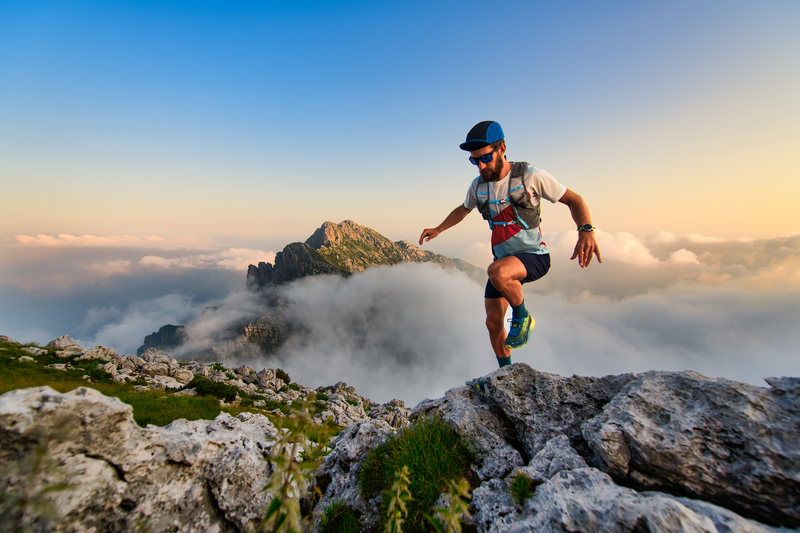
94% of researchers rate our articles as excellent or good
Learn more about the work of our research integrity team to safeguard the quality of each article we publish.
Find out more
CORRECTION article
Front. Immunol. , 15 November 2024
Sec. Cancer Immunity and Immunotherapy
Volume 15 - 2024 | https://doi.org/10.3389/fimmu.2024.1522632
This article is a correction to:
Unraveling the ecological landscape of mast cells in esophageal cancer through single-cell RNA sequencing
A Corrigendum on
Unraveling the ecological landscape of mast cells in esophageal cancer through single-cell RNA sequencing
By Zhang S, Zhang X, Xiahou Z, Zuo S, Xue J and Zhang Y (2024) Front. Immunol. 15:1470449. doi: 10.3389/fimmu.2024.1470449
In the published article, there was an error in affiliation 1. Instead of “Songjiang Hospital Affiliated to Shanghai Jiao Tong University School of Medicine, Shanghai, 201600, China.”, it should be “Department of Thoracic Surgery, Songjiang Hospital Affiliated to Shanghai Jiao Tong University School of Medicine, Shanghai, 201600, China.”.
The authors apologize for this error and state that this does not change the scientific conclusions of the article in any way. The original article has been updated.
All claims expressed in this article are solely those of the authors and do not necessarily represent those of their affiliated organizations, or those of the publisher, the editors and the reviewers. Any product that may be evaluated in this article, or claim that may be made by its manufacturer, is not guaranteed or endorsed by the publisher.
Keywords: single-cell RNA sequencing, mast cells, EGFR signaling pathway, prognostic model, esophageal cancer
Citation: Zhang S, Zhang X, Xiahou Z, Zuo S, Xue J and Zhang Y (2024) Corrigendum: Unraveling the ecological landscape of mast cells in esophageal cancer through single-cell RNA sequencing. Front. Immunol. 15:1522632. doi: 10.3389/fimmu.2024.1522632
Received: 04 November 2024; Accepted: 05 November 2024;
Published: 15 November 2024.
Approved by:
Frontiers Editorial Office, Frontiers Media SA, SwitzerlandCopyright © 2024 Zhang, Zhang, Xiahou, Zuo, Xue and Zhang. This is an open-access article distributed under the terms of the Creative Commons Attribution License (CC BY). The use, distribution or reproduction in other forums is permitted, provided the original author(s) and the copyright owner(s) are credited and that the original publication in this journal is cited, in accordance with accepted academic practice. No use, distribution or reproduction is permitted which does not comply with these terms.
*Correspondence: Yi Zhang, MjQwMjk0ODY2QHFxLmNvbQ==
†These authors have contributed equally to this work and share first authorship
Disclaimer: All claims expressed in this article are solely those of the authors and do not necessarily represent those of their affiliated organizations, or those of the publisher, the editors and the reviewers. Any product that may be evaluated in this article or claim that may be made by its manufacturer is not guaranteed or endorsed by the publisher.
Research integrity at Frontiers
Learn more about the work of our research integrity team to safeguard the quality of each article we publish.