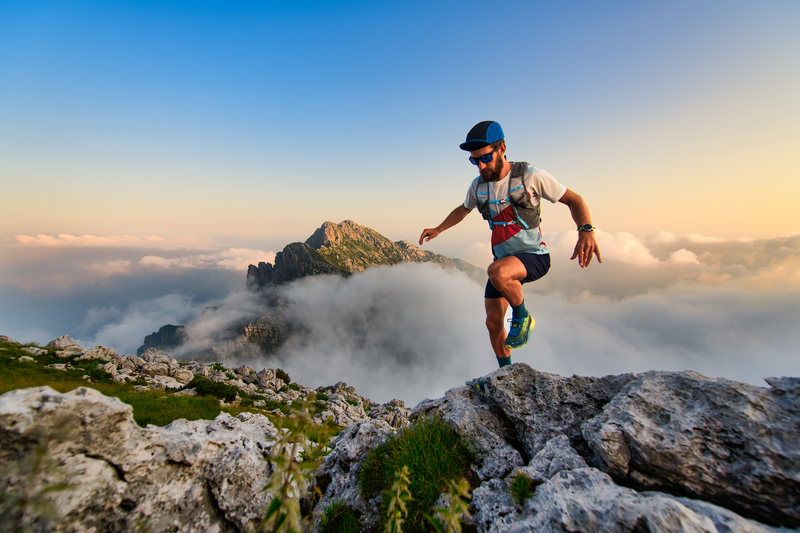
94% of researchers rate our articles as excellent or good
Learn more about the work of our research integrity team to safeguard the quality of each article we publish.
Find out more
ORIGINAL RESEARCH article
Front. Immunol.
Sec. Viral Immunology
Volume 16 - 2025 | doi: 10.3389/fimmu.2025.1487066
This article is part of the Research Topic Mathematical Modeling in Discovery and Analysis of Immune Responses View all 5 articles
The final, formatted version of the article will be published soon.
You have multiple emails registered with Frontiers:
Please enter your email address:
If you already have an account, please login
You don't have a Frontiers account ? You can register here
Vaccination against COVID-19 has been pivotal in reducing the global burden of the disease. However, Phase III trial results and observational studies underscore differences in efficacy across vaccine technologies and dosing regimens. Notably, mRNA vaccines have exhibited superior effectiveness compared to Adenovirus (AdV) vaccines, especially with extended dosing intervals. Using in-host mechanistic modelling, this study elucidates these variations and unravels the biological mechanisms shaping the immune responses at the cellular level. We used data on the change in memory B cells, plasmablasts, and antibody titres after the second dose of a COVID-19 vaccine for Australian healthcare workers.Alongside this dataset, we constructed a kinetic model of humoral immunity which jointly captured the dynamics of multiple immune markers, and integrated hierarchical effects into this kinetics model, including age, dosing schedule, and vaccine type. Our analysis estimated that mRNA vaccines induced 2.1 times higher memory B cell proliferation than AdV vaccines after adjusting for age, interval between doses and priming dose. Additionally, extending the duration between the second vaccine dose and priming dose beyond 28 days boosted neutralising antibody production per plasmablast concentration by 30%. We also found that antibody responses after the second dose were more persistent when mRNA vaccines were used over AdV vaccines and for longer dosing regimens. Reconstructing inhost kinetics in response to vaccination could help optimise vaccine dosing regimens, improve vaccine efficacy in different population groups, and inform the design of future vaccines for enhanced protection against emerging pathogens.
Keywords: SARS-CoV-2, immunology, mathematical modelling, Vaccination, B cell
Received: 27 Aug 2024; Accepted: 03 Mar 2025.
Copyright: © 2025 Hodgson, Liu, Carolan, Mahanty, Subbarao, Sullivan, Fox and Kucharski. This is an open-access article distributed under the terms of the Creative Commons Attribution License (CC BY). The use, distribution or reproduction in other forums is permitted, provided the original author(s) or licensor are credited and that the original publication in this journal is cited, in accordance with accepted academic practice. No use, distribution or reproduction is permitted which does not comply with these terms.
* Correspondence:
David Hodgson, London School of Hygiene and Tropical Medicine, University of London, London, United Kingdom
Disclaimer: All claims expressed in this article are solely those of the authors and do not necessarily represent those of their affiliated organizations, or those of the publisher, the editors and the reviewers. Any product that may be evaluated in this article or claim that may be made by its manufacturer is not guaranteed or endorsed by the publisher.
Research integrity at Frontiers
Learn more about the work of our research integrity team to safeguard the quality of each article we publish.