- State Key Laboratory of Oncology in South China, Guangdong Provincial Clinical Research Center for Cancer, Sun Yat-sen University Cancer Center, Guangzhou, China
Helicobacter pylori (H. pylori), a globally prevalent pathogen Group I carcinogen, presents a formidable challenge in gastric cancer prevention due to its increasing antimicrobial resistance and strain diversity. This comprehensive review critically analyzes the limitations of conventional antibiotic-based therapies and explores cutting-edge approaches to combat H. pylori infections and associated gastric carcinogenesis. We emphasize the pressing need for innovative therapeutic strategies, with a particular focus on precision medicine and tailored vaccine development. Despite promising advancements in enhancing host immunity, current Helicobacter pylori vaccine clinical trials have yet to achieve long-term efficacy or gain approval regulatory approval. We propose a paradigm-shifting approach leveraging artificial intelligence (AI) to design precision-targeted, multiepitope vaccines tailored to multiple H. pylori subtypes. This AI-driven strategy has the potential to revolutionize antigen selection and optimize vaccine efficacy, addressing the critical need for personalized interventions in H. pylori eradication efforts. By leveraging AI in vaccine design, we propose a revolutionary approach to precision therapy that could significantly reduce H. pylori -associated gastric cancer burden.
1 Introduction
Helicobacter pylori (H. pylori) is a ubiquitous human pathogen that colonizes the gastric mucosa of approximately half the world’s population (1). This gram-negative bacterium has been classified as a Group I carcinogen by the World Health Organization due to its pivotal role in the etiology of various gastric disorders, including chronic gastritis, peptic ulcers, and gastric adenocarcinoma (2, 3). The pathogenesis of H. pylori-associated Gastric cancer (GC) involves a complex interplay between microbial virulence factors, host genetic mutations and immune responses, and other exposure factors, such as high-nitrite diets, culminating in cascade of inflammatory events that can lead to severe gastric pathology.
Upon infection, H. pylori trigger a robust proinflammatory response, resulting in chronic gastritis. Persistent inflammation can induce gastric epithelial cell apoptosis and mucosal atrophy, setting the stage for compensatory hyperplasia and dysregulated cell differentiation. This altered cellular landscape often progresses to intestinal metaplasia, a precursor lesion that may further evolve into dysplasia, carcinoma in situ, and ultimately invasive adenocarcinoma (4–6). Leveraging cutting-edge Artificial intelligence (AI) tools to decipher H. pylori’s antigenic landscape is crucial for developing next-generation precision vaccines to combat H. pylori-associated malignancies.
2 H. pylori: unravling the molecular arsenal of a gastric pathogen
2.1 Decoding H. pylori’s molecular arsenal: implications for precision medicine and targeted therapy in gastric cancer
H. pylori’s pathogenicity is primarily driven by two key virulence factors: the vacuolating cytotoxin A (VacA) and the cag pathogenicity island (cagPAI)-encoded type IV secretion system (T4SS), along with its effector protein CagA (2, 7). Upon infection, these factors orchestrate a complex immune response characterized by a mixed Th1/Th17-mediated pro-inflammatory cascade. The T4SS facilitates the translocation of CagA into gastric epithelial cells (8–10) triggering chronic inflammation and activating pro-inflammatory signaling pathways, including nuclear factor-κB (NF-κB) and signal transducer and activator of transcription 3 (STAT3) (11). Concurrently, VacA activates the epidermal growth factor receptor (EGFR), initiating AKT and WNT/β-catenin signaling cascades that promote cell proliferation and oncogenic transformation (12, 13). These molecular mechanisms collectively drive H. pylori-induced gastritis and gastric carcinogenesis, which have emerged as key focal points in the landscape of precision medicine and targeted therapy.
Recent studies have unveiled the profound impact of H. pylori infection on the gastrointestinal microbiome. Infected individuals exhibit a distinct dysbiosis characterized by an enrichment of Proteobacteria, particularly within the Epsilonproteobacteria class, Campylobacterales order and Helicobacteraceae family, and Helicobacter genus. This alteration in microbial community structure is closely associated with H. pylori colonization (14–16) and its ability to modulate pH through urease activity. The resulting neutral microenvironment facilitates further microbial imbalances, potentially contributing to carcinogenesis.
Intriguingly, the oncogenic H. pylori extend beyond gastric malignancies. Emerging evidence suggests associations with colorectal, pancreatic, and hepatobiliary cancers (17–21), underscoring the far-reaching implications of this pathogen in gastrointestinal oncology. These findings highlight the need for a comprehensive understanding of H. pylori’s systemic effects and emphasize the importance of effective eradication strategies, including targeted therapy, in cancer prevention.
3 Global gastric cancer trends: mapping the H. pylori connection
Gastric Cancer represents a significant global health burden, ranking as the fifth most common cancer worldwide and a leading cause of cancer-related mortality. Analysis of data from The Global Cancer Observatory database (https://gco.iarc.fr/en/projects) reveals striking geographical variations in the age-standardized incidence rate (ASIR) of GC, with particularly high rates observed in Asia and neighboring regions. Notably, countries such as Japan (27.6/100,000), Azerbaijan (16.56/100,000), and China (13.72/100,000) exhibit elevated ASIRs, which are strongly associated with H. pylori infection and dietary factors.
The etiology of GC is multifactorial, with H. pylori infection identified as primary risk factor, alongside alcohol consumption and smoking. Dietary habits, particularly consumption of high-nitrite salt-preserved foods and low-fruit intake (22), like South Korea (26.98/100,000) and North Korea (13.91/100,000). In contrast, regions with lower H. pylori infection rates, such as North America and Europe, demonstrate comparatively lower GC incidence, exemplified by Finland (3.99/100,000) and Canada (4.65/100,000) (Figure 1).
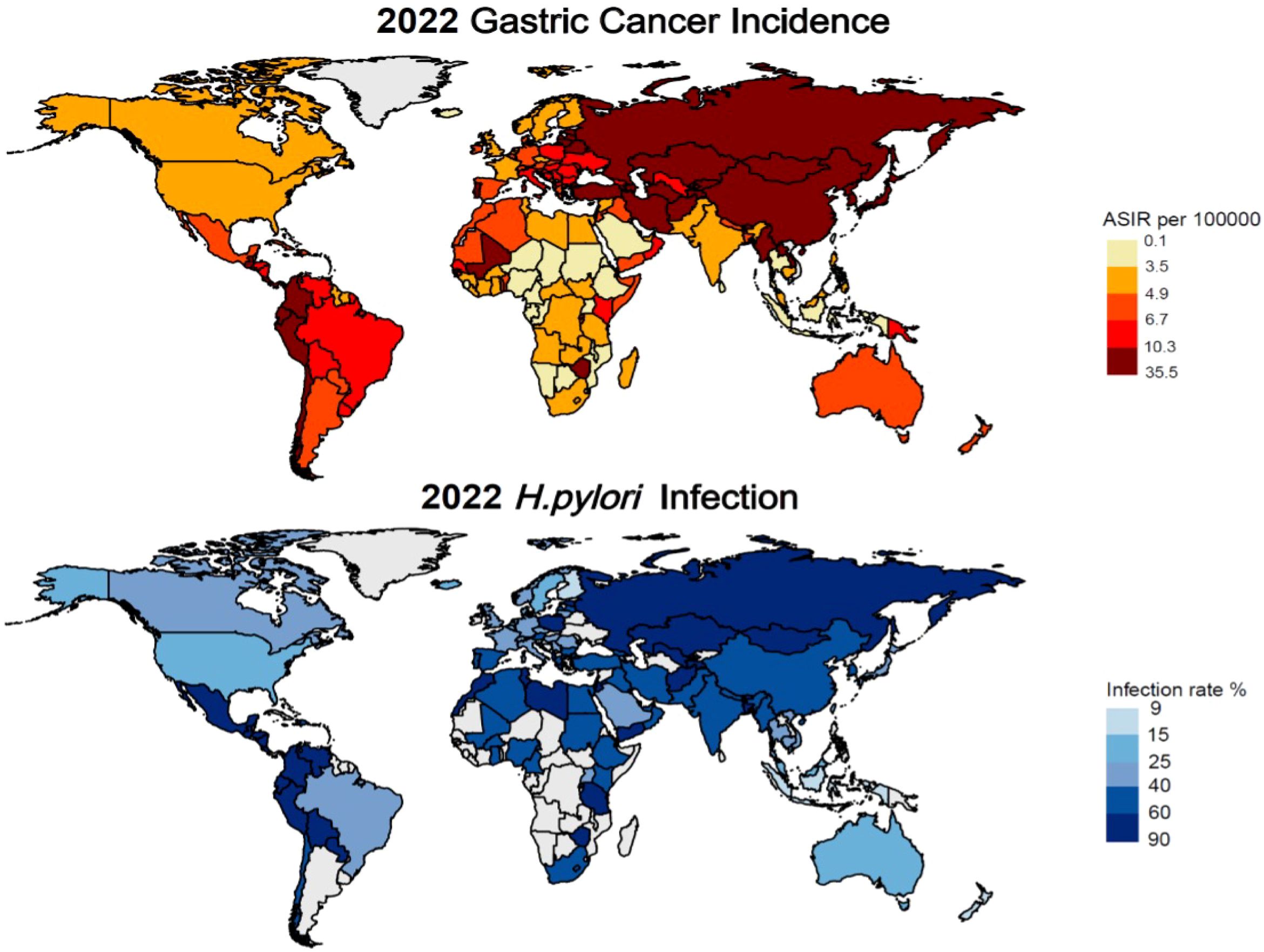
Figure 1. Latest global prevalence of gastric cancer incidence and Helicobacter pylori infection (as of 2022).
A comprehensive study examining H. pylori infection prevalence and gastric cancer incidence from 1980 to 2022 revealed significant regional variations. The highest infection rates among adults are observed in Africa and the Eastern Mediterranean, while lower rates were reported in Asia and Europe. Countries such as Jordan (88.6%), Ecuador (85.7%), and Guatemala (86.6%) exhibit the highest prevalence of infection. Conversely, countries like Finland (9.1%), and New Zealand (15.0%) demonstrate markedly lower infection rates (23, 24).
The globally prevalence of H. pylori and its role in initiating inflammatory responses that can lead to carcinogenesis underscore the critical need for effective management strategies. This review provides a comprehensive overview of current treatment approaches and explores innovative therapeutic strategies aimed at mitigating H. pylori infection and its associated health risk.
4 Rethinking H. pylori eradication: beyond antibiotics
Eradication of H. pylori remain the cornerstone of gastric disease prevention. Current treatment strategies primarily rely on empirical antibiotic regimens, with triple therapy as the gold standard. Despite achieving high eradication rates (>90%) in some populations (25), the global surge in antibiotic resistance poses a significant challenge. Triple therapy is no longer considered the gold standard in many regions (26). This escalating threat has spurred the development of more robust approaches, including quadruple therapy and adjunctive treatments, to combat this resilient pathogen.
4.1 Triple and quadruple therapies
H. pylori eradication strategies have evolved significantly since the introduction of proton pump inhibitor (PPI)-based triple therapy in 1997. The regimen, combining a PPI with amoxicillin and either clarithromycin, metronidazole, or levofloxacin, has been the global standard for over two decades (27). However, its efficacy has waned due to increasing antibiotic resistance particularly in China where resistance rates to metronidazole, clarithromycin, and levofloxacin have reached 78.2%, 22.1%,19.2%, respectively (28).
In response to this challenge, bismuth (subcitrate/subsalicylate)-based quadruple therapy (BQT) has emerged as a potent alternative. Comprises a PPI, bismuth, tetracycline HCl, and metronidazole, BQT achieves eradication rate between 90% and 95% when administered for 14 days (29). Recent guidelines now recommend BQT as first-line treatment in regions with high clarithromycin resistance (30, 31). Notably, a pediatric study reported a 95% eradication rate with excellent compliance using a 10-day BQT regimen (32). Despite its efficacy, BQT is not without limitations. Approximately 50% of patients experience side effects such as nausea and abdominal pain (29). Moreover, non-bismuth quadruple therapies, while effective, risk unnecessary antibiotic exposure. The optimal choice of eradication therapy should be guided by regional antibiotic susceptibility patterns, accessibility, and cost-effectiveness considerations.
Non-bismuth quadruple therapy, encompassing concomitant and sequential regimens, combines PPI, amoxicillin, metronidazole (or tinidazole), and clarithromycin, administered over a 14-day course (33). However, this approach faces challenges in regions with high antibiotic resistance. For instance, in Houston, clarithromycin and metronidazole resistance rate of 15% and 25%, respectively, render traditional triple therapies ineffective (34). Moreover, the potential for unnecessary antibiotic exposure raises concerns about antimicrobial stewardship, leading to recommendations against its use as a primary first-line treatment (35, 36). Probiotics have emerged as a promising adjunct in the treatment of H. pylori infections (37, 38), offering potential benefits in modulating the gut microbiome and enhancing host immune responses (39). However, the precise mechanisms underlying the role of probiotics in preventing H. pylori infection remain incompletely understood. Recent studies have shed light on potential mechanisms, suggesting that probiotics can stimulate the release of inflammatory cytokines, activate the NF-κB pathway, and induce the production of IL-8 (40). Additionally, certain probiotic strains, particularly lactic acid bacteria, have been shown to inhibit H. pylori colonization through the synthesis of bacteriocin-related antimicrobial substances and secreting of inhibitory compounds, including organic acids, hydrogen peroxide, and carbon dioxide. Despite these promising findings, it is important to note that the role of probiotics in H. pylori eradication is primarily adjunctive, enhancing the efficacy of antibiotic therapy rather than serving as a standalone treatment (41, 42). This limitation underscores the critical need for novel preventive strategies, particularly in light of the growing concern surrounding antibiotic resistance. Future research should focus on elucidating the complex interactions between probiotics, H. pylori, and the host microbiome, with the goal of developing more effective and targeted approaches to prevent and treat H. pylori infections.
4.2 Regional Variations in H. pylori Antibiotic Resistance: Insights from a Comprehensive Chinese Study
A large-scale study investigating the prevalence of H. pylori infection and antibiotic resistance patterns across China has revealed significant regional disparities, providing crucial insights for tailoring treatment strategies (43). This comprehensive analysis, encompassing 52 municipalities across 26 provinces and involving 12,902 participants, utilized quantitative polymerase chain reaction (qPCR) to assess resistance to two key antibiotics: clarithromycin and levofloxacin.
The study uncovered an overall H. pylori infection rate of 27.08% among the Chinese population, with alarming resistance rates of 50.83% for clarithromycin and 47.17% for levofloxacin. Notably, antibiotic resistance patterns exhibited significant variations across demographic factors and geographical regions:
- Gender differences: Women showed higher resistance rates (53.85% for clarithromycin, 49.01% for levofloxacin) compared to men (45.48% and 43.90%, respectively), possibly due to more frequent antibiotic use in treating gynecological infections.
- Age-related trends: Middle-aged and older individuals demonstrated elevated resistance rates (54.58% for clarithromycin, 54.54% for levofloxacin), surpassing the overall resistance rate. This trend may be attributed to increased cumulative antibiotic exposure over time.
- Geographical disparities: Northern provinces, such as Heilongjiang (77.08%) and Jilin (77.91%), exhibited markedly higher resistance rates compared to southern provinces like Hunan (27.78%). These regional variations likely reflect differences in socioeconomic conditions, sanitation practices, and antibiotic usage patterns across China.
These findings underscore the critical importance of considering regional and demographic factors when developing targeted strategies for H. pylori eradication. The observed disparities in antibiotic resistance highlight the need for tailored treatment approaches and emphasize the urgency of implementing more stringent antibiotic stewardship programs, particularly in high-resistance regions. Future research should focus on elucidating the underlying causes of these regional variations and developing innovative strategies to combat the growing challenge of antibiotic resistance in H. pylori management (43).
5 Challenges in eradicating H. pylori: rethinking gastric cancer prevention
Antibiotic resistance poses a formidable challenge to H. pylori eradication and gastric cancer prevention. The resistance primarily stems from point mutations in antimicrobial-associated genes (e.g., rrn23S, pbp-1, rdxA.) and the presence of drug efflux pumps. For instance, mutations in the 23S rRNA can reduce clarithromycin binding affinity, while efflux pumps actively expel antibiotics, maintaining suboptimal intracellular drug concentrations (44, 45). Additionally, H. pylori eradication efficacy is intricately linked to colonization density and virulence factors like cagA and vacA. This pathogen induces gastrointestinal microbiota dysbiosis, altering the host’s susceptibility (46, 47). Notably, Epstein-Barr virus (EB Virus) and H. pylori may synergistically exacerbate gastric inflammation, potentially accelerating carcinogenesis (48). These findings underscore the complex interplay between pathogen, host, and microbiome in H. pylori-associated gastric pathology, highlighting the need for multifaceted therapeutic approaches.
Genetic predisposition plays a crucial role in GC susceptibility. Mutations in the CDH1 and BRCA1/BRCA2 genes significantly increase risk (49). High mutation rates in TP53 (~43%), TTN (~42.5%), and MUC16 (~25.2%) underscore their potential as key drivers of GC (50–52). Additionally, lifestyle factors, such as smoking, alcohol consumption, high-salt/nitrite diets can impede H. pylori eradication and promote GC development (53–55) (Figure 2).
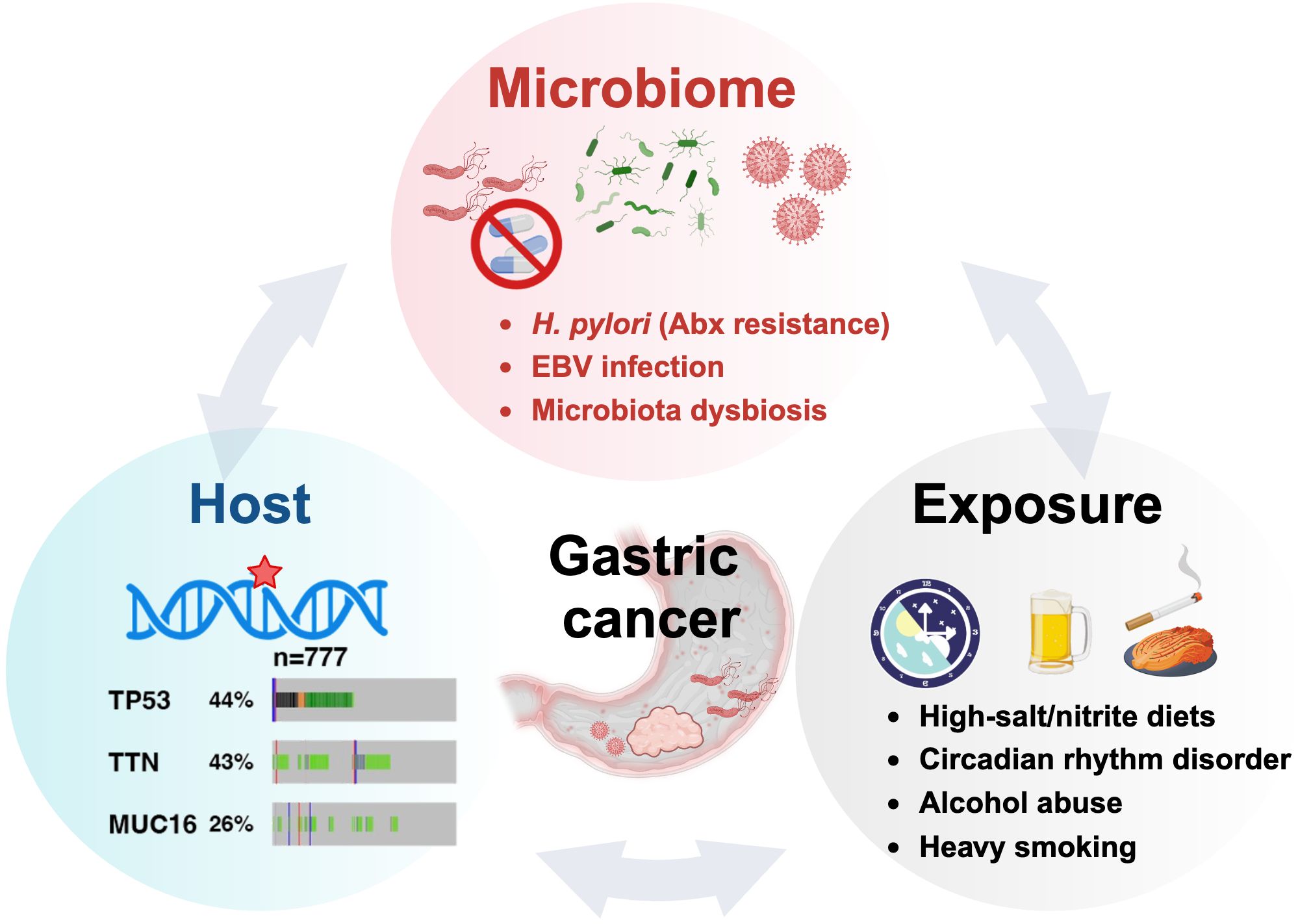
Figure 2. The oncogenic H. pylori interplays with the host and exposure factors in gastric carcinogenesis.
The multifaceted nature of H. pylori infection and its associated cancer risk necessitates innovative therapeutic approaches. Current strategies often fall short of complete eradication, highlighting the urgent need for novel interventions. One promising avenue involves harnessing the host’s adaptive immune system to generate specific, long-lasting memory B and T cells, potentially leading to more efficient H. pylori elimination.
6 Molecular camouflage: how H. pylori evades immunity in gastric cancer
H. pylori infection triggers a sophisticated immune response, orchestrating a complex interplay between innate and adaptive immunity. The innate immune system, acting as the first line of defense, employs pattern recognition receptors (PRRs) on gastric epithelial cells to detect pathogen-associated molecular patterns (PAMPs) (56, 57). This recognition cascade activates NOD-like receptor (NLR) and Toll-like receptors (TLRs), initiating NF-κB signaling and promoting the release of pro-inflammatory cytokines, particularly IL-8 (58).
The adaptive immune response further refines this defense strategy. Type 2 innate lymphocytes (ILC2) secrete IL-5, stimulating B cells to produce IgA, IgG, IgM antibodies. Concurrently, the H. pylori virulence factor CagA induces dendritic cells maturation, facilitating antigens presentation to naïve T cells and their subsequent differentiation into Th1/Th17/Tfh subsets (59).
Paradoxically, H. pylori has evolved mechanisms to subvert this immune assault. By promoting the differentiation of Th17 cells toward regulatory T cells (Tregs), which secrete the anti-inflammatory cytokine IL-10, the bacterium ingeniously establishes immune tolerance, enabling its long-term persistence in the gastric niches (Figure 3) (60). This delicate balance between immune activation and evasion underscores the complexity of H. pylori pathogenesis and highlights potential targets for therapeutic intervention.
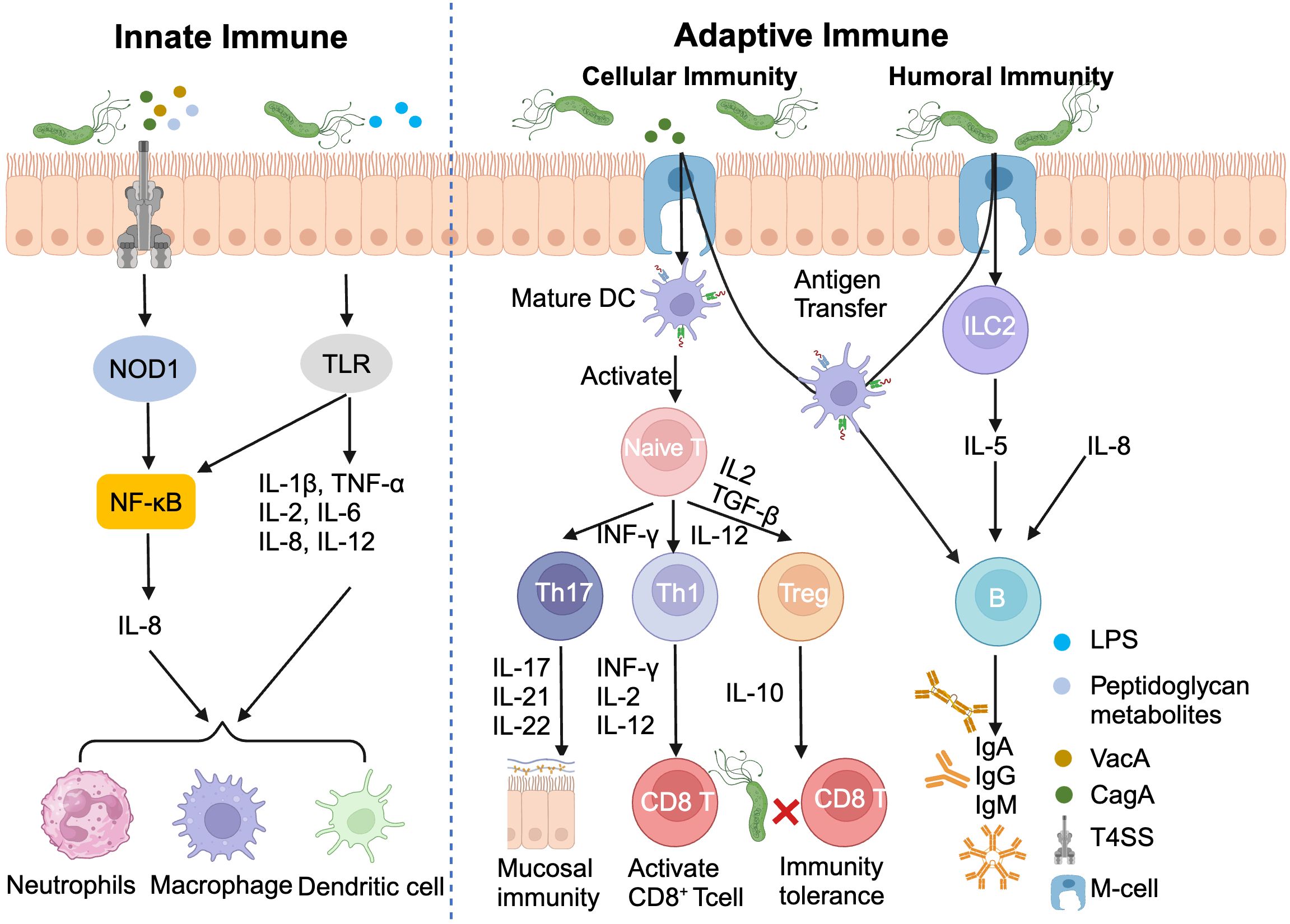
Figure 3. Helicobacter pylori infection induces innate and adaptive immunity. DC, Dendritic cell; ILC2, Type 2 innate lymphocytes; LPS, Lipopolysaccharide.
7 Harnessing host immunity in H. pylori vaccine development
The persistence of H. pylori infections, despite initial antibiotic success, underscores a critical challenge in global health. Antibiotic resistance and infection recurrence, particularly in resource-limited settings necessitate innovative strategies for sustainable eradication. Drawing parallels with the transformative impact of Human Papillomavirus (HPV) vaccines on cervical cancer prevention, the development of H. pylori vaccines emerges as a promising frontier in gastric cancer prevention (61, 62).
However, the genetic diversity of H. pylori strains presents a formidable obstacle to vaccine development. This variability, reminiscent of HPV’s subtype complexity, demands a nuanced approach to antigen selection and vaccine design (Table 1). In this context, precision medicine offers valuable insights by identifying strain-specific targets and tailoring immunological strategies accordingly.
Current vaccine candidates target an array of H. pylori antigens can enhance host immunity, despite the delivery method (oral, intranasal mucosal, subcutaneous injection) (68–70), and vaccine composition (primarily peptide vaccines and RNA vaccines) (71, 72). However, they have not yet achieved consistent, long-term protection against H. pylori infection. The immune response against H. pylori primarily orchestrated in the gastric mucosa, where reminiscent of intestinal Peyer’s patches play a crucial role (73). he recognition of bacterial virulence factors or pathogen-associated molecular patterns (PAMPs) by pattern recognition receptors, such as TLR4 or Nod1, triggers the activation of the MyD88-NF-κB pathway and other signaling cascades. This cascade leads to the release of inflammatory cytokines and the initiation of adaptive immune responses, particularly the CD4+ T-cell response.
T-cell assistance facilitates the activation and differentiation of follicular B-cells into plasma cells, which secrete IgA. Secretory IgA is pivotal in mucosal immunity, neutralizing various toxins, while IgG appears to play a less significant role in this context (74). The development of *H. pylori* vaccines have predominantly focused on subunit oral vaccines, designed to efficiently activate mucosal responses. These vaccines, containing single and highly specific peptides devoid of toxic components, theoretically should not elicit long-term intense inflammatory reactions.
Extensive research has demonstrated that oral subunit vaccines generally elicit Th1/Th17 responses and stimulate the production of secretory IgA upon entering the body, significantly enhancing pathogen clearance. However, a clinical trial in China revealed a gradual decline in the protective efficacy of oral vaccines over time, accompanied by decreasing antibody levels (75). Consequently, current research efforts are directed towards enhancing the sustained protective capacity of vaccines, which hinges on the immunogenicity of the vaccine peptides and the selection of appropriate adjuvants.
A recent breakthrough study demonstrated that an LNP-mRNA vaccine for influenza virus maintained superior lung mucosal immune responses compared to protein vaccines, facilitating the formation of germinal center follicular B-cells and memory T-cells (76). This research also highlighted the potential of LNP as a potent immunostimulatory adjuvant, not only for mRNA vaccines but also for enhancing the efficacy of various vaccine types. These findings open new avenues for vaccine development against H. pylori and other mucosal pathogens, potentially revolutionizing our approach to preventive medicine.
Recent advances in vaccine technology offer new avenues for exploration. A multi-epitope oral vaccine, incorporating four H. pylori virulence factors (Urease, NAP, HSP60, and HpaA), showed promising results in murine models, eliciting robust systemic and mucosal immune responses (77). This approach highlights the potential of tailoring comprehensive and multi-target vaccine strategies to overcome the challenges posed by H. pylori’s genetic diversity, a key component of precision therapy approaches.
The virulence of H. pylori strains is intricately linked to genetic factors, particularly the cagA and vacA genes. CagA-positive strains correlate with increased risk of peptic ulcers and gastric cancer, while vacA gene variations (s1/m1, s1/m2, s2/m2) modulate cytotoxicity and disease outcomes (78–82). These findings underscore the importance of tailoring vaccine strategies to address the molecular heterogeneity of H. pylori strains.
Nucleic acid vaccines represent a cutting-edge approach, offering the potential to induce robust humoral and cellular immunity against conserved epitopes across multiple H. pylori subtypes (83–85). This technology circumvents challenges associated with oral vaccine delivery, such as gastric acidity and mucosal immune induction, presenting a promising avenue for future research.
8 Current clinical trials and challenges in H. pylori vaccine development
The urgent need to combat H. pylori infection has driven the development of various vaccine candidates (Table 2). Despite extensive efforts, significant success remains elusive. Clinical studies primarily assess vaccine efficacy through measurements of specific antibody levels and post-vaccination adverse reactions. However, a critical gap exists in evaluating the vaccines’ ability to prevent H. pylori infection, limiting our understanding of their real-world effectiveness (70). A notable Phase I/II clinical trial conducted by Peter et al. investigated an oral vaccine containing three recombinant H. pylori antigens: VacA, CagA, and neutrophil-activating protein (NAP). While vaccinated volunteers exhibited significantly higher levels of H. pylori-specific antibodies compared to the control group, the vaccine failed to demonstrate superior infection prevention. Surprisingly, over half of the control group experienced spontaneous infection clearance, highlighting the complexity of H. pylori immunology and the challenges in vaccine development.
The limited efficacy of current vaccines can be attributed to several factors. H. pylori exhibits considerable genetic diversity, particularly in its virulence factors, which display high antigenic variability. Antigens with low conservation between different H. pylori strains are prone to evading immune recognition (86). Moreover, virulence factors targeted by vaccines may be lost following vaccination, rendering the immune response ineffective. The high genetic variability of H. pylori enables rapid adaptation to the selective pressure imposed by vaccination, posing a significant obstacle to vaccine efficacy. Enhancing vaccine immunogenicity while mitigating immune escape mechanisms remains a central challenge in developing effective immunization strategies against H. pylori (87).
The development of universal vaccines with multi-epitope tailored to multi-pathogenic H. pylori subtypes holds significant promise. However, realizing this potential requires overcoming the complexities of antigen variability and immunogenicity assessment. Leveraging advanced bioinformatics and machine learning or Artificial Intelligence algorithms may accelerate the design and optimization of next-generation H. pylori vaccines, potentially revolutionizing our approach to gastric cancer prevention through precision medicine and targeted therapy strategies.
9 AI breakthroughs redefine neoantigen-based anti-bacterial vaccines development
9.1 Computational tools in vaccine design
AI has revolutionized vaccine development, enhanced efficacy and accelerating timelines. By targeting microscopic molecules, modern vaccines offer advantages over traditional approaches, with AI methods playing a crucial role in their design (92). These methods reduced the number of proteins to be studied and was able to recognize small amounts of antigens present, enhance stability, streamlining the development process and offering new insights for precision medicine (93).
Reverse vaccinology (RV), introduced by Rino Rappuoli in 2001, leverages genomic data to identify potential vaccine targets (94). This approach has spawned numerous computational tools, including PanRV (95), VaxiJen (96), Vaxign (97), Antigenic (98) etc. NERVE, the pioneering bacterial vaccine prediction tool based on RV principles, considers factors such as antigen foreignness, adhesion status, and localization to improve vaccine safety and efficacy (99). Machine learning has further advanced vaccine development. Vaxign-ML, utilizing extreme gradient boosting, incorporates 509 features and was trained on a comprehensive dataset of bacterial protective antigens (100). Following this review provides a list of these methods from 2006 to 2021 and their key characteristics (Table 3). This approach exemplifies the potential of AI in vaccine design, offering rapid and accurate predictions of vaccine candidates.
Despite promising advancements, challenges remain. The limited availability of training data and the complexity of immunogenicity factors, including MHC affinity and TCR avidity, necessitate further refinement of these tools (101). However, these tools often lack consideration for these finer details during their design. For bacterial immunity, MHC class II affinity prediction is particularly crucial yet complex (102–104). NetMHCIIpan-4.0 represents a significant advancement in MHC class II binding affinity prediction. It is based on NNAlign MA machine learning framework, adopting deconvolution method and pseudo-labeling strategy (105). In detail, NetMHCIIpan-4.0 leverages binding affinity (BA) data from single MHC allele sequences to perform deconvolution, identifying anchor positions and amino acid preferences, which define binding motifs (105). Subsequently, it generates single-allele specific pseudo-labels from spectrometry-eluted ligand (EL) data (multi-allele MHC binding). The modeling method allowed NetMHCIIpan-4.0 to be trained on larger datasets, learning more comprehensive information (105). Moreover, compared to single-allele prediction tools, NetMHCIIpan-4.0 has been trained on data covering a total of 116 different MHC II molecules, which significantly enhances its overall generalization capability and allows for accurate MHC II affinity predictions (105). Recently, most tools have applied deep learning models, such as Convolutional Neural Networks, Transformers (106), and BERT (107), to project protein sequences into high-dimensional spaces, promise even more accurate predictions of MHC class II binding motifs. The list of tools predicting MHC class II affinity was as follow (Table 4).
As these computational methods evolve, incorporating increasingly complex biological factors, they pave the way for a new generation of vaccines with enhanced safety, specificity, and immunogenicity. This convergence of biology, computer science and precision medicine herald a new era in vaccine development, offering hope for rapid responses to emerging pathogens and improved global health outcomes through both vaccines and targeted therapies.
9.2 AI and bioinformatics applications in vaccine candidate discovery
To date, thousands of vaccine candidates have been developed using advanced technologies to target a wide range of lethal pathogens (108). In recent years, artificial intelligence (AI) and bioinformatics have emerged as pivotal tools in vaccine design, particularly for COVID-19. The integration of these technologies facilitated the rapid development of COVID-19 vaccines in under a year, significantly contributing to epidemic prevention and control—an efficiency unattainable through traditional methods (93).
Modern vaccine design now comprehensively creates safe, stable, and effective vaccines by determining epitopes, utilizing epitope immunoinformatics, and assessing vaccine epitope structure and stability. Reverse vaccinology (RV) is employed to identify genes with potential epitopes, with tools such as Vaxign ML being widely used (100, 109). BLASTp comparisons of epitope sequences against human genes help exclude sequences with homology to human epitopes, mitigating the risk of autoimmune reactions post-vaccination (110).
To identify suitable epitope antigens, it is crucial to utilize appropriate epitope immunoinformatics analysis tools to evaluate the potential of epitopes to elicit robust immune responses. Tools like NetMHCIIpan-4.0 and Epitopemap (111), aid in understanding the genetic polymorphisms of major histocompatibility complex (MHC) classes I and II in the target population, as well as predicting cytotoxic and helper T lymphocyte epitopes. Some studies employ I-TASSER (112) to elucidate the conformational characteristics of epitopes and to identify antigenic epitopes with enhanced immunogenicity.
The insights gained from these vaccine development experiences serve as valuable references for the development of H. pylori vaccines, potentially accelerating the process and improving efficacy.
9.3 Limitations of AI-designed vaccines
AI and bioinformatics-based vaccine design faces several key limitations. Firstly, epitope antigens are typically confined to protein-encoding genes, whereas traditional approaches can target a broader range of biological entities, including polysaccharides (113). Secondly, certain epitope antigens in viral proteins may evade human immune recognition, failing to elicit an effective immune response. Thirdly, while AI-designed vaccines are often evaluated based on B and T cell immune responses, such as specific antigen antibody levels, elevated antibodies do not always translate to strong preventive or therapeutic effects, as demonstrated by Peter et al. (70). Lastly, validating the efficacy of vaccines for certain pathogens is challenging due to the difficulty in selecting appropriate animal models (93).
Despite AI’s potential to expedite H. pylori vaccine development, its application faces hurdles. AI model training requires extensive, high-quality data, but biological experiments are typically low-throughput and time-intensive. For instance, the T cell activation experiments in the IEDB are insufficient for robust AI model training (114). High-throughput experiments, such as IP-MS studies on MHC II (including HLA-DR, HLA-DQ, and HLA-DP) under various conditions, can significantly expand training sets, potentially surpassing one million data points for MHC II affinity prediction (105). Integrating high and low-throughput biological experimental data could further enhance AI model performance.
Bias presents another significant challenge in AI model training. The tendency of researchers to prioritize positive results over negative ones leads to discrepancies between training and true distributions. This issue can be addressed through pseudo-label training, enabling AI models to learn within the true distribution (105).
Furthermore, the prevalence of weak functional molecules results in a severe imbalance between negative and positive results in unlabeled samples (115). This imbalance compromises the representativeness of negative data and hinders effective learning of positive data patterns when large amounts of negative data are introduced, ultimately impeding AI model training. Common strategies to address this include resampling to adjust the ratio of training data or applying weighted corrections.
10 Perspectives: precision therapy and AI-driven vaccine design
The persistent challenge of H. pylori infection, a Class I carcinogen, contributes to significantly global gastrointestinal diseases. Despite extensive research efforts, conventional therapeutic approaches have been hampered by antibiotic resistance and recurrence, underscoring the urgent need for innovative strategies. This review illuminates the potential of next-generation H. pylori vaccines in bolstering host immunity and mitigating infection-associated gastric cancer risk (1).
While recent advancements in understanding immune mechanisms and inflammatory responses have provided valuable insights, their translation into effective preventive vaccines remains elusive. Although some clinical trials have shown promise, significant hurdles persist, including suboptimal efficacy and adverse effect. We propose a paradigm-shifting approach rooted in precision medicine: the development of tailored, multiepitope antigens targeting diverse H. pylori pathogenic subtypes. By leveraging cutting-edge artificial intelligence tools, we envision the creation of highly specific H. pylori neoantigen vaccines for gastric cancer prevention. This innovative strategy not only promises to address the limitations of current triple-antibiotics therapies, but also has the potential to revolutionize our approach to oncogenic pathogens more broadly. The integration of AI-driven vaccine design with precision therapy concepts opens new avenues for personalized interventions, potentially offering a transformative solution to the global burden of H. pylori-associated diseases.
As we stand at the cusp of this exciting frontier, the convergence of AI, immunology, and precision medicine holds immense promise for reshaping the landscape of infectious disease management and cancer prevention. This approach may serve as a blueprint for tackling other persistent pathogen-associated carcinogenesis, heralding a new era of tailored therapeutic strategies with far-reaching implications for global health.
Author contributions
ZT: Formal analysis, Visualization, Writing – original draft, Writing – review & editing, Data curation, Project administration, Resources, Validation. YW: Data curation, Formal analysis, Project administration, Resources, Visualization, Writing – original draft, Writing – review & editing, Validation. JZL: Data curation, Formal analysis, Project administration, Resources, Writing – original draft, Software. JPL: Data curation, Formal analysis, Writing – original draft, Conceptualization, Funding acquisition, Investigation, Supervision, Visualization, Writing – review & editing.
Funding
The author(s) declare financial support was received for the research, authorship, and/or publication of this article. This work was financially supported by the National Natural Science Foundation of China (82373257 and 21HAA02056). This work was also supported by grants from the Young Talents Program of Sun Yat-sen University Cancer Center (YTP-SYSUCC-0056).
Conflict of interest
The authors declare that the research was conducted in the absence of any commercial or financial relationships that could be construed as a potential conflict of interest.
The handling editor JK declared a shared parent affiliation with the authors at the time of review.
Publisher’s note
All claims expressed in this article are solely those of the authors and do not necessarily represent those of their affiliated organizations, or those of the publisher, the editors and the reviewers. Any product that may be evaluated in this article, or claim that may be made by its manufacturer, is not guaranteed or endorsed by the publisher.
Abbreviations
H. pylori, Helicobacter pylori; AI, Artificial Intelligence; VacA, vacuolating cytotoxin A; cagPAI, cag pathogenicity island; T4SS, type IV secretion system; GC, Gastric Cancer; ASIR, age-standardized incidence rate; BQT, bismuth-based quadruple therapy; PRRs, pattern recognition receptors; PAMP, pathogen-associated molecular patterns; HPV, Human Papillomavirus; RV, Reverse vaccinology.
References
1. Maixner F, Krause-Kyora B, Turaev D, Herbig A, Hoopmann MR, Hallows JL, et al. The 5300-year-old Helicobacter pylori genome of the Iceman. Science. (2016) 351:162–5. doi: 10.1126/science.aad2545
2. Hatakeyama M. Structure and function of Helicobacter pylori CagA, the first-identified bacterial protein involved in human cancer. Proc Jpn Acad Ser B Phys Biol Sci. (2017) 93:196–219. doi: 10.2183/pjab.93.013
3. Parsonnet J, Friedman GD, Vandersteen DP, Chang Y, Vogelman JH, Orentreich N, et al. Helicobacter pylori infection and the risk of gastric carcinoma. N Engl J Med. (1991) 325:1127–31. doi: 10.1056/NEJM199110173251603
4. Cahill RJ, Kilgallen C, Beattie S, Hamilton H, O'Morain C. Gastric epithelial cell kinetics in the progression from normal mucosa to gastric carcinoma. Gut. (1996) 38:177–81. doi: 10.1136/gut.38.2.177
5. Poh AR, O'Donoghue RJ, Ernst M, Putoczki TL. Mouse models for gastric cancer: Matching models to biological questions. J Gastroenterol Hepatol. (2016) 31:1257–72. doi: 10.1111/jgh.13297
6. Uemura N, Okamoto S, Yamamoto S, Matsumura N, Yamaguchi S, Yamakido M, et al. Helicobacter pylori infection and the development of gastric cancer. N Engl J Med. (2001) 345:784–9. doi: 10.1056/NEJMoa001999
7. Foegeding NJ, Caston RR, McClain MS, Ohi MD, Cover TL. An overview of helicobacter pylori VacA toxin biology. Toxins (Basel). (2016) 8. doi: 10.3390/toxins8060173
8. Censini S, Lange C, Xiang Z, Crabtree JE, Ghiara P, Borodovsky M, et al. cag, a pathogenicity island of Helicobacter pylori, encodes type I-specific and disease-associated virulence factors. Proc Natl Acad Sci U.S.A. (1996) 93:14648–53. doi: 10.1073/pnas.93.25.14648
9. Shi Y, Liu XF, Zhuang Y, Zhang JY, Liu T, Yin Z, et al. Helicobacter pylori-induced Th17 responses modulate Th1 cell responses, benefit bacterial growth, and contribute to pathology in mice. J Immunol. (2010) 184:5121–9. doi: 10.4049/jimmunol.0901115
10. Stein M, Rappuoli R, Covacci A. Tyrosine phosphorylation of the Helicobacter pylori CagA antigen after cag-driven host cell translocation. Proc Natl Acad Sci U S A. (2000) 97:1263–8. doi: 10.1073/pnas.97.3.1263
11. Mejias-Luque R, Zoller J, Anderl F, Loew-Gil E, Vieth M, Adler T, et al. Lymphotoxin beta receptor signalling executes Helicobacter pylori-driven gastric inflammation in a T4SS-dependent manner. Gut. (2017) 66:1369–81. doi: 10.1136/gutjnl-2015-310783
12. Tabassam FH, Graham DY, Yamaoka Y. Helicobacter pylori activate epidermal growth factor receptor- and phosphatidylinositol 3-OH kinase-dependent Akt and glycogen synthase kinase 3beta phosphorylation. Cell Microbiol. (2009) 11:70–82. doi: 10.1111/j.1462-5822.2008.01237.x
13. Wang F, Qu N, Peng J, Yue C, Yuan L, Yuan Y. CagA promotes proliferation and inhibits apoptosis of GES-1 cells by upregulating TRAF1/4-1BB. Mol Med Rep. (2017) 16:1262–8. doi: 10.3892/mmr.2017.6757
14. Dash NR, Khoder G, Nada AM, Al Bataineh MT. Exploring the impact of Helicobacter pylori on gut microbiome composition. PLoS One. (2019) 14:e0218274. doi: 10.1371/journal.pone.0218274
15. Guo Y, Zhang Y, Gerhard M, Gao JJ, Mejias-Luque R, Zhang L, et al. Effect of Helicobacter pylori on gastrointestinal microbiota: a population-based study in Linqu, a high-risk area of gastric cancer. Gut. (2020) 69:1598–607. doi: 10.1136/gutjnl-2019-319696
16. Kienesberger S, Cox LM, Livanos A, Zhang XS, Chung J, Perez-Perez GI, et al. Gastric helicobacter pylori infection affects local and distant microbial populations and host responses. Cell Rep. (2016) 14:1395–407. doi: 10.1016/j.celrep.2016.01.017
17. Avenaud P, Marais A, Monteiro L, Le Bail B, Bioulac Sage P, Balabaud C, et al. Detection of Helicobacter species in the liver of patients with and without primary liver carcinoma. Cancer. (2000) 89:1431–9. doi: 10.1002/1097-0142(20001001)89:7<1431::AID-CNCR4>3.0.CO;2-5
18. Backert S, Tegtmeyer N, Oyarzabal OA, Osman D, Rohde M, Grutzmann R, et al. Unusual Manifestation of Live Staphylococcus saprophyticus, Corynebacterium urinapleomorphum, and Helicobacter pylori in the Gallbladder with Cholecystitis. Int J Mol Sci. (2018) 19. doi: 10.3390/ijms19071826
19. Nilsson HO, Stenram U, Ihse I, Wadstrom T. Helicobacter species ribosomal DNA in the pancreas, stomach and duodenum of pancreatic cancer patients. World J Gastroenterol. (2006) 12:3038–43. doi: 10.3748/wjg.v12.i19.3038
20. Risch HA, Yu H, Lu L, Kidd MS. ABO blood group, Helicobacter pylori seropositivity, and risk of pancreatic cancer: a case-control study. J Natl Cancer Inst. (2010) 102:502–5. doi: 10.1093/jnci/djq007
21. Varon C, Azzi-Martin L, Khalid S, Seeneevassen L, Menard A, Spuul P. Helicobacters and cancer, not only gastric cancer? Semin Cancer Biol. (2022) 86. doi: 10.1016/j.semcancer.2021.08.007
22. Salvatori S, Marafini I, Laudisi F, Monteleone G, Stolfi C. Helicobacter pylori and gastric cancer: pathogenetic mechanisms. Int J Mol Sci. (2023) 24. doi: 10.3390/ijms24032895
23. Chen YC, Malfertheiner P, Yu HT, Kuo CL, Chang YY, Meng FT, et al. Global prevalence of helicobacter pylori infection and incidence of gastric cancer between 1980 and 2022. Gastroenterology. (2024) 166:605–19. doi: 10.1053/j.gastro.2023.12.022
24. Dicken BJ, Bigam DL, Cass C, Mackey JR, Joy AA, Hamilton SM. Gastric adenocarcinoma: review and considerations for future directions. Ann Surg. (2005) 241:27–39. doi: 10.1097/01.sla.0000149300.28588.23
25. Graham DY. Helicobacter pylori update: gastric cancer, reliable therapy, and possible benefits. Gastroenterology. (2015) 148:719–31.e3. doi: 10.1053/j.gastro.2015.01.040
26. Malfertheiner P, Megraud F, Rokkas T, Gisbert JP, Liou JM, Schulz C, et al. Management of Helicobacter pylori infection: the Maastricht VI/Florence consensus report. Gut. (2022) 71:1724–62. doi: 10.1136/gutjnl-2022-327745
27. Savoldi A, Carrara E, Graham DY, Conti M, Tacconelli E. Prevalence of antibiotic resistance in helicobacter pylori: A systematic review and meta-analysis in world health organization regions. Gastroenterology. (2018) 155:1372–82.e17. doi: 10.1053/j.gastro.2018.07.007
28. Zhong Z, Zhang Z, Wang J, Hu Y, Mi Y, He B, et al. A retrospective study of the antibiotic-resistant phenotypes and genotypes of Helicobacter pylori strains in China. Am J Cancer Res. (2021) 11:5027–37.
29. Graham DY, Lee SY. How to effectively use bismuth quadruple therapy: the good, the bad, and the ugly. Gastroenterol Clin North Am. (2015) 44:537–63. doi: 10.1016/j.gtc.2015.05.003
30. Cheon JH, Kim N, Lee DH, Kim JW, Hwang JH, Park YS, et al. Trial of moxifloxacin-containing triple therapy after initial and second-line treatment failures for Helicobacter pylori infection. Korean J Gastroenterol. (2005) 45:111–7.
31. Suo B, Tian X, Zhang H, Lu H, Li C, Zhang Y, et al. Bismuth, esomeprazole, metronidazole, and minocycline or tetracycline as a first-line regimen for Helicobacter pylori eradication: A randomized controlled trial. Chin Med J (Engl). (2023) 136:933–40. doi: 10.1097/CM9.0000000000002629
32. Manfredi M, Gargano G, Gismondi P, Ferrari B, Iuliano S. Therapeutic eradication choices in Helicobacter pylori infection in children. Therap Adv Gastroenterol. (2023) 16:17562848231170052. doi: 10.1177/17562848231170052
33. Graham DY, Lee YC, Wu MS. Rational Helicobacter pylori therapy: evidence-based medicine rather than medicine-based evidence. Clin Gastroenterol Hepatol. (2014) 12:177–86.e3. doi: 10.1016/j.cgh.2013.05.028
34. Wu JY, Liou JM, Graham DY. Evidence-based recommendations for successful Helicobacter pylori treatment. Expert Rev Gastroenterol Hepatol. (2014) 8:21–8. doi: 10.1586/17474124.2014.859522
35. Graham DY, Dore MP, Lu H. Understanding treatment guidelines with bismuth and non-bismuth quadruple Helicobacter pylori eradication therapies. Expert Rev Anti Infect Ther. (2018) 16:679–87. doi: 10.1080/14787210.2018.1511427
36. Tong JL, Ran ZH, Shen J, Xiao SD. Sequential therapy vs. standard triple therapies for Helicobacter pylori infection: a meta-analysis. J Clin Pharm Ther. (2009) 34:41–53. doi: 10.1111/j.1365-2710.2008.00969.x
37. Francavilla R, Lionetti E, Castellaneta SP, Magista AM, Maurogiovanni G, Bucci N, et al. Inhibition of Helicobacter pylori infection in humans by Lactobacillus reuteri ATCC 55730 and effect on eradication therapy: a pilot study. Helicobacter. (2008) 13:127–34. doi: 10.1111/j.1523-5378.2008.00593.x
38. Ryan KA, Jayaraman T, Daly P, Canchaya C, Curran S, Fang F, et al. Isolation of lactobacilli with probiotic properties from the human stomach. Lett Appl Microbiol. (2008) 47:269–74. doi: 10.1111/j.1472-765x.2008.02416.x
39. Losurdo G, Cubisino R, Barone M, Principi M, Leandro G, Ierardi E, et al. Probiotic monotherapy and Helicobacter pylori eradication: A systematic review with pooled-data analysis. World J Gastroenterol. (2018) 24:139–49. doi: 10.3748/wjg.v24.i1.139
40. Perdigon G, Medina M, Vintini E, Valdez JC. Intestinal pathway of internalisation of lactic acid bacteria and gut mucosal immunostimulation. Int J Immunopathol Pharmacol. (2000) 13:141–50.
41. Nabavi-Rad A, Sadeghi A, Asadzadeh Aghdaei H, Yadegar A, Smith SM, Zali MR. The double-edged sword of probiotic supplementation on gut microbiota structure in Helicobacter pylori management. Gut Microbes. (2022) 14:2108655. doi: 10.1080/19490976.2022.2108655
42. Ismail NI, Nawawi KNM, Hsin DCC, Hao KW, Mahmood N, Chearn GLC, et al. Probiotic containing Lactobacillus reuteri DSM 17648 as an adjunct treatment for Helicobacter pylori infection: A randomized, double-blind, placebo-controlled trial. Helicobacter. (2023) 28:e13017. doi: 10.1111/hel.13017
43. Wang L, Li Z, Tay CY, Marshall BJ, Gu B, Guangdong Center for Quality Control of Clinical Gene T, et al. Multicentre, cross-sectional surveillance of Helicobacter pylori prevalence and antibiotic resistance to clarithromycin and levofloxacin in urban China using the string test coupled with quantitative PCR. Lancet Microbe. (2024) 5:e512–e3. doi: 10.1016/S2666-5247(24)00027-2
44. Versalovic J, Shortridge D, Kibler K, Griffy MV, Beyer J, Flamm RK, et al. Mutations in 23S rRNA are associated with clarithromycin resistance in Helicobacter pylori. Antimicrob Agents Chemother. (1996) 40:477–80. doi: 10.1128/AAC.40.2.477
45. van Amsterdam K, Bart A, van der Ende A. A Helicobacter pylori TolC efflux pump confers resistance to metronidazole. Antimicrob Agents Chemother. (2005) 49:1477–82. doi: 10.1128/AAC.49.4.1477-1482.2005
46. Bessede E, Megraud F. Microbiota and gastric cancer. Semin Cancer Biol. (2022) 86:11–7. doi: 10.1016/j.semcancer.2022.05.001
47. Chen CC, Liou JM, Lee YC, Hong TC, El-Omar EM, Wu MS. The interplay between Helicobacter pylori and gastrointestinal microbiota. Gut Microbes. (2021) 13:1–22. doi: 10.1080/19490976.2021.1909459
48. Noh JH, Shin JY, Lee JH, Park YS, Lee IS, Kim GH, et al. Clinical significance of Epstein-Barr virus and helicobacter pylori infection in gastric carcinoma. Gut Liver. (2023) 17:69–77. doi: 10.5009/gnl210593
49. Momozawa Y, Sasai R, Usui Y, Shiraishi K, Iwasaki Y, Taniyama Y, et al. Expansion of cancer risk profile for BRCA1 and BRCA2 pathogenic variants. JAMA Oncol. (2022) 8:871–8. doi: 10.1001/jamaoncol.2022.0476
50. Guo YA, Chang MM, Huang W, Ooi WF, Xing M, Tan P, et al. Mutation hotspots at CTCF binding sites coupled to chromosomal instability in gastrointestinal cancers. Nat Commun. (2018) 9:1520. doi: 10.1038/s41467-018-03828-2
51. Wang K, Yuen ST, Xu J, Lee SP, Yan HH, Shi ST, et al. Whole-genome sequencing and comprehensive molecular profiling identify new driver mutations in gastric cancer. Nat Genet. (2014) 46:573–82. doi: 10.1038/ng.2983
52. Wang K, Kan J, Yuen ST, Shi ST, Chu KM, Law S, et al. Exome sequencing identifies frequent mutation of ARID1A in molecular subtypes of gastric cancer. Nat Genet. (2011) 43:1219–23. doi: 10.1038/ng.982
53. Islami F, Boffetta P, Ren JS, Pedoeim L, Khatib D, Kamangar F. High-temperature beverages and foods and esophageal cancer risk–a systematic review. Int J Cancer. (2009) 125:491–524. doi: 10.1002/ijc.24445
54. Sexton RE, Al Hallak MN, Diab M, Azmi AS. Gastric cancer: a comprehensive review of current and future treatment strategies. Cancer Metastasis Rev. (2020) 39:1179–203. doi: 10.1007/s10555-020-09925-3
55. Zullo A, De Francesco V, Hassan C. Predicting Helicobacter pylori eradication: how to teach an old dog new tricks! J Clin Gastroenterol. (2012) 46:259–61. doi: 10.1097/MCG.0b013e318247177e
56. Cadamuro AC, Rossi AF, Maniezzo NM, Silva AE. Helicobacter pylori infection: host immune response, implications on gene expression and microRNAs. World J Gastroenterol. (2014) 20:1424–37. doi: 10.3748/wjg.v20.i6.1424
57. Muller A, Oertli M, Arnold ICH. pylori exploits and manipulates innate and adaptive immune cell signaling pathways to establish persistent infection. Cell Commun Signal. (2011) 9:25. doi: 10.1186/1478-811X-9-25
58. Ying L, Ferrero RL. Role of NOD1 and ALPK1/TIFA signalling in innate immunity against helicobacter pylori infection. Curr Top Microbiol Immunol. (2019) 421:159–77. doi: 10.1007/978-3-030-15138-6_7
59. Walduck AK, Raghavan S. Immunity and vaccine development against helicobacter pylori. Adv Exp Med Biol. (2019) 1149:257–75. doi: 10.1007/5584_2019_370
60. Fan J, Zhu J, Xu H. Strategies of Helicobacter pylori in evading host innate and adaptive immunity: insights and prospects for therapeutic targeting. Front Cell Infect Microbiol. (2024) 14:1342913. doi: 10.3389/fcimb.2024.1342913
61. Xue Y, Zhou LY, Lu HP, Liu JZ. Recurrence of Helicobacter pylori infection: incidence and influential factors. Chin Med J (Engl). (2019) 132:765–71. doi: 10.1097/CM9.0000000000000146
62. Machalek DA, Garland SM, Brotherton JML, Bateson D, McNamee K, Stewart M, et al. Very low prevalence of vaccine human papillomavirus types among 18- to 35-year old Australian women 9 years following implementation of vaccination. J Infect Dis. (2018) 217:1590–600. doi: 10.1093/infdis/jiy075
63. Amalia R, Panenggak NSR, Doohan D, Rezkitha YAA, Waskito LA, Syam AF, et al. A comprehensive evaluation of an animal model for Helicobacter pylori-associated stomach cancer: Fact and controversy. Helicobacter. (2023) 28:e12943. doi: 10.1111/hel.12943
64. Hong KS, Ki MR, Ullah HMA, Lee EJ, Kim YD, Chung MJ, et al. Preventive effect of anti-VacA egg yolk immunoglobulin (IgY) on Helicobacter pylori-infected mice. Vaccine. (2018) 36:371–80. doi: 10.1016/j.vaccine.2017.11.082
65. Mimuro H, Suzuki T, Nagai S, Rieder G, Suzuki M, Nagai T, et al. Helicobacter pylori dampens gut epithelial self-renewal by inhibiting apoptosis, a bacterial strategy to enhance colonization of the stomach. Cell Host Microbe. (2007) 2:250–63. doi: 10.1016/j.chom.2007.09.005
66. Asim M, Chikara SK, Ghosh A, Vudathala S, Romero-Gallo J, Krishna US, et al. Draft genome sequence of gerbil-adapted carcinogenic helicobacter pylori strain 7.13. Genome Announc. (2015) 3. doi: 10.1128/genomeA.00641-15
67. Kudo T, Lu H, Wu JY, Ohno T, Wu MJ, Genta RM, et al. Pattern of transcription factor activation in Helicobacter pylori-infected Mongolian gerbils. Gastroenterology. (2007) 132:1024–38. doi: 10.1053/j.gastro.2007.01.009
68. Kotloff KL, Sztein MB, Wasserman SS, Losonsky GA, DiLorenzo SC, Walker RI. Safety and immunogenicity of oral inactivated whole-cell Helicobacter pylori vaccine with adjuvant among volunteers with or without subclinical infection. Infect Immun. (2001) 69:3581–90. doi: 10.1128/IAI.69.6.3581-3590.2001
69. Li H, Zhang J, He Y, Li B, Chen L, Huang W, et al. Intranasal immunization with an epitope-based vaccine results in earlier protection, but not better protective efficacy, against Helicobacter pylori compared to subcutaneous immunization. Immunol Res. (2015) 62:368–76. doi: 10.1007/s12026-015-8666-9
70. Malfertheiner P, Selgrad M, Wex T, Romi B, Borgogni E, Spensieri F, et al. Efficacy, immunogenicity, and safety of a parenteral vaccine against Helicobacter pylori in healthy volunteers challenged with a Cag-positive strain: a randomised, placebo-controlled phase 1/2 study. Lancet Gastroenterol Hepatol. (2018) 3:698–707. doi: 10.1016/S2468-1253(18)30125-0
71. Arevalo CP, Bolton MJ, Le Sage V, Ye N, Furey C, Muramatsu H, et al. A multivalent nucleoside-modified mRNA vaccine against all known influenza virus subtypes. Science. (2022) 378:899–904. doi: 10.1126/science.abm0271
72. Xue RY, Liu C, Wang JQ, Deng Y, Feng R, Li GC, et al. Synthetic Self-Adjuvanted Lipopeptide Vaccines Conferred Protection against Helicobacter pylori Infection. Adv Healthc Mater. (2023) 12:e2300085. doi: 10.1002/adhm.202300085
73. Algood HM, Cover TL. Helicobacter pylori persistence: an overview of interactions between H. pylori and host immune defenses. Clin Microbiol Rev. (2006) 19:597–613. doi: 10.1128/CMR.00006-06
74. Cerutti A, Chen K, Chorny A. Immunoglobulin responses at the mucosal interface. Annu Rev Immunol. (2011) 29:273–93. doi: 10.1146/annurev-immunol-031210-101317
75. Zeng M, Mao XH, Li JX, Tong WD, Wang B, Zhang YJ, et al. Efficacy, safety, and immunogenicity of an oral recombinant Helicobacter pylori vaccine in children in China: a randomised, double-blind, placebo-controlled, phase 3 trial. Lancet. (2015) 386:1457–64. doi: 10.1016/S0140-6736(15)60310-5
76. Alameh MG, Tombacz I, Bettini E, Lederer K, Sittplangkoon C, Wilmore JR, et al. Lipid nanoparticles enhance the efficacy of mRNA and protein subunit vaccines by inducing robust T follicular helper cell and humoral responses. Immunity. (2021) 54:2877–92.e7. doi: 10.1016/j.immuni.2021.11.001
77. Zhang F, Ni L, Zhang Z, Luo X, Wang X, Zhou W, et al. Recombinant L. lactis vaccine LL-plSAM-WAE targeting four virulence factors provides mucosal immunity against H. pylori infection. Microb Cell Fact. (2024) 23:61. doi: 10.1186/s12934-024-02321-4
78. Yamaoka Y. Mechanisms of disease: Helicobacter pylori virulence factors. Nat Rev Gastroenterol Hepatol. (2010) 7:629–41. doi: 10.1038/nrgastro.2010.154
79. Hanafiah A, Lopes BS. Genetic diversity and virulence characteristics of Helicobacter pylori isolates in different human ethnic groups. Infect Genet Evol. (2020) 78:104135. doi: 10.1016/j.meegid.2019.104135
80. van Doorn LJ, Figueiredo C, Sanna R, Pena S, Midolo P, Ng EK, et al. Expanding allelic diversity of Helicobacter pylori vacA. J Clin Microbiol. (1998) 36:2597–603. doi: 10.1128/JCM.36.9.2597-2603.1998
81. Van Doorn LJ, Figueiredo C, Megraud F, Pena S, Midolo P, Queiroz DM, et al. Geographic distribution of vacA allelic types of Helicobacter pylori. Gastroenterology. (1999) 116:823–30. doi: 10.1016/s0016-5085(99)70065-x
82. Atherton JC, Peek RM Jr., Tham KT, Cover TL, Blaser MJ. Clinical and pathological importance of heterogeneity in vacA, the vacuolating cytotoxin gene of Helicobacter pylori. Gastroenterology. (1997) 112:92–9. doi: 10.1016/s0016-5085(97)70223-3
83. Chehelgerdi M, Doosti A. Effect of the cagW-based gene vaccine on the immunologic properties of BALB/c mouse: an efficient candidate for Helicobacter pylori DNA vaccine. J Nanobiotechnol. (2020) 18:63. doi: 10.1186/s12951-020-00618-1
84. Gonzalez RJ, Cryer AM, von Andrian UH, Artzi N. Immunology-guided biomaterial design for mucosal cancer vaccines. Adv Mater. (2020) 32:e1903847. doi: 10.1002/adma.201903847
85. Suberi A, Grun MK, Mao T, Israelow B, Reschke M, Grundler J, et al. Polymer nanoparticles deliver mRNA to the lung for mucosal vaccination. Sci Transl Med. (2023) 15:eabq0603. doi: 10.1126/scitranslmed.abq0603
86. Suerbaum S. Genetic variability within Helicobacter pylori. Int J Med Microbiol. (2000) 290:175–81. doi: 10.1016/S1438-4221(00)80087-9
87. Nell S, Estibariz I, Krebes J, Bunk B, Graham DY, Overmann J, et al. Genome and Methylome Variation in Helicobacter pylori With a cag Pathogenicity Island During Early Stages of Human Infection. Gastroenterology. (2018) 154:612–23.e7. doi: 10.1053/j.gastro.2017.10.014
88. Michetti P, Kreiss C, Kotloff KL, Porta N, Blanco JL, Bachmann D, et al. Oral immunization with urease and Escherichia coli heat-labile enterotoxin is safe and immunogenic in Helicobacter pylori-infected adults. Gastroenterology. (1999) 116:804–12. doi: 10.1016/s0016-5085(99)70063-6
89. Banerjee S, Medina-Fatimi A, Nichols R, Tendler D, Michetti M, Simon J, et al. Safety and efficacy of low dose Escherichia coli enterotoxin adjuvant for urease based oral immunisation against Helicobacter pylori in healthy volunteers. Gut. (2002) 51:634–40. doi: 10.1136/gut.51.5.634
90. Metzger WG, Mansouri E, Kronawitter M, Diescher S, Soerensen M, Hurwitz R, et al. Impact of vector-priming on the immunogenicity of a live recombinant Salmonella enterica serovar typhi Ty21a vaccine expressing urease A and B from Helicobacter pylori in human volunteers. Vaccine. (2004) 22:2273–7. doi: 10.1016/j.vaccine.2003.11.020
91. Aebischer T, Bumann D, Epple HJ, Metzger W, Schneider T, Cherepnev G, et al. Correlation of T cell response and bacterial clearance in human volunteers challenged with Helicobacter pylori revealed by randomised controlled vaccination with Ty21a-based Salmonella vaccines. Gut. (2008) 57:1065–72. doi: 10.1136/gut.2007.145839
92. Arnold C. How computational immunology changed the face of COVID-19 vaccine development. Nat Med. (2020). doi: 10.1038/d41591-020-00027-9
93. Chukwudozie OS, Duru VC, Ndiribe CC, Aborode AT, Oyebanji VO, Emikpe BO. The relevance of bioinformatics applications in the discovery of vaccine candidates and potential drugs for COVID-19 treatment. Bioinform Biol Insights. (2021) 15:11779322211002168. doi: 10.1177/11779322211002168
94. Rappuoli R. Reverse vaccinology, a genome-based approach to vaccine development. Vaccine. (2001) 19:2688–91. doi: 10.1016/s0264-410x(00)00554-5
95. Naz K, Naz A, Ashraf ST, Rizwan M, Ahmad J, Baumbach J, et al. PanRV: Pangenome-reverse vaccinology approach for identifications of potential vaccine candidates in microbial pangenome. BMC Bioinf. (2019) 20:123. doi: 10.1186/s12859-019-2713-9
96. Doytchinova IA, Flower DR. VaxiJen: a server for prediction of protective antigens, tumour antigens and subunit vaccines. BMC Bioinf. (2007) 8:4. doi: 10.1186/1471-2105-8-4
97. He Y, Xiang Z, Mobley HL. Vaxign: the first web-based vaccine design program for reverse vaccinology and applications for vaccine development. J BioMed Biotechnol. (2010) 2010:297505. doi: 10.1155/2010/297505
98. Rahman MS, Rahman MK, Saha S, Kaykobad M, Rahman MS. Antigenic: An improved prediction model of protective antigens. Artif Intell Med. (2019) 94:28–41. doi: 10.1016/j.artmed.2018.12.010
99. Vivona S, Bernante F, Filippini F. NERVE: new enhanced reverse vaccinology environment. BMC Biotechnol. (2006) 6:35. doi: 10.1186/1472-6750-6-35
100. Ong E, Wang H, Wong MU, Seetharaman M, Valdez N, He Y. Vaxign-ML: supervised machine learning reverse vaccinology model for improved prediction of bacterial protective antigens. Bioinformatics. (2020) 36:3185–91. doi: 10.1093/bioinformatics/btaa119
101. Saxena M, van der Burg SH, Melief CJM, Bhardwaj N. Therapeutic cancer vaccines. Nat Rev Cancer. (2021) 21:360–78. doi: 10.1038/s41568-021-00346-0
102. Pishesha N, Harmand TJ, Ploegh HL. A guide to antigen processing and presentation. Nat Rev Immunol. (2022) 22:751–64. doi: 10.1038/s41577-022-00707-2
103. Andreatta M, Karosiene E, Rasmussen M, Stryhn A, Buus S, Nielsen M. Accurate pan-specific prediction of peptide-MHC class II binding affinity with improved binding core identification. Immunogenetics. (2015) 67:641–50. doi: 10.1007/s00251-015-0873-y
104. Blass E, Ott PA. Advances in the development of personalized neoantigen-based therapeutic cancer vaccines. Nat Rev Clin Oncol. (2021) 18:215–29. doi: 10.1038/s41571-020-00460-2
105. Reynisson B, Alvarez B, Paul S, Peters B, Nielsen M. NetMHCpan-4.1 and NetMHCIIpan-4.0: improved predictions of MHC antigen presentation by concurrent motif deconvolution and integration of MS MHC eluted ligand data. Nucleic Acids Res. (2020) 48:W449–W54. doi: 10.1093/nar/gkaa379
106. Yu X, Negron C, Huang L, Veldman G. TransMHCII: a novel MHC-II binding prediction model built using a protein language model and an image classifier. Antib Ther. (2023) 6:137–46. doi: 10.1093/abt/tbad011
107. Cheng J, Bendjama K, Rittner K, Malone B. BERTMHC: improved MHC-peptide class II interaction prediction with transformer and multiple instance learning. Bioinformatics. (2021) 37:4172–9. doi: 10.1093/bioinformatics/btab422
108. De Groot AS, Moise L, Terry F, Gutierrez AH, Hindocha P, Richard G, et al. Better epitope discovery, precision immune engineering, and accelerated vaccine design using immunoinformatics tools. Front Immunol. (2020) 11:442. doi: 10.3389/fimmu.2020.00442
109. Ullah MA, Sarkar B, Islam SS. Exploiting the reverse vaccinology approach to design novel subunit vaccines against Ebola virus. Immunobiology. (2020) 225:151949. doi: 10.1016/j.imbio.2020.151949
110. Alawam AS, Alwethaynani MS. Construction of an aerolysin-based multi-epitope vaccine against Aeromonas hydrophila: an in silico machine learning and artificial intelligence-supported approach. Front Immunol. (2024) 15:1369890. doi: 10.3389/fimmu.2024.1369890
111. Farrell D, Gordon SV. Epitopemap: a web application for integrated whole proteome epitope prediction. BMC Bioinf. (2015) 16:221. doi: 10.1186/s12859-015-0659-0
112. Wu S, Skolnick J, Zhang Y. Ab initio modeling of small proteins by iterative TASSER simulations. BMC Biol. (2007) 5:17. doi: 10.1186/1741-7007-5-17
113. Rappuoli R. Reverse vaccinology. Curr Opin Microbiol. (2000) 3:445–50. doi: 10.1016/s1369-5274(00)00119-3
114. Vita R, Mahajan S, Overton JA, Dhanda SK, Martini S, Cantrell JR, et al. The immune epitope database (IEDB): 2018 update. Nucleic Acids Res. (2019) 47:D339–D43. doi: 10.1093/nar/gky1006
Keywords: gastric cancer, Helicobacter pylori, precision medicine, targeted therapy, neoantigen vaccines, artificial intelligence
Citation: Tu Z, Wang Y, Liang J and Liu J (2024) Helicobacter pylori-targeted AI-driven vaccines: a paradigm shift in gastric cancer prevention. Front. Immunol. 15:1500921. doi: 10.3389/fimmu.2024.1500921
Received: 24 September 2024; Accepted: 08 November 2024;
Published: 28 November 2024.
Edited by:
Jianqiu Kong, Sun Yat-sen Memorial Hospital, ChinaReviewed by:
Wendi Ma, University of Miami Health System, United StatesPing Han, Baylor College of Medicine, United States
Yuan Liu, University of Illinois at Urbana-Champaign, United States
Copyright © 2024 Tu, Wang, Liang and Liu. This is an open-access article distributed under the terms of the Creative Commons Attribution License (CC BY). The use, distribution or reproduction in other forums is permitted, provided the original author(s) and the copyright owner(s) are credited and that the original publication in this journal is cited, in accordance with accepted academic practice. No use, distribution or reproduction is permitted which does not comply with these terms.
*Correspondence: Jinping Liu, bGl1anBAc3lzdWNjLm9yZy5jbg==
†These authors have contributed equally to this work and share first authorship