- 1Department of Radiology, The Affiliated Yuebei People’s Hospital of Shantou University Medical College, Shaoguan, Guangdong, China
- 2Advanced Neuroimaging Laboratory, The Affiliated Yuebei People’s Hospital of Shantou University Medical College, Shaoguan, Guangdong, China
- 3Department of Radiology, Beijing Tiantan Hospital, Capital Medical University, Beijing, China
- 4Department of Pathology, The Affiliated Yuebei People’s Hospital of Shantou University Medical College, Shaoguan, Guangdong, China
- 5Department of Neurosurgery, Peking University Third Hospital, Peking University, Beijing, China
- 6Department of Imaging Sciences, University of Rochester Medical Center, Rochester, NY, United States
Cancer immunotherapy has emerged as a novel clinical therapeutic option for a variety of solid tumors over the past decades. The application of immunotherapy in primary and metastatic brain tumors continues to grow despite limitations due to the physiological characteristics of the immune system within the central nervous system (CNS) and distinct pathological barriers of malignant brain tumors. The post-immunotherapy treatment imaging is more complex. In this review, we summarize the clinical application of immunotherapies in solid tumors beyond the CNS. We provide an overview of current immunotherapies used in brain tumors, including immune checkpoint inhibitors (ICIs), oncolytic viruses, vaccines, and CAR T-cell therapies. We focus on the imaging criteria for the assessment of treatment response to immunotherapy, and post-immunotherapy treatment imaging patterns. We discuss advanced imaging techniques in the evaluation of treatment response to immunotherapy in brain tumors. The imaging characteristics of immunotherapy treatment-related complications in CNS are described. Lastly, future imaging challenges in this field are explored.
1 Background
In the last few decades, clinical immunotherapy has provided exceptional achievements for many solid tumors. The clinical development of novel immunotherapies for the treatment of CNS tumors has also continued to grow. Compared to traditional tumor treatment (e.g. radiotherapy, chemotherapy, or surgery), the imaging evaluation of primary and metastatic brain tumors following immunotherapy treatment is much more challenging. To better understand post-immunotherapy treatment imaging characteristics in brain tumors, we provide an overview of the current clinical application of immunotherapies, with a focus on the advances of immunotherapeutic approaches employed in brain tumors. The imaging criteria for the assessment of treatment response to immunotherapy are summarized, with the highlight of post-immunotherapy treatment imaging patterns. The clinical experiences of advanced imaging techniques used for the evaluation of treatment response to immunotherapy in brain tumors are reviewed. The imaging characteristics of immunotherapy treatment-related complications in CNS are described. Lastly, we discuss future imaging challenges in this field.
2 Development of immunotherapy and clinical application in solid tumors
The development of immunotherapy has significantly advanced cancer treatment. As early as the 19th century, William B. Coley attempted the first cancer immunotherapy by injecting bacterial toxins into cancer patients (1). From the 1980s to the early 2000s, monoclonal antibody drugs were successively approved by the Food and Drug Administration (FDA) for preventing organ transplant rejection and treating B-cell non-Hodgkin lymphoma, marking the application of monoclonal antibodies in cancer treatment (2). In 2001, Brentuximab Vedotin was approved for Hodgkin lymphoma and systemic anaplastic large cell lymphoma (3). In 2010, the first therapeutic cancer vaccine, Sipuleucel-T, was approved by the FDA for prostate cancer, successfully utilizing the patient’s own immune cells for treatment (4).
In 2011, Ipilimumab was approved by the FDA for metastatic melanoma, becoming the first approved immune checkpoint inhibitor (ICI) (5). That same year, Ralph Steinman was awarded the Nobel Prize in Physiology or Medicine for his discovery of dendritic cells and their role in adaptive immunity. In 2014, programmed cell death protein 1 (PD-1) inhibitors, such as Pembrolizumab and Nivolumab, were approved for advanced melanoma, revolutionizing the field of cancer immunotherapy (6). In 2017, the FDA approved the first CAR-T cell therapy, Kymriah (Tisagenlecleucel), for acute lymphoblastic leukemia (ALL) and Yescarta (Axicabtagene Ciloleucel) for large B-cell lymphoma, marking a new era of personalized immunotherapy (3). In 2018, James P. Allison and Tasuku Honjo were awarded the Nobel Prize in Physiology or Medicine for their contributions to the development of ICIs Figure 1.
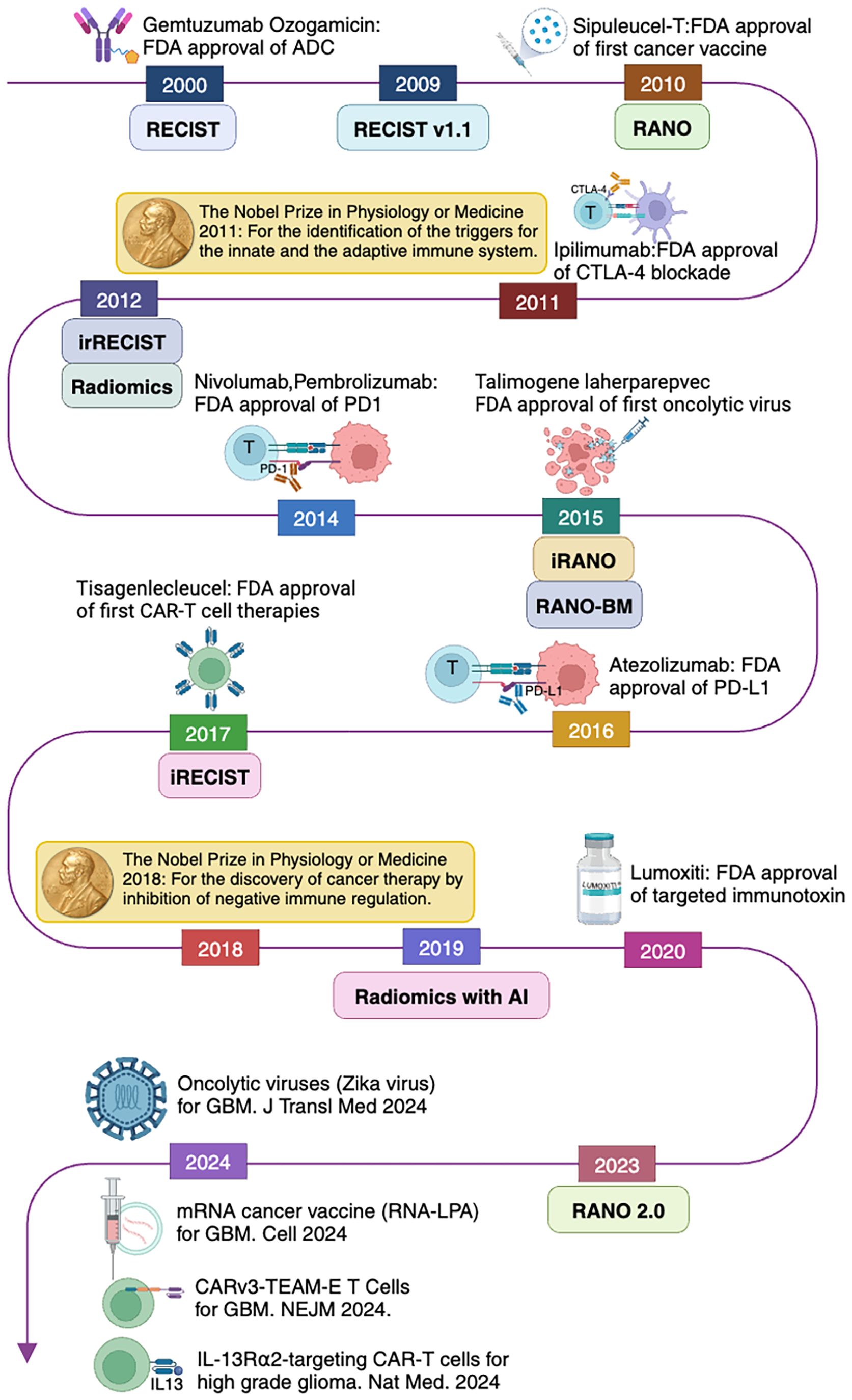
Figure 1. Evolution of cancer immunotherapy and imaging response assessment criteria related to brain tumors following immunotherapy.
Currently, clinical immunotherapy primarily includes ICIs, cancer vaccines, oncolytic viruses, adoptive cell therapy (ACT), and cytokines Table 1.
2.1 The application of immune checkpoint inhibitors in solid tumors (beyond the CNS)
ICIs have become standard treatments for various solid tumors beyond the CNS (7). PD-1 or programmed death ligand-1 (PD-L1) inhibitors primarily act on T cells within the tumor microenvironment, restoring their cytotoxic function by blocking the interaction between PD-1 and PD-L1. Representative drugs include PD-1 inhibitors nivolumab and pembrolizumab, as well as PD-L1 inhibitors atezolizumab, avelumab, and durvalumab, all of which have been approved by the FDA. These inhibitors are commonly used for treating malignant tumors such as melanoma, non-small cell lung cancer (NSCLC), renal cell carcinoma (RCC), triple-negative breast cancer (TNBC), gastric cancer, and gastroesophageal junction cancer (8. Cytotoxic T-Lymphocyte-Associated Protein 4 (CTLA-4) inhibitors act mainly during the early stages of T cell activation, blocking the negative regulatory effects of CTLA-4 that reduce T cell activation and proliferation. A representative drug is Ipilimumab, the first FDA-approved CTLA-4 inhibitor, indicated for melanoma and often used in combination with other ICIs for treating RCC and NSCLC (5).
PD-1/PD-L1 and CTLA-4 inhibitors have shown significant efficacy in cancer treatment but also face issues such as resistance, side effects, and limited response rates. Therefore, the other novel immune inhibitors that regulate immune responses through different mechanisms have emerged in recent years. These new inhibitors have the potential to address these shortcomings and demonstrate synergistic effects when used in combination with existing ICIs, further enhancing antitumor efficacy. For example, the combination of T cell immunoglobulin and mucin domain-containing protein 3 (TIM-3) inhibitors with PD-1/PD-L1 inhibitors has shown significant synergy in hepatocellular carcinoma (HCC) (9). T cell immunoreceptor with Ig and immunoreceptor tyrosine‐based inhibition motif domains (TIGIT) inhibitors combined with PD-1 inhibitors have been used to treat small cell lung cancer (SCLC) (10). Although the initial response to ICIs treatment is encouraging, many patients still can develop resistance after an initial response, resulting in the exploration of new combination therapy strategies and the identification of biomarkers to predict the efficacy of ICIs as a current research focus.
2.2 The application of cancer vaccines in solid tumors (beyond the CNS)
Cancer vaccines, by activating the patient’s immune system to recognize and attack tumor cells, have become a forefront area of immunotherapy research. The development of cancer vaccines aims to induce an immune response by introducing specific tumor antigens, thereby stimulating the body’s own immune protection mechanisms to achieve the treatment of tumors and/or the prevention of recurrence. The success of cancer vaccines requires the highly regulated cooperation of many branches of the immune system, including antigen-presenting cells, helper and cytotoxic T cells, natural killer (NK) cells, and tumor-resident myeloid cells (11).
The earliest cancer vaccines, peptide vaccines, emerged in the 1990s. By injecting tumor-associated antigen peptide fragments, these vaccines enhance the recognition and attack of tumor cells and are suitable for breast cancer, melanoma, RCC, and ovarian cancer (12). Subsequently, DNA vaccines appeared, activating T cells and B cells by injecting DNA encoding tumor antigens. These are commonly used to treat melanoma, prostate cancer, cervical cancer, and head and neck cancers (13).
In recent years, emerging dendritic cell vaccines and mRNA vaccines have made significant progress in the treatment of solid tumors. Dendritic cell vaccines activate T cells by loading them with tumor antigens (14). Sipuleucel-T, which appeared in 2010, is the first FDA-approved therapeutic cancer vaccine for hormone-refractory prostate cancer. mRNA vaccines induce immune responses by delivering mRNA encoding tumor antigens (4). Between 2018 and 2021, personalized mRNA cancer vaccines and vaccines combined with immune inhibitors were applied to melanoma, breast cancer, lung cancer, bladder cancer, pancreatic cancer, and colorectal cancer (15). Other vaccines, such as human papillomavirus (HPV) and hepatitis B virus (HBV) vaccines, have been approved by the FDA to prevent virus-related cervical cancer and liver cancer (16). With technological advances, the application of cancer vaccines in the treatment of solid tumors continues to grow.
2.3 The application of oncolytic viruses in solid tumors (beyond the CNS)
Oncolytic viruses achieve anticancer effects through selective infection and lysis of tumor cells. The molecular and cellular mechanisms are not yet fully understood but may involve several factors: direct cell lysis, induction of immunogenic cell death to activate specific antitumor immune responses, alteration of the tumor microenvironment to enhance immune cell activity, carrying antitumor genes to further boost effects of synergistic therapies, and disrupting the tumor vascular system to deprive tumor cells of nutrients and oxygen (17, 18). Over the past few decades, many different oncolytic virus candidates have been developed and tested (17). Most recently, scientists have combined oncolytic viruses with other current immunotherapies to turn “immune-cold” tumors into “immune-hot” tumors (19). These mechanisms make oncolytic viruses a promising cancer immunotherapy approach.
Oncolytic viruses have demonstrated the potential for various mechanisms in cancer treatment. By selectively infecting and lysing tumor cells, oncolytic viruses, such as T-VEC and Oncorine (H101), are currently used for the treatment of melanoma and head and neck cancers (20, 21). Immunoactivating viruses, such as Reolysin (Pelareorep) and Pexa-Vec (JX-594), are commonly applied to head and neck cancer, breast cancer, and HCC (22, 23). Viruses that modify the tumor microenvironment to enhance immune cell activity, such as the measles virus, are often used for melanoma and ovarian cancer (24). Viruses that carry and express antitumor genes to enhance immune responses, such as poxvirus and adenovirus, are used for breast cancer, colorectal cancer, and prostate cancer (25, 26).
2.4 The application of adoptive cell therapy in solid tumors (beyond the CNS)
ACT involves extracting a patient’s own immune cells, particularly T cells, expanding and genetically modifying them in vitro, and then reinfusing them back into the patient to enhance the anti-tumor immune response. ACT has shown significant clinical efficacy in treating various malignant tumors and has become a crucial area of research in current cancer immunotherapy. The three main forms of ACT include Tumor-Infiltrating Lymphocytes (TIL), T-cell receptors (TCR) targeting specific cancer antigens, and Chimeric Antigen Receptor T cells (CAR-T) (27).
TIL therapy involves extracting TIL with anti-tumor activity from the patient’s tumor tissues, expanding them in vitro, and reinfusing them into the patient to enhance the anti-tumor immune response. It is currently being tested in melanoma, cervical cancer, and ovarian cancer (28). Unlike TIL therapy, TCR therapy involves expanding and reinfusing T cells that have been genetically engineered to express specific antigen-recognizing TCRs, commonly used for melanoma and synovial sarcoma (29). Like TCR therapy, CAR-T therapy also reinfuses T cells. However, CAR-T involves introducing a chimeric antigen receptor that recognizes specific antigens on the surface of cancer cells into the patient’s T cells. CAR-T is commonly used for B-cell ALL, large B-cell lymphoma, and other hematological malignancies (30).
2.5 The application of cytokine therapy in solid tumors (beyond the CNS)
Cytokine therapy is a treatment method that regulates and enhances the immune system’s response against tumors through cytokines. Cytokines are small, secreted proteins released by various cells under stress, such as interferon-α, interferon-γ, interleukin-2 (IL-2), interleukin-15 (IL-15), interleukin-21 (IL-21), and interleukin-12 (IL-12) (31). They play powerful immunomodulatory roles in the body and are critical components of the tumor microenvironment, where they can either inhibit or promote tumor development.
Common cytokine therapies include interferons, interleukins, and tumor necrosis factor (TNF). Interferons work by activating immune cells (e.g. NK cells and macrophages), enhancing antigen presentation, and boosting the antiviral and antitumor activity of cells. They are commonly used for treating melanoma, chronic myelogenous leukemia, and RCC (32). Interleukins promote the proliferation and activation of T cells and NK cells, thereby enhancing the immune system’s ability to attack cancer cells. They are frequently used for metastatic RCC and melanoma (33, 34). Tumor necrosis factor (TNF) combats tumors through multiple mechanisms including tumor cell apoptosis, disrupting tumor vasculature, and activating immune cells. TNF is often used for locally advanced soft tissue sarcoma and melanoma (35, 36).
3 Immunotherapy for brain tumors
High grade glioma is the most common and aggressive primary malignant brain tumor of the CNS, and brain metastasis is the most common intracranial malignancy in adults (37). Recently, immunotherapy treatments were used in primary and secondary brain tumors and provided encouraging results (38, 39). However, their efficacy and outcomes are still low.
Immunotherapy for brain tumors faces many challenges (40–42). Protected by the blood-brain barrier (BBB), many immunotherapeutic drugs have difficulty entering the brain, resulting in insufficient concentrations in brain tumors and limited therapeutic efficacy (43, 44). Compared to brain tumors, organs and tissues where body tumors are located (such as the lungs, liver, and kidneys) do not have the physical impedance of the BBB, allowing drugs to reach the tumor site more easily and exert their therapeutic effects. Drugs can directly reach the tumor site through the bloodstream, penetrate the tumor microenvironment, and more effectively stimulate the immune system to attack tumor cells (45). Additionally, although recent studies have confirmed the existence of a glymphatic system and various antigen-presenting cells (such as microglia, macrophages, astrocytes, and dendritic cells) in the brain, brain tissue lacks the classical lymphatic drainage system and has a limited inflammatory response compared to other organs. As a result, the brain has been termed immune-privileged (46, 47).
Compared to other tumors, brain tumors have fewer TIL and immune effector cells. As a result, they exhibit characteristics of “cold tumors,” which significantly affect the efficacy of therapies such as immune checkpoint blockade (48). Additionally, the tumor microenvironment in brain tumors contains many immunosuppressive cells and molecules, creating a highly immunosuppressive microenvironment (31, 49, 50). This not only inhibits the activity of the immune system, but also promotes immune evasion by the tumor, and allows tumor cells to avoid attack by the immune system. Additionally, brain tumor cells exhibit immunoresistance, further weakening the effectiveness of immunotherapy (51). The heterogeneity of tumor cell populations and frequent genetic mutations within brain tumors leads to varied responses to treatment among different subpopulations of tumor cells, subsequently increasing the complexity of treatment, and making it difficult for targeted therapies to cover all tumor cells (52, 53). Table 2. Therefore, future research needs to focus on developing comprehensive treatment strategies that can overcome these immunosuppressive mechanisms and heterogeneity challenges to improve the efficacy of immunotherapy for brain tumors.
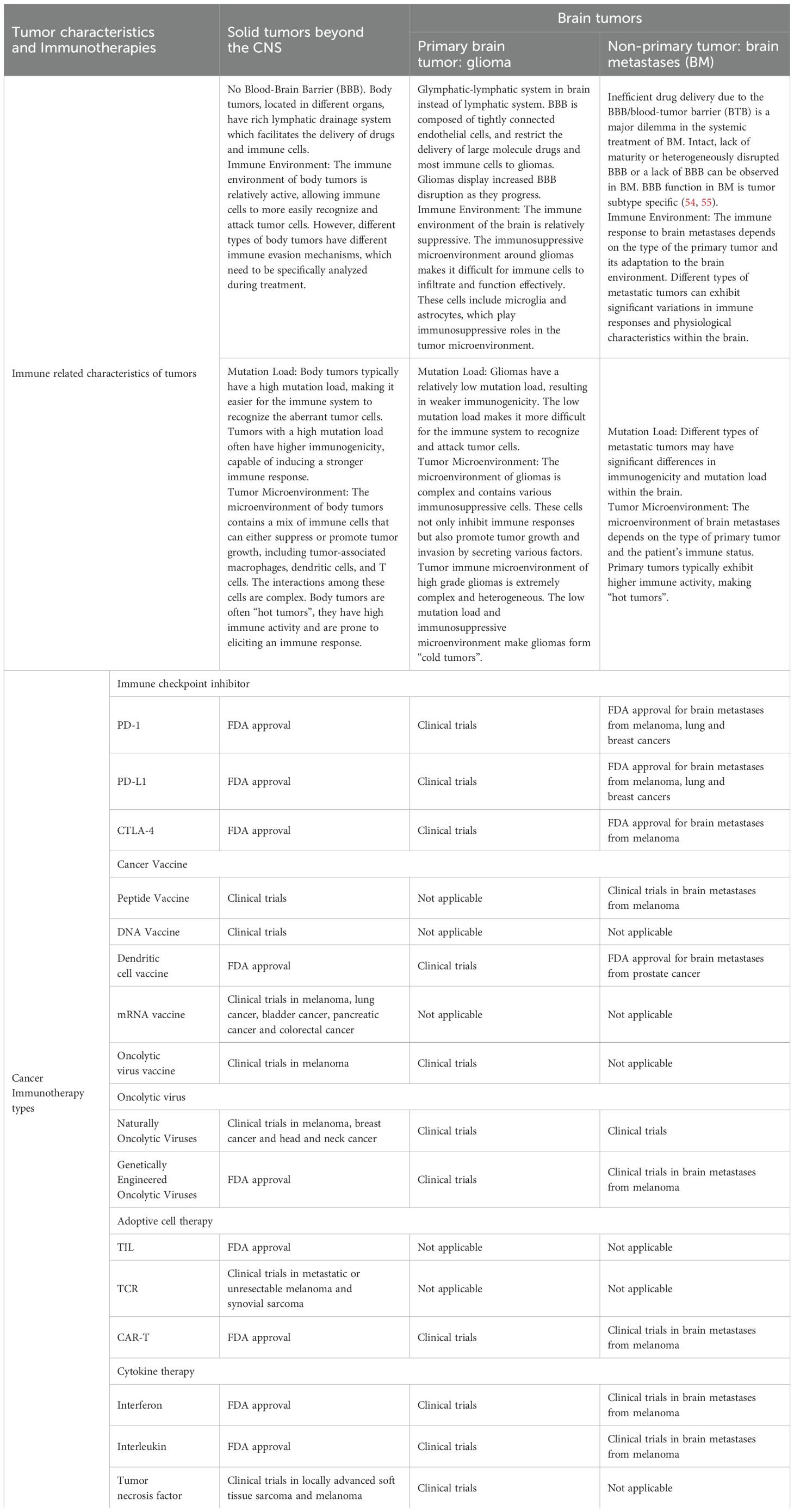
Table 2. Immuno-related with features and current status of immunotherapies in primary and metastatic brain tumors.
We will separately introduce the immunotherapy of primary malignant gliomas and brain metastases (BM). By deeply analyzing the immunotherapy of these specific types of brain tumors, Table 2. We hope to provide valuable insights and guidance for future research and clinical practice.
3.1 Immunotherapy for gliomas
Glioblastoma multiforme (GBM) is the most common type of primary malignant brain tumor in adults (56). The current standard of care, proposed by Stupp et al. in 2005, includes maximal surgical resection followed by radiotherapy and concurrent chemotherapy with temozolomide (57). This regimen has been widely accepted and is the global standard for treating GBM. Despite this multimodal approach, the prognosis for patients remains poor, with a median overall survival of approximately 14-16 months (58). In recent years, immunotherapy has significantly improved outcomes in many systemic tumors, bringing new hope for improved treatment of high-grade gliomas (59). Here are some of the main immunotherapy approaches applied to gliomas.
3.1.1 The application of immune checkpoint inhibitors in gliomas
Research on ICIs for gliomas has primarily focused on the PD-1/PD-L1 and CTLA-4 pathways. In gliomas, the use of common PD-1 inhibitors, such as nivolumab and pembrolizumab, faces several challenges, including the limitations of the BBB and the complexity of tumor immune evasion mechanisms. However, some clinical trials have shown certain efficacy (59–61). For example, trials of nivolumab in patients with recurrent GBM have indicated that some patients respond to the treatment and have extended survival (62). The common CTLA-4 inhibitor ipilimumab has also been shown to be effective for the treatment of recurrent GBM. Although its effectiveness as a monotherapy is limited, combining it with other treatments may enhance outcomes (62). Currently, there are multiple ongoing research on the combination of PD-1 and CTLA-4 inhibitors, with the hope of improving overall response rates in patients (63).
Targeted therapy, via inhibition of specific cancer-related signaling pathways such as EGFR and VEGF, has shown significant efficacy in various tumors (64–66). Combining targeted therapy with ICIs is expected to enhance treatment effects through multiple mechanisms (67). Several clinical studies are exploring the combined application of ICIs and targeted therapies in GBM. For example, the combination of the VEGF-targeted drug bevacizumab with a PD-1 inhibitor can reduce tumor angiogenesis and improve immune cell infiltration into tumors.
3.1.2 The application of cancer vaccines in gliomas
Cancer vaccines are designed to induce the immune system to recognize and attack specific tumor antigens (13, 68). Research on vaccines for gliomas has primarily focused on the EGFRvIII vaccine and dendritic cell vaccines (69–71). EGFRvIII is a mutation commonly found in GBM. Vaccines developed against EGFRvIII, such as Rindopepimut, have shown promising immune responses in clinical trials (72). Although it did not significantly extend patient survival in Phase III clinical trials, research on this vaccine continues. Dendritic cell vaccines for gliomas have also shown some immune responses and extended survival in initial clinical trials, but their efficacy still needs further validation (73). In 2024, novel mRNA cancer vaccines applied to brain tumors have significantly improved treatment effects by activating anti-tumor immune responses (74). Additionally, the attenuated Zika virus vaccine (ZIKV-LAV) has shown potential therapeutic effects against human GBM (75).
3.1.3 The application of oncolytic viruses in gliomas
In the research on immunotherapy for gliomas, oncolytic virotherapy has shown significant potential and emerged as a promising treatment strategy (76–78). Talimogene laherparepvec (T-VEC), a genetically modified herpes simplex virus, has achieved success in melanoma and is now being studied for gliomas (79). It can directly kill tumor cells and elicit a strong immune response by releasing tumor antigens and cytokines. Additionally, the modified adenovirus DNX-2401 has demonstrated selective killing of GBM cells in clinical trials, significantly reducing tumor size and achieving long-term survival in some patients (80, 81).
Toca 511, a non-lytic amphotropic retroviral replicating vector, has shown encouraging efficacy in early studies of recurrent high-grade gliomas, with some patients achieving complete remission (82). G207, a genetically modified type I oncolytic herpes simplex virus, has been shown to significantly increase the number of TIL in gliomas and successfully convert “cold” tumors into “hot” tumors, paving the way for new directions in glioma immunotherapy (83, 84). New research indicates that CAN-3110, another oncolytic virus derived from herpes simplex virus, has also demonstrated good immune activation effects and potential therapeutic benefits in clinical trials (85). Overall, the application of oncolytic virotherapy in gliomas is advancing and may provide effective complementation to traditional treatments and potential efficacy improvements.
3.1.4 The application of adoptive cell therapy in gliomas
The application of ACT in gliomas shows promising prospects, particularly in the innovative research on CAR-T cell therapy, TIL therapy, TCR-T cell therapy, NK cell therapy, and CAR-NK cell therapy (86–89). CAR-T cell therapy has achieved remarkable success in hematologic malignancies, but its application in gliomas is still under exploration. CAR-T cell therapies targeting EGFRvIII and IL-13Rα2 are currently in clinical trials. In a recent phase I clinical trial for recurrent high-grade gliomas, local administration of IL-13Rα2-targeted CAR-T cells demonstrated safety and preliminary efficacy, with some patients achieving stable disease (SD) or even remission (90). However, due to the heterogeneity and immune evasion mechanisms of gliomas, the durability and overall efficacy of this therapy require further investigation.
TIL therapy has shown efficacy in melanoma, but research in gliomas is still in the early stages. Some preliminary studies indicate its potential to induce anti-tumor immune responses (28, 91). Like CAR-T cell therapy, TCR-T cell therapy faces challenges such as the BBB, tumor heterogeneity, and immune evasion. Clinical trials for TCR-T cell therapy targeting gliomas are ongoing (92).
NK cell therapy has shown some anti-tumor potential in gliomas, especially when combined with other immunotherapy methods (93). CAR-NK (Chimeric Antigen Receptor-Natural Killer) cell therapy applies CAR technology to NK cells, giving them the ability to specifically recognize and attack tumor cells. The application of CAR-NK cell therapy in gliomas is still in the early research stages, but preliminary results show promise (94).
3.1.5 The application of cytokine therapy in gliomas
Common cytokine therapies include IL-2, interferon-γ (IFN-γ), IL-12, TNF-α, and IL-15 (95). Due to the strong immune activation effects of cytokines, they bring potential toxicity and side effects. As a result, the systemic use of cytokine therapy in the treatment of GBM is limited. Currently, the application of cytokine therapy to GBM is still in the research stage (96, 97).
3.2 Immunotherapy for brain metastases
BM is the most common intracranial malignancy in adult cancer patients, with an estimated 30-40% of patients with solid malignancies developing intracranial metastases during their illness (98). Over the past decade, the incidence of BM has increased. As the population ageing and the number of cancer diagnoses markedly increase, the likelihood of BM also increases. Additionally, improvements in systemic treatments have extended the survival time of cancer patients, leading to a projected continued increase in the incidence of BM (99). Common primary cancers that metastasize to the brain include lung cancer, breast cancer, melanoma, and kidney cancer (100).
Historically, the diagnosis of BM has been considered fatal, almost akin to a death sentence, with only a few treatment options available to alleviate symptoms or extend life (100). Among the limited treatment options, brain radiation therapy and surgical resection have been the mainstays of treatment (100). In recent years, immunotherapy, either used alone or in combination with traditional treatments, has emerged as a significant force in combating the spread of BM and reducing tumor burden (100). Next, we will introduce the application of immunotherapy in the treatment of BM from various types of cancer.
3.2.1 Application of immune checkpoint inhibitors in brain metastases
ICIs have shown significant efficacy in the treatment of BM from various types of cancer (101). In recent years, ICIs such as ipilimumab, pembrolizumab, and nivolumab have been increasingly used in patients with melanoma BM (102–104). In the application for lung cancer, studies have found that PD-1/PD-L1 inhibitors as monotherapy have demonstrated good results in advanced NSCLC BM patients (105). Some case reports have shown that patients with SCLC BM experienced a complete disappearance of recurrent BM and sustained complete remission after receiving a combination of multi-kinase inhibitors and PD-1/PD-L1 inhibitors (106).
Compared to melanoma and lung cancer, the application of immune inhibitors in breast cancer BM is less developed. Some recent studies have found that combining anti-PD-1 therapy with anti-estrogen therapy can significantly extend progression-free survival in patients, although results still require more research for validation (107). Colorectal cancer and RCC have a relatively lower incidence of BM (108, 109), but immunotherapy has also shown some promise in these cancer types. For example, the use of nivolumab in patients with RCC BM has shown good safety and efficacy (110).
3.2.2 The application of cancer vaccines in brain metastases
Dendritic cell vaccines are an important direction in cancer vaccine research. Studies have found that these vaccines can significantly enhance tumor antigen-specific T-cell responses and prolong overall survival in patients with BM (111). Combining dendritic cell vaccines with ICIs is another strategy to improve therapeutic outcomes. For example, using an anti-HER2/HER3 dendritic cell vaccine in combination with Pembrolizumab (a PD-1 inhibitor) can significantly improve CNS response rates and progression-free survival in patients with HER2-positive breast cancer BM (112). A study showed that a patient with colorectal cancer BM who received a dendritic cell vaccine pulsed with tumor antigens exhibited a significant antitumor response (113). Despite the promising effects of cancer vaccines in treating BM, further research is needed to optimize vaccine design and treatment strategies.
3.2.3 The application of oncolytic viruses in brain metastases
In the treatment of BM, research on oncolytic viruses has primarily focused on several specific viruses, such as adenovirus, herpes simplex virus, and measles virus (114). T-VEC, an oncolytic virus based on HSV-1, has been approved for the treatment of advanced melanoma and has shown potential in treating BM (115). In combination with other treatment modalities, oncolytic viruses have also demonstrated significant synergistic effects. For example, the combination of oncolytic viruses with ICIs has been used to treat unresectable melanoma and has shown promising therapeutic outcomes in cases involving BM (116, 117). Research indicates that oncolytic viruses can enhance the efficacy of ICIs by lysing tumor cells and releasing tumor antigens. Additionally, the combination of oncolytic viruses with radiotherapy has shown promising results (118) as radiotherapy can increase the immunogenicity of tumor cells, thereby enhancing the antitumor effects of oncolytic viruses (115).
3.2.4 Adoptive cell therapy in the treatment of brain metastases
The application of ACT in BM primarily focuses on several common types of BM. TILs therapy has shown significant efficacy in melanoma BM. In one study, a notable proportion of patients receiving TILs therapy exhibited tumor reduction and prolonged survival (119). CAR-T cell therapy has shown potential in treating breast cancer BM and CAR-T cell therapy targeting HER2-positive breast cancer is currently undergoing clinical trials (120). The application of ACT in NSCLC BM is less studied, but preliminary research suggests that TCR-T cell therapy has the potential to specifically recognize and kill tumor cells (121).
3.2.5 The application of cytokine therapy in brain metastases
IL-2 and IL-12 are among the cytokines most extensively studied in the treatment of BM. Benefiting from the similarity between BM and primary body tumors, some cytokine therapies that have shown significant efficacy in melanoma also demonstrate promising performance in the treatment of BM. Studies have shown that the combination therapy of IFN-α and IL-2 can significantly extend the progression-free survival (PFS) and overall survival (OS) of some melanoma patients with BM (122).
3.3 Immunotherapy for primary central nervous system lymphoma
Primary central nervous system lymphoma (PCNSL) is a rare and aggressive form of non-Hodgkin lymphoma that primarily affects the lymphatic tissue in the brain, spinal cord, and eyes (123). Chemotherapy and radiotherapy are the main treatment modalities for PCNSL. Although traditional chemotherapy and radiotherapy can be effective, the recurrence rate of PCNSL remains high (124). In recent years, immunotherapy has shown great promise in the treatment of primary central nervous system lymphoma, especially with the advent of CAR T-cell therapy and ICIs (125, 126). While these methods are currently in the clinical research phase, they hold the potential to bring new treatment options to PCNSL patients, potentially extending survival and improving quality of life.
3.4 Immunotherapy for meningioma
Meningioma is the most common type of primary intracranial tumor, with the majority being grade I, which can often be cured with complete surgical resection. However, grade II and grade III meningiomas frequently recur after surgery and radiotherapy, making their treatment more challenging (127). Traditional alkylating agent chemotherapy and targeted therapies are generally ineffective against these high-grade meningiomas.
In recent years, immunotherapy for high-grade meningiomas has garnered widespread attention. Research has found that the expression of immune checkpoint molecules, such as PD-L1, in meningiomas is positively correlated with tumor grade. This suggests that high-grade meningiomas may be more responsive to treatment with ICIs (128, 129). A phase II trial using nivolumab for patients with recurrent grade II and III meningiomas showed an improvement in median progression-free survival compared to previous studies with other therapies (127). With the advancement of immunotherapy, future research should focus on gaining a better understanding of the impact of various cellular components within the tumor microenvironment of meningiomas on treatment outcomes, in order to further enhance the precision and effectiveness of these therapies.
3.5 Summary
The application of immunotherapy in brain tumors, particularly in primary brain tumors and BM, demonstrates tremendous potential. By further understanding the immune microenvironment of brain tumors, overcoming the obstacles posed by the BBB, and developing new immunotherapeutic approaches, immunotherapy is poised to become a significant modality in the treatment of brain tumors.
4 Evolution of cancer imaging evaluation criteria
Post-treatment cancer imaging is critically important for clinical management in oncology practice. For better treatment response evaluation, multiple cancer imaging evaluation strategies have been proposed, including World Health Organization criteria (130), which were introduced in 1979, and RECIST 1.0, which were introduced in 2000 (131) and revised (RECIST 1.1) in 2009 (132), Figure 1. The first set of imaging criteria for evaluating immunotherapy treatment was the immune-related response criteria, which were developed in 2009 (133). Further modifications were attempted to accurately characterize immune-related response patterns. These efforts resulted in modified criteria including immune-related RECIST (irRECIST) which was developed in 2013 (134), and iRECIST in 2017 (135).
In the field of neuro-oncology, the Response Assessment in Neuro-Oncology (RANO) Working Group published a series of response criteria for high-grade gliomas (RANO-HGG) in 2010 (136), low-grade gliomas (RANO-LGG) in 2011 (137), the Immunotherapy RANO Criteria (iRANO) (138), and the RANO Criteria for BM (RANO-BM) (139) in 2015, and the Modified RANO Criteria (mRANO) in 2017 (140). The latest updated RANO criteria were RANO 2.0 which includes both high-grade and low-grade gliomas, were published in 2023 (141). The iRANO, RANO-BM, and RANO 2.0 are three RANO criteria related to immunotherapy treatment response evaluation for malignant glioma and BM. We compare these criteria, including brain tumor types, definitions of the measurement methods, imaging evaluation baseline, targets, and response criteria including complete response (CR) (203), partial response (PR), SD, and progressive disease (PD) in Table 3, Figures 2 and 3.
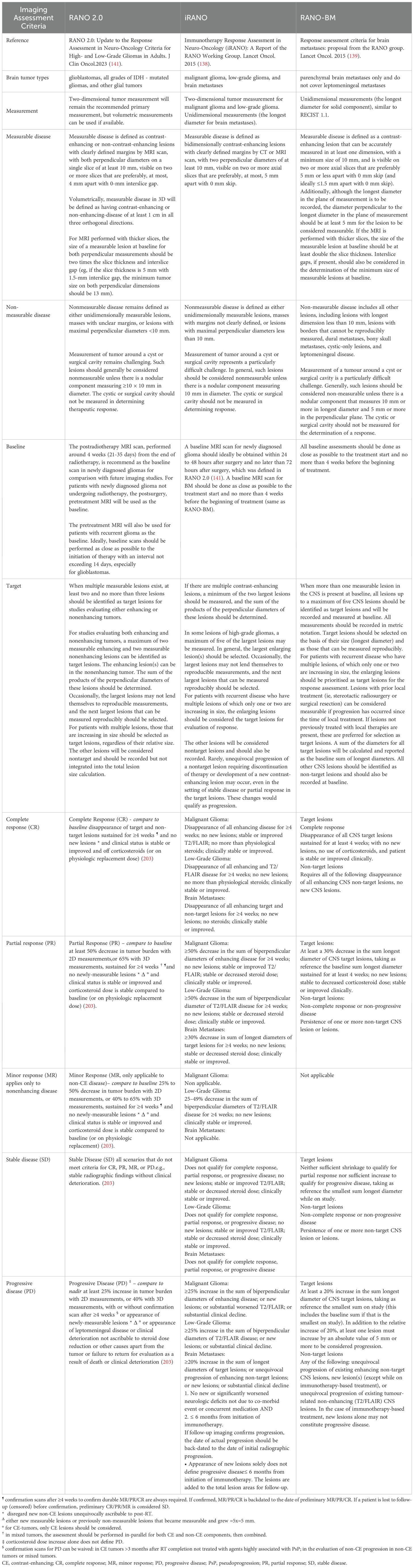
Table 3. Comparison of RANO2.0, iRANO, RANO-BM criteria in the response of brain tumors treated with immunotherapy.
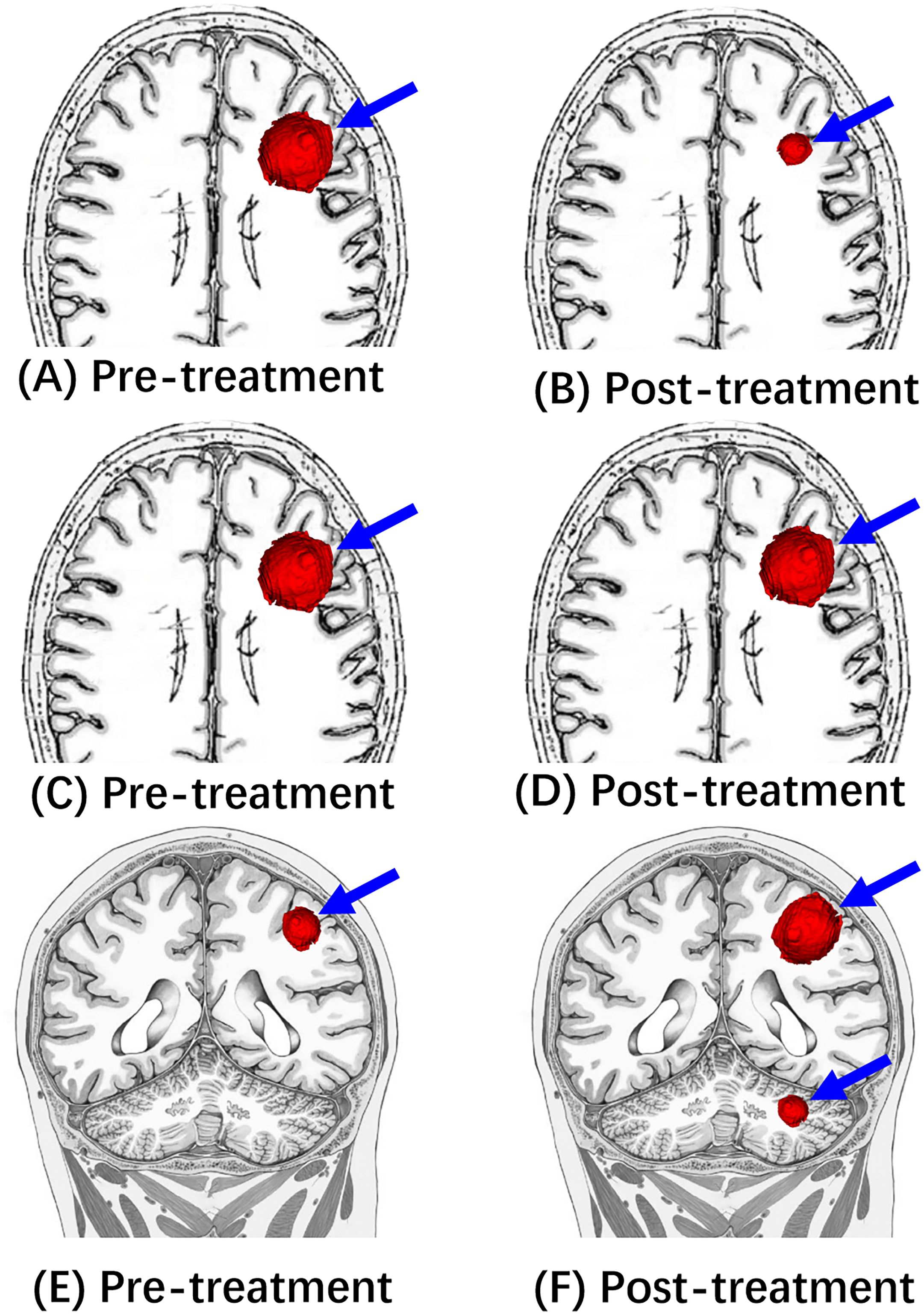
Figure 2. Illustrative diagrams of Response (PR), Stable (SD), and Progression (PD). (A, C, E) Are pre-treatment MRI showing a left frontal mass (blue arrow). After the immunotherapy treatment, (B) shows the decreased size of this mass (blue arrow), which is consistent with “Response”(PR). (D) Shows “stable” (SD) tumor size following the immunotherapy treatment. (F) Shows “progression” (PD) of tumor size following the immunotherapy treatment.
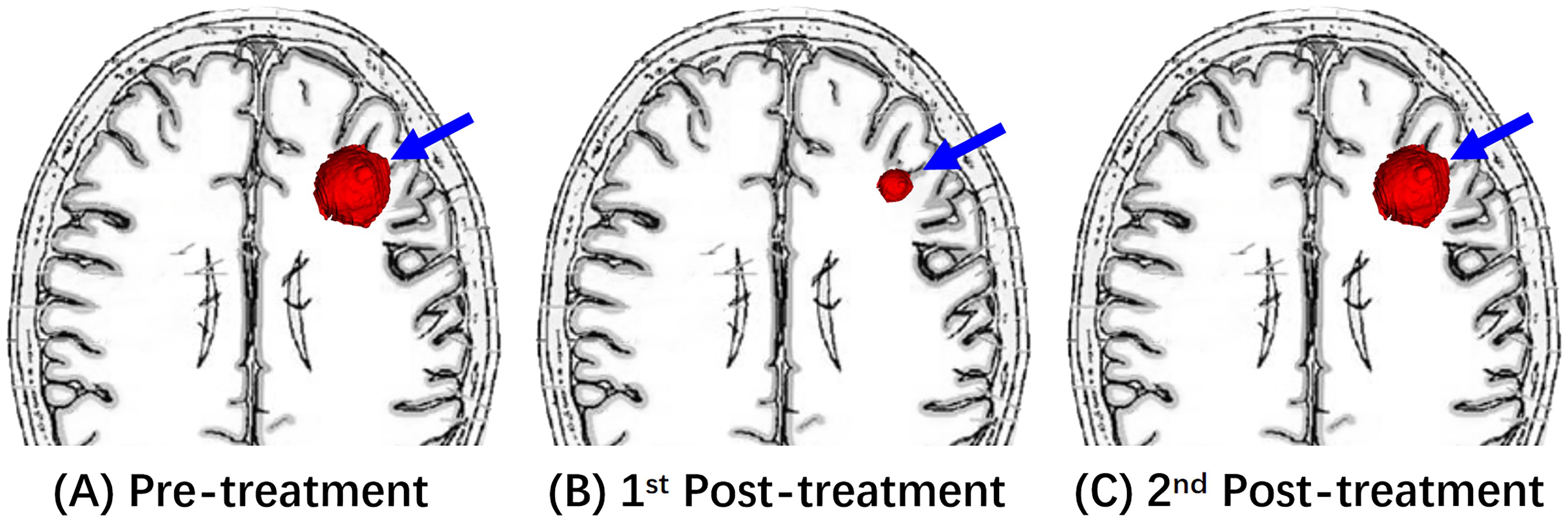
Figure 3. An illustrative diagram of Response (PR). (A) Shows a left frontal mass on pre-treatment MRI (blue arrow). After the immunotherapy treatment, (B) of the 1st post-treatment MRI showed the decreased size of this mass. The 2nd post-treatment MRI of (C) reveals re-enlargement of this enhancing mass (blue arrow).
Compared to the unidimensional measurement for BM in RANO-BM and iRANO, two-dimensional measurements (the sum of bi-perpendicular diameters) were used in both iRANO and RANO 2.0 for malignant and low-grade gliomas. Volumetric analysis is added in RANO 2.0.
RANO criteria have been used in different clinical studies, leading to variability in response assessments. Chen et al. found that RANO-HGG and iRANO had high concordance for assessing PD in patients within 6 months of ICIs initiation (142). Youssef et al. (143) showed that RANO-HGG and mRANO demonstrated similar correlations between progression-free survival and overall survival in GBM patients. However, iRANO criteria did not add significant benefit in GBM patients who received ICIs. Douri et al. (144) demonstrated that RANO-BM criteria unreliably identified clinically relevant tumor progression (TP) in BM after stereotactic radiosurgery.
It should be noted that in the case of immunotherapy, new lesions alone cannot constitute progressive disease in RANO-BM, iRANO, and RANO 2.0. Often immunotherapy-based treatments require the induction of immune cell infiltrates (e.g. CD8+ T cell lymphocytes) which takes time to mount an effective immune response against the tumor. Thus, for patients with the appearance of new lesions within 6 months of the initiation of immunotherapy alone and without substantial neurological decline, the iRANO working committee recommends confirmation of radiographic progression by follow-up imaging in 3 months after initial radiographic evidence of progressive disease. RANO-BM group recommends progressive disease should not be solely defined by the appearance of new lesions but rather as a minimum 20% increase in the sum longest diameter of CNS target and new lesions, unequivocal progression of existing enhancing non-target CNS lesions, unequivocal progression of existing non-enhancing (T2/FLAIR) CNS lesions, or clinical decline related to the tumor. A short interval scan is advised if immune response-related radiographical changes are suspected. In contrast, RANO 2.0 defines any new measurable (≥10mm×10 mm) enhancing lesions as PD in the case where the baseline or best response demonstrates no measurable enhancing disease. If there is uncertainty regarding progression, close observation (e.g. re-evaluated at 4-week intervals) is recommended.
5 Atypical patterns of response to immunotherapy
Beyond the standard response and progression patterns in Table 3, atypical patterns after immunotherapy including pseudoprogression (PsP), hyperprogression, and dissociated response, are important for radiologists and oncology physicians, and summarized in Table 4. Due to the increasing growth of combination treatments of immunotherapy, radiosurgery, and Bevacizumab, these atypical patterns are becoming common. The radiographic phenomena of radiation necrosis (RN), abscopal response, and pseudoresponse are summarized in Table 4 and are discussed in the following section.
5.1 Pseudoprogression
PsP is an imaging phenomenon describing the initial and transient growth of primary lesion(s) or the appearance of new lesion(s) after chemoradiotherapy or immunotherapy treatment, followed by stabilization or shrinkage. PsP is typically observed in the first 12 weeks (about 3 months) after completion of radiochemotherapy for GBMs (occurring in up to 30%-40% of patients) (141). This phenomenon can be observed in several different cancers following immunotherapies, including solid tumors, lymphomas, and brain tumors. Thus, PsP is one of the primary factors driving updates of the RANO criteria over the past 14 years.
The overall incidence of PsP ranges from 6% -14.8% (3, 145), varying with the type of tumor treated with ICIs. One study noted PsP in 17.9% of pembrolizumab treated patients with reported progression who were re-imaged after continued therapy despite reported progression (146). The PsP has also been reported in oncolytic viruses (141), ADCs, and CAR-T cell therapies (3). The mechanism underlying PsP is due to infiltration of T cells into the tumor environment leading to increased tumor appearance rather than true proliferation of tumor cells. Unrecognized PsP may cause early treatment cessation in patients who would benefit from continued immunotherapy (3).
5.2 Radiation necrosis
Due to the increasing application of combination treatment of radiotherapy and immunotherapy, and some BM patients had SRS before immunotherapy treatment, the term “RN” should be recognized in the treatment response evaluation. RN is defined as the occurrence of necrotic brain tissue after radiation therapy and usually occurs between three months and one year after radiotherapy (54). RN occurs in approximately 20% of radiotherapy in GBM patients (54) and 5%-25% of patients with BM treated with SRS (147).
PsP and RN are two different types of therapeutic effects and require completely different clinical approaches. RN can be treated conservatively with steroids or surgical removal due to a space-occupying aspect, or antiangiogenic drugs to alleviate brain edema. A pseudoprogression is usually treated conservatively.
5.3 Hyperprogresssion
Hyperprogresssion is immunotherapy-induced acceleration of tumor growth leading to premature death (3, 145, 148, 149), Figure 4.
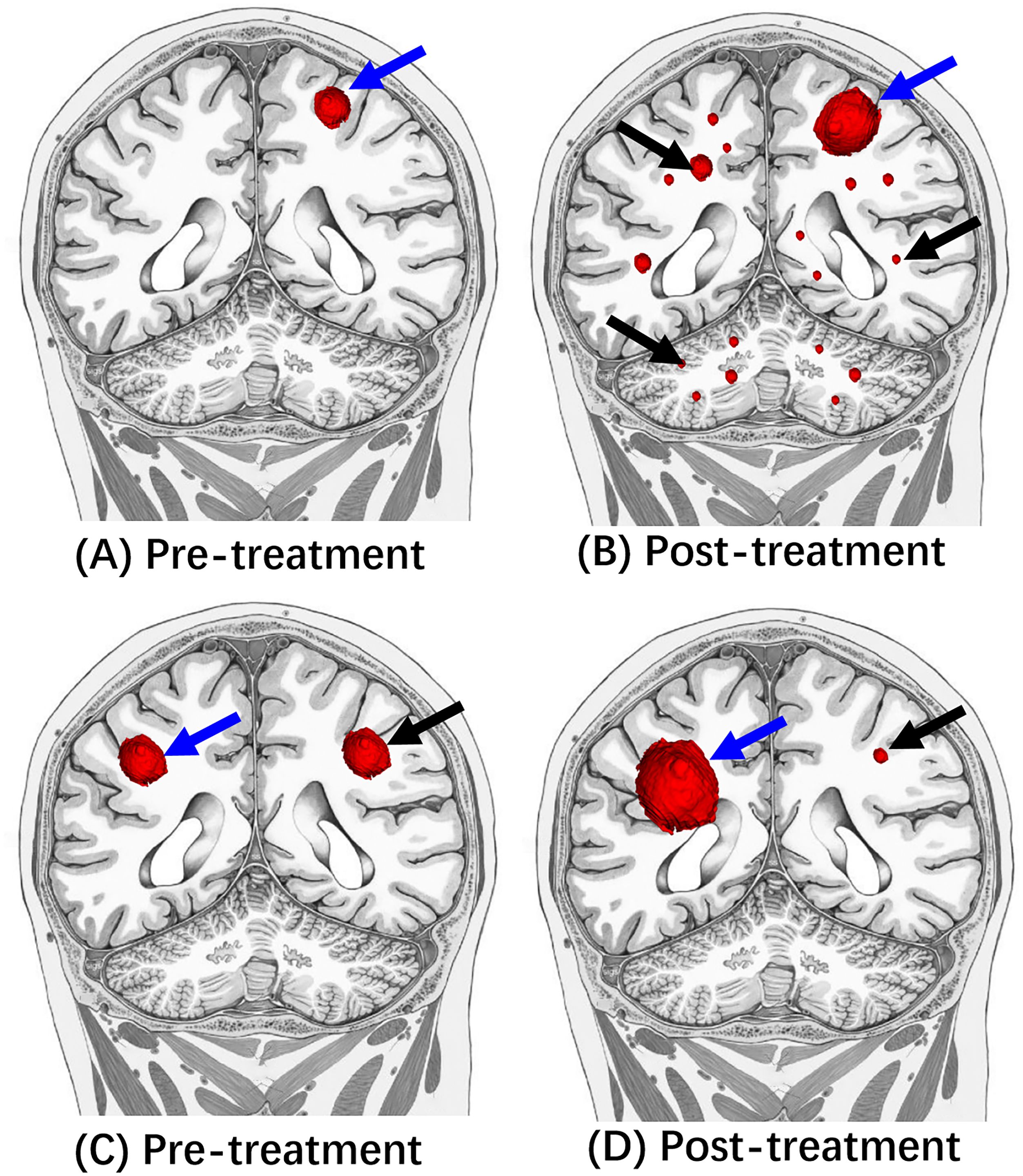
Figure 4. Illustrative diagrams of Hyperprogression and dissociated response. (A, C) Are pre-treatment MRI. Compared to (A, B) showed enlarged left frontal mass (blue arrow) and multiple new small masses (black arrow) in bilateral cerebral hemispheres and cerebellums after the immunotherapy treatment. Compared to the (C, D) showed the decreased size of left frontal mass (black arrow) after the immunotherapy treatment. In contrast, the right frontal mass (red arrow) increased the tumor size.
Various definitions of hyperprogression have been used in published studies. The pure radiological criteria define and analyze the variation of tumoral burden before and after initiation of immunotherapy, including at least three radiological assessments (one several weeks before baseline, one at baseline, and one after initiation of ICIs) (145).
Champiat et al. described “hyperprogressive disease” as a RECIST progression at the first evaluation and a twofold or greater increase in the tumor growth rate (TGR, the percentage increase in tumor volume per month) after starting PD-1/PD-L1 inhibitor therapy compared with the period before initiation of PD-1/PD-L1 inhibitor therapy (150).
Saada-Bouzid et al. evaluated tumor growth kinetics (TGK) based on the difference of the sum of the largest diameters of RECIST target lesions per unit of time between two evaluations. Hyperprogression was defined as TGK ratio (ratio of TGK after immunotherapy to pre-treatment TGK) ≥ 2 (145, 151).
Overall, the incidence of hyperprogression in solid tumors treated with immunotherapy (ICIs) differs widely in the literature, ranging from 4-29% (145, 149).
Statistically, worse overall survival was observed in patients experiencing hyperprogression than in patients experiencing standard progression. Therefore, hyperprogression has a potentially deleterious and fatal impact. Early identification of hyperprogression is crucial for patients treated with immunotherapy. Current immunotherapy regimens should be stopped promptly in case of suspected hyperprogression, and transition to other effective therapies must be performed (3, 145, 148, 149).
5.4 Dissociated response
Dissociated response, also known as a mixed response or disproportionate response, is a phenomenon demonstrating increased tumor size of some target lesions (which may qualify as progression) and decreased size or stability of additional target lesions following immunotherapy (3, 149), Figure 4.
Dissociated response may reflect the heterogeneity of tissue-specific tumor microenvironments and has been reported in less than 10% of patients (149).
Clinical management of dissociated response is still contested. Dercle et al. recommended continuing immunotherapy for durable clinical benefit despite RECIST1.1 progression (149). Kim et al. suggested combining immunotherapy with local treatment in the case of oligometastatic disease progression (3).
5.5 Abscopal response
In 1953, Mole first used the term ‘abscopal’ to report a phenomenon of out-of-field regression of unirradiated distant tumor sites following irradiation of a single tumor lesion (152). The abscopal response is an immune-mediated response to radiation by tumor cells located distant from the irradiated site. The mechanism may be related to released systemic tumor antigens (from local treatment), which activate the antitumor immune system, resulting in remote tumor regression outside of the radiation field (149). This once rare phenomenon may become more common in an era of increasing combination treatments of radiotherapy and immunotherapy (153).
5.6 Pseudo-response
Pseudo-response is a phenomenon of progression that has been reported in high-grade glioma patients treated with antiangiogenic agents and is characterized by decreased contrast enhancement and enlargement of the non-enhancing lesion on T2WI/T2-FLAIR (140). Pseudo-response indicates un-enhancing tumor infiltrative dissemination (154). The clinical use of combination therapy of antiangiogenic treatment and immunotherapy highlights the imaging challenges of treatment response assessment criteria for immunotherapy which traditionally emphasizes enhancing lesion(s) (138).
6 Advanced imaging techniques
Advances in imaging have played a significant role in improving the evaluation and outcomes of brain tumor immunotherapy, particularly in accurately identifying pseudoprogression, optimizing treatment decisions, and enhancing the ability to predict treatment response. Immunotherapy is often accompanied by complex imaging changes, making these advancements crucial for more precise assessments. One of the major challenges in post-immunotherapy treatment imaging is the assessment of treatment effects, (i.e. distinguishing RN, PsP from TP, detection and monitoring of immune-related adverse events(irAEs)) which guide optimal clinical decision-making.
It is usually difficult to distinguish between RN-PsP and TP, as they may have similar morphology, contrast enhancement, edema, and mass effect on conventional MR. Advanced imaging techniques including MR perfusion-weighted imaging (PWI), magnetic resonance spectroscopy (MRS), chemical exchange saturation transfer (CEST), and positron emission tomography (PET), have been widely used in treatment response assessment of brain tumors, and have been suggested in RANO 2.0 criteria (141). These technologies provide precise data on tumor metabolism, blood flow, and biochemical characteristics, enabling clinicians to detect the actual response to immunotherapy earlier and more accurately and improve the post-treatment differential diagnosis between RN-PsP and TP.
Furthermore, the integration of radiomics and artificial intelligence (AI) into modern imaging techniques has enhanced the level of personalization in treatment. By efficiently analyzing large-scale imaging data and extracting hidden biological features, AI provides precise treatment feedback, enabling the accurate identification of patients who are responsive to immunotherapy. This improves treatment outcomes and extends patient survival. This data-driven decision-making approach has already influenced clinical treatment pathways, making immunotherapy for brain tumor patients more targeted and effective.
6.1 MR PWI
Of all advanced imaging techniques, MR PWI is the most common clinical practice to discriminate RN-PsP from TP. MR PWI provides hemodynamic information regarding tumor angiogenesis in brain tumors. There are three MR PWI sequences, including dynamic susceptibility contrast (DSC), dynamic contrast-enhanced (DCE), and arterial spin labeling (ASL). Compared to DCE, which is less commonly used, DSC and ASL are major and validated perfusion imaging tools for the diagnosis and management of brain tumors. MR DSC-PWI estimates the relative cerebral blood volume (rCBV) based on T2* signal intensity changes from the first passage of paramagnetic contrast agents through the cerebrovascular system. ASL measures cerebral blood flow (CBF) noninvasively using endogenous tracers of magnetized blood instead of contrast agents.
A key pathology characteristic of TP and tumor recurrence in malignant brain tumors is angiogenesis, which results in increased rCBV. In contrast, inflammation and necrotic tissue damage lead to decreased rCBV in RN and PsP. TP has significantly higher rCBV, associated with increased metabolic activity and increased neovascularization, than RN and PsP. These differences help differentiate between RN-PsP and TP in the era of radiation and chemoradiation treatment.
Multiple studies have demonstrated that the mean and maximal rCBV (rCBVmax) of TP in malignant gliomas were significantly higher than in PsP lesions (155–158). Anil et al. suggested the optimum standardized rCBV threshold of 1.64 for distinguishing tumor recurrence and post-treatment radiation effects (with a sensitivity of 84.48%, specificity of 84.97%, and accuracy of 84.73%). In contrast, the objective threshold of 1.75 obtains a sensitivity of 81.94%, specificity of 87.23%, and accuracy of 84.58% (157).
Previous studies also found that rCBV values of RN in BM were significantly lower than the rCBV values of TP and tumor recurrence (159–163). The recommended rCBV cut-off points in these studies ranged from 1.23 to 2.12. Mitsuya et al. analyzed 7 recurrent BM lesions and 21 RN lesions in 27 patients with BM undergoing SRS; they reported the best distinguishing cutoff was 2.1, with a sensitivity of 100% and a specificity of 95% (160). Umemura et al. assessed the ability of dynamic contrast-enhanced T1 MRI (DCE-MRI) perfusion to identify pseudoprogression in melanoma brain metastases, reporting an AUC of 0.75 for this diagnostic method (164).
There are few MR PWI studies evaluating the treatment response assessment in brain tumors following immunotherapy (165, 166), Figures 2 and 4. Cuccarini et al. analyzed 18 TP and 8 PsP lesions in 22 patients with newly diagnosed GBM treated with dendritic cell immunotherapy; they found that the difference of △rCBVmax could effectively differentiate tumor recurrence from PsP, with a sensitivity of 67% and specificity of 75% (p = 0.004), suggesting that the rCBV modifications over time might be more helpful to distinguish PsP from TP (167). In a retrospective study by Vrabec et al. (168), the authors reviewed 32 follow-up MRI examinations in 8 recurrent GBM patients treated with dendritic cell immunotherapy; they found the highest rCBVmax value (9.25 ± 2.68) was observed in the contrast-enhancing area of TP lesions, and the rCBVmax value was higher even at time points before definite progression (4.87 ± 1.61) compared to the rCBVmax value of stable lesions (1.22 ± 0.47). They suggested that the rCBVmax value was a potential radiological indicator to distinguish between immunotherapy-induced inflammatory response and recurrent GBM tumor growth. In a study that enrolled 79 examinations with DSC-PWI in 6 surgically/immunogene-treated GBM patients and two surgically treated GBM patients, Stenberg et al. showed that elevated rCBV, corresponding to the contrast-enhancing lesion, supports the diagnosis of recurrent GBM (169).
In a study conducted by Song et al. (170), they demonstrated that out of the 19 patients with recurrent GBM treated with ICIs, 12 were determined to have TP. In contrast, 7 had treatment responses after 6 months of ICI treatment. The authors found that pre-ICI and post-ICI treatment rCBV values of the TP group were higher (2.16 and 2.2 respectively) than the rCBV values of the treatment response group (pre-treatment, 1.67, p=0.25, and post-ICI treatment, 1.90, 0.89). However, it is interesting that the post-ICI treatment rCBV values of both the TP group and the treatment response group were increased compared to the pre-ICI treatment rCBV values. The authors thought that the absolute rCBV value or interval change in rCBV was not indicative of treatment response within 6 months. A possible reason for the absence of a significant difference in rCBV parameters between the TP group and the treatment response group was speculated to be related to the confounding effects of anti-angiogenesis treatment (165). In Song et al’s study, there were 5 patients (26.3%) treated with bevacizumab before the commencement of ICIs and remained on this therapy throughout the entire period of study follow-up. The bevacizumab can result in “pseudo-response” non-enhancing TP accompanied by decreased rCBV in malignant gliomas which may limit the generalizability of the findings.
6.2 MRS
MRS is a non-invasive molecular imaging technique that detects the local resonance frequencies of hydrogen nuclei in various biochemical compounds, enabling in vivo quantification of metabolites within tissues (54, 171). The major metabolites obtained in MRS include N-acetyl-aspartate (NAA), a neuronal viability marker, choline (Cho), indicative of cell membrane turnover, creatine (Cr), a stable internal standard, lactate, marking anaerobic metabolism, and myo-inositol, a marker of gliosis. These metabolites provide crucial insights into the biochemical environment of brain tumors and have been widely used in multiple studies and clinical practice (172).
MRS can differentiate between primary brain tumors and BM based on spectral patterns of metabolites (171). Primary brain tumors, such as GBMs, generally shows elevated Cho/Cr and Cho/NAA ratios due to increased cell membrane turnover and proliferation. BM is characterized by elevated Cho, lactate, and lipids, and the absence of NAA. The metabolite ratios of lipid/Cho and lactate/Cr can be used to differentiate RN-PsP from TP (171). A recent meta-analysis suggested that MRS had the highest diagnostic accuracy in distinguishing between treatment-related changes and tumor recurrence, with a sensitivity and specificity of 91% and 95% (172).
Furthermore, Floeth et al. found that the metabolic data of MRS may help to distinguish between tumor recurrence and PsP after local immunotherapy of GBM (173). However, MRS studies in the BM treated with immunotherapy are still limited.
6.3 CEST
CEST is another non-invasive advanced molecular imaging technique that focuses on the concentration and exchange rates of mobile proteins and peptides within brain tissue by monitoring the exchange of protons between mobile proteins or peptides and surrounding water molecules (174). For instance, amide proton transfer-chemical exchange saturation transfer (APT-CEST) can be used to measure protein concentrations, and glucoCEST can assess glucose metabolism; both processes are higher in brain tumors compared to normal brain tissue (99). CEST provides endogenous MRI contrast without the need for exogenously administered contrast agents. Thus, CEST is a valuable tool in the analysis of tumor microenvironment (174).
CEST shows great potential in glioma grading and monitoring treatment response (172).
In the context of post-immunotherapy treatment imaging, CEST imaging, especially APT-CEST, has shown significant promise. Studies have demonstrated its ability to distinguish between tumor recurrence and treatment-related changes such as PsP in patients with BM and gliomas (172). Due to increased protein and peptide content in viable tumor components, TP or tumor recurrence of gliomas presents a high signal on APT-weighted CEST images, while PsP shows iso- to mild hyperintensity (172). Ma et al. (175) applied amide proton transfer-weighted (APTW) MRI to distinguish pseudoprogression from true progression in 32 patients with malignant gliomas after chemoradiation. They reported an optimal cutoff APTWmean of 2.42%, achieving a sensitivity of 85.0% and a specificity of 100%. CEST could accurately and promptly distinguish between PsP and TP in brain tumors after immunotherapy. Thus, CEST imaging greatly improves the comprehensive post-immunotherapy treatment assessment and clinical management in patients with brain tumors.
6.4 PET
PET is a nuclear medicine imaging technique that evaluates metabolic activity using radioactive tracers (147, 171). PET can distinguish between RN-PsP and TP based on metabolic differences. Specifically, TP and tumor recurrence lesions usually demonstrate active/increased metabolism. In contrast, RN and PsP have decreased/inactive decreased/tracer uptake (147, 171). Although the 18F-fluorodeoxyglucose (FDG) is the only FDA-approval tracer in the US, the effectiveness of FDG-PET in the treatment response assessment of brain tumors is limited, primarily due to underlying brain metabolism and spatial resolution (3, 171).
Various amino acid-based radiotracers, such as L-methyl-11C-methionine (MET), O-2-18F-fluoroethyl-L-tyrosine (FET), and 3,4-dihydroxy-6-18fluoro-L-phenylalanine (FDOPA), can effectively distinguish between tumor recurrence, PsP, and RN in malignant gliomas and BM (174). Previous studies have demonstrated the potential of PET/MRI in evaluating the therapeutic response of GBM, the potential benefits of FLT-PET/MRI for diagnosing melanoma BM and monitoring targeted immunotherapy treatments (176). In a study of immunotherapy with DC vaccination in GBM patients, 18F-FET PET imaging showed more accurate discernment than contrast-enhanced MRI initially (177). In addition, Joseph et al. speculated that the PET probe for deoxycytidine kinase (dCK) could be used to distinguish between immune inflammatory response and enhancement foci caused by other factors in contrast-enhanced MRI imaging (178). Galldiks et al. assessed 18F-FET PET for monitoring immune checkpoint and targeted therapy responses in 40 patients with melanoma and lung cancer BM, identifying a tumor-to-brain ratios threshold of 1.95 to distinguish relapse from treatment effects, with an accuracy of 85% (179).
Immuno-PET is an advanced imaging technique that shifts the focus from traditional tumor cell visualization to the comprehensive analysis of the tumor’s immune environment. This method employs radiotracers to target specific immune markers such as PD-L1, CD8, and various macrophage-associated biomarkers, providing valuable insights into the density, composition, and functional state of tumor-infiltrating leukocytes. Such imaging can predict the efficacy of immunotherapy and overall prognosis more accurately than traditional methods. This method offers a whole-tumor perspective that surpasses the limitations of single biopsy samples. In the future, the optimal strategy may shift from non-immune-specific imaging biomarkers to immune-specific biomarkers assessed with immuno-PET (180).
The performances of advanced imaging modalities in distinguishing treatment-related effects from true tumor progression are summarized in Table 5.
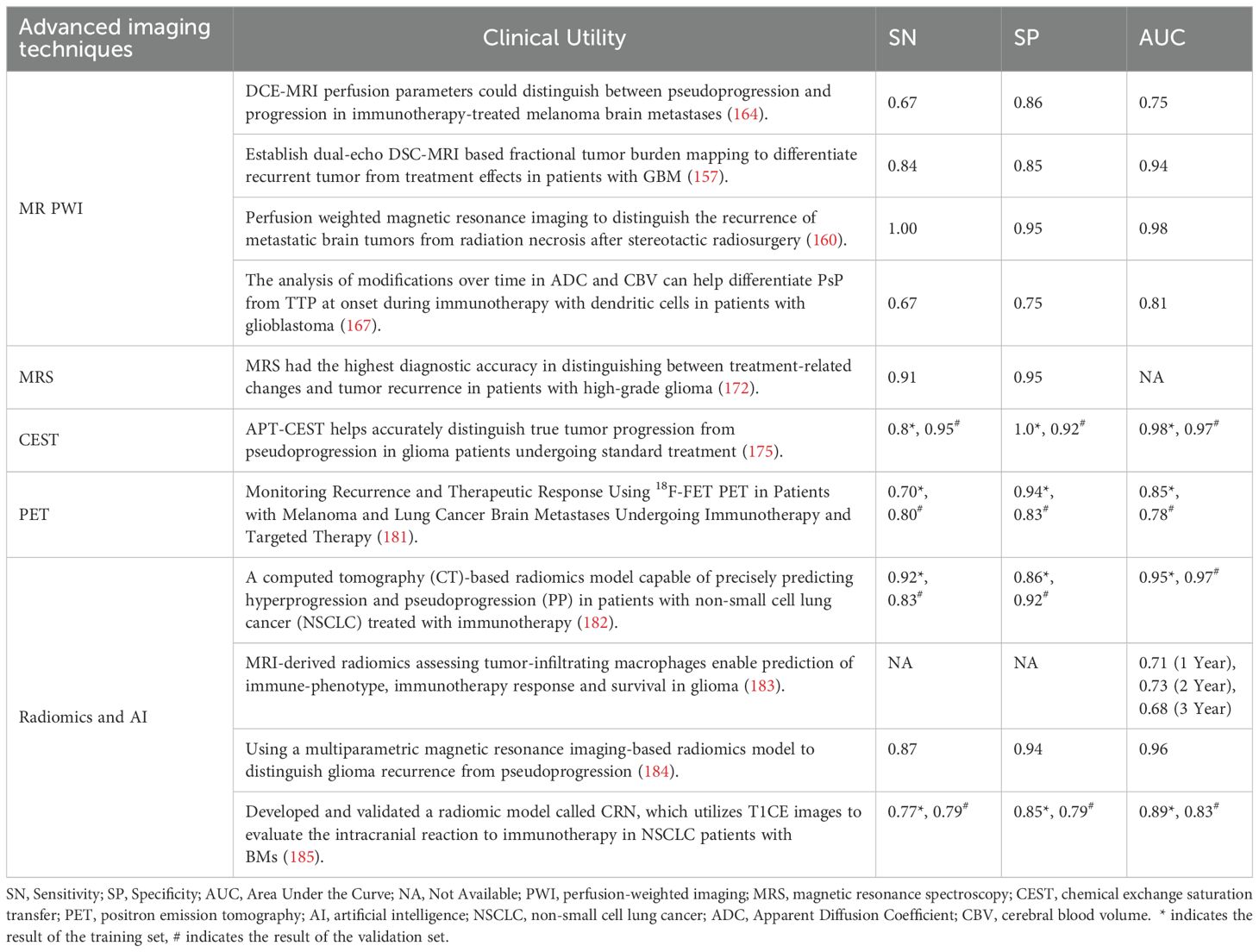
Table 5. Performance review of advanced imaging modalities in differentiating treatment-related effects with true tumor progression.
6.5 Radiomics and AI
Radiomics is a rapidly developing field of research focused on the extraction of quantitative features from medical images, thus converting these digital images into minable, high-dimensional data, which offer unique biological information that can enhance our understanding of disease processes and provide clinical decision support (186). Artificial intelligence (AI) essentially simulates human cognitive and decision-making abilities through machine learning and deep learning algorithms. It relies on continuously adapting and learning from large-scale data to optimize models, thereby enhancing their capability to handle complex tasks (187).
The combination of artificial intelligence methods and radiomics has been widely applied in tumor diagnosis, classifications (e.g., primary and secondary tumors), discriminations between treatment effects (pseudoprogression, radiation necrosis) and true progression, survival prediction, inflammation, and identification of tumor biomarkers (188). These models can predict the efficacy of ICI through imaging features, accurately identifying patients who may benefit from ICI therapy, thus advancing the development of personalized immunotherapy. In addition, AI can discover new immunotherapy targets, aiding in the design of targeted treatment strategies. Through deep learning techniques, AI has revealed mechanisms of resistance and patterns of recurrence in tumor immunotherapy by exploring multi-omics data, helping to understand the molecular mechanisms of resistance.
Currently, the application of AI algorithms and radiomics in solid tumors beyond the CNS has demonstrated great potential in predicting the efficacy of immunotherapy (189). Sinha and colleagues have summarized that AI or machine-learning (ML) models can accurately predict immune therapy responses, progression-free survival, and overall survival in NSCLC patients (189). Li and colleagues used radiomics to develop predictive models for pseudoprogression and high progression in NSCLC patients receiving ICI therapy. The AUC values for the training and validation sets were 0.95 and 0.88, respectively (182). Additionally, Shu and colleagues (190) emphasized that radiomics helps predict immune checkpoint inhibitor-related pneumonitis (ICIP) in NSCLC patients, providing new methods and insights for the early diagnosis and management of severe adverse reactions caused by immunotherapy. Furthermore, Prelaj and colleagues (191) demonstrated that AI-based approaches, combined with radiomics and pathology, have expanded the discovery of new biomarkers for immunotherapy.
Compared to research on solid tumors in other body systems,the studies on the application of AI are relatively fewer in brain tumors. In particular, there are still many unmet needs in predicting the response to immunotherapy. The timing of pseudoprogressive changes in BM patients treated with ICIs has not been fully explored, reports on hyperprogression after initiation of ICI monotherapy remain scarce (181). However, preliminary studies have shown that AI algorithms exhibit potential for predicting survival outcomes and treatment responses after immunotherapy (99, 192).
The use of AI in glioblastoma patients primarily focuses on diagnosis and treatment planning (193). Studies have found that MRI-derived radiomics assessing tumor-infiltrating macrophages enable prediction of immune-phenotype, immunotherapy response and survival in glioma (183). The immunoradiomics model they developed could allow for non-invasive assessment of the absolute density of tumor-associated macrophages from MRI images in HGG patients, and effectively predicted the patients’ survival outcomes (183). Additionally, Fu and colleagues (184) developed a radiomics-based model to differentiate between glioma recurrence and pseudoprogression, with the best predictive model achieving an AUC of 0.96 (Table 5). AI models have also shown potential in predicting immune-related adverse events (irAEs) and patient survival, helping to mitigate the risks associated with more potent immunotherapies (180). Sinigaglia and colleagues found that ML algorithms and AI signatures were trained to predict overall survival in patients with solid tumors treated with ICM based on pretreatment-imaging biomarkers. These biomarkers, are identified as predictors of poorer outcomes (193).
In brain metastases (BMs), AI and radiomics are not only used to identify the type and mutation status of the primary tumor but are also increasingly applied to assess tumor response after immunotherapy (99). Xu and colleagues (185) successfully used AI and radiomics to predict progression-free survival and overall survival in brain metastasis patients receiving ICIs. Their research suggests that pre-treatment radiomic features may help in the early prediction of treatment benefits. Galldiks and colleagues found that imaging parameters obtained through amino acid PET, including radiomic features, have significant value in the diagnosis of recurrence and evaluation of treatment efficacy in brain metastasis patients (194). Xu and colleagues’ research further indicated that radiomic biomarkers from pre-treatment MRI images can effectively predict intracranial response (185). However, studies on the use of neuroimaging biomarkers for non-invasive detection remain relatively limited.
AI and radiomics have demonstrated great potential in neuro-oncology, providing advanced tools for diagnosis, treatment monitoring, and prognostic evaluation. By characterizing tumor biology through medical imaging, radiomics combined with AI-derived biomarkers offers hope for personalized medicine in the era of immunotherapy. Compared to traditional imaging techniques, AI demonstrates higher sensitivity and accuracy in assessing immune responses in neuro-oncology. Using radiomics, AI extracts complex micro-level features and can detect early immune responses before visible changes in the tumor (195). AI reduces human error and improves the consistency and standardization of diagnostic and treatment decisions. Additionally, it tailors predictions based on individual patient characteristics (e.g., imaging, genetics, clinical data), providing strong support for personalized and precise treatments (196).
Despite the rapid development of ML technologies in recent years, their broad application in neuro-oncological radiology still faces numerous challenges. Firstly, AI models rely on large datasets for training, but the limited sample sizes in neuro-oncology immune therapy studies reduce their effectiveness. Secondly, inconsistencies in imaging data from different devices lead to unstable model performance across datasets, limiting widespread AI adoption. Additionally, poor model interpretability and a lack of transparency in decision-making reduce clinical trust (197). Lastly, most AI models rely on static imaging data, while immune therapy effects evolve over time, limiting the models’ ability to predict dynamic responses (198).
In summary, despite the significant potential of AI in predicting immune therapy responses and outcomes, future research must address issues such as data sample limitations, cross-device compatibility, and model interpretability. Resolving these challenges is essential for the broader clinical application of AI and to enhance precision in personalized medicine.
7 Immune-related CNS adverse events and the spectrum of imaging manifestations
Immunotherapy can produce a spectrum of toxicity involving organs due to autoimmune effects from misdirected stimulation of the immune system, termed irAEs. The irAEs include pneumonitis, colitis, hepatitis, pancreatitis, thyroiditis, hypophysitis, synovitis, arthritis, and even sarcoid-like granulomatosis and lymphadenopathy. irAEs have been an increasingly recognized concept in cancer imaging over the past few years. The mechanism of irAEs is presumed to be autoimmune effects resulting from misdirected stimulation of the immune system during immunotherapy. In addition to the autoimmune effects, the T-cell inflammatory responses can produce swelling in the targeted tumor microenvironments that resemble tumor growth, as well as disease spread into susceptible healthy tissues. Thus, it can be difficult to distinguish between response and progression during early treatment. The accurate recognition of pseudoprogression and early diagnosis of irAEs is essential to the clinical management of immunotherapies (148, 149).
Due to the rapidly increasing uses of immunotherapy in clinical neuro-oncology practices, there are increasing demands for neuroradiologists and clinicians to be familiar with imaging manifestations of irAEs in CNS. The irAEs in CNS include encephalopathy, ICIs-induced autoimmune or limbic encephalitis, hypophysitis, posterior reversible encephalopathy syndrome (PRES), multiple sclerosis (MS), aseptic meningitis, transverse myelitis, necrotizing myelopathy, and vasculitis (199).
Hypophysitis is the most common pattern of the CNS irAEs, this immune-related endocrinopathy can be observed in approximately 10%–13% of patients with melanoma treated with ipilimumab, Figure 5.
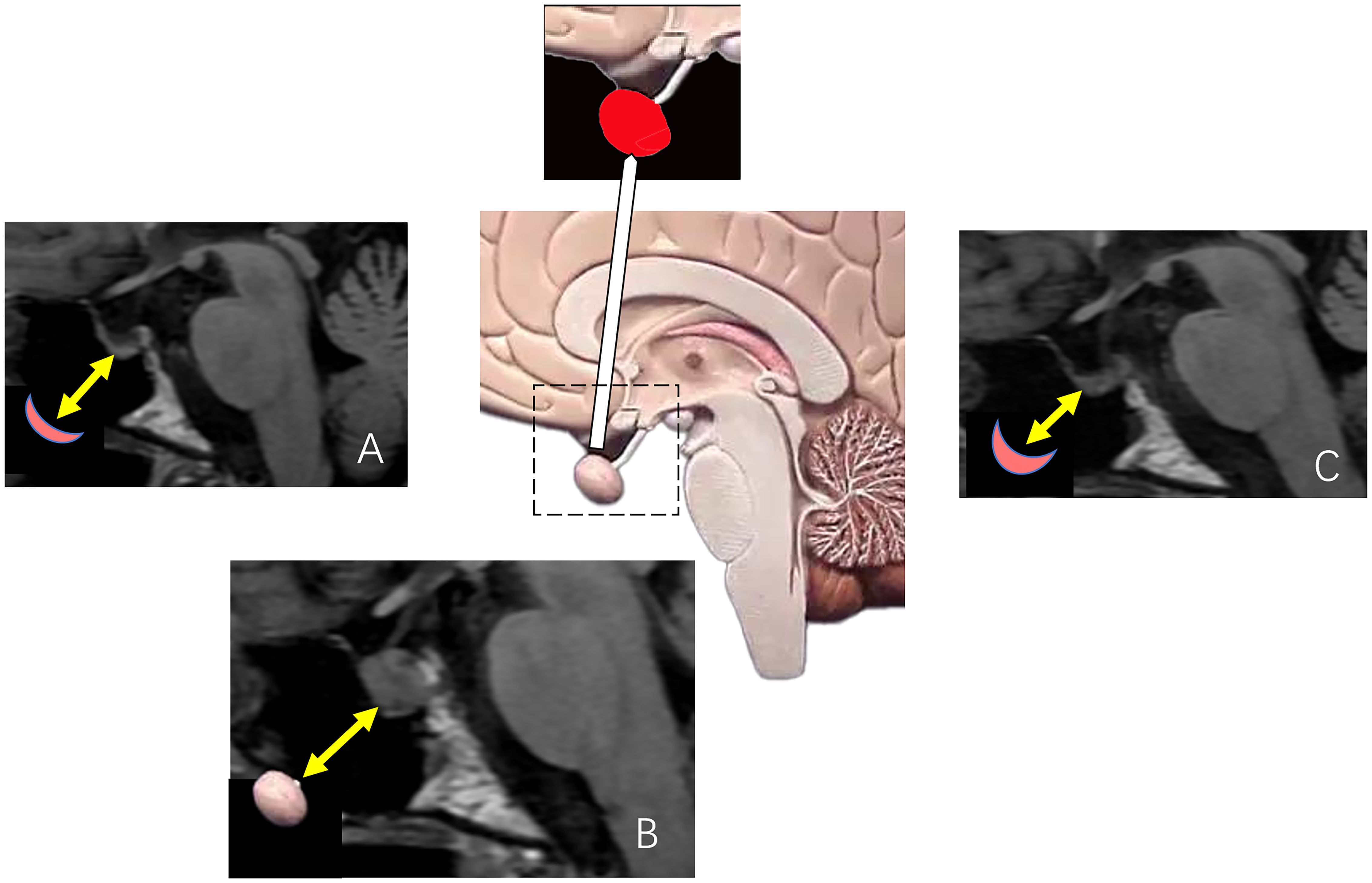
Figure 5. The diagram of the pituitary gland and an illustrative case of hypophysitis induced by ipilimumab treatment. (A–C) Are sagittal T1-FLAIR images of a patient with melanoma treated with ipilimumab. (A) Is a pre-immunotherapy MRI showing the unremarkable size of the pituitary gland (yellow arrow). During the ipilimumab treatment, the patient presented a sudden onset of intractable headache and nausea. (B) Demonstrated significant and heterogeneous enlargement of the pituitary gland (yellow arrow). Follow-up MRI after 6 weeks, (C) showed resolution of pituitary enlargement (yellow arrow).
The clinical symptoms of immune-related hypophysitis are commonly headache, fatigue, weakness, and/or rarely visual defects (likely because the enlargement of the pituitary gland is usually mild). Hypophysitis diagnosis is generally based on the development of new hypopituitarism and pituitary enlargement at imaging after initiation of immunotherapy without an alternative etiology. Enlarged pituitary glands may demonstrate homogeneous or heterogeneous enhancement at MRI and thickening of the pituitary stalk may also be present. Resolution of pituitary enlargement after high-dose corticosteroid administration treatment can be observed at follow-up MRI (148, 149, 199).
Kurokawa et al. suggested an additional MRI feature of ICI-induced hypophysitis as hypo-enhancing geographical areas of low T2 signal in the anterior pituitary without blooming artifact. This pattern typically reflects fibrosis rather than necrosis or hemorrhage (200).
This specific MRI characteristic provides a way to distinguish ICI-induced hypophysitis from other types of hypophysitis or neoplasms (148, 149, 199, 200).
Autoimmune encephalitis induced by ICIs lacks distinctive MRI patterns, MRI may reveal T2/FLAIR hyperintensities affecting white and deep gray matter, including the lentiform nuclei and external capsule/claustrum (201). Limbic encephalitis has also been reported with characteristic MRI appearance of symmetrical inflammatory changes in bilateral mesial temporal lobes. The variability in MRI findings, coupled with often negative results in auto-immune antibody panels, contributes to diagnostic challenges (202).
8 Challenges and perspective
Despite many promising neuro-imaging studies in brain tumors treated with immunotherapy, there are still multiple challenges in this field, especially for the successful application of advanced imaging techniques.
8.1 Physical limitations: size and location
1a. Due to the inherent limitation of imaging resolution, advanced imaging techniques can’t be used for small lesions. Additionally, these imaging techniques are often limited for cystic gliomas or cystic BMs, where the thin wall may result in an undetectable rCBV (54, 171, 174).
1b. The proximity of brain tumor lesions to the cerebral cortex and skull limits the application of advanced imaging techniques, primarily due to artifactual signals.
8.2 Pathological limitations
2a. A leptomeningeal lesion, especially leptomeningeal metastasis, is an important TP pattern in malignant brain tumors. However, the evaluation of a solitary leptomeningeal lesion and/or ependymal lesion (Figure 6) is often limited by advanced imaging techniques due to their location and they were excluded from the treatment response assessment of the RANO criteria.
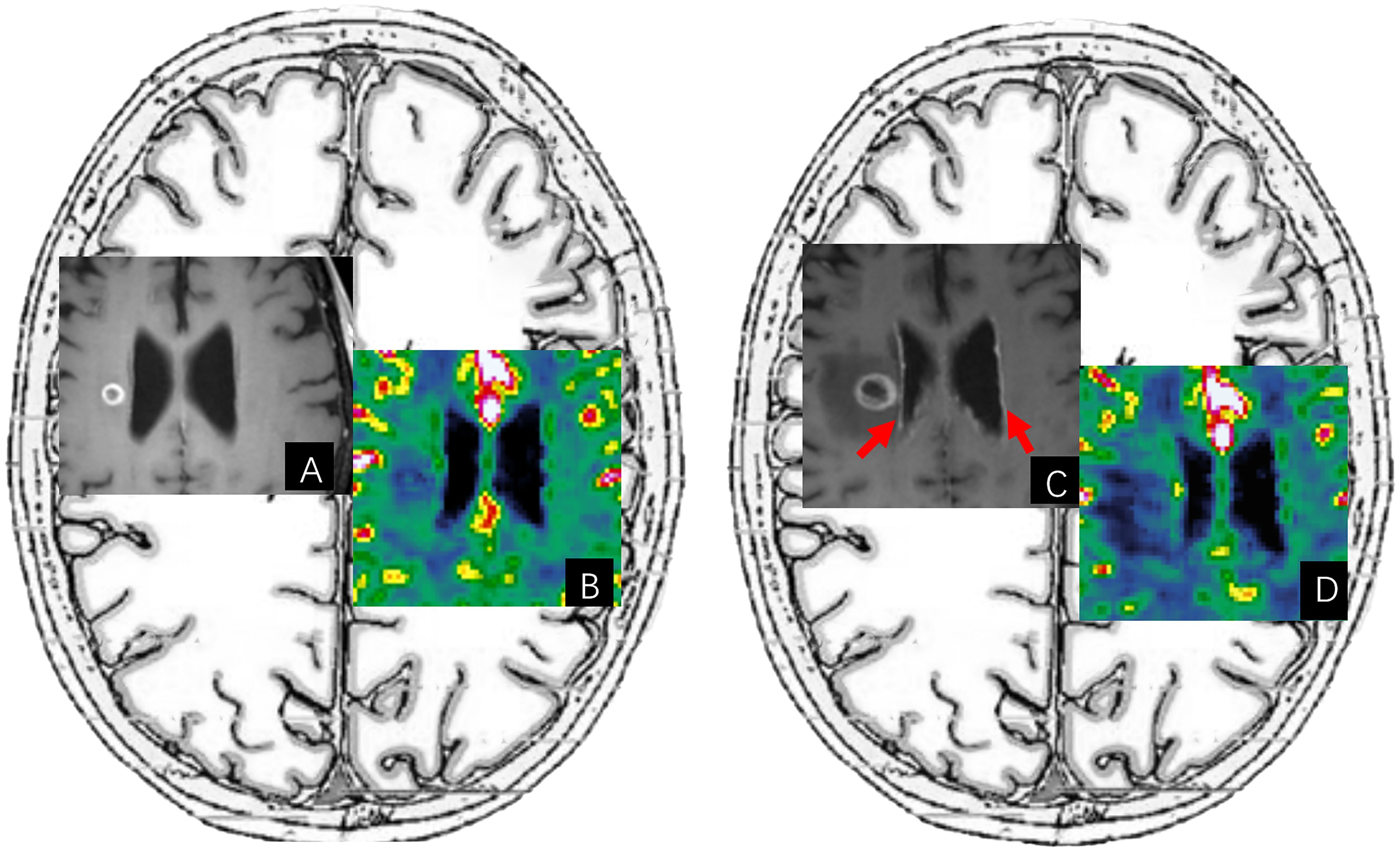
Figure 6. An illustrative case of diffuse subependymal progression after immunotherapy. (A, B) Are pre-immunotherapy post-contrast T1-FLAIR images and CBV maps of a patient with BM from renal cell carcinoma. After immunotherapy treatment, (C) of the post-contrast T1-FLAIR image showed diffuse subependymal enhancement (red arrow) in the lateral ventricles, accompanied by an enlarged ring-enhancing lesion and increased surrounding edema. These foci of subependymal enhancement were too small to be evaluated on the CBV map of (D).
2b. RN or PsP in the acute phase can present elevated rCBV which can create diagnostic uncertainty (171).
2c. Hemorrhage is a common pathologic feature, especially in post-treatment brain tumors. The MR PWI is limited by magnetic susceptibility artifacts due to petechial hemorrhage or melanin in melanomas.
2d. It has been noted that a large overlap of CBVmax values exists between the RN-PsP group and the TP group, leading to image interpretation challenges. This effect may be explained by pathologic characteristics including tumor heterogeneity and the similarity between microvascular density in active tumors and hyperplastic dilated blood vessels in post-radiation changes (54).
2e. Biopsy with histopathologic evaluation remains the gold standard to differentiate RN-PsP from TP. However, stereotactic biopsies can introduce sampling bias (147). In addition, it is common for the co-existence of RN and some active tumor/tumor or tumor recurrence in the tissue samples RN, the percentage is variable and possibly depends on the time of evolution (171).
2f. Bevacizumab, an antiangiogenic agent, inhibits vascular endothelial growth factor and thereby decreases endothelial cell proliferation and new blood vessel formation. After the administration of Bevacizumab, a rapid decrease in contrast enhancement and edema can be observed transiently (54). Bevacizumab can cause “tumor vasculature normalization” which subsequently leads to a decrease in perfusion within the tumor. Thus, combining immunotherapy and antiangiogenic treatment in malignant brain tumors may result in confounding rCBV interpretation, especially in the atypical non-enhancing TP pattern of “pseudoresponse”.
Despite the above imaging challenges, there is an urgent need for better imaging concepts to adapt to the novel patterns of immunotherapy response, including hyperprogression, PsP, and irAEs. Furthermore, the identification of risk factors and the establishment of comprehensive predictive models could help clinicians evaluate the benefit-risk ratio before starting immunotherapy in the future.
9 Conclusion
MRI plays an important role in the assessment of immunotherapy related treatment response, progression of brain tumors, and detection/monitoring for irAEs. The post-immunotherapy treatment imaging changes in brain tumors are more complicated. Novel imaging advancements should be implemented for improved post-immunotherapy treatment assessment in brain tumors, better personalized medicine, and improved management of such primary and metastatic brain malignancies patients.
Author contributions
XL: Investigation, Methodology, Resources, Writing – original draft, Writing – review & editing. HC: Data curation, Investigation, Methodology, Writing – original draft, Writing – review & editing. GT: Investigation, Supervision, Writing – original draft, Writing – review & editing. LZ: Conceptualization, Data curation, Investigation, Writing – review & editing. HJ: Investigation, Methodology, Writing – review & editing. SS: Investigation, Supervision, Validation, Writing – review & editing. HW: Methodology, Resources, Supervision, Writing – original draft, Writing – review & editing.
Funding
The author(s) declare financial support was received for the research, authorship, and/or publication of this article. Project Supported for Scientists in the Bureau of Science and Technology of ShaoGuan (Grant No. 230329238033088). Project Supported for Scientists in the Bureau of Science and Technology of ShaoGuan (Grant No. 220531214533619). Project Supported for the National Natural Science Foundation of China (No. 82202983). Project Supported for the Peking University Clinical Scientist Training Program, funded by the Fundamental Research Funds for the Central Universities.
Conflict of interest
The authors declare that the research was conducted in the absence of any commercial or financial relationships that could be construed as a potential conflict of interest.
Publisher’s note
All claims expressed in this article are solely those of the authors and do not necessarily represent those of their affiliated organizations, or those of the publisher, the editors and the reviewers. Any product that may be evaluated in this article, or claim that may be made by its manufacturer, is not guaranteed or endorsed by the publisher.
Glossary
CNS: central nervous system
FDA: food and drug administration
PD-1: programmed cell death protein 1
PD-L1: programmed death ligand-1
CTLA-4: cytotoxic T-lymphocyte-associated protein 4
ICI: Immune checkpoint inhibitor
NSCLC: non-small cell lung cancer
RCC: renal cell carcinoma
TNBC: triple-negative breast cancer
TIM-3: T cell immunoglobulin and mucin domain-containing protein 3
HCC: hepatocellular carcinoma
TIGIT: T cell immunoreceptor with Ig and immunoreceptor tyrosine‐based inhibition motif domains
SCLC: small cell lung cancer
NK: natural killer
HPV: human papillomavirus
HBV: hepatitis B virus
ACT: Adoptive Cell Therapy
TIL: Tumor-Infiltrating Lymphocytes
TCR: T-cell receptors
CAR-T: Chimeric Antigen Receptor T cells
ALL: acute lymphoblastic leukemia
IL-2: interleukin-2
IL-15: interleukin-15
IL-21: interleukin-21
TNF: Tumor necrosis factor
BBB: blood-brain barrier
GBM: Glioblastoma multiforme
BM: brain metastases
T-VEC: Talimogene laherparepvec
CAR-NK: Chimeric Antigen Receptor-Natural Killer
PCNSL: Primary central nervous system lymphoma
RANO: Response Assessment in Neuro-Oncology
RANO-HGG: response criteria for high-grade gliomas
RANO-LGG: response criteria for low-grade gliomas
RANO-BM: RANO Criteria for brain metastases
mRANO: Modified RANO Criteria
CR: complete response
PR: partial response
SD: stable disease
PD: progressive disease
TGR: tumor growth rate
TGK: tumor growth kinetics
RN: radiation necrosis
PsP: Pseudoprogression
TP: tumor progression
PWI: perfusion-weighted imaging
MRS: magnetic resonance spectroscopy
CEST: chemical exchange saturation transfer
PET: positron emission tomography
DSC: dynamic susceptibility contrast
DCE: dynamic contrast-enhanced
ASL: Arterial spin labeling
rCBV: relative cerebral blood volume
CBF: cerebral blood flow
irAEs: immune-related adverse events immune-related adverse events
NAA: N-acetyl-aspartate
Cho: choline
Cr: creatine
APT-CEST: amide proton transfer-chemical exchange saturation transfer
FDG: 18F-fluorodeoxyglucose
MET: L-methyl-11C methionine
FET: O-2-18F-fluoroethyl-L-tyrosine
FDOPA: 3,4-dihydroxy-6-18fluoro-L-phenylalanine
AI: artificial intelligence
ML: machine learning
MS: multiple sclerosis
PRES: posterior reversible encephalopathy syndrome
References
2. Falchi L, Vardhana SA, Salles GA. Bispecific antibodies for the treatment of B-cell lymphoma: promises, unknowns, and opportunities. Blood. (2023) 141:467–80. doi: 10.1182/blood.2021011994
3. Kim N, Lee ES, Won SE, Yang M, Lee AJ, Shin Y, et al. Evolution of radiological treatment response assessments for cancer immunotherapy: from irecist to radiomics and artificial intelligence. Korean J Radiol. (2022) 23:1089–101. doi: 10.3348/kjr.2022.0225
4. Handy CE, Antonarakis ES. Sipuleucel-T for the treatment of prostate cancer: novel insights and future directions. Future Oncol. (2018) 14:907–17. doi: 10.2217/fon-2017-0531
5. Korman AJ, Garrett-Thomson SC, Lonberg N. The foundations of immune checkpoint blockade and the ipilimumab approval decennial. Nat Rev Drug Discovery. (2022) 21:509–28. doi: 10.1038/s41573-021-00345-8
6. Ivashko IN, Kolesar JM. Pembrolizumab and nivolumab: pd-1 inhibitors for advanced melanoma. Am J Health Syst Pharm. (2016) 73:193–201. doi: 10.2146/ajhp140768
7. Smith DA, Kikano E, Tirumani SH, de Lima M, Caimi P, Ramaiya NH. Imaging-based toxicity and response pattern assessment following car T-cell therapy. Radiology. (2022) 302:438–45. doi: 10.1148/radiol.2021210760
8. Marei HE, Hasan A, Pozzoli G, Cenciarelli C. Cancer immunotherapy with immune checkpoint inhibitors (ICIs): potential, mechanisms of resistance, and strategies for reinvigorating T cell responsiveness when resistance is acquired. Cancer Cell Int. (2023) 23:64. doi: 10.1186/s12935-023-02902-0
9. Tian T, Li Z. Targeting Tim-3 in cancer with resistance to PD-1/PD-L1 blockade. Front Oncol. (2021) 11:731175. doi: 10.3389/fonc.2021.731175
10. Harjunpää H, Guillerey C. Tigit as an emerging immune checkpoint. Clin Exp Immunol. (2020) 200:108–19. doi: 10.1111/cei.13407
11. Janes ME, Gottlieb AP, Park KS, Zhao Z, Mitragotri S. Cancer vaccines in the clinic. Bioeng Transl Med. (2024) 9:e10588. doi: 10.1002/btm2.10588
12. Abd-Aziz N, Poh CL. Development of peptide-based vaccines for cancer. J Oncol. (2022) 2022:9749363. doi: 10.1155/2022/9749363
13. Lopes A, Vandermeulen G, Préat V. Cancer DNA vaccines: current preclinical and clinical developments and future perspectives. J Exp Clin Cancer Res. (2019) 38:146. doi: 10.1186/s13046-019-1154-7
14. Gote V, Bolla PK, Kommineni N, Butreddy A, Nukala PK, Palakurthi SS, et al. A comprehensive review of mRNA vaccines. Int J Mol Sci. (2023) 24:2700. doi: 10.3390/ijms24032700
15. Shemesh CS, Hsu JC, Hosseini I, Shen BQ, Rotte A, Twomey P, et al. Personalized cancer vaccines: clinical landscape, challenges, and opportunities. Mol Ther. (2021) 29:555–70. doi: 10.1016/j.ymthe.2020.09.038
16. Buonaguro L, Petrizzo A, Tornesello ML, Buonaguro FM. Translating tumor antigens into cancer vaccines. Clin Vaccine Immunol. (2011) 18:23–34. doi: 10.1128/CVI.00286-10
17. Kaufman HL, Kohlhapp FJ, Zloza A. Oncolytic viruses: A new class of immunotherapy drugs. Nat Rev Drug Discovery. (2015) 14:642–62. doi: 10.1038/nrd4663
18. Ma R, Li Z, Chiocca EA, Caligiuri MA, Yu J. The emerging field of oncolytic virus-based cancer immunotherapy. Trends Cancer. (2023) 9:122–39. doi: 10.1016/j.trecan.2022.10.003
19. Oh CM, Chon HJ, Kim C. Combination immunotherapy using oncolytic virus for the treatment of advanced solid tumors. Int J Mol Sci. (2020) 21:7743. doi: 10.3390/ijms21207743
20. Liang M. Oncorine, the world first oncolytic virus medicine and its update in China. Curr Cancer Drug Targets. (2018) 18:171–6. doi: 10.2174/1568009618666171129221503
21. Zhang T, Jou TH, Hsin J, Wang Z, Huang K, Ye J, et al. Talimogene laherparepvec (T-vec): A review of the recent advances in cancer therapy. J Clin Med. (2023) 12:1098. doi: 10.3390/jcm12031098
22. Li X, Sun X, Wang B, Li Y, Tong J. Oncolytic virus-based hepatocellular carcinoma treatment: current status, intravenous delivery strategies, and emerging combination therapeutic solutions. Asian J Pharm Sci. (2023) 18:100771. doi: 10.1016/j.ajps.2022.100771
23. Lundstrom K. Viral vectors in gene therapy: where do we stand in 2023? Viruses. (2023) 15:698. doi: 10.3390/v15030698
24. Engeland CE, Ungerechts G. Measles virus as an oncolytic immunotherapy. Cancers (Basel). (2021) 13:544. doi: 10.3390/cancers13030544
25. Sato-Dahlman M, LaRocca CJ, Yanagiba C, Yamamoto M. Adenovirus and immunotherapy: advancing cancer treatment by combination. Cancers (Basel). (2020) 12:1295. doi: 10.3390/cancers12051295
26. Xu L, Sun H, Lemoine NR, Xuan Y, Wang P. Oncolytic vaccinia virus and cancer immunotherapy. Front Immunol. (2023) 14:1324744. doi: 10.3389/fimmu.2023.1324744
27. Zhang P, Zhang G, Wan X. Challenges and new technologies in adoptive cell therapy. J Hematol Oncol. (2023) 16:97. doi: 10.1186/s13045-023-01492-8
28. Monberg TJ, Borch TH, Svane IM, Donia M. Til therapy: facts and hopes. Clin Cancer Res. (2023) 29:3275–83. doi: 10.1158/1078-0432.Ccr-22-2428
29. Shafer P, Kelly LM, Hoyos V. Cancer therapy with TCR-engineered T cells: current strategies, challenges, and prospects. Front Immunol. (2022) 13:835762. doi: 10.3389/fimmu.2022.835762
30. Zhang X, Zhu L, Zhang H, Chen S, Xiao Y. CAR-T cell therapy in hematological Malignancies: current opportunities and challenges. Front Immunol. (2022) 13:927153. doi: 10.3389/fimmu.2022.927153
31. Propper DJ, Balkwill FR. Harnessing cytokines and chemokines for cancer therapy. Nat Rev Clin Oncol. (2022) 19:237–53. doi: 10.1038/s41571-021-00588-9
32. Temizoz B, Ishii KJ. Type I and II interferons toward ideal vaccine and immunotherapy. Expert Rev Vaccines. (2021) 20:527–44. doi: 10.1080/14760584.2021.1927724
33. Silk AW, Margolin K. Cytokine therapy. Hematol Oncol Clin North Am. (2019) 33:261–74. doi: 10.1016/j.hoc.2018.12.004
34. Briukhovetska D, Dörr J, Endres S, Libby P, Dinarello CA, Kobold S. Interleukins in cancer: from biology to therapy. Nat Rev Cancer. (2021) 21:481–99. doi: 10.1038/s41568-021-00363-z
35. Wu C, You M, Nguyen D, Wangpaichitr M, Li YY, Feun LG, et al. Enhancing the effect of tumor necrosis factor-related apoptosis-inducing ligand signaling and arginine deprivation in melanoma. Int J Mol Sci. (2021) 22:7628. doi: 10.3390/ijms22147628
36. Siegmund D, Wagner J, Wajant H. TNF receptor associated factor 2 (TRAF2) signaling in cancer. Cancers (Basel). (2022) 14:4055. doi: 10.3390/cancers14164055
37. Lah TT, Novak M, Breznik B. Brain Malignancies: glioblastoma and brain metastases. Semin Cancer Biol. (2020) 60:262–73. doi: 10.1016/j.semcancer.2019.10.010
38. Montoya ML, Kasahara N, Okada H. Introduction to immunotherapy for brain tumor patients: challenges and future perspectives. Neurooncol Pract. (2020) 7:465–76. doi: 10.1093/nop/npaa007
39. Ardizzone A, Basilotta R, Filippone A, Crupi L, Lanza M, Lombardo SP, et al. Recent emerging immunological treatments for primary brain tumors: focus on chemokine-targeting immunotherapies. Cells. (2023) 12:841. doi: 10.3390/cells12060841
40. Desbaillets N, Hottinger AF. Immunotherapy in glioblastoma: A clinical perspective. Cancers. (2021) 13:3721. doi: 10.3390/cancers13153721
41. Brozos-Vázquez EM, Rodríguez-López C, Cortegoso-Mosquera A, López-Landrove S, Muinelo-Romay L, García-González J, et al. Immunotherapy in patients with brain metastasis: advances and challenges for the treatment and the application of circulating biomarkers. Front Immunol. (2023) 14:1221113. doi: 10.3389/fimmu.2023.1221113
42. Mishchenko TA, Turubanova VD, Gorshkova EN, Krysko O, Vedunova MV, Krysko DV. Glioma: bridging the tumor microenvironment, patient immune profiles and novel personalized immunotherapy. Front Immunol. (2024) 14:1299064. doi: 10.3389/fimmu.2023.1299064
43. Khatami SH, Karami N, Taheri-Anganeh M, Taghvimi S, Tondro G, Khorsand M, et al. Exosomes: promising delivery tools for overcoming blood-brain barrier and glioblastoma therapy. Mol Neurobiol. (2023) 60:4659–78. doi: 10.1007/s12035-023-03365-0
44. Stamp MEM, Halwes M, Nisbet D, Collins DJ. Breaking barriers: exploring mechanisms behind opening the blood-brain barrier. Fluids Barriers CNS. (2023) 20:87. doi: 10.1186/s12987-023-00489-2
45. Zheng XX, Yang JY, Hou YW, Fang Y, Wu KY, Song YN, et al. Current non-invasive strategies for brain drug delivery: overcoming blood-brain barrier transport. Mol Biol Rep. (2024) 51:25. doi: 10.1007/s11033-023-08968-3
46. Lenz KM, Nelson LH. Microglia and beyond: innate immune cells as regulators of brain development and behavioral function. Front Immunol. (2018) 9:698. doi: 10.3389/fimmu.2018.00698
47. Goddery EN, Fain CE, Lipovsky CG, Ayasoufi K, Yokanovich LT, Malo CS, et al. Microglia and perivascular macrophages act as antigen presenting cells to promote CD8 T cell infiltration of the brain. Front Immunol. (2021) 12:726421. doi: 10.3389/fimmu.2021.726421
48. Ouyang P, Wang LJ, Wu JL, Tian Y, Chen CY, Li DS, et al. Overcoming cold tumors: A combination strategy of immune checkpoint inhibitors. Front Immunol. (2024) 15:1344272. doi: 10.3389/fimmu.2024.1344272
49. Czajka-Francuz P, Prendes MJ, Mankan A, Quintana A, Pabla S, Ramkissoon S, et al. Mechanisms of immune modulation in the tumor microenvironment and implications for targeted therapy. Front Oncol. (2023) 13:1200646. doi: 10.3389/fonc.2023.1200646
50. Liu WY, Zhou HY, Lai WJ, Hu CP, Xu RF, Gu P, et al. The immunosuppressive landscape in tumor microenvironment. Immunologic Res. (2024) 72:566–82. doi: 10.1007/s12026-024-09483-8
51. Gillette JS, Wang EJ, Dowd RS, Toms SA. Barriers to overcoming immunotherapy resistance in glioblastoma. Front Med. (2023) 10:1175507. doi: 10.3389/fmed.2023.1175507
52. Ottaiano A, Ianniello M, Santorsola M, Ruggiero R, Sirica R, Sabbatino F, et al. From chaos to opportunity: decoding cancer heterogeneity for enhanced treatment strategies. Biology-Basel. (2023) 12:1183. doi: 10.3390/biology12091183
53. Imodoye SO, Adedokun KA, Bello IO. From complexity to clarity: unravelling tumor heterogeneity through the lens of tumor microenvironment for innovative cancer therapy. Histochem Cell Biol. (2024) 161:299–323. doi: 10.1007/s00418-023-02258-6
54. Mangesius J, Mangesius S, Demetz M, Uprimny C, Di Santo G, Galijasevic M, et al. A multi-disciplinary approach to diagnosis and treatment of radionecrosis in Malignant gliomas and cerebral metastases. Cancers (Basel). (2022) 14:6264. doi: 10.3390/cancers14246264
55. Arvanitis CD, Ferraro GB, Jain RK. The blood-brain barrier and blood-tumour barrier in brain tumours and metastases. Nat Rev Cancer. (2020) 20:26–41. doi: 10.1038/s41568-019-0205-x
56. Sledzinska P, Bebyn MG, Furtak J, Kowalewski J, Lewandowska MA. Prognostic and predictive biomarkers in gliomas. Int J Mol Sci. (2021) 22:10373. doi: 10.3390/ijms221910373
57. Stupp R, Mason WP, van den Bent MJ, Weller M, Fisher B, Taphoorn MJB, et al. Radiotherapy plus concomitant and adjuvant temozolomide for glioblastoma. New Engl J Med. (2005) 352:987–96. doi: 10.1056/NEJMoa043330
58. Latifyan S, de Micheli R, Hottinger AF. Physical approaches to treat glioblastoma. Curr Opin Oncol. (2020) 32:640–9. doi: 10.1097/cco.0000000000000689
59. Yasinjan F, Xing Y, Geng HY, Guo R, Yang L, Liu ZL, et al. Immunotherapy: A promising approach for glioma treatment. Front Immunol. (2023) 14:1255611. doi: 10.3389/fimmu.2023.1255611
60. Grady C, Melnick K, Porche K, Dastmalchi F, Hoh DJ, Rahman M, et al. Glioma immunotherapy: advances and challenges for spinal cord gliomas. Neurospine. (2022) 19:13–29. doi: 10.14245/ns.2143210.605
61. Rong L, Li N, Zhang ZZ. Emerging therapies for glioblastoma: current state and future directions. J Exp Clin Cancer Res. (2022) 41:142. doi: 10.1186/s13046-022-02349-7
62. Regmi M, Wang YJ, Liu WH, Dai YW, Liu SK, Ma K, et al. From glioma gloom to immune bloom: unveiling novel immunotherapeutic paradigms-a review. J Exp Clin Cancer Res. (2024) 43:47. doi: 10.1186/s13046-024-02973-5
63. Chen JJ, Li SN, Yao QG, Du NN, Fu XJ, Lou YM, et al. The efficacy and safety of combined immune checkpoint inhibitors (Nivolumab plus ipilimumab): A systematic review and meta-analysis. World J Surg Oncol. (2020) 18:150. doi: 10.1186/s12957-020-01933-5
64. Choi H, Chang JE. Targeted therapy for cancers: from ongoing clinical trials to FDA-approved drugs. Int J Mol Sci. (2023) 24:13618. doi: 10.3390/ijms241713618
65. Ortega MA, Pekarek L, Navarro F, Fraile-Martínez O, García-Montero C, Alvarez-Mon MA, et al. Updated views in targeted therapy in the patient with non-small cell lung cancer. J Personalized Med. (2023) 13:167. doi: 10.3390/jpm13020167
66. Kang Y, Li HT, Liu YP, Li Z. Regulation of VEGF-a expression and VEGF-a-targeted therapy in Malignant tumors. J Cancer Res Clin Oncol. (2024) 150:221. doi: 10.1007/s00432-024-05714-5
67. Li B, Jin J, Guo DC, Tao ZH, Hu XC. Immune checkpoint inhibitors combined with targeted therapy: the recent advances and future potentials. Cancers. (2023) 15:2858. doi: 10.3390/cancers15102858
68. Liu J, Fu MY, Wang MN, Wan DD, Wei YQ, Wei XW. Cancer vaccines as promising immuno-therapeutics: platforms and current progress. J Hematol Oncol. (2022) 15:28. doi: 10.1186/s13045-022-01247-x
69. Hotchkiss KM, Batich KA, Mohan A, Rahman R, Piantadosi S, Khasraw M. Dendritic cell vaccine trials in gliomas: untangling the lines. Neuro-Oncology. (2023) 25:1752–62. doi: 10.1093/neuonc/noad088
70. Zhou J, Li LH, Jia MQ, Liao QJ, Peng GP, Luo GQ, et al. Dendritic cell vaccines improve the glioma microenvironment: influence, challenges, and future directions. Cancer Med. (2023) 12:7207–21. doi: 10.1002/cam4.5511
71. Sahm K, Weiss T. Immunotherapy against gliomas. Nervenarzt. (2024) 95:111–6. doi: 10.1007/s00115-023-01590-5
72. Weller M, Butowski N, Tran DD, Recht LD, Lim M, Hirte H, et al. Rindopepimut with temozolomide for patients with newly diagnosed, EGFRvIII-expressing glioblastoma (ACT IV): A randomised, double-blind, international phase 3 trial. Lancet Oncol. (2017) 18:1373–85. doi: 10.1016/s1470-2045(17)30517-x
73. Zheng Y, Ma XY, Feng SC, Zhu HT, Chen X, Yu XJ, et al. Dendritic cell vaccine of gliomas: challenges from bench to bed. Front Immunol. (2023) 14:1259562. doi: 10.3389/fimmu.2023.1259562
74. Mendez-Gomez HR, DeVries A, Castillo P, von Roemeling C, Qdaisat S, Stover BD, et al. RNA aggregates harness the danger response for potent cancer immunotherapy. Cell. (2024) 187:2521–35.e21. doi: 10.1016/j.cell.2024.04.003
75. Victorio CBL, Novera W, Ganasarajah A, Ong J, Thomas M, Wu J, et al. Repurposing of Zika virus live-attenuated vaccine (ZIKV-LAV) strains as oncolytic viruses targeting human glioblastoma multiforme cells. J Trans Med. (2024) 22:126. doi: 10.1186/s12967-024-04930-4
76. Zeng J, Li X, Sander M, Zhang H, Yan G, Lin Y. Oncolytic viro-immunotherapy: an emerging option in the treatment of gliomas. Front Immunol. (2021) 12:721830. doi: 10.3389/fimmu.2021.721830
77. Asija S, Chatterjee A, Goda JSS, Yadav S, Chekuri G, Purwar R. Oncolytic immunovirotherapy for high-grade gliomas: A novel and an evolving therapeutic option. Front Immunol. (2023) 14:1118246. doi: 10.3389/fimmu.2023.1118246
78. Hamad A, Yusubalieva GM, Baklaushev VP, Chumakov PM, Lipatova AV. Recent developments in glioblastoma therapy: oncolytic viruses and emerging future strategies. Viruses-Basel. (2023) 15:547. doi: 10.3390/v15020547
79. Hua L, Wakimoto H. Oncolytic herpes simplex virus therapy for Malignant glioma: current approaches to successful clinical application. Expert Opin Biol Ther. (2019) 19:845–54. doi: 10.1080/14712598.2019.1614557
80. Lang FF, Conrad C, Gomez-Manzano C, Yung WKA, Sawaya R, Weinberg JS, et al. Phase I study of DNX-2401 (Delta-24-RGD) oncolytic adenovirus: replication and immunotherapeutic effects in recurrent Malignant glioma. J Clin Oncol. (2018) 36:1419. doi: 10.1200/jco.2017.75.8219
81. Philbrick B, Adamson DC. Dnx-2401: an investigational drug for the treatment of recurrent glioblastoma. Expert Opin Investigational Drugs. (2019) 28:1041–9. doi: 10.1080/13543784.2019.1694000
82. Philbrick BD, Adamson DC. Early clinical trials of Toca 511 and Toca FC show a promising novel treatment for recurrent Malignant glioma. Expert Opin Investigational Drugs. (2019) 28:207–16. doi: 10.1080/13543784.2019.1572112
83. Friedman GK, Johnston JM, Bag AK, Bernstock JD, Li R, Aban I, et al. Oncolytic HSV-1 G207 immunovirotherapy for pediatric high-grade gliomas. New Engl J Med. (2021) 384:1613–22. doi: 10.1056/NEJMoa2024947
84. Li J, Meng Q, Zhou X, Zhao H, Wang K, Niu H, et al. Gospel of Malignant glioma: oncolytic virus therapy. Gene. (2022) 818:146217. doi: 10.1016/j.gene.2022.146217
85. Ling AL, Solomon IH, Landivar AM, Nakashima H, Woods JK, Santos A, et al. Clinical trial links oncolytic immunoactivation to survival in glioblastoma. Nature. (2023) 623:157. doi: 10.1038/s41586-023-06623-2
86. Wang J, Shen F, Yao Y, Wang LL, Zhu Y, Hu J. Adoptive cell therapy: A novel and potential immunotherapy for glioblastoma. Front Oncol. (2020) 10:59. doi: 10.3389/fonc.2020.00059
87. Pan C, Zhai Y, Li G, Jiang T, Zhang W. NK cell-based immunotherapy and therapeutic perspective in gliomas. Front Oncol. (2021) 11:751183. doi: 10.3389/fonc.2021.751183
88. Brown CE, Rodriguez A, Palmer J, Ostberg JR, Naranjo A, Wagner JR, et al. Off-the-shelf, steroid-resistant, IL13Rα2-specific car T cells for treatment of glioblastoma. Neuro Oncol. (2022) 24:1318–30. doi: 10.1093/neuonc/noac024
89. Xiong Q, Zhu J, Zhang Y, Deng H. CAR-NK cell therapy for glioblastoma: what to do next? Front Oncol. (2023) 13:1192128. doi: 10.3389/fonc.2023.1192128
90. Brown CE, Hibbard JC, Alizadeh D, Blanchard MS, Natri HM, Wang D, et al. Locoregional delivery of IL-13Rα2-targeting CAR-T cells in recurrent high-grade glioma: A phase 1 trial. Nat Med. (2024) 30:1001–12. doi: 10.1038/s41591-024-02875-1
91. Chan HY, Choi J, Jackson C, Lim M. Combination immunotherapy strategies for glioblastoma. J Neurooncol. (2021) 151:375–91. doi: 10.1007/s11060-020-03481-0
92. Krämer C, Kilian M, Chih YC, Kourtesakis A, Hoffmann DC, Boschert T, et al. NLGN4X TCR transgenic T cells to treat gliomas. Neuro Oncol. (2024) 26:266–78. doi: 10.1093/neuonc/noad172
93. Morimoto T, Nakazawa T, Maeoka R, Nakagawa I, Tsujimura T, Matsuda R. Natural killer cell-based immunotherapy against glioblastoma. Int J Mol Sci. (2023) 24:2111. doi: 10.3390/ijms24032111
94. Burger MC, Zhang C, Harter PN, Romanski A, Strassheimer F, Senft C, et al. CAR-engineered NK cells for the treatment of glioblastoma: turning innate effectors into precision tools for cancer immunotherapy. Front Immunol. (2019) 10:2683. doi: 10.3389/fimmu.2019.02683
95. Rybchenko VS, Aliev TK, Panina AA, Kirpichnikov MP, Dolgikh DA. Targeted cytokine delivery for cancer treatment: engineering and biological effects. Pharmaceutics. (2023) 15:336. doi: 10.3390/pharmaceutics15020336
96. Lichtor T, Tang B, Roy EJ. Cytokine gene vaccine therapy for treatment of a brain tumor. Brain Sci. (2023) 13:1505. doi: 10.3390/brainsci13111505
97. Aghajani M, Jalilzadeh N, Aghebati-Maleki A, Yari A, Tabnak P, Mardi A, et al. Current approaches in glioblastoma multiforme immunotherapy. Clin Transl Oncol. (2024) 26:1584–612. doi: 10.1007/s12094-024-03395-7
98. Enrique G-V, Irving S-R, Ricardo B-I, Jesús F-L, Alan R-M, Iñigo VAA, et al. Diagnosis and management of brain metastases: an updated review from a radiation oncology perspective. J Cancer Metastasis Treat. (2019) 5:54. doi: 10.20517/2394-4722.2019.20
99. Derks S, van der Veldt AAM, Smits M. Brain metastases: the role of clinical imaging. Br J Radiol. (2022) 95:20210944. doi: 10.1259/bjr.20210944
100. Ahmad A, Khan P, Rehman AU, Batra SK, Nasser MW. Immunotherapy: an emerging modality to checkmate brain metastasis. Mol Cancer. (2023) 22:111. doi: 10.1186/s12943-023-01818-7
101. Aquilanti E, Brastianos PK. Immune checkpoint inhibitors for brain metastases: A primer for neurosurgeons. Neurosurgery. (2020) 87:E281–E8. doi: 10.1093/neuros/nyaa095
102. Margolin K, Ernstoff MS, Hamid O, Lawrence D, McDermott D, Puzanov I, et al. Ipilimumab in patients with melanoma and brain metastases: an open-label, phase 2 trial. Lancet Oncol. (2012) 13:459–65. doi: 10.1016/s1470-2045(12)70090-6
103. Rulli E, Legramandi L, Salvati L, Mandala M. The impact of targeted therapies and immunotherapy in melanoma brain metastases: A systematic review and meta-analysis. Cancer. (2019) 125:3776–89. doi: 10.1002/cncr.32375
104. Tawbi HA, Forsyth PA, Hodi FS, Algazi AP, Hamid O, Lao CD, et al. Long-term outcomes of patients with active melanoma brain metastases treated with combination nivolumab plus ipilimumab (Checkmate 204): final results of an open-label, multicentre, phase 2 study. Lancet Oncol. (2021) 22:1692–704. doi: 10.1016/s1470-2045(21)00545-3
105. Isla D, Sánchez A, Casal J, Cobo M, Majem M, Reguart N, et al. PD-1/PD-L1 inhibitors as monotherapy in the first-line treatment of advanced non-small cell lung cancer patients with high PD-L1 expression: an expert position statement. J Clin Med. (2023) 12:5063. doi: 10.3390/jcm12155063
106. Khasraw M, Yalamanchili P, Santhanagopal A, Wu CT, Salas M, Meng J, et al. Clinical management of patients with non-small cell lung cancer, brain metastases, and actionable genomic alterations: A systematic literature review. Adv Ther. (2024) 41:1815–42. doi: 10.1007/s12325-024-02799-9
107. Schlam I, Gatti-Mays ME. Immune checkpoint inhibitors in the treatment of breast cancer brain metastases. Oncologist. (2022) 27:538–47. doi: 10.1093/oncolo/oyac064
108. Shindorf ML, Jafferji MS, Goff SL. Incidence of asymptomatic brain metastases in metastatic colorectal cancer. Clin Colorectal Cancer. (2020) 19:263–9. doi: 10.1016/j.clcc.2020.09.002
109. Hasanov E, Yeboa DN, Tucker MD, Swanson TA, Beckham TH, Rini B, et al. An interdisciplinary consensus on the management of brain metastases in patients with renal cell carcinoma. Ca-a Cancer J Clin. (2022) 72:454–89. doi: 10.3322/caac.21729
110. Emamekhoo H, Olsen MR, Carthon BC, Drakaki A, Percent IJ, Molina AM, et al. Safety and efficacy of nivolumab plus ipilimumab in patients with advanced renal cell carcinoma with brain metastases: checkmate 920. Cancer. (2022) 128:966–74. doi: 10.1002/cncr.34016
111. Yu JF, Sun H, Cao WJ, Song YP, Jiang ZX. Research progress on dendritic cell vaccines in cancer immunotherapy. Exp Hematol Oncol. (2022) 11:3. doi: 10.1186/s40164-022-00257-2
112. Santisteban M, Solans BP, Hato L, Urrizola A, Mejías LD, Salgado E, et al. Final results regarding the addition of dendritic cell vaccines to neoadjuvant chemotherapy in early HER2-negative breast cancer patients: clinical and translational analysis. Ther Adv Med Oncol. (2021) 13:17588359211064653. doi: 10.1177/17588359211064653
113. Barth RJ, Fisher DA, Wallace PK, Channon JY, Noelle RJ, Gui JA, et al. A randomized trial of ex vivo CD40L activation of a dendritic cell vaccine in colorectal cancer patients: tumor-specific immune responses are associated with improved survival. Clin Cancer Res. (2010) 16:5548–56. doi: 10.1158/1078-0432.Ccr-10-2138
114. Nieblas-Bedolla E, Nayyar N, Singh M, Sullivan RJ, Brastianos PK. Emerging immunotherapies in the treatment of brain metastases. Oncologist. (2021) 26:231–41. doi: 10.1002/onco.13575
115. Soldozy S, Mulligan KM, Zheng DX, Levoska MA, Cullison CR, Elarjani T, et al. Oncolytic virotherapy for melanoma brain metastases, a potential new treatment paradigm? Brain Sci. (2021) 11:1260. doi: 10.3390/brainsci11101260
116. Andtbacka RHI, Kaufman HL, Collichio F, Amatruda T, Senzer N, Chesney J, et al. Talimogene laherparepvec improves durable response rate in patients with advanced melanoma. J Clin Oncol. (2015) 33:2780–U98. doi: 10.1200/jco.2014.58.3377
117. Puzanov I, Milhem MM, Minor D, Hamid O, Li A, Chen LS, et al. Talimogene laherparepvec in combination with ipilimumab in previously untreated, unresectable stage IIIB-IV melanoma. J Clin Oncol. (2016) 34:2619–U109. doi: 10.1200/jco.2016.67.1529
118. Mirbahari SN, Da Silva M, Zúñiga AIM, Zamani NK, St-Laurent G, Totonchi M, et al. Recent progress in combination therapy of oncolytic vaccinia virus. Front Immunol. (2024) 15:1272351. doi: 10.3389/fimmu.2024.1272351
119. Mehta GU, Malekzadeh P, Shelton T, White DE, Butman JA, Yang JC, et al. Outcomes of adoptive cell transfer with tumor-infiltrating lymphocytes for metastatic melanoma patients with and without brain metastases. J Immunotherapy. (2018) 41:241–7. doi: 10.1097/cji.0000000000000223
120. Sammons S, Van Swearingen AED, Chung C, Anders CK. Advances in the management of breast cancer brain metastases. Neurooncol Adv. (2021) 3:v63–74. doi: 10.1093/noajnl/vdab119
121. Luo L, Liu P, Zhao K, Zhao W, Zhang X. The immune microenvironment in brain metastases of non-small cell lung cancer. Front Oncol. (2021) 11:698844. doi: 10.3389/fonc.2021.698844
122. Li S, Wu X, Chen P, Pei Y, Zheng K, Wang W, et al. Interferon-Α Versus interleukin-2 in Chinese patients with Malignant melanoma: A randomized, controlled, trial. Anticancer Drugs. (2019) 30:402–9. doi: 10.1097/CAD.0000000000000741
123. Schaff LR, Grommes C. Primary central nervous system lymphoma. Blood. (2022) 140:971–9. doi: 10.1182/blood.2020008377
124. Ferreri AJM, Calimeri T, Cwynarski K, Dietrich J, Grommes C, Hoang-Xuan K, et al. Primary central nervous system lymphoma. Nat Rev Dis Primers. (2023) 9:29. doi: 10.1038/s41572-023-00439-0
125. Alcantara M, Fuentealba J, Soussain C. Emerging landscape of immunotherapy for primary central nervous system lymphoma. Cancers (Basel). (2021) 13:5061. doi: 10.3390/cancers13205061
126. Karschnia P, Blobner J, Teske N, Schöberl F, Fitzinger E, Dreyling M, et al. Car T-cells for CNS lymphoma: driving into new terrain? Cancers (Basel). (2021) 13:2503. doi: 10.3390/cancers13102503
127. Kaley T, Barani I, Chamberlain M, McDermott M, Panageas K, Raizer J, et al. Historical benchmarks for medical therapy trials in surgery- and radiation-refractory meningioma: A rano review. Neuro Oncol. (2014) 16:829–40. doi: 10.1093/neuonc/not330
128. Bi WL, Greenwald NF, Abedalthagafi M, Wala J, Gibson WJ, Agarwalla PK, et al. Genomic landscape of high-grade meningiomas. NPJ Genom Med. (2017) 2:15. doi: 10.1038/s41525-017-0014-7
129. Li YD, Veliceasa D, Lamano JB, Lamano JB, Kaur G, Biyashev D, et al. Systemic and local immunosuppression in patients with high-grade meningiomas. Cancer Immunol Immunother. (2019) 68:999–1009. doi: 10.1007/s00262-019-02342-8
130. Miller AB, Hoogstraten B, Staquet M, Winkler A. Reporting results of cancer treatment. Cancer. (1981) 47:207–14. doi: 10.1002/1097-0142(19810101)47:1<207::aid-cncr2820470134>3.0.co;2-6
131. Therasse P, Arbuck SG, Eisenhauer EA, Wanders J, Kaplan RS, Rubinstein L, et al. New guidelines to evaluate the response to treatment in solid tumors. European organization for research and treatment of cancer, national cancer institute of the United States, national cancer institute of Canada. J Natl Cancer Inst. (2000) 92:205–16. doi: 10.1093/jnci/92.3.205
132. Eisenhauer EA, Therasse P, Bogaerts J, Schwartz LH, Sargent D, Ford R, et al. New response evaluation criteria in solid tumours: revised recist guideline (Version 1.1). Eur J Cancer. (2009) 45:228–47. doi: 10.1016/j.ejca.2008.10.026
133. Wolchok JD, Hoos A, O'Day S, Weber JS, Hamid O, Lebbé C, et al. Guidelines for the evaluation of immune therapy activity in solid tumors: immune-related response criteria. Clin Cancer Res. (2009) 15:7412–20. doi: 10.1158/1078-0432.Ccr-09-1624
134. Nishino M, Giobbie-Hurder A, Gargano M, Suda M, Ramaiya NH, Hodi FS. Developing a common language for tumor response to immunotherapy: immune-related response criteria using unidimensional measurements. Clin Cancer Res. (2013) 19:3936–43. doi: 10.1158/1078-0432.CCR-13-0895
135. Seymour L, Bogaerts J, Perrone A, Ford R, Schwartz LH, Mandrekar S, et al. Irecist: guidelines for response criteria for use in trials testing immunotherapeutics. Lancet Oncol. (2017) 18:e143–e52. doi: 10.1016/S1470-2045(17)30074-8
136. Wen PY, Macdonald DR, Reardon DA, Cloughesy TF, Sorensen AG, Galanis E, et al. Updated response assessment criteria for high-grade gliomas: response assessment in neuro-oncology working group. J Clin Oncol. (2010) 28:1963–72. doi: 10.1200/jco.2009.26.3541
137. van den Bent MJ, Wefel JS, Schiff D, Taphoorn MJ, Jaeckle K, Junck L, et al. Response assessment in neuro-oncology (a report of the rano group): assessment of outcome in trials of diffuse low-grade gliomas. Lancet Oncol. (2011) 12:583–93. doi: 10.1016/s1470-2045(11)70057-2
138. Okada H, Weller M, Huang R, Finocchiaro G, Gilbert MR, Wick W, et al. Immunotherapy response assessment in neuro-oncology: A report of the RANO working group. Lancet Oncol. (2015) 16:e534–e42. doi: 10.1016/S1470-2045(15)00088-1
139. Lin NU, Lee EQ, Aoyama H, Barani IJ, Barboriak DP, Baumert BG, et al. Response assessment criteria for brain metastases: proposal from the RANO group. Lancet Oncol. (2015) 16:e270–8. doi: 10.1016/s1470-2045(15)70057-4
140. Ellingson BM, Wen PY, Cloughesy TF. Modified criteria for radiographic response assessment in glioblastoma clinical trials. Neurotherapeutics. (2017) 14:307–20. doi: 10.1007/s13311-016-0507-6
141. Wen PY, van den Bent M, Youssef G, Cloughesy TF, Ellingson BM, Weller M, et al. RANO 2.0: update to the response assessment in neuro-oncology criteria for high- and low-grade gliomas in adults. J Clin Oncol. (2023) 41:5187–99. doi: 10.1200/JCO.23.01059
142. Chen X, Lim-Fat MJ, Qin L, Li A, Bryant A, Bay CP, et al. A comparative retrospective study of immunotherapy RANO versus standard RANO criteria in glioblastoma patients receiving immune checkpoint inhibitor therapy. Front Oncol. (2021) 11:679331. doi: 10.3389/fonc.2021.679331
143. Youssef G, Rahman R, Bay C, Wang W, Lim-Fat MJ, Arnaout O, et al. Evaluation of standard response assessment in neuro-oncology, modified response assessment in neuro-oncology, and immunotherapy response assessment in neuro-oncology in newly diagnosed and recurrent glioblastoma. J Clin Oncol. (2023) 41:3160–71. doi: 10.1200/jco.22.01579
144. Douri K, Iorio-Morin C, Mercure-Cyr R, Figueiredo G, Touchette CJ, Masson-Côté L, et al. Response assessment in brain metastases managed by stereotactic radiosurgery: A reappraisal of the RANO-BM criteria. Curr Oncol. (2023) 30:9382–91. doi: 10.3390/curroncol30110679
145. Frelaut M, du Rusquec P, de Moura A, Le Tourneau C, Borcoman E. Pseudoprogression and hyperprogression as new forms of response to immunotherapy. BioDrugs. (2020) 34:463–76. doi: 10.1007/s40259-020-00425-y
146. Ahmed FS, Dercle L, Goldmacher GV, Yang H, Connors D, Tang Y, et al. Comparing recist 1.1 and irecist in advanced melanoma patients treated with pembrolizumab in a phase II clinical trial. Eur Radiol. (2021) 31:1853–62. doi: 10.1007/s00330-020-07249-y
147. Lee D, Riestenberg RA, Haskell-Mendoza A, Bloch O. Brain metastasis recurrence versus radiation necrosis: evaluation and treatment. Neurosurg Clin N Am. (2020) 31:575–87. doi: 10.1016/j.nec.2020.06.007
148. Nishino M, Hatabu H, Hodi FS. Imaging of cancer immunotherapy: current approaches and future directions. Radiology. (2019) 290:9–22. doi: 10.1148/radiol.2018181349
149. Dercle L, Sun S, Seban RD, Mekki A, Sun R, Tselikas L, et al. Emerging and evolving concepts in cancer immunotherapy imaging. Radiology. (2023) 306:32–46. doi: 10.1148/radiol.210518
150. Champiat S, Dercle L, Ammari S, Massard C, Hollebecque A, Postel-Vinay S, et al. Hyperprogressive disease is a new pattern of progression in cancer patients treated by anti-PD-1/PD-L1. Clin Cancer Res. (2017) 23:1920–8. doi: 10.1158/1078-0432.Ccr-16-1741
151. Saâda-Bouzid E, Defaucheux C, Karabajakian A, Coloma VP, Servois V, Paoletti X, et al. Hyperprogression during anti-PD-1/PD-L1 therapy in patients with recurrent and/or metastatic head and neck squamous cell carcinoma. Ann Oncol. (2017) 28:1605–11. doi: 10.1093/annonc/mdx178
152. Mole RH. Whole body irradiation; radiobiology or medicine? Br J Radiol. (1953) 26:234–41. doi: 10.1259/0007-1285-26-305-234
153. Tubin S, Yan W, Mourad WF, Fossati P, Khan MK. The future of radiation-induced abscopal response: beyond conventional radiotherapy approaches. Future Oncol. (2020) 16:1137–51. doi: 10.2217/fon-2020-0063
154. Hygino da Cruz LC Jr., Rodriguez I, Domingues RC, Gasparetto EL, Sorensen AG. Pseudoprogression and pseudoresponse: imaging challenges in the assessment of posttreatment glioma. AJNR Am J Neuroradiol. (2011) 32:1978–85. doi: 10.3174/ajnr.A2397
155. Wang S, Martinez-Lage M, Sakai Y, Chawla S, Kim SG, Alonso-Basanta M, et al. Differentiating tumor progression from pseudoprogression in patients with glioblastomas using diffusion tensor imaging and dynamic susceptibility contrast MRI. AJNR Am J Neuroradiol. (2016) 37:28–36. doi: 10.3174/ajnr.A4474
156. Roques M, Catalaa I, Raveneau M, Attal J, Siegfried A, Darcourt J, et al. Assessment of the hypervascularized fraction of glioblastomas using a volume analysis of dynamic susceptibility contrast-enhanced MRI may help to identify pseudoprogression. PloS One. (2022) 17:e0270216. doi: 10.1371/journal.pone.0270216
157. Anil A, Stokes AM, Chao R, Hu LS, Alhilali L, Karis JP, et al. Identification of single-dose, dual-echo based CBV threshold for fractional tumor burden mapping in recurrent glioblastoma. Front Oncol. (2023) 13:1046629. doi: 10.3389/fonc.2023.1046629
158. Nierobisch N, Ludovichetti R, Kadali K, Fierstra J, Hüllner M, Michels L, et al. Comparison of clinically available dynamic susceptibility contrast post processing software to differentiate progression from pseudoprogression in post-treatment high grade glioma. Eur J Radiol. (2023) 167:111076. doi: 10.1016/j.ejrad.2023.111076
159. Barajas RF, Chang JS, Sneed PK, Segal MR, McDermott MW, Cha S. Distinguishing recurrent intra-axial metastatic tumor from radiation necrosis following gamma knife radiosurgery using dynamic susceptibility-weighted contrast-enhanced perfusion MR imaging. AJNR Am J Neuroradiol. (2009) 30:367–72. doi: 10.3174/ajnr.A1362
160. Mitsuya K, Nakasu Y, Horiguchi S, Harada H, Nishimura T, Bando E, et al. Perfusion weighted magnetic resonance imaging to distinguish the recurrence of metastatic brain tumors from radiation necrosis after stereotactic radiosurgery. J Neurooncol. (2010) 99:81–8. doi: 10.1007/s11060-009-0106-z
161. Huang J, Wang AM, Shetty A, Maitz AH, Yan D, Doyle D, et al. Differentiation between intra-axial metastatic tumor progression and radiation injury following fractionated radiation therapy or stereotactic radiosurgery using MR spectroscopy, perfusion MR imaging or volume progression modeling. Magn Reson Imaging. (2011) 29:993–1001. doi: 10.1016/j.mri.2011.04.004
162. Wang B, Zhao B, Zhang Y, Ge M, Zhao P, Na S, et al. Absolute CBV for the differentiation of recurrence and radionecrosis of brain metastases after gamma knife radiotherapy: A comparison with relative CBV. Clin Radiol. (2018) 73:758.e1–e7. doi: 10.1016/j.crad.2018.04.006
163. Morabito R, Alafaci C, Pergolizzi S, Pontoriero A, Iati G, Bonanno L, et al. DCE and DSC perfusion MRI diagnostic accuracy in the follow-up of primary and metastatic intra-axial brain tumors treated by radiosurgery with cyberknife. Radiat Oncol. (2019) 14:65. doi: 10.1186/s13014-019-1271-7
164. Umemura Y, Wang D, Peck KK, Flynn J, Zhang Z, Fatovic R, et al. DCE-MRI perfusion predicts pseudoprogression in metastatic melanoma treated with immunotherapy. J Neurooncol. (2020) 146:339–46. doi: 10.1007/s11060-019-03379-6
165. Chawla S, Shehu V, Gupta PK, Nath K, Poptani H. Physiological imaging methods for evaluating response to immunotherapies in glioblastomas. Int J Mol Sci. (2021) 22:3867. doi: 10.3390/ijms22083867
166. Lee J, Chen MM, Liu HL, Ucisik FE, Wintermark M, Kumar VA. Mr perfusion imaging for gliomas. Magn Reson Imaging Clin N Am. (2024) 32:73–83. doi: 10.1016/j.mric.2023.07.003
167. Cuccarini V, Aquino D, Gioppo A, Anghileri E, Pellegatta S, Schettino C, et al. Advanced MRI assessment during dendritic cell immunotherapy added to standard treatment against glioblastoma. J Clin Med. (2019) 8:2007. doi: 10.3390/jcm8112007
168. Vrabec M, Van Cauter S, Himmelreich U, Van Gool SW, Sunaert S, De Vleeschouwer S, et al. MR perfusion and diffusion imaging in the follow-up of recurrent glioblastoma treated with dendritic cell immunotherapy: A pilot study. Neuroradiology. (2011) 53:721–31. doi: 10.1007/s00234-010-0802-6
169. Stenberg L, Englund E, Wirestam R, Siesjö P, Salford LG, Larsson EM. Dynamic susceptibility contrast-enhanced perfusion magnetic resonance (MR) imaging combined with contrast-enhanced MR imaging in the follow-up of immunogene-treated glioblastoma multiforme. Acta Radiol. (2006) 47:852–61. doi: 10.1080/02841850600815341
170. Song J, Kadaba P, Kravitz A, Hormigo A, Friedman J, Belani P, et al. Multiparametric mri for early identification of therapeutic response in recurrent glioblastoma treated with immune checkpoint inhibitors. Neuro Oncol. (2020) 22:1658–66. doi: 10.1093/neuonc/noaa066
171. Ortiz de Mendivil A, Martín-Medina P, García-Cañamaque L, Jiménez-Munarriz B, Ciérvide R, Diamantopoulos J. Challenges in radiological evaluation of brain metastases, beyond progression. Radiologia (Engl Ed). (2024) 66:166–80. doi: 10.1016/j.rxeng.2024.03.003
172. Li Y, Ma Y, Wu Z, Xie R, Zeng F, Cai H, et al. Advanced imaging techniques for differentiating pseudoprogression and tumor recurrence after immunotherapy for glioblastoma. Front Immunol. (2021) 12:790674. doi: 10.3389/fimmu.2021.790674
173. Floeth FW, Wittsack HJ, Engelbrecht V, Weber F. Comparative follow-up of enhancement phenomena with MRI and proton MR spectroscopic imaging after intralesional immunotherapy in glioblastoma–report of two exceptional cases. Zentralbl Neurochir. (2002) 63:23–8. doi: 10.1055/s-2002-31579
174. Mayo ZS, Billena C, Suh JH, Lo SS, Chao ST. The dilemma of radiation necrosis from diagnosis to treatment in the management of brain metastases. Neuro Oncol. (2024) 26:S56–65. doi: 10.1093/neuonc/noad188
175. Ma B, Blakeley JO, Hong X, Zhang H, Jiang S, Blair L, et al. Applying amide proton transfer-weighted MRI to distinguish pseudoprogression from true progression in Malignant gliomas. J Magn Reson Imaging. (2016) 44:456–62. doi: 10.1002/jmri.25159
176. Nguyen NC, Yee MK, Tuchayi AM, Kirkwood JM, Tawbi H, Mountz JM. Targeted therapy and immunotherapy response assessment with F-18 fluorothymidine positron-emission tomography/magnetic resonance imaging in melanoma brain metastasis: A pilot study. Front Oncol. (2018) 8:18. doi: 10.3389/fonc.2018.00018
177. Kristin Schmitz A, Sorg RV, Stoffels G, Grauer OM, Galldiks N, Steiger HJ, et al. Diagnostic impact of additional O-(2-[18F]Fluoroethyl)-L-tyrosine ((18)F-FET) PET following immunotherapy with dendritic cell vaccination in glioblastoma patients. Br J Neurosurg. (2021) 35:736–42. doi: 10.1080/02688697.2019.1639615
178. Antonios JP, Soto H, Everson RG, Moughon DL, Wang AC, Orpilla J, et al. Detection of immune responses after immunotherapy in glioblastoma using PET and MRI. Proc Natl Acad Sci U.S.A. (2017) 114:10220–5. doi: 10.1073/pnas.1706689114
179. Galldiks N, Abdulla DSY, Scheffler M, Wolpert F, Werner JM, Hüllner M, et al. Treatment monitoring of immunotherapy and targeted therapy using (18)F-FET PET in patients with melanoma and lung cancer brain metastases: initial experiences. J Nucl Med. (2021) 62:464–70. doi: 10.2967/jnumed.120.248278
180. Dercle L, Sun S, Seban RD, Mekki A, Sun R, Tselikas L, et al. Emerging and evolving concepts in cancer immunotherapy imaging. Radiology. (2023) 306:e239003. doi: 10.1148/radiol.239003
181. Galldiks N, Kocher M, Ceccon G, Werner JM, Brunn A, Deckert M, et al. Imaging challenges of immunotherapy and targeted therapy in patients with brain metastases: response, progression, and pseudoprogression. Neuro Oncol. (2020) 22:17–30. doi: 10.1093/neuonc/noz147
182. Li Y, Wang P, Xu J, Shi X, Yin T, Teng F. Noninvasive radiomic biomarkers for predicting pseudoprogression and hyperprogression in patients with non-small cell lung cancer treated with immune checkpoint inhibition. Oncoimmunology. (2024) 13:2312628. doi: 10.1080/2162402X.2024.2312628
183. Chen D, Zhang R, Huang X, Ji C, Xia W, Qi Y, et al. MRI-derived radiomics assessing tumor-infiltrating macrophages enable prediction of immune-phenotype, immunotherapy response and survival in glioma. biomark Res. (2024) 12:14. doi: 10.1186/s40364-024-00560-6
184. Fu FX, Cai QL, Li G, Wu XJ, Hong L, Chen WS. The efficacy of using a multiparametric magnetic resonance imaging-based radiomics model to distinguish glioma recurrence from pseudoprogression. Magn Reson Imaging. (2024) 111:168–78. doi: 10.1016/j.mri.2024.05.003
185. Xu J, Wang P, Li Y, Shi X, Yin T, Yu J, et al. Development and validation of an MRI-based nomogram to predict the effectiveness of immunotherapy for brain metastasis in patients with non-small cell lung cancer. Front Immunol. (2024) 15:1373330. doi: 10.3389/fimmu.2024.1373330
186. McCague C, Ramlee S, Reinius M, Selby I, Hulse D, Piyatissa P, et al. Introduction to radiomics for a clinical audience. Clin Radiol. (2023) 78:83–98. doi: 10.1016/j.crad.2022.08.149
187. LeCun Y, Bengio Y, Hinton G. Deep learning. Nature. (2015) 521:436–44. doi: 10.1038/nature14539
188. Zhu M, Li S, Kuang Y, Hill VB, Heimberger AB, Zhai L, et al. Artificial intelligence in the radiomic analysis of glioblastomas: A review, taxonomy, and perspective. Front Oncol. (2022) 12:924245. doi: 10.3389/fonc.2022.924245
189. Sinha T, Khan A, Awan M, Bokhari SFH, Ali K, Amir M, et al. Artificial intelligence and machine learning in predicting the response to immunotherapy in non-small cell lung carcinoma: A systematic review. Cureus. (2024) 16:e61220. doi: 10.7759/cureus.61220
190. Shu Y, Xu W, Su R, Ran P, Liu L, Zhang Z, et al. Clinical applications of radiomics in non-small cell lung cancer patients with immune checkpoint inhibitor-related pneumonitis. Front Immunol. (2023) 14:1251645. doi: 10.3389/fimmu.2023.1251645
191. Prelaj A, Miskovic V, Zanitti M, Trovo F, Genova C, Viscardi G, et al. Artificial intelligence for predictive biomarker discovery in immuno-oncology: A systematic review. Ann Oncol. (2024) 35:29–65. doi: 10.1016/j.annonc.2023.10.125
192. Mayo ZS, Halima A, Broughman JR, Smile TD, Tom MC, Murphy ES, et al. Radiation necrosis or tumor progression? A review of the radiographic modalities used in the diagnosis of cerebral radiation necrosis. J Neurooncol. (2023) 161:23–31. doi: 10.1007/s11060-022-04225-y
193. Sinigaglia M, Assi T, Besson FL, Ammari S, Edjlali M, Feltus W, et al. Imaging-guided precision medicine in glioblastoma patients treated with immune checkpoint modulators: research trend and future directions in the field of imaging biomarkers and artificial intelligence. EJNMMI Res. (2019) 9:78. doi: 10.1186/s13550-019-0542-5
194. Galldiks N, Wollring M, Werner JM, Friedrich M, Fink GR, Langen KJ, et al. An updated review on the diagnosis and assessment of post-treatment relapse in brain metastases using PET. Expert Rev Neurother. (2022) 22:915–21. doi: 10.1080/14737175.2022.2162880
195. Vladimirov N, Perlman O. Molecular MRI-based monitoring of cancer immunotherapy treatment response. Int J Mol Sci. (2023) 24:3151. doi: 10.3390/ijms24043151
196. Chen Y, Yang C, Sheng L, Jiang H, Song B. The era of immunotherapy in hepatocellular carcinoma: the new mission and challenges of magnetic resonance imaging. Cancers. (2023) 15:4677. doi: 10.3390/cancers15194677
197. Coudray N, Ocampo PS, Sakellaropoulos T, Narula N, Snuderl M, Fenyo D, et al. Classification and mutation prediction from non-small cell lung cancer histopathology images using deep learning. Nat Med. (2018) 24:1559. doi: 10.1038/s41591-018-0177-5
198. Hellwig K, Ellmann S, Eckstein M, Wiesmueller M, Rutzner S, Semrau S, et al. Predictive value of multiparametric MRI for response to single-cycle induction chemo-immunotherapy in locally advanced head and neck squamous cell carcinoma. Front Oncol. (2021) 11:734872. doi: 10.3389/fonc.2021.734872
199. Isaac MFG, Alkhatib R, Ho CL. MRI characteristics of chemotherapy-related central neurotoxicity: A pictorial review. Insights Imaging. (2024) 15:12. doi: 10.1186/s13244-023-01602-7
200. Kurokawa R, Ota Y, Gonoi W, Hagiwara A, Kurokawa M, Mori H, et al. Mri findings of immune checkpoint inhibitor-induced hypophysitis: possible association with fibrosis. AJNR Am J Neuroradiol. (2020) 41:1683–9. doi: 10.3174/ajnr.A6692
201. Müller-Jensen L, Zierold S, Versluis JM, Boehmerle W, Huehnchen P, Endres M, et al. Characteristics of immune checkpoint inhibitor-induced encephalitis and comparison with HSV-1 and anti-LGI1 encephalitis: A retrospective multicentre cohort study. Eur J Cancer. (2022) 175:224–35. doi: 10.1016/j.ejca.2022.08.009
202. Salam S, Lavin T, Turan A. Limbic encephalitis following immunotherapy against metastatic Malignant melanoma. BMJ Case Rep. (2016) 2016:bcr2016215012. doi: 10.1136/bcr-2016-215012
Keywords: immunotherapy, brain metastasis, malignant glioma, tumor progression, pseudoprogression, advanced imaging, MR perfusion imaging
Citation: Liu X, Chen H, Tan G, Zhong L, Jiang H, Smith SM and Wang HZ (2024) A comprehensive neuroimaging review of the primary and metastatic brain tumors treated with immunotherapy: current status, and the application of advanced imaging approaches and artificial intelligence. Front. Immunol. 15:1496627. doi: 10.3389/fimmu.2024.1496627
Received: 15 September 2024; Accepted: 28 October 2024;
Published: 28 November 2024.
Edited by:
Qiang Wen, Stanford University, United StatesReviewed by:
Xinyan Xu, University of Texas MD Anderson Cancer Center, United StatesBo Cheng, Shandong University, China
Copyright © 2024 Liu, Chen, Tan, Zhong, Jiang, Smith and Wang. This is an open-access article distributed under the terms of the Creative Commons Attribution License (CC BY). The use, distribution or reproduction in other forums is permitted, provided the original author(s) and the copyright owner(s) are credited and that the original publication in this journal is cited, in accordance with accepted academic practice. No use, distribution or reproduction is permitted which does not comply with these terms.
*Correspondence: Xiang Liu, bGl1eGlhbmd4bDcyQDE2My5jb20=; Henry Z. Wang, SGVucnlfV2FuZ0BVUk1DLlJvY2hlc3Rlci5lZHU=