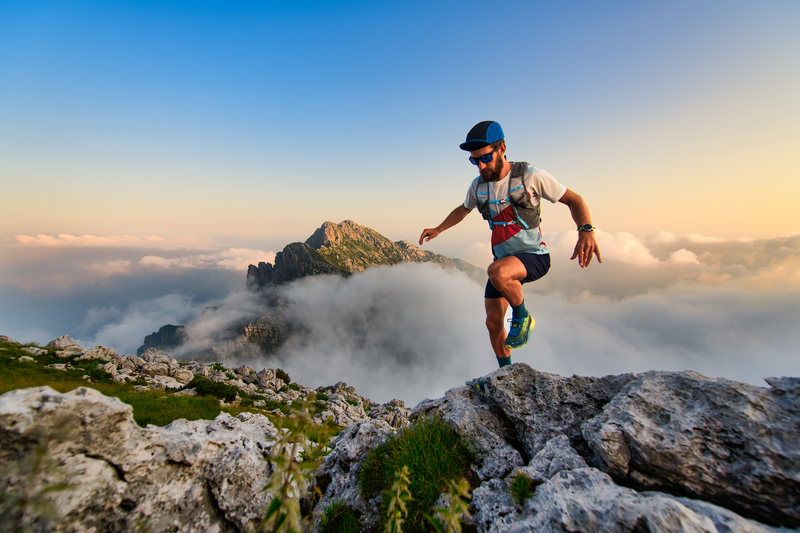
94% of researchers rate our articles as excellent or good
Learn more about the work of our research integrity team to safeguard the quality of each article we publish.
Find out more
ORIGINAL RESEARCH article
Front. Immunol.
Sec. Cancer Immunity and Immunotherapy
Volume 16 - 2025 | doi: 10.3389/fimmu.2025.1514273
This article is part of the Research Topic Sepsis Awareness Month 2024 View all articles
The final, formatted version of the article will be published soon.
You have multiple emails registered with Frontiers:
Please enter your email address:
If you already have an account, please login
You don't have a Frontiers account ? You can register here
Background: Sepsis is a severe complication in leukemia patients, contributing to high mortality rates. Identifying early predictors of sepsis is crucial for timely intervention. This study aimed to develop and validate a predictive model for sepsis risk in leukemia patients using machine learning techniques. Methods: This retrospective study included 4,310 leukemia patients admitted to the Affiliated Hospital of Guangdong Medical University from 2005 to 2024, using 70% for training and 30% for validation. Feature selection was performed using univariate logistic regression, LASSO, and the Boruta algorithm, followed by multivariate logistic regression analysis. Seven machine learning models were constructed and evaluated using receiver operating characteristic (ROC) curves and decision curve analysis (DCA). Shapley additive explanations (SHAP) were applied to interpret the results, and restricted cubic spline (RCS) regression explored the nonlinear relationships between variables and sepsis risk. Results: The random forest (RF) model outperformed all others, achieving an AUC of 0.765 in the training cohort and 0.700 in the validation cohort. Key predictors of sepsis identified by SHAP analysis included C-reactive protein (CRP), procalcitonin (PCT), neutrophil count (Neut), lymphocyte count (Lymph), thrombin time (TT), red blood cell count (RBC), total bile acid (TBA), and systolic blood pressure (SBP). RCS analysis revealed significant non-linear associations between CPR, PCT, Neut, Lymph, TT, RBC and SBP with sepsis risk. Conclusion: The RF model exhibited robust predictive power for sepsis in leukemia patients, providing clinicians with a valuable tool for early risk assessment and the optimization of treatment strategies.
Keywords: Leukemia, Sepsis, Prediction model, biomarkers, machine learning
Received: 20 Oct 2024; Accepted: 22 Jan 2025.
Copyright: © 2025 Kou, Tian, Ha, Wang, Sun, Lv, Luo, Yang and Qin. This is an open-access article distributed under the terms of the Creative Commons Attribution License (CC BY). The use, distribution or reproduction in other forums is permitted, provided the original author(s) or licensor are credited and that the original publication in this journal is cited, in accordance with accepted academic practice. No use, distribution or reproduction is permitted which does not comply with these terms.
* Correspondence:
Ling Qin, The First Affiliated Hospital of Henan University of Science and Technology, Luoyang, China
Disclaimer: All claims expressed in this article are solely those of the authors and do not necessarily represent those of their affiliated organizations, or those of the publisher, the editors and the reviewers. Any product that may be evaluated in this article or claim that may be made by its manufacturer is not guaranteed or endorsed by the publisher.
Research integrity at Frontiers
Learn more about the work of our research integrity team to safeguard the quality of each article we publish.