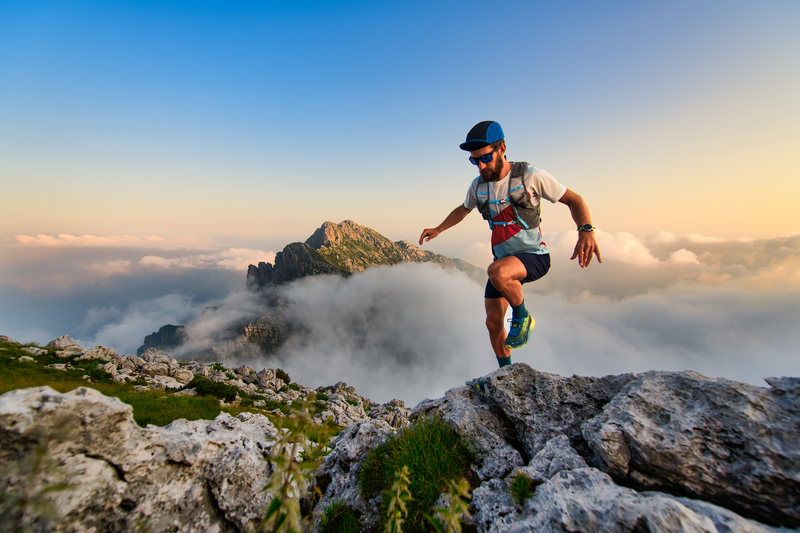
94% of researchers rate our articles as excellent or good
Learn more about the work of our research integrity team to safeguard the quality of each article we publish.
Find out more
ORIGINAL RESEARCH article
Front. Immunol.
Sec. Cancer Immunity and Immunotherapy
Volume 16 - 2025 | doi: 10.3389/fimmu.2025.1504387
The final, formatted version of the article will be published soon.
You have multiple emails registered with Frontiers:
Please enter your email address:
If you already have an account, please login
You don't have a Frontiers account ? You can register here
Background: PD-1 inhibitors, in combination with chemotherapy, have become the first-line treatment option for patients with advanced metastatic gastric cancer. However, some patients still do not benefit from this treatment, highlighting an urgent need for simple and reliable markers to predict the efficacy of immunotherapy.Methods: Immunotherapy efficacy was evaluated using RECIST 1.1 and categorized into complete remission (CR), partial remission (PR), stable disease (SD), and disease progression (PD). Patients with CR, PR, and SD were classified as non-PD responders, while PD patients were categorized as PD responders. Clinical characteristics and CT imaging features of gastric cancer patients from two centers who received PD-1 inhibitor combination chemotherapy were retrospectively analyzed. Clinical characteristics and CT imaging features of gastric cancer patients from two centers, before receiving PD-1 inhibitor combination chemotherapy, were retrospectively analyzed.A univariate logistic regression analysis was performed for each variable, and separate models for clinical and imaging characteristics, as well as a nomogram, were developed. Area under the curve (AUC), accuracy, sensitivity, specificity, and decision curve analysis (DCA) were used to evaluate all models. Results: Data from 272 patients (non-PD responders = 206, PD responders = 66) from Center 1 were collected for this study. Data from 76 patients (non-PD responders = 54, PD responders = 22) from Center 2 were used as an external validation cohort to verify the robustness of the models. We developed a clinical model, an imaging features model, and a nomogram. The nomogram, combining clinical and imaging features, demonstrated superior performance with an AUC of 0.904 (95% CI: 0.862-0.947) in the training set and an AUC of 0.801 (95% CI: 0.683-0.918) in the validation set, with sensitivity, specificity, and accuracy of 0.889, 0.682, and 0.829, respectively. Calibration curves further confirmed the agreement between actual results and predictions.Conclusions: A nomogram combining clinical features and CT imaging features before treatment was developed, which can effectively and simply predict the efficacy response of advanced gastric cancer patients treated with PD-1 inhibitors combined with chemotherapy. This tool can aid in optimizing treatment strategies in clinical practice.
Keywords: nomogram, gastric cancer, computed tomography, Programmed cell death 1 inhibitors, Chemo-immunotherapy
Received: 30 Sep 2024; Accepted: 12 Mar 2025.
Copyright: © 2025 Ma, Wang, Qiu, Xiao, Wu, Han, Xu and Wang. This is an open-access article distributed under the terms of the Creative Commons Attribution License (CC BY). The use, distribution or reproduction in other forums is permitted, provided the original author(s) or licensor are credited and that the original publication in this journal is cited, in accordance with accepted academic practice. No use, distribution or reproduction is permitted which does not comply with these terms.
* Correspondence:
Hui Xu, Shandong First Medical University, Tai'an, 271000, Shandong, China
Haiyan Wang, Department of Radiology, Shandong Provincial Hospital Affiliated to Shandong First Medical University, Jinan, China
Disclaimer: All claims expressed in this article are solely those of the authors and do not necessarily represent those of their affiliated organizations, or those of the publisher, the editors and the reviewers. Any product that may be evaluated in this article or claim that may be made by its manufacturer is not guaranteed or endorsed by the publisher.
Research integrity at Frontiers
Learn more about the work of our research integrity team to safeguard the quality of each article we publish.