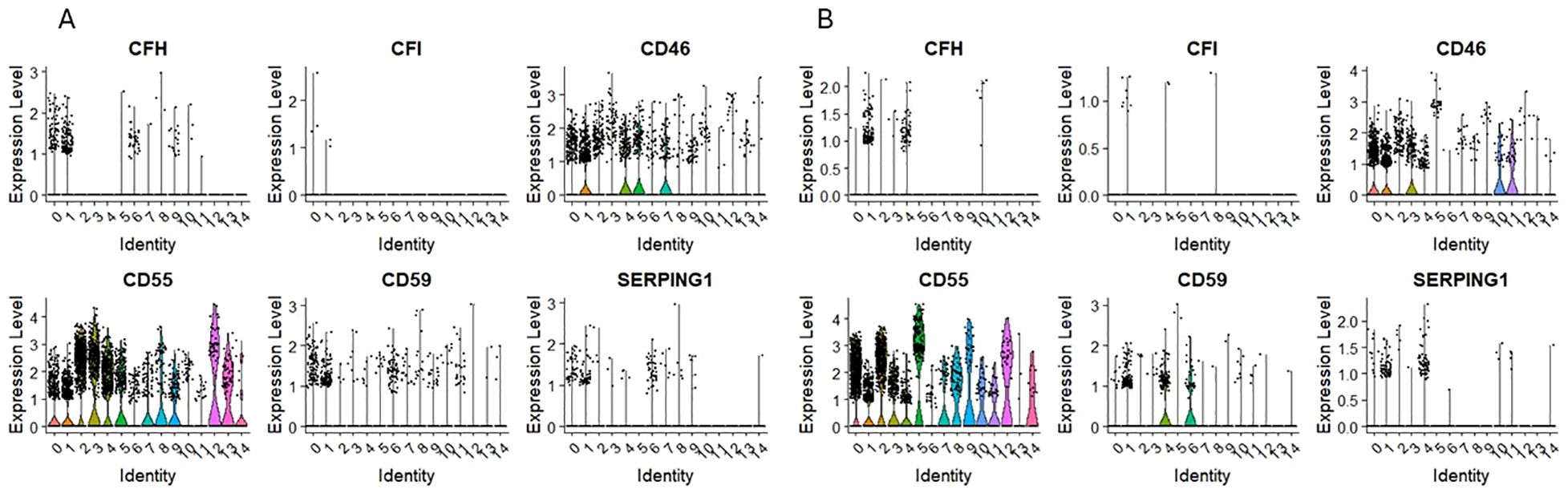
Figure 8. The expression of complement system regulatory genes in CD34+lin-CD45+ (A) and CD133+lin-CD45+ (B) cells with the division to clusters. Violin plots show differential expression of CFH, CFI, CD46, CD55, CD59, and SERPING1 in different subpopulations of both datasets.
3.8 Comparative analysis of proteins identified in HSPCs datasets
Using untargeted mass spectrometry for proteomic analysis, we identified 3161 and 4489 proteins for CD34+ and CD133+ HSPCs datasets (Figure 9A). Among those, 1257 proteins were common for both datasets. The highly enriched GO biological pathways and the number of proteins were involved in innate and adaptive immunity and inflammation (Figure 9B). Heatmap comparison of mean normalized intensities (Figure 9B) shows the comparative analysis of Toll-like receptors (TLR3, TLR4, TLR5) more abundant in CD133+lin-CD45+ dataset, proteins with pyrin (PYD) domain, that are most recognized for its role in signaling innate immunological responses, specifically in the formation of large protein complexes called inflammasomes, which enable the activation of caspase-1 by promoted proximity-mediated activation and the subsequent release of pro-inflammatory cytokines (60). We also detected the presence of AIM2 and ACS in our datasets, however a high abundance of AIM 2 was detected for CD34+lin-CD45+ HSCS, whereas higher mean normalized intensity was observed for ASC CD133+lin-CD45+ HSPCs.
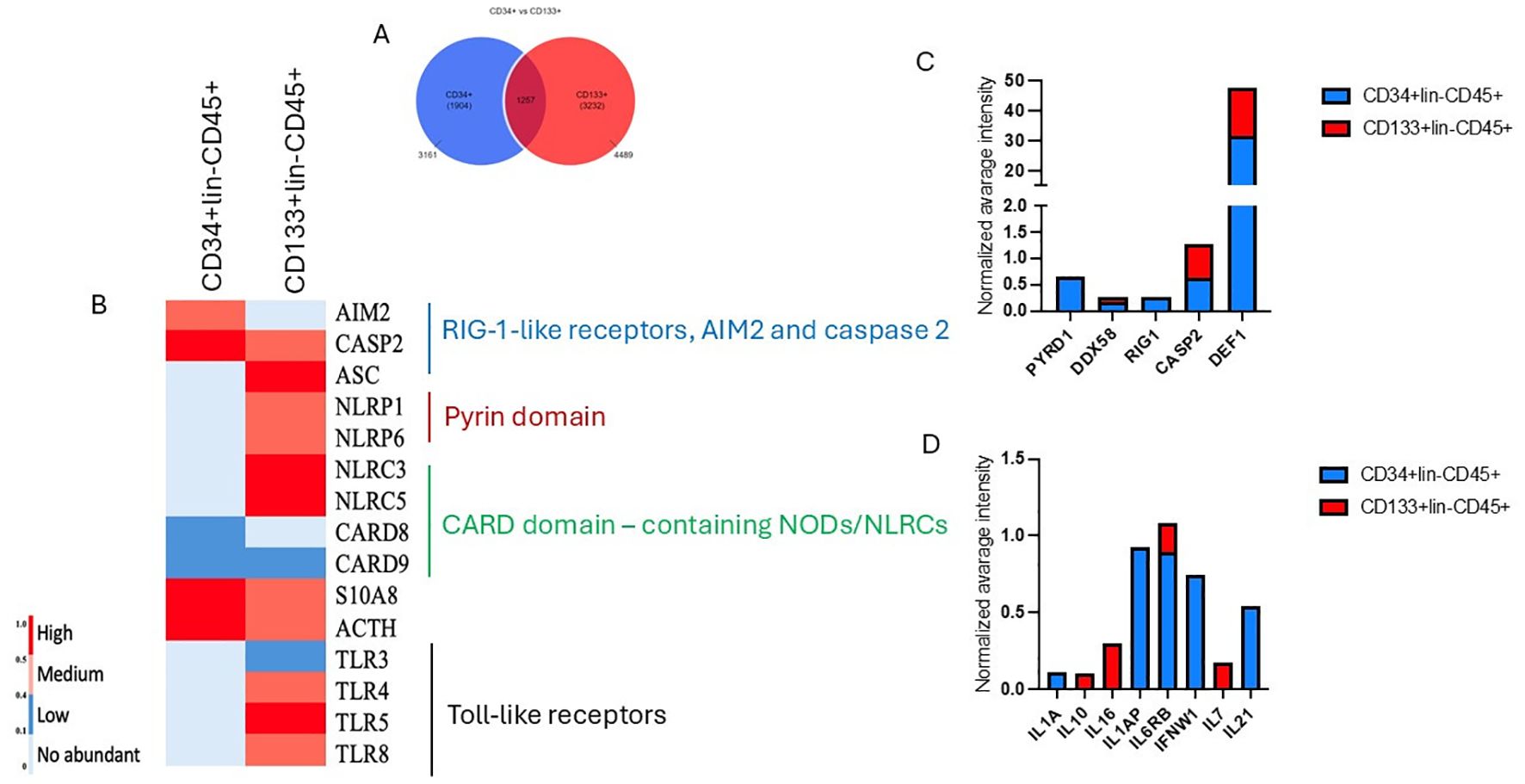
Figure 9. Analysis of annotated proteins to CD34+lin-CD45+ and CD133+lin-CD45+ HSPCs dataset. (A) Vienna plot of annotated proteins for both datasets. (B) Comparative analysis of the mean normalized heat map for CD34+ and CD133+HSPCs dataset presents annotated common proteins corresponding to GO biological processes regulating immune response, inflammation, and cell death. (C, D) The mean normalized intensity displays the abundance of annotated proteins playing a pivotal role in the inflammation.
Simultaneously, we detected increased caspase 2 and defensin for the CD133+ HSPCs dataset (Figure 9C). We also employed the Uniprot database and identified several immunomodulatory cytokines, including IL1, IFN, IL10, IL21 and IL-7 (Figure 9D).
4 Discussion
Using scRNA-seq, we confirmed that mRNA for some receptor and protein components of the immune system are expressed in purified human HSPCs, indicating cell subpopulations in which these genes are up or down regulated. There are published studies in which mRNA isolated as bulk from cells enriched for HSPCs were employed for RT-PCR analysis (18, 22, 61). To address this issue better, we used prospectively isolated by FASCs human umbilical cord blood (UCB)-derived CD34+Lin-CD45+ and CD133+Lin-CD45+ cells and performed single-cell RNA-seq (scRNA-seq) analysis to detect mRNA of genes involved in immune responses. We analysed our mRNA expression data sets employing the Uniform Manifold Approximation and Projection for Dimension Reduction (UMAP) technique, which allows us to visualize separate cell clusters (36). Since both CD34+Lin-CD45+ and CD133+Lin-CD45+ cell populations are enriched for the cells at different levels of specification toward hematopoietic and lymphoid lineages, it was challenging to evaluate the expression of innate immunity markers in these cells. In particular, we were interested in UMAP clusters enriched for mRNA encoding antigens CD34, CD133, and c-kit receptors. We also employed untargeted mass spectrometry for proteomic analysis and identified several biological pathways and the number of proteins involved in innate and adaptive immunity and inflammation.
First, we evaluated the expression of membrane-bound and intracellular pattern recognition receptors (PPRs). PPRs are germline-encoded host sensors, which detect molecules typical for the pathogens (pathogen-associated molecular patterns – PAMPs) or release from damaged/apoptotic tissues (damage-associated molecular patterns DAMPs) (62–64). They are highly expressed by innate immunity cells, including dendritic cells, macrophages, monocytes, and epithelial cells (64). Interestingly, as reported, BM cells from TLR4−/−, TLR9−/−, and MyD88−/−mice have a repopulating advantage when transplanted competitively with wild-type marrow into lethally irradiated recipient animals (27, 65, 66). This suggests that TLR signaling may contribute to the maintenance of HSPCs under homeostatic conditions and that endogenous TLR ligands, such as oligosaccharide (LPS) produced by normal gut flora, may regulate HSPCs under steady-state conditions after interacting with selected TLRs (67). By employing scRNA-seq, we identified that cells enriched for mRNA encoding CD34, CD133, and c-kit express also TLR1, TLR2, and TLR6. We also detected mRNA for MYD88, which plays an important role in intracellular signalling from these receptors. Humans lack TLR11, TLR12, and TLR13 in contrast to rodents (62). The expression of Toll-like receptors is corroborated by mRNA expression for MYD88, an adaptor protein required for transducing signals from the activated Toll-like receptors (68). The family of Toll-like receptors is a cell surface-expressed protein class that plays a key role in innate immune system responses. They are expressed on sentinel cells, including macrophages and dendritic cells, which recognize structurally conserved molecules derived from microbes (69). It has been postulated that some of them are also expressed in HSPCs (24). The receptors TLR1, TLR2, TLR4, TLR5, TLR6, and TLR10 are located on the cell membrane, whereas TLR3, TLR7, TLR8, and TLR9 are located in cytosol (70, 71). In one of the reports, it has been postulated that human UCB CD34+ cells express TLR2 and TLR4 and are involved in the specification of CD34+ cells into megakaryopoiesis/thrombopoiesis (72). In another report, it was demonstrated that human HSPCs express TLR4 and can directly sense pathogens and, in response, produce cytokines that promote the emergency of granulopoiesis (73, 74). It has also been shown that signalling through TLRs 7/8 induces the differentiation of human BM CD34+ cells along the myeloid lineage and, in another report, that PAM3CSK4, a synthetic triacylated lipopeptide known as TLRs 1/2 agonist, instructs human HSPCs to become specified into myeloid lineages (75). However, these studies still need to address which subpopulation of HSPCs are responsible for these effects at which specification level.
Next, we analysed the expression of cytoplasmic PRRs, including NOD-like receptors (NLRs) and RIG-I-like receptors (RLRs) (51, 76). We noticed that primitive clusters of HSPCs express NOD1, NOD3 (NLRC3), NOD4 (NLRC5), NOD5 (NLRX1), NLRA (CIITA), and NLRB1 (NAIP). We also found the expression in these cells of mRNA for RIG-I-like receptors MDA5/(IFIH1) and DDX58. RIG-1-like receptors are critical sensors of virus infection, mediating the transcriptional induction of type I interferons and other genes that collectively establish an antiviral host response (76, 77). Expression of these receptors was recently demonstrated during the specification of murine embryonic cells into haematopoiesis (78) and hematopoietic precursors in zebrafish (78). To support this in human embryogenesis, NOD1, as part of the RAC1-NOD1-RIPK2-NF-kB axis, is a critical intrinsic inductor that primes endothelial cells before hemogenic endothelial fate switch and HSPCs specification (79). The next member of this family, NOD3 (Nlrc3), is highly expressed in hematopoietic differentiation stages in vivo and in vitro and is required in hematopoiesis in zebrafish (80). Mechanistically, NOD3 activates the Notch pathway and is indispensable in hematopoietic stem and progenitor cell emergence and expansion of HSPCs (80). Similarly, it has been reported that RIG-I-like receptors are also involved in the specification of HSPCs in the zebrafish model (81).
Interferon-inducible protein AIM2, also known as absent in melanoma 2, is a protein that in humans is a cytoplasmic sensor found in hematopoietic cells that recognizes the presence of double-stranded DNA (dsDNA) of microbial or host cellular origin (82). In our study, AIM2 expression was found however, its level was very slight and observed only in single cells of both HSPCs populations.
The analysis of another group of NOD-like receptors known as NLRPs (83) revealed expression of NLRP1, NLRP2, NLRP3, NLRP4 (PAN2) in human UCB-purified HSPCs expressing mRNA for CD34, CD133, and c-kit. While NLRP1 controls hematopoietic reconstitution after transplantation and NLRP1-KO in the bone marrow microenvironment could significantly relieve bone marrow inflammatory response and promote hematopoietic reconstitution (84), NLRP2 has a similar role (85). In contrast, NLRP3 has been demonstrated to be expressed in human HSPCs and plays an important role in the migration of these cells and in regulating cell metabolism and survival (86). Moreover, NLRP3 inflammasome is activated in aged HSPCs due to mitochondrial stress and SIRT2 inactivation, contributing to the functional decline of these cells during aging (58).
Next, based on exciting data that some types of cells, including lymphocytes, may express complement proteins (complosome) that may be functional inside cells (17, 20, 21), we evaluated the expression of mRNA for major components of the complement cascade in our purified from UCB HSPCs and focused on cell clusters enriched for mRNA for CD34, CD133, and c-kit. We noticed expressions of C5, C3, and C5aR1. Increased expression was observed mainly in the case of C3AR1 and C5AR1 but also C5AR2 in both HSPCs populations. which are receptors of C3a and C5a subunits. C3AR1 receptor, after binding to C3a, can initiate a signaling cascade leading to inflammatory reactions such as mast cell degranulation, cytokine secretion, and leukocyte chemotaxis. Respectively, the C5AR1 receptor, after binding to C5a, one of the most potent inflammatory mediators, can lead to various cellular responses, including increases in blood vessel permeability, neutrophil chemotaxis, and the release of proinflammatory cytokines (87). A low level of expression of C3 and C5 genes with the increased expression level of C3AR1, C5AR1, and C5AR2 genes may indicate a later stage of complosome activation in these cells. Novel data indicates that complement proteins are not synthesized only in the liver. Still, normal lymphocytes also express them and as reported, activate intracellular complement receptors intracrine-dependently (20). This novel regulatory loop operating in lymphocytes has been named complosome (17, 21), and in addition to lymphocytes (20), we have demonstrated it to be expressed in normal HSPCs (22). Some complement protein components expression corroborated our previous studies with bulk mRNA extractions (18, 22). We confirmed Dr. Kemper’s data that complosome is expressed at higher levels in cells committed to lymphocytic lineage (18). Furthermore, we found that it is also expressed in HSPCs, playing an important role in the regulation of their trafficking, metabolism and proliferation (23). Our data with human HSPCs reveal that the more critical component of the complosome is C5. However, since these cells do not express all the components of proximal ComC proteins and C3 activating cathepsin L, other proteases must be involved in the activation/cleavage of C5.
As it was mentioned above, the expression of complosome regulatory elements was found in the primitive, quiescent subpopulations in both HSPCs datasets. Complement factor H (CFH) expression was found only in cells expressing CD34, CD133, and c-KIT in CD34+lin-CD45+ and CD133+lin-CD45+ cells. This regulatory protein controls the activity of the complement system to prevent damage to host tissues. It inhibits the alternative pathway by binding to C3b, accelerating the decay of C3 convertase (C3bBb), and acting as a cofactor for Factor I-mediated cleavage of C3b. Due to this, by differentiating self from non-self, the complement system does not attack its cells. The expression of CFH in quiescent cells confirms their role in preserving inactive cells for further needs. SERPING1 (C1NH) is a gene that codes for a C1 inhibitor. It is crucial in regulating the complement system’s functions, controlling inflammation, and preventing excessive complement activation. We observed its expression mostly in primitive, quiescent subpopulations of HSPCs. Some expression traces were observed in other clusters of both datasets, but their level was very low. CD59, also known as protectin, is a membrane-bound glycoprotein that plays a critical role in regulating the complement system functioning, mainly by inhibiting the formation of the membrane attack complex (MAC). In our study, its expression was found primarily on primitive subpopulations; however, in the case of CD34+lin-CD45+, it was also found in cells expressing GYPA. The complement cascade is tightly regulated to protect host cells, including HSPCs, from indiscriminate attacks by its activated components (13). Complement inhibitors include the plasma serine proteinase inhibitor serpin (C1 inactivator) (88). The classical pathway is also inhibited by surface-bound proteins CD55 (also known as decay accelerating factor or DAF) (89) and CD46 (also known as membrane co-factor protein or MCP) (90). Furthermore, CD59 is an essential regulatory protein of the complement terminal pathway, the membrane attack complex (MAC) (91). In contrast, complement factor H (CFH) is a key regulator of the alternative complement pathway, ensuring that the complement activation is directed against pathogens or harmful materials while preventing damage to host tissue (92). At the same time, complement factor I (CFI), also known as C3b/C4b inactivator, controls complement activation by cleaving C3b and C4b in both cell-bound or fluid phases (93).
Thus, our data on purified human HSPCs analysed based on transcriptome signature and categorized into subpopulations revealed that cells expressing mRNA of CD34, CD133, and c-kit also express several genes characteristic of innate and acquired immunity cells. It is important to realize that even though we sorted cells based on their very early hematopoietic development phenotype characteristic for HSPCs, only a few such cells are clonogenic in in vitro functional progenitor assays. These clonogenic cells, however, correspond to UMAP clusters enriched in mRNA for CD34, CD133, and c-kit.
We also employed untargeted mass spectrometry for proteomic analysis and identified several biological pathways and the number of proteins involved in innate and adaptive immunity and inflammation. We observed some similarities and differences between both populations of HSPCs.
For example, Toll-like receptors (TLR3, TLR4, TLR5) and proteins with pyrin (PYD) domain were more abundant in CD133+lin-CD45+ datasets. On the other hand, AIM2 and ACS proteins were detected in both datasets.
5 Conclusion
In this study, we provided a comprehensive analysis of human hematopoietic stem and progenitor cells (HSPCs) purified from umbilical cord blood (UCB) using single-cell RNA sequencing (scRNA-seq) and proteomic approaches. Our findings confirm that mRNA for receptors and proteins associated with innate and adaptive immunity are expressed in HSPCs, particularly in subpopulations enriched for CD34, CD133, and c-kit markers. Using scRNA-seq and UMAP clustering, we identified specific patterns of gene expression that shed light on the roles of these immune components in early hematopoietic development.
Notably, we detected the expression of Toll-like receptors (e.g., TLR1, TLR2, and TLR6) and the adaptor protein MYD88, suggesting potential involvement of these receptors in HSPC maintenance and lineage specification. Additionally, cytoplasmic pattern recognition receptors, including NOD-like and RIG-I-like receptors, were expressed in primitive HSPC clusters, implicating these receptors in hematopoietic development and immune response regulation. Furthermore, our proteomic analysis corroborated these findings and revealed differences in the abundance of immune-related proteins between CD34+Lin-CD45+ and CD133+Lin-CD45+ cell populations.
Importantly, we observed the expression of complement components, such as C3AR1, C5AR1, and C5AR2, and regulatory proteins like CFH and SERPING1 in quiescent HSPCs. These findings highlight the potential involvement of intracellular complement signaling (complosome) in preserving HSPC integrity and modulating their response to environmental stimuli.
Collectively, our results provide new insights into the immune properties of human HSPCs, underscoring their potential role in innate and adaptive immune responses. These findings enhance our understanding of HSPC biology and may inform future therapeutic strategies aimed at harnessing the immune capabilities of these cells for regenerative medicine and immune modulation.
Data availability statement
The datasets presented in this study can be found in online repositories. The names of the repository/repositories and accession number(s) can be found below: https://www.ncbi.nlm.nih.gov/genbank/, PRJNA1128409.
Ethics statement
The studies involving humans were approved by Warsaw Bioethics Committee permission number KB/50/2022. The studies were conducted in accordance with the local legislation and institutional requirements. The participants provided their written informed consent to participate in this study.
Author contributions
JJ: Formal analysis, Investigation, Methodology, Software, Writing – original draft. KT: Data curation, Resources, Software, Writing – original draft. DW: Data curation, Resources, Writing – original draft. KB: Data curation, Methodology, Resources, Writing – original draft. MZR: Supervision, Writing – original draft, Writing – review & editing. MK: Conceptualization, Project administration, Supervision, Writing – original draft, Writing – review & editing.
Funding
The author(s) declare that financial support was received for the research and/or publication of this article. This work was supported by NCN OPUS 2022/45/B/NZ6/00475 grant from the National Science Center to MR.
Conflict of interest
The authors declare that the research was conducted in the absence of any commercial or financial relationships that could be construed as a potential conflict of interest.
Generative AI statement
The author(s) declare that no Generative AI was used in the creation of this manuscript.
Publisher’s note
All claims expressed in this article are solely those of the authors and do not necessarily represent those of their affiliated organizations, or those of the publisher, the editors and the reviewers. Any product that may be evaluated in this article, or claim that may be made by its manufacturer, is not guaranteed or endorsed by the publisher.
References
1. Mikkola HK, Orkin SH. The journey of developing hematopoietic stem cells. Development. (2006) 133:3733–44. doi: 10.1242/dev.02568
2. Calvanese V, Mikkola HKA. The genesis of human hematopoietic stem cells. Blood. (2023) 142:519–32. doi: 10.1182/blood.2022017934
3. Ratajczak MZ, Kucia M. Hematopoiesis and innate immunity: an inseparable couple for good and bad times, bound together by an hormetic relationship. Leukemia. (2022) 36:23–32. doi: 10.1038/s41375-021-01482-0
4. Ratajczak MZ, Bujko K, Brzezniakiewicz-Janus K, Ratajczak J, Kucia M. Hematopoiesis revolves around the primordial evolutional rhythm of purinergic signaling and innate immunity - A journey to the developmental roots. Stem Cell Rev Rep. (2024) 20:827–38. doi: 10.1007/s12015-024-10692-9
5. Orkin SH, Zon LI. Hematopoiesis: an evolving paradigm for stem cell biology. Cell. (2008) 132:631–44. doi: 10.1016/j.cell.2008.01.025
6. Galloway JL, Zon LI. Ontogeny of hematopoiesis: examining the emergence of hematopoietic cells in the vertebrate embryo. Curr Top Dev Biol. (2003) 53:139–58. doi: 10.1016/S0070-2153(03)53004-6
7. Medvinsky A, Dzierzak E. Definitive hematopoiesis is autonomously initiated by the AGM region. Cell. (1996) 86:897–906. doi: 10.1016/S0092-8674(00)80165-8
8. Chen MJ, Lummertz da Rocha E, Cahan P, Kubaczka C, Hunter P, Sousa P, et al. Transcriptome dynamics of hematopoietic stem cell formation revealed using a combinatorial runx1 and ly6a reporter system. Stem Cell Rep. (2020) 14:956–71. doi: 10.1016/j.stemcr.2020.03.020
9. Gazit R, Garrison BS, Rao TN, Shay T, Costello J, Ericson J, et al. Transcriptome analysis identifies regulators of hematopoietic stem and progenitor cells. Stem Cell Rep. (2013) 1:266–80. doi: 10.1016/j.stemcr.2013.07.004
10. Georgantas RW 3rd, Tanadve V, Malehorn M, Heimfeld S, Chen C, Carr L, et al. Microarray and serial analysis of gene expression analyses identify known and novel transcripts overexpressed in hematopoietic stem cells. Cancer Res. (2004) 64:4434–41. doi: 10.1158/0008-5472.CAN-03-3247
11. Broxmeyer HE, Kim CH. Regulation of hematopoiesis in a sea of chemokine family members with a plethora of redundant activities. Exp Hematol. (1999) 27:1113–23. doi: 10.1016/S0301-472X(99)00045-4
12. Chambers SM, Boles NC, Lin KY, Tierney MP, Bowman TV, Bradfute SB, et al. Hematopoietic fingerprints: an expression database of stem cells and their progeny. Cell Stem Cell. (2007) 1:578–91. doi: 10.1016/j.stem.2007.10.003
13. Ricklin D, Lambris JD. Complement in immune and inflammatory disorders: pathophysiological mechanisms. J Immunol. (2013) 190:3831–8. doi: 10.4049/jimmunol.1203487
14. Cedzynski M, Swierzko AS, Kilpatrick DC. Factors of the lectin pathway of complement activation and their clinical associations in neonates. J BioMed Biotechnol. (2012) 2012:363246. doi: 10.1155/2012/363246
15. Suresh R, Chandrasekaran P, Sutterwala FS, Mosser DM. Complement-mediated 'bystander' damage initiates host NLRP3 inflammasome activation. J Cell Sci. (2016) 129:1928–39. doi: 10.1242/jcs.179291
16. Dunkelberger JR, Song WC. Complement and its role in innate and adaptive immune responses. Cell Res. (2010) 20:34–50. doi: 10.1038/cr.2009.139
17. West EE, Kemper C. Complosome - the intracellular complement system. Nat Rev Nephrol. (2023) 19:426–39. doi: 10.1038/s41581-023-00704-1
18. Bujko K, Brzenziakiewicz-Janus K, Kucia M, Ratajczak MZ. Intracellular complement (Complosome) is expressed in several types of human adult bone marrow-derived stem cells. Stem Cell Rev Rep. (2024) 20:437–9. doi: 10.1007/s12015-023-10650-x
19. West EE, Kolev M, Kemper C. Complement and the regulation of T cell responses. Annu Rev Immunol. (2018) 36:309–38. doi: 10.1146/annurev-immunol-042617-053245
20. Cardone J, Le Friec G, Vantourout P, Roberts A, Fuchs A, Jackson I, et al. Complement regulator CD46 temporally regulates cytokine production by conventional and unconventional T cells. Nat Immunol. (2010) 11:862–71. doi: 10.1038/ni.1917
21. Arbore G, Kemper C, Kolev M. Intracellular complement - the complosome - in immune cell regulation. Mol Immunol. (2017) 89:2–9. doi: 10.1016/j.molimm.2017.05.012
22. Ratajczak MZ, Adamiak M, Abdelbaset-Ismail A, Bujko K, Thapa A, Chumak V, et al. Intracellular complement (complosome) is expressed in hematopoietic stem/progenitor cells (HSPCs) and regulates cell trafficking, metabolism and proliferation in an intracrine Nlrp3 inflammasome-dependent manner. Leukemia. (2023) 37:1401–5. doi: 10.1038/s41375-023-01894-0
23. Boiko JR, Borghesi L. Hematopoiesis sculpted by pathogens: Toll-like receptors and inflammatory mediators directly activate stem cells. Cytokine. (2012) 57:1–8. doi: 10.1016/j.cyto.2011.10.005
24. Nagai Y, Garrett KP, Ohta S, Bahrun U, Kouro T, Akira S, et al. Toll-like receptors on hematopoietic progenitor cells stimulate innate immune system replenishment. Immunity. (2006) 24:801–12. doi: 10.1016/j.immuni.2006.04.008
25. Herman AC, Monlish DA, Romine MP, Bhatt ST, Zippel S, Schuettpelz LG. Systemic TLR2 agonist exposure regulates hematopoietic stem cells via cell-autonomous and cell-non-autonomous mechanisms. Blood Cancer J. (2016) 6:e437. doi: 10.1038/bcj.2016.45
26. Heidegger S, van den Brink MR, Haas T, Poeck H. The role of pattern-recognition receptors in graft-versus-host disease and graft-versus-leukemia after allogeneic stem cell transplantation. Front Immunol. (2014) 5:337. doi: 10.3389/fimmu.2014.00337
27. Takizawa H, Fritsch K, Kovtonyuk LV, Saito Y, Yakkala C, Jacobs K, et al. Pathogen-induced TLR4-TRIF innate immune signaling in hematopoietic stem cells promotes proliferation but reduces competitive fitness. Cell Stem Cell. (2017) 21:225–40 e5. doi: 10.1016/j.stem.2017.06.013
28. He Q, Zhang C, Wang L, Zhang P, Ma D, Lv J, et al. Inflammatory signaling regulates hematopoietic stem and progenitor cell emergence in vertebrates. Blood. (2015) 125:1098–106. doi: 10.1182/blood-2014-09-601542
29. Espin-Palazon R, Stachura DL, Campbell CA, Garcia-Moreno D, Del Cid N, Kim AD, et al. Proinflammatory signaling regulates hematopoietic stem cell emergence. Cell. (2014) 159:1070–85. doi: 10.1016/j.cell.2014.10.031
30. Li Y, Li Y, Cao X, Jin X, Jin T. Pattern recognition receptors in zebrafish provide functional and evolutionary insight into innate immune signaling pathways. Cell Mol Immunol. (2017) 14:80–9. doi: 10.1038/cmi.2016.50
31. Su M, Pan T, Chen QZ, Zhou WW, Gong Y, Xu G, et al. Data analysis guidelines for single-cell RNA-seq in biomedical studies and clinical applications. Mil Med Res. (2022) 9:68. doi: 10.1186/s40779-022-00434-8
32. Popescu DM, Botting RA, Stephenson E, Green K, Webb S, Jardine L, et al. Decoding human fetal liver haematopoiesis. Nature. (2019) 574:365–71. doi: 10.1038/s41586-019-1652-y
33. Flohr Svendsen A, Yang D, Kim K, Lazare S, Skinder N, Zwart E, et al. A comprehensive transcriptome signature of murine hematopoietic stem cell aging. Blood. (2021) 138:439–51. doi: 10.1182/blood.2020009729
34. Zeng Y, He J, Bai Z, Li Z, Gong Y, Liu C, et al. Tracing the first hematopoietic stem cell generation in human embryo by single-cell RNA sequencing. Cell Res. (2019) 29:881–94. doi: 10.1038/s41422-019-0228-6
35. Jarczak J, Bujko K, Ratajczak MZ, Kucia M. scRNA-seq revealed transcriptional signatures of human umbilical cord primitive stem cells and their germ lineage origin regulated by imprinted genes. Sci Rep. (2024) 14:29264. doi: 10.1038/s41598-024-79810-4
36. McInnes L, Healy J, Saul N, Grobberger L. UMAP: uniform manifold approximation and projection. J Open Source Software. (2018) 3(29):861. doi: 10.21105/joss.00861
37. Becht E, McInnes L, Healy J, Dutertre CA, Kwok IWH, Ng LG, et al. Dimensionality reduction for visualizing single-cell data using UMAP. Nat Biotechnol. (2018) 37:38–44. doi: 10.1038/nbt.4314
38. Bujko K, Ciechanowicz AK, Kucia M, Ratajczak MZ. Molecular analysis and comparison of CD34(+) and CD133(+) very small embryonic-like stem cells purified from umbilical cord blood. Cytometry A. (2023) 103:703–11. doi: 10.1002/cyto.a.v103.9
39. Suszynska M, Zuba-Surma EK, Maj M, Mierzejewska K, Ratajczak J, Kucia M, et al. The proper criteria for identification and sorting of very small embryonic-like stem cells, and some nomenclature issues. Stem Cells Dev. (2014) 23:702–13. doi: 10.1089/scd.2013.0472
40. Ratajczak J, Zuba-Surma E, Klich I, Liu R, Wysoczynski M, Greco N, et al. Hematopoietic differentiation of umbilical cord blood-derived very small embryonic/epiblast-like stem cells. Leukemia. (2011) 25:1278–85. doi: 10.1038/leu.2011.73
41. Hao Y, Stuart T, Kowalski MH, Choudhary S, Hoffman P, Hartman A, et al. Dictionary learning for integrative, multimodal and scalable single-cell analysis. Nat Biotechnol. (2024) 42:293–304. doi: 10.1038/s41587-023-01767-y
42. Satija R, Farrell JA, Gennert D, Schier AF, Regev A. Spatial reconstruction of single-cell gene expression data. Nat Biotechnol. (2015) 33:495–502. doi: 10.1038/nbt.3192
44. Chen S, Zhou Y, Chen Y, Gu J. fastp: an ultra-fast all-in-one FASTQ preprocessor. Bioinformatics. (2018) 34:i884–i90. doi: 10.1093/bioinformatics/bty560
45. Team RC. R: A language and environment for statistical computing. R Foundation for Statistical Computing (2021).
46. Wickham H, Averick M, Bryan J, Chang W, D’Agostino McGowan L, François R, et al. Welcome to the tidyverse. J Open Source Software. (2019) 4:1686. doi: 10.21105/joss.01686
47. Wickham H, Bryan J. Readxl: Read Excel Files R Package Version 1.3.1. (2019). Available online at: https://CRAN.R-project.org/package=readxl.
48. Wickham H, François R, Henry L, Müller K. Dplyr: A Grammar of Data Manipulation. R Package Version 0.8.4. (2020). Available online at: https://CRAN.R-project.org/package=dplyr.
49. Lionel H, Wickham H. Purrr: Functional Programming Tools. (2020). Available online at: https://cran.r-project.org/web/packages/purrr/index.html.
50. Ooms J. The writexl package: zero dependency xlsx writer for R. (2017). Available online at: https://cran.r-project.org/web/packages/writexl/index.html.
51. Carneiro LA, Travassos LH, Girardin SE. Nod-like receptors in innate immunity and inflammatory diseases. Ann Med. (2007) 39:581–93. doi: 10.1080/07853890701576172
52. Franchi L, Warner N, Viani K, Nunez G. Function of Nod-like receptors in microbial recognition and host defense. Immunol Rev. (2009) 227:106–28. doi: 10.1111/j.1600-065X.2008.00734.x
53. Swanson KV, Deng M, Ting JP. The NLRP3 inflammasome: molecular activation and regulation to therapeutics. Nat Rev Immunol. (2019) 19:477–89. doi: 10.1038/s41577-019-0165-0
54. Ratajczak MZ, Bujko K, Cymer M, Thapa A, Adamiak M, Ratajczak J, et al. The Nlrp3 inflammasome as a "rising star" in studies of normal and Malignant hematopoiesis. Leukemia. (2020) 34:1512–23. doi: 10.1038/s41375-020-0827-8
55. De Nardo D, Latz E. NLRP3 inflammasomes link inflammation and metabolic disease. Trends Immunol. (2011) 32:373–9. doi: 10.1016/j.it.2011.05.004
56. Lenkiewicz AM, Adamiak M, Thapa A, Bujko K, Pedziwiatr D, Abdel-Latif AK, et al. The Nlrp3 inflammasome orchestrates mobilization of bone marrow-residing stem cells into peripheral blood. Stem Cell Rev Rep. (2019) 15:391–403. doi: 10.1007/s12015-019-09890-7
57. Adamiak M, Abdel-Latif A, Bujko K, Thapa A, Anusz K, Tracz M, et al. Nlrp3 inflammasome signaling regulates the homing and engraftment of hematopoietic stem cells (HSPCs) by enhancing incorporation of CXCR4 receptor into membrane lipid rafts. Stem Cell Rev Rep. (2020) 16:954–67. doi: 10.1007/s12015-020-10005-w
58. Luo H, Mu WC, Karki R, Chiang HH, Mohrin M, Shin JJ, et al. Mitochondrial stress-initiated aberrant activation of the NLRP3 inflammasome regulates the functional deterioration of hematopoietic stem cell aging. Cell Rep. (2019) 26:945–54 e4. doi: 10.1016/j.celrep.2018.12.101
59. Espinoza JL, Kamio K, Lam VQ, Takami A. The impact of NLRP3 activation on hematopoietic stem cell transplantation. Int J Mol Sci. (2021) 22(21):11845. doi: 10.3390/ijms222111845
60. Zheng D, Liwinski T, Elinav E. Inflammasome activation and regulation: toward a better understanding of complex mechanisms. Cell Discovery. (2020) 6:36. doi: 10.1038/s41421-020-0167-x
61. Adamiak M, Lenkiewicz AM, Cymer M, Kucia M, Ratajczak J, Ratajczak MZ. Novel evidence that an alternative complement cascade pathway is involved in optimal mobilization of hematopoietic stem/progenitor cells in Nlrp3 inflammasome-dependent manner. Leukemia. (2019) 33:2967–70. doi: 10.1038/s41375-019-0530-9
62. Mahla RS, Reddy MC, Prasad DV, Kumar H. Sweeten PAMPs: role of sugar complexed PAMPs in innate immunity and vaccine biology. Front Immunol. (2013) 4:248. doi: 10.3389/fimmu.2013.00248
63. Roh JS, Sohn DH. Damage-associated molecular patterns in inflammatory diseases. Immune Netw. (2018) 18:e27. doi: 10.4110/in.2018.18.e27
64. Gong T, Liu L, Jiang W, Zhou R. DAMP-sensing receptors in sterile inflammation and inflammatory diseases. Nat Rev Immunol. (2020) 20:95–112. doi: 10.1038/s41577-019-0215-7
65. Bennett LF, Mumau MD, Li Y, Speck NA. MyD88-dependent TLR signaling oppositely regulates hematopoietic progenitor and stem cell formation in the embryo. Development. (2022) 149(8):dev200025. doi: 10.1242/dev.200025
66. Paracatu LC, Schuettpelz LG. Contribution of aberrant toll like receptor signaling to the pathogenesis of myelodysplastic syndromes. Front Immunol. (2020) 11. doi: 10.3389/fimmu.2020.01236
67. Velders GA, van Os R, Hagoort H, Verzaal P, Guiot HF, Lindley IJ, et al. Reduced stem cell mobilization in mice receiving antibiotic modulation of the intestinal flora: involvement of endotoxins as cofactors in mobilization. Blood. (2004) 103:340–6. doi: 10.1182/blood-2002-07-2270
68. Fitzgerald KA, Palsson-McDermott EM, Bowie AG, Jefferies CA, Mansell AS, Brady G, et al. Mal (MyD88-adapter-like) is required for Toll-like receptor-4 signal transduction. Nature. (2001) 413:78–83. doi: 10.1038/35092578
69. Kawai T, Akira S. The role of pattern-recognition receptors in innate immunity: update on Toll-like receptors. Nat Immunol. (2010) 11:373–84. doi: 10.1038/ni.1863
70. Kemball CC, Alirezaei M, Whitton JL. Type B coxsackieviruses and their interactions with the innate and adaptive immune systems. Future Microbiol. (2010) 5:1329–47. doi: 10.2217/fmb.10.101
71. Hansson GK, Edfeldt K. Toll to be paid at the gateway to the vessel wall. Arterioscler Thromb Vasc Biol. (2005) 25:1085–7. doi: 10.1161/01.ATV.0000168894.43759.47
72. D'Atri LP, Rodríguez CS, Miguel CP, Pozner RG, Wilczyñski JMO, Negrotto S, et al. Activation of toll-like receptors 2 and 4 on CD34 cells increases human megakaryo/thrombopoiesis induced by thrombopoietin. J Thromb Haemostasis. (2019) 17:2196–210. doi: 10.1111/jth.14605
73. Paracatu LC, Monlish DA, Greenberg ZJ, Fisher DAC, Walter MJ, Oh ST, et al. Toll-like receptor and cytokine expression throughout the bone marrow differs between patients with low- and high-risk myelodysplastic syndromes. Exp Hematol. (2022) 110:47–59. doi: 10.1016/j.exphem.2022.03.011
74. Sasaki Y, Guo YM, Goto T, Ubukawa K, Asanuma K, Kobayashi I, et al. IL-6 generated from human hematopoietic stem and progenitor cells through TLR4 signaling promotes emergency granulopoiesis by regulating transcription factor expression. J Immunol. (2021) 207:1078–86. doi: 10.4049/jimmunol.2100168
75. Bono C, Guerrero P, Erades A, Jordan-Pla A, Yanez A, Gil ML. Direct TLR2 signaling through mTOR and TBK1 induces C/EBPbeta and IRF7-dependent macrophage differentiation in hematopoietic stem and progenitor cells. Stem Cells. (2022) 40:949–62. doi: 10.1093/stmcls/sxac053
76. Onomoto K, Onoguchi K, Yoneyama M. Regulation of RIG-I-like receptor-mediated signaling: interaction between host and viral factors. Cell Mol Immunol. (2021) 18:539–55. doi: 10.1038/s41423-020-00602-7
77. Yoneyama M, Kikuchi M, Natsukawa T, Shinobu N, Imaizumi T, Miyagishi M, et al. The RNA helicase RIG-I has an essential function in double-stranded RNA-induced innate antiviral responses. Nat Immunol. (2004) 5:730–7. doi: 10.1038/ni1087
78. Lefkopoulos S, Polyzou A, Derecka M, Bergo V, Clapes T, Cauchy P, et al. Repetitive elements trigger RIG-I-like receptor signaling that regulates the emergence of hematopoietic stem and progenitor cells. Immunity. (2020) 53:934–51 e9. doi: 10.1016/j.immuni.2020.10.007
79. Cheng X, Barakat R, Pavani G, Usha MK, Calderon R, Snella E, et al. Nod1-dependent NF-kB activation initiates hematopoietic stem cell specification in response to small Rho GTPases. Nat Commun. (2023) 14:7668. doi: 10.1038/s41467-023-43349-1
80. Cai S, Li H, Tie R, Shan W, Luo Q, Wang S, et al. Nlrc3 signaling is indispensable for hematopoietic stem cell emergence via Notch signaling in vertebrates. Nat Commun. (2024) 15:226. doi: 10.1038/s41467-023-44251-6
81. Wang YY, Nie L, Xu XX, Shao T, Fan DD, Lin AF, et al. Essential role of RIG-I in hematopoietic precursor emergence in primitive hematopoiesis during zebrafish development. Immunohorizons. (2022) 6:283–98. doi: 10.4049/immunohorizons.2200028
82. Man SM, Zhu Q, Zhu L, Liu Z, Karki R, Malik A, et al. Critical role for the DNA sensor AIM2 in stem cell proliferation and cancer. Cell. (2015) 162:45–58. doi: 10.1016/j.cell.2015.06.001
83. Schroder K, Tschopp J. The inflammasomes. Cell. (2010) 140:821–32. doi: 10.1016/j.cell.2010.01.040
84. Hong F, Chen Y, Gao H, Shi J, Lu W, Ju W, et al. NLRP1 in bone marrow microenvironment controls hematopoietic reconstitution after transplantation. Transplant Cell Ther. (2021) 27:908 e1–e11. doi: 10.1016/j.jtct.2021.07.016
85. Yang L, Hu M, Lu Y, Han S, Wang J. Inflammasomes and the maintenance of hematopoietic homeostasis: new perspectives and opportunities. Molecules. (2021) 26(2):309. doi: 10.3390/molecules26020309
86. Frame JM, Kubaczka C, Long TL, Esain V, Soto RA, Hachimi M, et al. Metabolic regulation of inflammasome activity controls embryonic hematopoietic stem and progenitor cell production. Dev Cell. (2020) 55:133–49 e6. doi: 10.1016/j.devcel.2020.07.015
87. Ricklin D, Reis ES, Lambris JD. Complement in disease: a defence system turning offensive. Nat Rev Nephrol. (2016) 12:383–401. doi: 10.1038/nrneph.2016.70
88. Davis AE 3rd. Biological effects of C1 inhibitor. Drug News Perspect. (2004) 17:439–46. doi: 10.1358/dnp.2004.17.7.863703
89. Brodbeck WG, Kuttner-Kondo L, Mold C, Medof ME. Structure/function studies of human decay-accelerating factor. Immunology. (2000) 101:104–11. doi: 10.1046/j.1365-2567.2000.00086.x
90. Johnstone RW, Loveland BE, McKenzie IF. Identification and quantification of complement regulator CD46 on normal human tissues. Immunology. (1993) 79:341–7.
91. Couves EC, Gardner S, Voisin TB, Bickel JK, Stansfeld PJ, Tate EW, et al. Structural basis for membrane attack complex inhibition by CD59. Nat Commun. (2023) 14:890. doi: 10.1038/s41467-023-36441-z
92. Ferreira VP, Pangburn MK, Cortes C. Complement control protein factor H: the good, the bad, and the inadequate. Mol Immunol. (2010) 47:2187–97. doi: 10.1016/j.molimm.2010.05.007
Keywords: innate immunity, complement cascade, toll-like receptors, NOD-like receptors, NLRP3 inflammasome, complosome, scRNA-seq
Citation: Jarczak J, Thetchinamoorthy K, Wierzbicka D, Bujko K, Ratajczak MZ and Kucia M (2025) Expression of innate immunity genes in human hematopoietic stem/progenitor cells – single cell RNA-seq analysis. Front. Immunol. 16:1515856. doi: 10.3389/fimmu.2025.1515856
Received: 23 October 2024; Accepted: 19 March 2025;
Published: 08 April 2025.
Edited by:
Maciej Cedzynski, Polish Academy of Sciences, PolandReviewed by:
Alessandro Mangogna, University of Udine, ItalyPrzemyslaw Lewkowicz, Medical University of Lodz, Poland
Copyright © 2025 Jarczak, Thetchinamoorthy, Wierzbicka, Bujko, Ratajczak and Kucia. This is an open-access article distributed under the terms of the Creative Commons Attribution License (CC BY). The use, distribution or reproduction in other forums is permitted, provided the original author(s) and the copyright owner(s) are credited and that the original publication in this journal is cited, in accordance with accepted academic practice. No use, distribution or reproduction is permitted which does not comply with these terms.
*Correspondence: Magdalena Kucia, magdalena.kucia@wum.edu.pl; Mariusz Z. Ratajczak, mzrata01@louisville.edu