- 1Children’s Hospital Oakland Research Institute, Oakland, CA, United States
- 2University of California San Francisco, Oakland, CA, United States
More than 50 years have elapsed since the association of human leukocyte antigens (HLA) with type 1 diabetes (T1D) was first reported. Since then, methods for identification of HLA have progressed from cell based to DNA based, and the number of recognized HLA variants has grown from a few to tens of thousands. Current genotyping methodology allows for exact identification of all HLA-encoding genes in an individual’s genome, with statistical analysis methods evolving to digest the enormous amount of data that can be produced at an astonishing rate. The HLA region of the genome has been repeatedly shown to be the most important genetic risk factor for T1D, and the original reported associations have been replicated, refined, and expanded. Even with the remarkable progress through 50 years and over 5,000 reports, a comprehensive understanding of all effects of HLA on T1D remains elusive. This report represents a summary of the field as it evolved and as it stands now, enumerating many past and present challenges, and suggests possible paradigm shifts for moving forward with future studies in hopes of finally understanding all the ways in which HLA influences the pathophysiology of T1D.
1 Introduction
The human leukocyte antigen (HLA) region genes are well established to be the strongest contributors to genetic risk for type 1 diabetes (T1D) (1, 2). The association of HLA with T1D was first reported more than 50 years ago (3), closely following a report of “no relation” of HL-A with juvenile diabetes (4). Since then, more than 5,000 reports have been published on the study of HLA-associated T1D risk. Early studies reported data generated from cell-based, serologic assays. With the introduction of polymerase chain reaction (PCR) in the 1980s, DNA-based HLA genotyping methods were developed, first using differential amplification- (SSP: sequence-specific priming) or hybridization- (SSOP: sequence-specific oligonucleotide probes) based methods, progressing to DNA sequencing of amplified targets (SBT: sequence-based typing), and leading to next generation sequencing (NGS) of increasingly larger regions of the HLA genes. The latest improvement in sequencing technology methods is long read sequencing, which was dubbed the “method of the year” for 2022 and can allow sequence reads tens of thousands of bases long (5). This method enables detection of all polymorphisms in exons, introns, and untranslated sequences in any given HLA gene and facilitates direct determination of phase (assignment of sequence reads to a particular chromosome) and direct determination of haplotypes (sets of HLA alleles found in cis on one chromosome). The number of HLA loci, the extreme polymorphism of those loci, and the strong linkage disequilibrium (LD: non-random tendency of alleles to be found together) between particular loci, create a complex system where results can easily be confounded and misinterpreted. Other polymorphic immune system genes, such as killer-cell immunoglobulin-like receptors (KIRs), found on NK cells, and leukocyte immunoglobulin-like receptors (LILR), primarily found on myeloid antigen-presenting cells, are less well understood, although some have been implicated in T1D risk. Even with more than 50 years of study, the basis of HLA-associated T1D risk is not completely elucidated. The recent Food and Drug Administration approval of teplizumab as a treatment to delay beta-cell loss increases the urgency for early detection of T1D (6–9). Identification of individuals at high T1D risk is important for optimal disease monitoring and patient stratification for use of teplizumab and other, future treatment and prevention strategies. This report is intended to provide historical perspective on the association of HLA with T1D, summarize the current state of the field, and provide suggestions for moving the field forward with the goal that, one day, the complexity of HLA-associated T1D risk will finally be fully understood and will play a key role in accurate diagnosis, management, precision treatment, and even prevention of the disease.
2 The basics of HLA structure and function
2.1 HLA antigens
Classical HLA antigens are cell-surface molecules that include a peptide binding groove in which either endogenous (for HLA class I) or exogenous (for HLA class II) peptides bind and are presented to the T-cell receptor, creating the “tri-molecular complex.” Signaling through this complex initiates the T cell response to the antigenic peptide, which can result in anergy, regulatory function, or effector function. Both HLA class I and class II proteins have a similar external structure, with the peptide-binding groove formed by amino acid residues in two immunoglobulin-like domains; however, class II antigens are heterodimeric proteins comprised of separately encoded alpha and beta polypeptides, while class I antigens are comprised of a single polypeptide chain that forms a complex with the relatively invariant β2-microglobulin molecule (2).
The genes encoding classical HLA (class I A, B, and C and class II DR, DQ, and DP) are the most polymorphic known in the human genome. As of December 2023, the number of alleles assigned in the IPD-IMGT/HLA allele database for classical HLA loci was 38,008 (10). Early, cell-based genotyping systems only distinguished a small number of categories for each locus. Rapid increases in identification of individual alleles began with the advent of DNA-based typing methods in the early 1990s. Even more rapid expansion of the catalog of named alleles was fueled by the development of NGS-based HLA genotyping methods around 2010, and, more recently, advances in long-read sequencing technology have led to the ability to sequence through and phase the entire ~4 Mb HLA region. Not surprisingly, most polymorphic sites in HLA molecules are located in the exons encoding the peptide-binding groove, creating the basis for presentation of a large repertoire of peptides for each of the classical HLA antigens. Exons encoding the peptide-binding groove (exon 2 in class II and exons 2 and 3 in class I) were the first to be targeted in molecular genotyping methods. Current methods can determine the entire sequence of an HLA gene, including exons, introns, and untranslated sequences, allowing exact identification of the alleles in a genotype. In addition, high-depth coverage and long read sequencing allow phase determination and assignment of individual alleles to haplotypes on each chromosome (11).
2.2 Nomenclature
Nomenclature for HLA has evolved over many years. The current standard includes the locus name, followed by an asterisk separator, followed by up to four numeric fields with colon separators, and, in some cases, by a letter (N, L, S, C, A, or Q) indicating expression status (12). For most loci, the first field describes the allele group, generally related to defined serologic specificity, the second field represents the individual allele within the group as defined by differences in the encoded amino acids, the third field reflects silent changes in the codons, and the fourth field reflects variation in intron and untranslated sequences. The DPB1 locus differs from others in that most alleles have been discovered since DNA-based genotyping was implemented. For nearly all DPB1 alleles, the first field simply represents the order in which the alleles were discovered, rather than a particular, serologically defined allele group, and the second field is almost exclusively “01.” A review of the history of HLA nomenclature was recently published (13).
Given the cumbersome nature of the individual allele designations, for example, HLA-A*01:01:01:01N, many reports use abbreviated definitions for particular alleles. For example, the allele DRB1*03:01:01:01 is sometimes referred to as simply “DR3” but can be referred to using up to four fields of resolution. In some reports, the term “DR3” may refer to the individual allele at the DRB1 locus or may refer to haplotypes that carry the DRB1*03:01:01:01 allele. Thus, interpretation of data from association studies must be done with careful consideration of the genotyping methodology, the resolution, and the authors’ preferences in reporting nomenclature. Despite efforts to standardize reporting information for HLA genotyping, results for individual studies still vary in the nomenclature used (14).
Class I antigens (HLA-A, -B, and -C) are formed by a single polypeptide chain (combined with β2-microglobulin); their designations refer to a single locus but can vary from simple designations that only reflect specificity at the serologic level (e.g., “A1”) to complete whole gene (four-field) specificity with expression data (e.g., A*01:01:01:01N). Class II antigens (HLA-DR, -DQ, and -DP) are heterodimers composed of the products of two genes (“A” and “B,” encoding the α and β polypeptide chains, respectively). For genes encoding DR, nearly all the polymorphism is found in the DRB genes, with the variability in the DRA1 gene so low that the locus is usually not genotyped. Thus, nomenclature for DR-encoding loci generally consists simply of a description of the DRB allele. All HLA regions contain a DRB1 gene; however, other DRB genes, both the expressed DRB3, DRB4, and DRB5 loci and the pseudogenes DRB2, DRB6, DRB7, DRB8, and DRB9, are present on some, but not all, chromosomes (Figure 1). They are found in distinct LD patterns with particular DRB1 alleles. Each chromosome carries a maximum of one secondary, expressed DRB locus (i.e., DRB3, DRB4, DRB5) (see section 3.7). Thus, although they are separate loci, DRB3, DRB4, and DRB5 can be analyzed as if they were alleles of one locus.
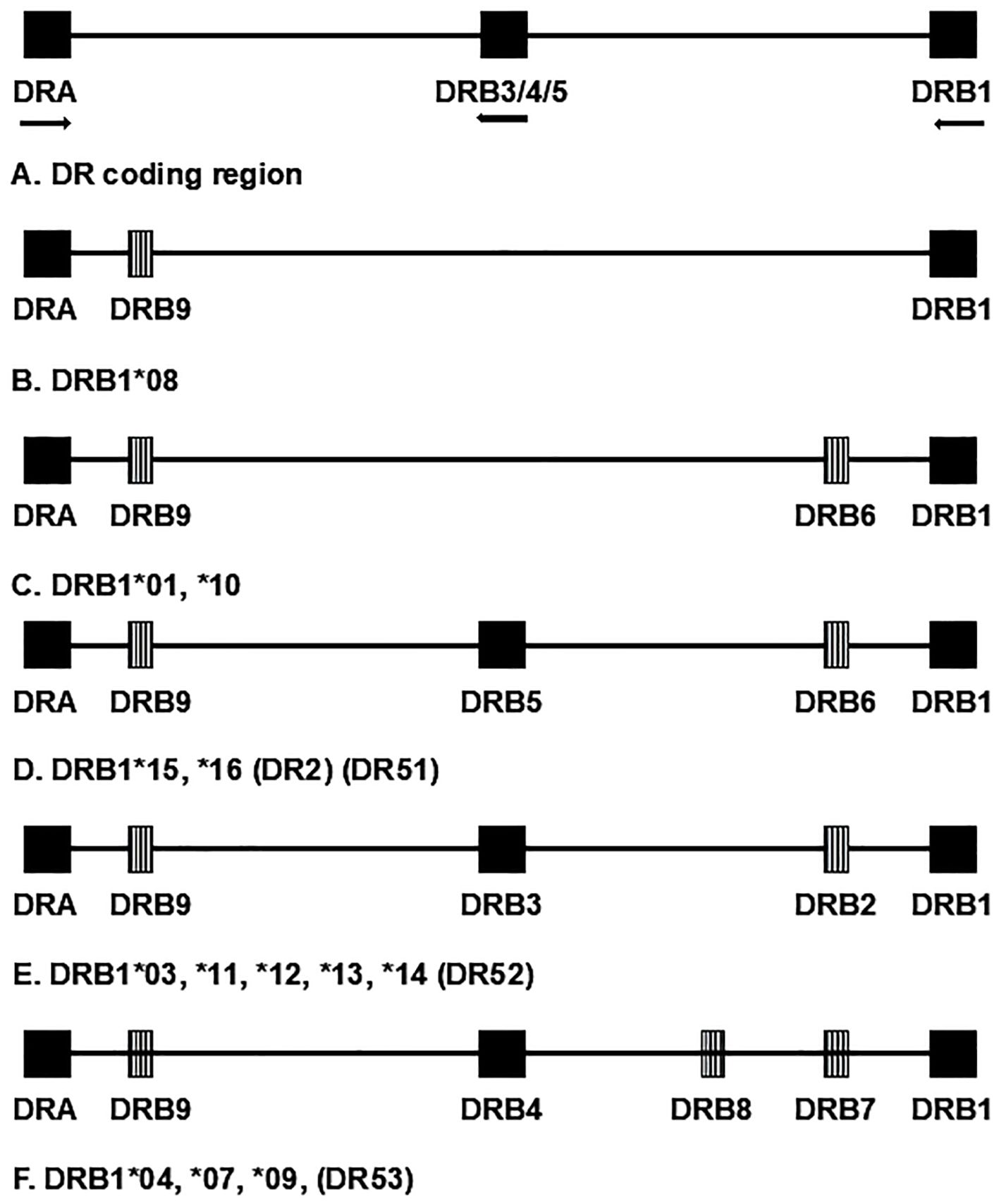
Figure 1. Schematic representation of chromosomal region encoding HLA-DR antigens; not to scale. Although they are separate loci, DRB3, DRB4, and DRB5 are separate loci but are represented as a single locus for convenience. Expressed loci are represented by solid blocks. Striped blocks represent pseudogenes. Arrows designate direction of transcription for expressed genes. Chromosome groups are designated by the first-field nomenclature, for example, DRB1*01, which represents the corresponding serologic group. Alternate, historical name categories for the groups are given in parentheses.
In contrast, for genes encoding DQ, both the DQA1 and DQB1 loci are polymorphic, and both must be genotyped to fully describe the DQ antigen. One way to do this is to write out the full DQA1-DQB1 haplotype (e.g., DQA1*05:01~DQB1*02:01); however, for brevity, many reports use abbreviations to represent the haplotype. In fact, these abbreviations describe the heterodimeric antigen formed by the combination of polypeptide products of the DQA1 and DQB1 loci. Common DQ abbreviations relevant to T1D risk include DQ2.5 (DQA1*05:01~DQB1*02:01), DQ8 (DQA1*03:01~DQB1*03:02), DQ7 (DQA1*05:01~DQB1*02:01), DQ6.2 (DQA1*01:02~DQB1*06:02), and DQ9.3 (DQA1*03:02~DQB1*03:03).
For DP, as for DQ, genes encoding both chains of the antigen are polymorphic. Consistent with other class II antigens, the DPB1 gene is far more polymorphic than is the DPA1 gene, with 2,486 and 639 reported alleles, respectively (10). Until recently, DPA1 was rarely genotyped, and DP data, when available, were nearly always reported in the same way as DR data, simply by using the allele designation for the DPB1 gene. As genotyping technology evolves, more reagents are becoming available for genotyping DPA1, particularly since many assay systems are now multiplexed. Therefore, the proportion of reported DPA1~DPB1 haplotype data, compared to DPB1 data alone, is increasing. While some DPB1 alleles are seen in haplotype with a single DPA1 allele, others, like DPB1*02:01, can be seen in cis with one of multiple DPA1 alleles (15). Data reports for DP association do not utilize abbreviations analogous to those used for DQ association. Interpretation of DP genotyping results requires information about whether the data presented represent only the DPB1-encoded portion of the antigen (β chain) or both the DPA1 and DPB1-encoded portions (α and β chains). Since both the alpha and the beta chains contribute to the shape of the antigen-binding groove, identification of both chains is important to fully understand the structure of the resulting DP antigen. Given the fact that both DPA1 and a DPB1 products contribute to the peptide binding groove of the DP antigen, and given the discovery that some DP antigens can serve as ligands for NK cell proteins and influence innate immunity, identification of both the DPA1 and DPB1 loci in an individual is becoming increasingly important (16, 17).
2.3 Resolution
Generation and analysis of data at the highest resolution possible (four-field) for any study involving HLA genotyping may seem optimal, but highest resolution is not always the best basis for an association study. Increased resolution of a genotyping study generally involves increased cost. The perfect disease association study would sample every individual in the entire global population and sequence every genome in its entirety, providing a complete data set that could be stratified by any genetic criteria desired, for example, using Ancestry Informative Markers (AIMs), before application of any association analysis method. Clearly, such a study is neither practical nor even possible. In the absence of this ideal, each study design must consider the level of resolution necessary to best address the hypothesis being tested, taking into account the resources available for the study. Most studies of HLA and disease association report data at a resolution of two fields (previously referred to as “4-digit” resolution), because the first two fields contain all the information defining the amino acids encoded for the protein. Changes in the third or fourth field do not affect which amino acids are included in a given allele. In rare cases, information found in third or fourth fields might be relevant to gene expression, through potential mechanisms such as altered codon usage, changes in splice donor or acceptor sequences, or presence of an enhancer. On a practical level, however, third and fourth field information adds little to a disease association study. The primary function of HLA molecules is to present peptides; thus, two molecules that contain coding sequence for the same string of amino acid residues are expected to have the same structure and bind the same repertoire of peptides. If the coding sequence for those two antigens differs by silent changes in codons or untranslated sequences, considering them as different alleles in an association analysis could effectively decrease the statistical power of the study and mask association effects. Since three- or four-field resolution data can be collapsed to two-field resolution, generating data at the highest level of resolution within the scope of the study is desirable. However, for cases in which generating data at four-field resolution is not possible or greatly reduces the number of samples that can be tested with the resources available for a project, generating data at the two-field level (coding sequence only) may be the preferred option. Each disease association project is unique, and many studies have been, and continue to be, performed with low- or moderate-resolution output, creating useful data but also creating challenges for comparing or combining data among studies.
Varying resolution represents a significant problem not only for disease association studies but also for combining data in any HLA-related study involving samples that were genotyped at varied levels of resolution. For example, large transplant registries, such as the National Marrow Donor Program (NMDP), have data spanning the evolution of genotyping technology, creating challenges for optimizing matching of potential donors to recipients. One report from the NMDP approached this issue by examination of 6.59 million subjects categorized into 21 race categories, with over 1.2 million European Caucasian subjects, using expectation maximization algorithms to resolve allelic ambiguity and phase haplotypes (18). A similar strategy may be applicable for combining data from a large number of disease association studies. For resolution, decreasing the level is simple. Increasing the level is more complex and must be done by informatics technology. While not as accurate as direct genotyping of all samples, imputation of high-resolution data from low-resolution data can aid in allowing the generation of large and meaningful datasets without additional, and perhaps cost-prohibitive, direct genotyping assays. That said, drawing conclusions from imputed data must always be done with caution and take into account that all data were not experimentally determined. For data with direct clinical consequences, such as matching donor-recipient pairs, imputed data must be confirmed experimentally before performing clinical procedures.
3 HLA allele, haplotype, and genotype associations with T1D
3.1 Discovery
Early publications of HLA association with T1D reported serologic association of class I antigens “HL-A8” and “W15.” (3, 19, 20) In 1980, Barbosa reported increased frequency of class II antigens then termed “Dw3” and “Dw4” and decreased frequency of “Dw2” as well as increased frequency of the Dw3/Dw4 heterozygous genotype (21). These results still stand, having been replicated and published in thousands of studies. Although the full extent of the effect of HLA on T1D susceptibility remains to be determined, the depth and breadth of the field has expanded enormously over 50 years. T1D risk for HLA has consistently been demonstrated to be strongest for genes encoding HLA-DR and -DQ antigens, with risk clearly apparent for each locus in nearly every published study. The vast majority of T1D risk studies report association data in the form of Odds Ratio (OR), which reflects the frequency of the tested allele, haplotype, or genotype in T1D patients compared to that in controls, and each OR is given with a measure of statistical significance in the form of a probability (p) value. An OR of 1.0 denotes no association between the tested parameter and disease; OR greater than 1 denotes potential positive association (predisposition to disease), and OR less than 1 denotes potential negative association (protection from disease). Significance is generally accepted as a p-value of 0.05 or lower after appropriate statistical corrections.
3.2 DR3, DR4, and the DR3/DR4 genotype
The DRB1*03:01~DQA1*05:01~DQB1*02:01 haplotype, sometimes referred to as “DR3-DQ2.5,” or even simply “DR3,” is positively associated with T1D in nearly every population reported to date, although the strength of the association varies among populations. While the extreme polymorphism of the HLA genes creates a daunting number of potential antigens, in practice, only a small subset of named alleles, and, therefore, antigens encoded by them, is present in any given population. At the two-field level of resolution, 211 DRB1*03 alleles have been reported (10). However, only two of those alleles, DRB1*03:01 and DRB1*03:02 (the latter seen almost exclusively in Black populations) are found at appreciable frequency (≥0.5%), with the remaining alleles too rare to be of use for disease association studies (18). The DQ-encoding haplotype DQA1*05:01~DQB1*02:01 is in very strong LD with DRB1*03:01; thus, stratification on the DRB1*03:01~DQA1*05:01~DQB1*02:01 haplotype allows evaluation of risk for alleles at other HLA loci (e.g., class I, DPB1) and non-HLA loci (e.g., TNFA) in the region without confounding by heterogeneity of DR- and DQ-encoding loci. Some HLA haplotypes are highly conserved, including the DR3 haplotype sometimes referred to as “B8-DR3,” “A1-B8-DR3,” or “8.1,” which was the second most common haplotype observed in a set of over 6 million samples from the NMDP repository (18). The observation that DR3 haplotypes containing HLA-B*08:01 confers less T1D risk than other DR3 haplotypes, particularly “B18-DR3,” has been consistently reported, beginning as early as 1988 (22–26). Other extended “DR3” haplotypes have been seen in varying populations. For example, A2-B8-DR3, A2-B50-DR3, A33-B58-DR3, A24-B8-DR3, and A26-B8-DR3 are all T1D risk haplotypes in the Indian population, while A33-B17-DR3 was suggested as a risk haplotype in Chinese (27, 28).
Additional class II susceptibility is attributable to “DR4,” which denotes DRB1*04:xx alleles, or haplotypes carrying them. DR4-associated T1D risk is more complex than that of DR3, because DR4 haplotypes are far more varied than DR3. To date, 375 DRB1*04 alleles have been named (10). At least nine of those are reported to be at a frequency ≥1% in at least one population (18). A hierarchy of risk exists for DRB1*04:xx alleles, with DRB1*04:05 representing very high risk, followed by others, including DRB1*04:01, DRB1*04:02, and progressing to DRB1*04:03, which has no risk, but is actually protective, for T1D (2). In addition, the DQ-encoding haplotypes that are found coupled to these DRB1 alleles can vary. In particular, DQA1*03:01~DQB1*03:02 (DQ8) is well-established to be a T1D susceptibility haplotype, while DQA1*03:01~DQB1*03:01 (DQ7) is generally T1D protective, suggesting that T1D risk is more dependent on DQ than on DR (29, 30). On the other hand, the haplotype DRB1*04:01~DQA1*03:01~DQB1*03:02 is an established T1D risk haplotype, while DRB1*04:03~DQA1*03:01~DQB1*03:02 is T1D protective, suggesting that the T1D risk is attributable to DR and not DQ. This conundrum creates a need for large and diverse data sets to evaluate the effects of individual alleles and haplotypes. In some populations, such as those of European descent, 90%–95% of T1D patients carry either DR3 or DR4 on one chromosome. Due to the increasing availability of large sample sets, studies of the subset of patients who carry neither have become feasible to help elucidate predictors for T1D in individuals without the highest risk (31).
In addition to risk provided by individual HLA alleles and haplotypes, particular HLA genotypes are known to confer risk that is not explained by the additive effects of the alleles or haplotypes that are included in the genotype. The most well-known example of this is the very high risk conferred by the heterozygous “DR3/DR4” genotype, in which one chromosome carries the DR3 haplotype and the other carries a high-risk DR4 haplotype (excluding those containing DRB1*04:03 or DQA1*03:01~DQB1*03:01). Augmented risk for the DR3/DR4 genotype was reported as early as 1980, and the genotype can be seen in up to 45% of patients in some studies (21, 30). In fact, the risk for the DR3/DR4 genotype is so strong that many studies stratify by those patients and analyze them as a separate group from the remaining patients (32–35). For decades, a comprehensive explanation for the more than additive risk of the DR3/DR4 genotype has remained elusive; however, a leading hypothesis involves DQ heterodimeric antigens encoded in trans (2). Figure 2A depicts the two DR-DQ haplotypes that are commonly found in a high-T1D risk individual of European descent and illustrates the four combinations of DQA1 and DQB1 loci whose products could theoretically combine to create a DQ antigen. The combination of DQA1*03:01 and DQB1*02:01, depicted here in trans, is frequently seen encoded in cis and has been reported to be associated with increased T1D risk (36). Although the combination of DQA1*05:01 and DQB1*03:02 has not been described encoded in cis, one report provides functional data to support the genetics-based hypothesis that an antigen formed from the products of those two alleles may increase T1D risk (37). Differences were demonstrated in antigenic peptide repertoire binding profiles for the traditional cis-encoded “DQ8” (DQA1*03:01~DQB1*03:02 = “DQ8cis”) antigen and for the trans-encoded antigen, (DQA1*05:01~DQB1*03:02 = “DQ8trans”), that are consistent with higher T1D risk for DQ8trans than for DQ8cis. The DR3/DR4 heterozygous genotype seen in Figure 2A allows the expression of DQA1*05:01 and DQB1*03:02 in the same individual, creating a unique opportunity for formation of a particularly high-risk DQ molecule.
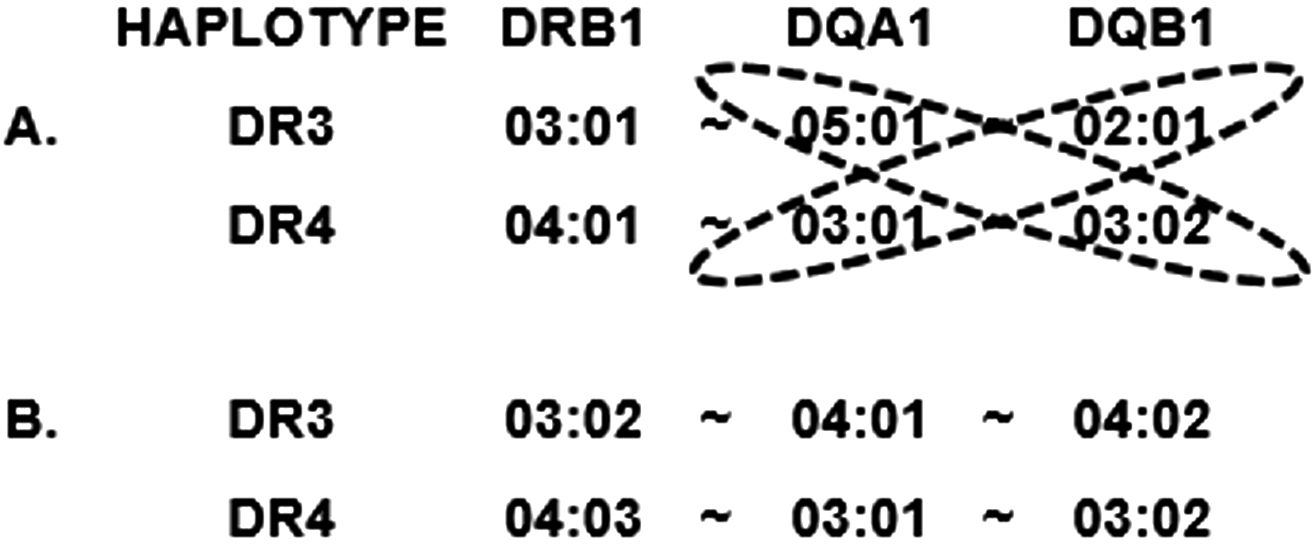
Figure 2. Representation of individual haplotypes that can be found in a “DR3/DR4” heterozygous individual. (A) Typical DR3/DR4 genotype for a high-risk individual of European descent. Potential allele pairs that could produce trans-encoded DQ molecules are circled. (B) Potential DR3/DR4 haplotype from mixed-population individual with one Asian and one Black parent, composed of two T1D-protective haplotypes.
3.3 Other DR and DQ T1D risk alleles, haplotypes, and genotypes
While DR3, DR4, and the DR3/DR4 genotype are the most commonly recognized HLA risk factors for T1D, many other alleles, haplotypes, and genotypes have been reported in 50 years of study. Thomson et al. used a method termed Relative Predispositional Effects (RPE) to look for additional T1D risk alleles beyond DR3 and DR4 and found evidence for risk from DR8 and DR1 (38, 39). Perhaps the most comprehensive study of HLA and T1D risk was the Type 1 Diabetes Genetics Consortium (T1DGC) (40, 41). Notably, HLA association with T1D was not originally intended as a primary outcome for the T1DGC; the purpose of the international study was to discover all the T1D susceptibility loci other than the classical HLA loci, including both immune system and non-immune system genes. However, because of the extremely strong T1D association with HLA, proper evaluation of all genetics-based T1D association studies should include HLA context. This is especially true for loci in the same chromosomal region as the HLA loci (chromosome 6p), including genes in the class III region. As part of the T1DGC effort, over 14,000 individuals, from multiple populations, were genotyped for all classical HLA loci (except DRB3, DRB4, and DRB5) at two-field level with exon-based resolution using PCR-Sequence-Specific Oligonucleotide Probe (PCR-SSOP) technology. Data for DR- and DQ-encoding genes, DP-encoding genes, and HLA class I-encoding genes were analyzed and published (15, 29, 42). Table 1 shows the most common predisposing and protective DRB1~DQA1~DQB1 haplotypes for T1DGC.
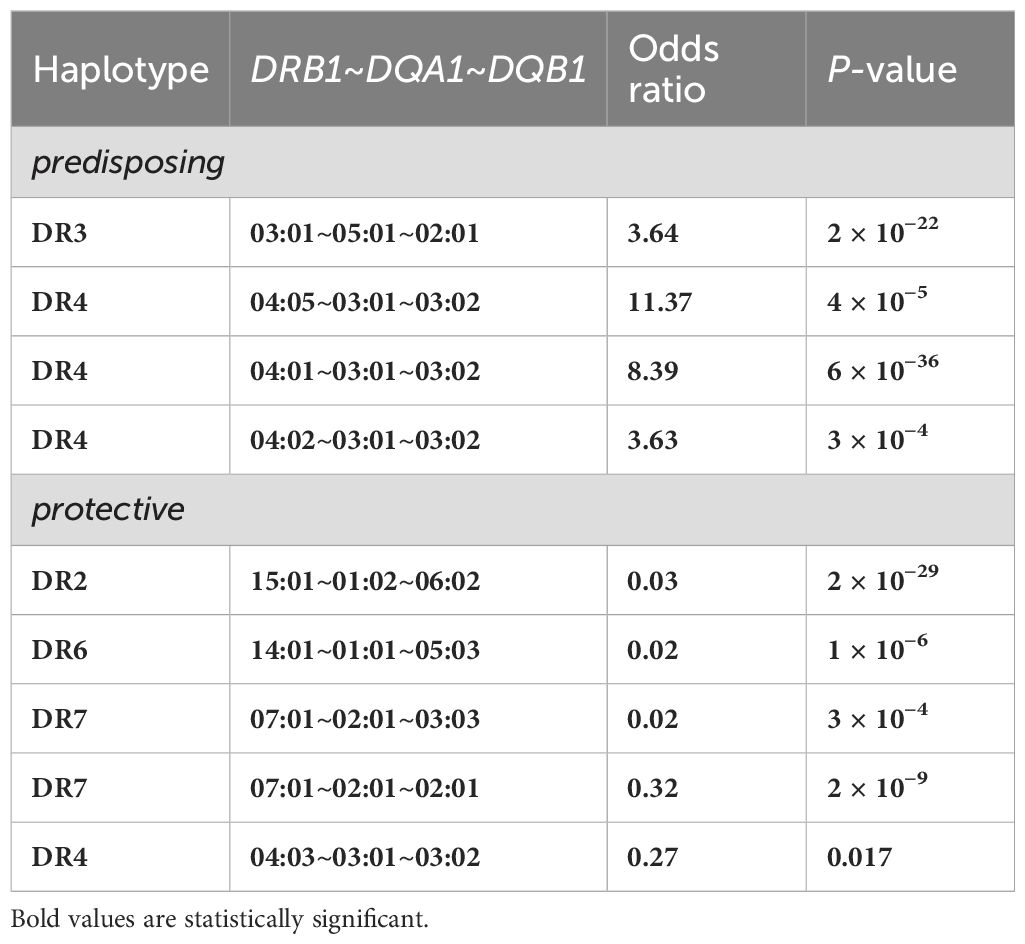
Table 1. Highly-significant DR-DQ encoding haplotypes for subjects of European descent resulting from the Type 1 Diabetes Genetics Consortium (T1DGC) study (29).
Most studies of HLA and T1D risk have been performed on individuals of European descent, not only due to the high prevalence of T1D in that group but also due to the relative ease of recruiting subjects compared to other races and ethnicities. Lack of diversity in published studies can create challenges for thorough understanding of HLA associations with T1D and is described more fully in section 4.1 below. HLA allele frequencies vary enormously among populations as do the combinations of those alleles into haplotypes. Studies of subjects not of European descent are critical for comprehensive understanding of HLA-associated T1D risk. An illustrative example can be seen for DR9. The frequency of DRB1*09:01 is high (up to ~15%) in Asian populations, including Japanese, Chinese, and Vietnamese but very low (~1%) in European populations (18). In Europeans, an association of DRB1*09:01 with T1D would require a very large study to be observed; however, association with DR9, and particularly with the DR3/DR9 genotype, was reported many decades ago in Asian populations (27, 43–45). Another genotype that is relatively uncommon, DR4/DR8, has been shown to be a risk factor for T1D, both in individuals of European descent and in Japanese (46). The increasing admixture among populations can further confound data interpretation. For example, the DR3 haplotype that is exceedingly rare in Europeans but common in Africans (DRB1*03:02~DQA1*04:01~DQB1*04:02) (see section 3.2) is actually protective, rather than predisposing, for T1D (36). Similarly, the DR4 haplotype common in Asians (DRB1*04:03~DQA1*03:01~DQB1*03:02) is protective, rather than predisposing, for T1D. Finding both of these haplotypes in a single individual is unlikely in non-admixed populations but could easily happen in an admixed population, where a child might have one Asian and one Black parent. In that case, a low-resolution genotyping could produce a result of a called DR3/DR4 genotype, leading to an assumption of high T1D risk, in an individual whose HLA genotype is highly protective. This hypothetical example (depicted in Figure 2B) highlights the fact that both resolution and population context are important in evaluating data from HLA and T1D association studies.
3.4 T1D protection from DR and DQ-encoding alleles
HLA not only confers risk for T1D but also strong protection. The 1980 publication that implicated class II, rather than class I, HLA in T1D risk also reported a decrease in the “Dw2” antigen, which is sometimes termed “DR2” and includes the DRB1*15 and DRB1*16 allele groups (21). In studies of individuals of European descent, the haplotype DRB1*15:01~DQA1*01:02~DQB1*06:02 is, by far, the most frequent DR2-encoding haplotype observed and is consistently associated with T1D protection (29, 30, 39). The closely related haplotype DRB1*15:03~DQA1*01:02~DQB1*06:02 (African-specific) is also highly protective for T1D (36). Importantly, however, the alleles included in DR2-encoding haplotypes and their frequencies vary widely among populations and not all DR2-endocding haplotypes are highly protective (18, 36, 47). DQB1 genes that encode aspartic acid at position 57 are usually protective for T1D, although not universally (48–50). DR2-based T1D protection appears dominant, although the mechanism for the protection is, as yet, unknown. One leading hypothesis is “epitope stealing,” that is, competition for binding of antigenic peptides (51, 52). Another is related to the high stability of the DP antigen encoded by DQA1*01:02~DQB1*06:02 (53–56). DR2 appears to be protective throughout the course of disease, from reducing risk of developing autoantibodies to reducing risk of progression to overt diabetes in individuals with antibody positivity (57).
Other class II haplotypes appear protective for T1D, including DRB1*14:01~DQA1*01:01~DQB1*05:03 and DRB1*07:01~DQA1*02:01~DQB1*02:02 (29). DR7-based T1D protection was observed in early studies of European-descent individuals but not as frequently in individuals of African descent (30, 58). The African version of the DR7 haplotype is DRB1*07:01~DQA1*03:01~DQB1*02:02, differing from the European version only at DQA1. That difference modifies the risk for the haplotype from protective (OR = 0.34) to predisposing (OR = 3.96), demonstrating the need for genotyping a more than a single locus to determine T1D risk (59). Other alleles and haplotypes can be T1D protective as well, but with less uniformity of observed risk effects. Alleles in the DRB1*11 and DRB1*13 groups can be found encoded in cis with many different DQA1-DBB1 haplotypes. T1D risk effects from DR11 and DR13 haplotypes vary widely within and among populations.
3.5 DPB1
HLA-DP is the remaining of the three class II classical HLA antigens. Like DR and DQ, DP is encoded by polymorphic genes and presents peptide antigens to T-cell receptors, making it a logical candidate to convey T1D risk. Association of the DPB1 locus with T1D has been examined in many studies and in many populations, although some reports have suggested no association of DP with T1D (15, 26, 34, 60–65). The association of DPB1 with T1D tends to be less strong and less consistent than that of the DR- and DQ-encoding loci (15). Perhaps the most consistently observed T1D association for DPB1 and T1D is a protective effect for DPB1*04:02, particularly notable on DR3 haplotypes; however, a recent study from Mali showed a very highly predisposing effect for DPB1*04:02 (OR = 12.73) in that population, which, upon closer scrutiny, appeared to be attributable to the presence of DPB1*04:02 on a highly T1D-predisposing extended HLA haplotype rather than to an effect of the allele itself (30, 34, 60, 62, 66). The mechanism(s) for the influence of DPB1 on T1D remain to be fully elucidated. Possibilities include direct effects of peptide binding for adaptive immunity, LD with other risk loci, or a novel mechanism that may involve the innate immune system (see section 5.2 below).
3.6 HLA class I A, B, and C
Original reports for associations of HLA with T1D were for class I serotypes; however, those associations were later shown to be attributable to HLA class II loci in LD with loci encoding those serotypes (3, 19–21). T1D is characterized by an adaptive immune response that leads to destruction of pancreatic beta cells. T-cell killing involves class I HLA molecules; thus, a reasonable assumption is that HLA class I genes might confer susceptibility to T1D, particularly affecting beta cell destruction and disease progression. To be performed properly, HLA class I analyses must account for LD with class II DR- and DQ-encoding genes. Many studies have been published examining class I associated T1D risk (HLA-A, -B, and -C) (26, 33, 42, 67–69). A*24, specifically, A*24:02, is the most commonly reported T1D risk allele for HLA-A (33, 42, 69, 70). A*24 has been associated with low residual beta-cell function, rapidly progressing disease, and poor outcome for islet allografts (71–75). HLA-B*39:06 is the most commonly and consistently T1D-associated allele reported for the HLA-B locus and, like A*24:02, is reported to drive disease progression, including in a humanized mouse model (42, 67–70, 75, 76). HLA-C is the least commonly studied of the class I loci for T1D risk, and no allele has shown consistent associations among studies (42, 67, 77). In fact, in a recent study of HLA and T1D in Malian populations, The HLA-C and DPA1 loci were the only classical HLA loci not to reach statistically significant association at the locus level (66). Observed associations of particular HLA-C alleles with T1D can frequently be attributed to LD with class II or with HLA-B alleles. The lack of HLA-C alleles demonstrating consistent T1D risk effects among populations leads to the notion that the influence of HLA-C on T1D risk may be attributable to a mechanism different from the traditional presentation of antigen to T cells through the tri-molecular complex. Other mechanisms could include the role of the HLA-C antigen as a ligand for Killer cell Immunoglobulin-like Receptors (KIR, see section 5.2) or a different, as yet unidentified, mechanism unrelated to traditional antigen presentation.
3.7 DRB3, DRB4, and DRB5
The HLA DRB3, DRB4, and DRB5 genes, like DRB1, encode DRβ polypeptides that can form heterodimers with the product of the DRA gene to produce functional DR antigens. Unlike DRB1, these loci are not found on every copy of chromosome 6 but are present, in predictable LD patterns, in HLA regions with particular DRB1 loci. Each chromosome has a maximum of one secondary, coding DRB locus, shown schematically in Figure 1 (18). The similarity of these loci to DRB1 can interfere with DRB1 genotyping. In fact, for many years, most genotyping methods were specifically designed to exclude DRB3, DRB4, and DRB5 loci to prevent confounding of DRB1 genotyping data. Thus, historically, very few studies have reported data for these loci. In addition, the secondary DRB loci are far less polymorphic than DRB1. Most DRB1 alleles are found in combination with only one specific secondary allele. DRB1*03:01 represents one exception in that it can commonly be found in cis with either DRB3*01:01 or DRB1*02:02 in some populations. The secondary DRB loci have been examined for T1D susceptibility in a limited number of studies with varying results (78–81). Currently, many genotyping products include DRB3, DRB4, and DRB5; thus, future studies should allow better assessment of their T1D risk.
4 Challenges and requirements for determining T1D genetic risk
4.1 Diverse population studies
Because of the diversity of HLA alleles and genotype combinations of alleles among populations, limiting the scope of HLA association studies by studying only one or a small number of populations limits the ability to fully understand the full extent of HLA association with T1D. An allele with a strong risk effect could be missed if that allele is present at a frequency too low for proper analysis in the study subjects. Conversely, even modest risk effects can be revealed when an allele is present at a high frequency. In addition, strong LD of some haplotypes creates challenges in the interpretation of data. Effects for one allele in a conserved haplotype may be masked by effects of another allele in that haplotype.
Disease association studies rely on measuring differences between affected and unaffected individuals within a group. A properly designed study must have both case and control groups representing the same genetic background. Given resources to generate sufficient genetic information, this can be determined experimentally, for example, using AIMs; however, in practice, keeping genetic background consistent between cases and controls must be incorporated into selection strategies for study participants. Both consanguinity and population admixture can confound disease association studies; therefore, the selection of study participants is critical. Studies from distinct, carefully selected populations represents a good strategy for understanding HLA-associated T1D risk.
Many studies are performed on the basis of country of origin; however, political boundaries do not necessarily separate race or ethnicity. Conversely, geographic proximity does not necessarily indicate racial or ethnic similarity. One example can be seen in DRB1 data from Bangladesh and Pakistan (82, 83). Although these two countries both border North India, the HLA associations with T1D are very different, with mild association primarily due to DRB1*04:01 for Bangladesh and strong association, primarily due to DRB1*03:01 for Pakistan. In fact, the pattern of DRB1 association with T1D for Pakistan mush more closely resembles that for Somalia than for Bangladesh (84).
Most early HLA association studies as well as most large-scale studies have been performed on subjects of European descent; however, the number of diverse populations being examined is increasing. A PubMed search using the terms “HLA” and “T1D” returned more than 5,000 publications. Figure 3 depicts a world map with sources of studies indicated. Although some reports list ancestry or region as the source of subjects, most are reported by country. The data on the map are represented by country; any country from which HLA data have been reported in a T1D study is highlighted, even if the subjects were restricted to a small population or small geographic area. Other than for well-studied areas like the USA, Europe (especially Scandinavia), Japan, China, and India, many reports are from the past 8 years, and most lack replication. The map is not intended to provide the location of each specific population but simply to illustrate the current extent of the world coverage for HLA and T1D association studies. At a glance, the coverage seems quite broad. However, the reported studies vary greatly in the numbers of participants and the geographic area sampled, as well as which HLA loci were tested, which technology was used for genotyping, and what resolution level was reported. Table 2 lists references seen in PubMed for HLA and T1D studies from 2016 through June of 2024; Table 2 was used to inform Figure 3. Clearly, more data from Africa, South America, and South Asia are needed.
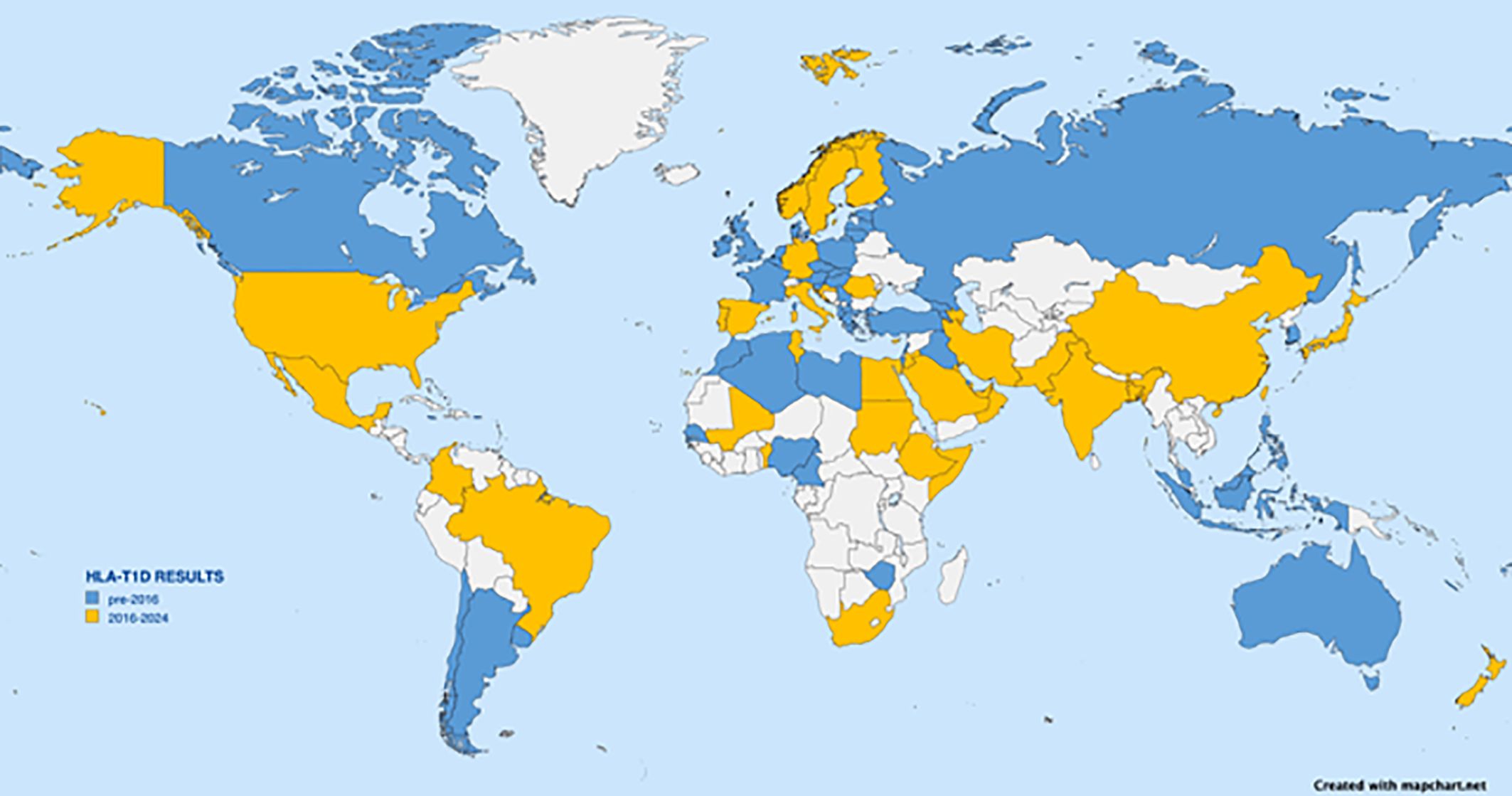
Figure 3. World map depicting countries from which HLA association with T1D have been reported. Any report from a given country, even if from a small region, results in indication of the country. Countries with reports dating from 2016 to June 2024 are shown in yellow. Citations from those countries are listed in Table 2 and include Albania, Algeria, Argentina, Armenia, Bahrain, Cameroon, Canary Islands (Spain), Chile, Czech Republic, Denmark, Estonia, Fiji, France, Georgia, Georgia, Greece, Hungary, Iraq, Israel, Jamaica, Java (Indonesia), South Korea, La Reunion Island, Latvia, Lebanon, Libya, Lithuania, North Macedonia, Malaysia, Martinique, Morocco, Naura, New Caledonia, Newfoundland (Canada), Nigeria, Philippines, Poland, Puerto Rico, Russia, Russia, Senegal, Serbia, Slovakia, Slovenia, Turkey, Uruguay, Wallis Islands, Zimbabwe. Countries with earlier reported data are shown in blue.
4.2 Good clinical characterization of subjects
An additional confounding factor for T1D studies is the clinical heterogeneity of the disease itself. Traditionally, diabetes has been considered a disease with two categories: type 1, previously referred to as “juvenile” or “insulin-dependent,” and type 2, previously called “adult-onset” or “non-insulin-dependent.” However, many other types of diabetes are now recognized (85). A recent addition to the field is the observation of diabetes as an adverse effect of immune checkpoint inhibitor therapy for cancer (86). Diseases in the category “T1D” are not clinically homogeneous. Presentation of T1D varies among patients from somewhat mild, with minimal insulin requirements, to fulminant, with rapid beta cell loss. Different forms of T1D, termed “endotypes,” are currently being reported, with a recognized need for better global epidemiology (87–89). Different clinical presentation and manifestation of disease may have different HLA associations. Combining subjects with different disease phenotypes could result in inability to recognize some HLA effects.
Yet another variable for T1D involves the antigen presented by the HLA molecules. The search for “the diabetes antigen” has been ongoing for decades. That more than one diabetes antigen exists has become well established, which helps explain why more than just one HLA allele is associated with T1D. Diabetes antigens include peptides formed from proteins recognized by the autoantibodies commonly seen in T1D patients, insulin (IAA), glutamic acid decarboxylase 65 (GAD65) Islet antigen 2 (IA-2), and Zinc transporter 8 (ZnT8). The specific autoantibodies found in T1D patients differ among patients both in the timing of their appearance (IAA tend to appear at earlier age) and the HLA haplotype(s) of the patients in whom they are found (IAA are commonly found in patients who carry DR4 haplotypes, while GADA are more commonly seen in patients with DR3) (90). The discovery that certain antigenic peptides, such as insulin B:9-23, can bind HLA in different registers, with different immunologic consequences, further complicates the immunology of T1D (91, 92). The recent discovery of hybrid insulin peptides (HIPs) adds even more complexity to the diabetes antigen repertoire (93–106). HIPs are created by the fusion of peptide fragments from more than one protein, for example, insulin and chromogranin A. Thus, the number of potential peptide antigens for T1D is much greater than what could be produced from the individual, recognized T1D associated antigens, further complicating the immunologic landscape of the disease.
4.3 In-depth analysis of HLA features, rather than alleles
HLA antigens for any given locus are structurally similar with small variations due to the amino acids encoded at a given position. Perhaps the most well-known T1D risk association attributed to a specific amino acid residue in an HLA allele is the effect of position 57 in the DQB1 locus identified in the 1980s (section 3.4) (49, 50). In a much more recent study, effects of individual amino acids on T1D susceptibility were examined in a large cohort of more than 18,000 subjects using imputation of SNP data from the Immunochip array to call HLA genotypes (107). In that study, position 57 of the DQB1 locus had the strongest effect on T1D risk, with strong associations also seen for DRB1 positions 13 and 71. In addition, independent T1D associations were observed for amino acid residues in HLA-A, -B, -DRB1, -DQA1, -DQB1, and -DPB1 loci; however, no significant associations were observed for either HLA-C or -DPA1. Consistent with that observation, a recent study of T1D association with HLA in a Maliian cohort showed overall T1Dassociation for all classical HLA loci except HLA-C or -DPA1 (66). Looking at T1D association more closely than at the level of the named allele, for example, at the amino acid level, may provide a broader perspective and generate hypotheses to test among studies of diverse populations. An illustrative example can be seen in two recent studies, one from Kuwait and one from Mali (mentioned above), that reported not only allele level but also amino-acid level analysis of T1D patients and controls for the DRB1, DQA1, and DQB1 loci (66, 108). These studies on two different populations, one Middle Eastern and one African Black, were of similar size, and both were performed with high-resolution sequencing and analyzed with the BIGDAWG software package (109). Figure 4A schematically describes the data observed for each population at the allele level (for those alleles with sufficient frequency to analyze, i.e., not in the “binned” category). Alleles that demonstrate significant T1D association are shown in black. Although they have some overlap, their distributions in these two populations appear different. Figure 4B represents the data at the level of individual amino acid residue encoding positions that appear T1D associated in the DRB1, DQA1, and DQB1 loci. These data appear more consistent between the countries, with a large proportion of positions showing consistency between the two data sets. One advantage of looking at this level is that it allows examination of all data, regardless of assigned allele names, obviating the need for binning infrequent alleles. Analysis at the amino acid level is likely to provide additional information about T1D risk that could be overlooked at the allele level and may provide a means to pool data from different populations of varying sizes, thereby increasing statistical power.
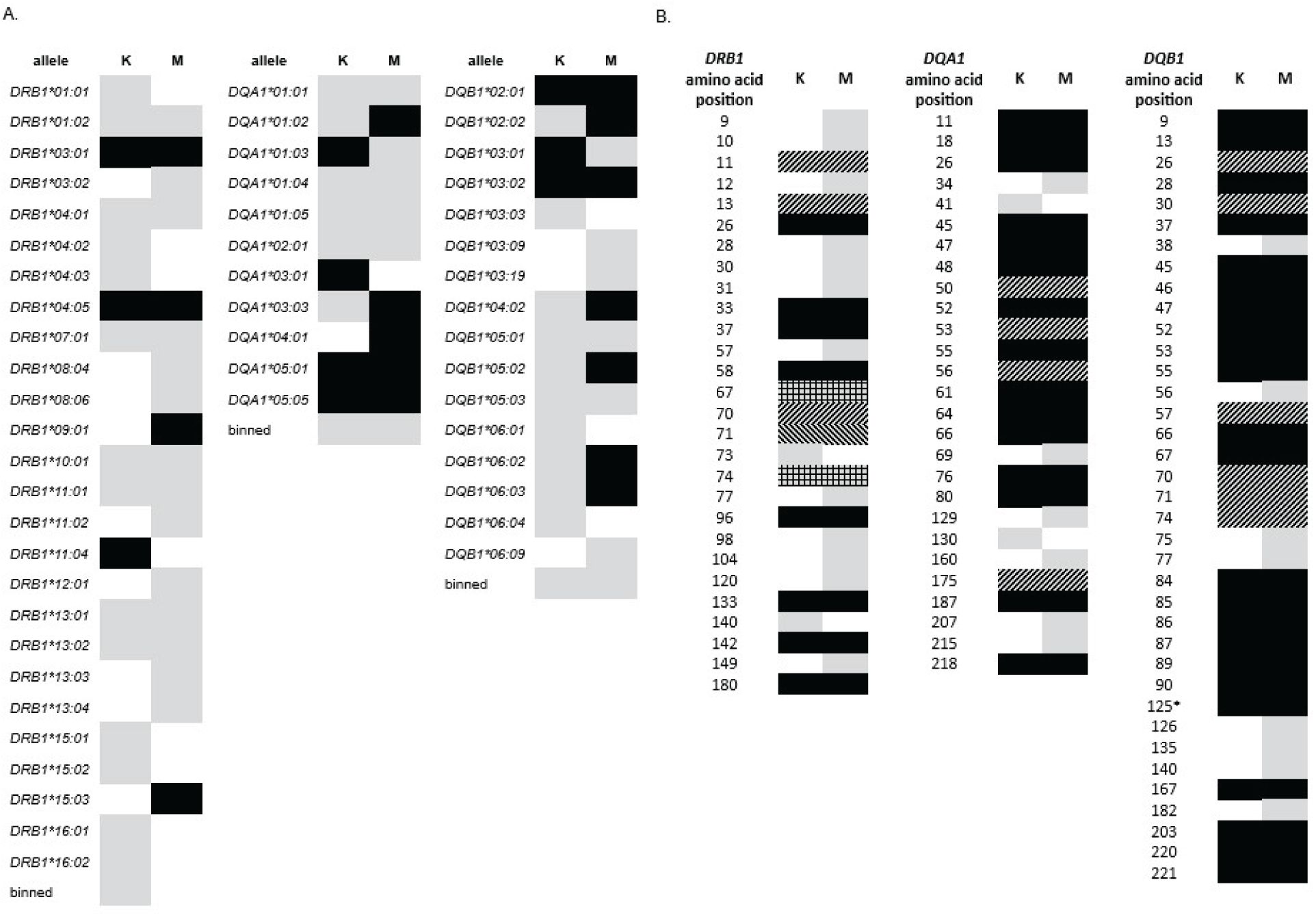
Figure 4. Comparison of data from HLA-DRB1, -DQA1, and -DQB1 association with T1D in Kuwait (K) and Mali (M) (108). (A) Allele level data. Gray: allele was present in the population at sufficiency frequency for association analysis. Black: allele was significantly associated with disease. “Binned” category contains all alleles that were present but at frequencies too low for individual association analysis. (B) Amino Acid position level data. Gray: positions with at least one amino acid residue that shows T1D association not seen in data for the other country. Black: positions in which the same amino acid residues with consistent directions of effect (e.g., predisposing or protective) are seen in both countries. With one exception, each of these positions represents two amino acids, one predisposing and one protective. *Position 125 of DQB1 has only one T1D-associated amino acid residue for each country, but the amino acid and effect direction are consistent. Diagonal lines: positions for which one country shows a greater number of associated amino acids than the other; in all cases, effect direction is consistent for amino acids that appear in both. All but one of these positions shows more associated amino acids for Mali that Kuwait. Only one position, DRB1 position 71, shows more associated amino acids in Kuwait than in Mali. Lines are shown in the opposite diagonal direction to indicate this difference. Crosshatch: positions for which the two countries share one significant amino acid association, but each has an additional, unique association. Notably, no individual amino acid had an effect that differed in direction between the two countries (i.e., predisposing in one dataset but protective in the other).
4.4 Strategies to mitigate the high cost of comprehensive HLA genotyping
Given the availability of highly informative DNA-sequencing technology and the knowledge that HLA genes are the most significant contributors to T1D risk, why are full, comprehensive HLA genotyping data available in so few reported studies? Genotyping cost is a major contributing factor. While the evolution of sequencing technology has enabled increasingly comprehensive and accurate genotyping with which to study T1D association, the cost of that genotyping is still quite prohibitive. The probability that full HLA genotyping, or full genome sequencing including HLA data, will soon become inexpensive enough to be applied to the general population, for example, in a newborn screening program, seems quite low. The cost of HLA genotyping has led to the development of techniques for imputation of HLA genotypes from single nucleotide polymorphism (SNP) data. An important consideration for imputed HLA data is that those data, while often correct, represent highly educated guesses and are not experimentally determined. Accuracy of those guesses is dependent on the input data used to train the imputation programs and on the assumptions made in the algorithms. Imputation results for the same data can vary depending on the method used for the analysis (110). However, SNP data are far less costly to obtain than direct HLA genotypes, and can have relatively good predictive power in well-characterized populations, particularly when combined with data for some of the more than ninety other known T1D associated genes (1). This strategy is the basis of development of the genetic risk score (GRS), sometimes referred to as polygenic risk score (PRS) (111–114). A perfect GRS test would allow the prediction of T1D as well as its differential diagnosis from T2D and other forms of diabetes. In individuals of European descent, the GRS performs well to predict T1D in the highest-risk individuals; however, the GRS is reported to perform less well as a diagnostic tool for other races and ethnicities (115–118). Ethnic and racial disparities for GRS or PRS is a general problem and not limited to T1D. Modifications of original GRS panels have improved performance across multiple races and ethnicities (119, 120). Recent reports still vary in conclusions about the utility of current tests, with one report suggesting that the GRS diagnostics are ready for widespread use in the United States while another finds the GRS of only modest predictive power in Australia (121, 122). Undoubtedly, continued refinement of the GRS will create a more universally applicable diagnostic test, but refinement is dependent on overcoming a second hurdle in the quest to fully understand the basis of HLA-associated T1D risk.
Frequencies of HLA alleles differ among ethnicities, as do the haplotype combinations of alleles see section 4.1). Achieving the highest predictive value for a genetic diagnostic test requires knowledge of HLA risk in all populations. Population-specific tests are one way to increase predictive power. However, not all individuals have a single ethnic origin. The United States is sometimes referred to as a “melting pot” with respect to ethnicity and race; consequently, many ethnicities and races are represented in the U.S. population, and many individuals are part of more than one group. Because of the low cost of SNP testing and the ability to multiplex large numbers of tests, an alternate means of increasing predictive value would be to add SNPs for known HLA-associated alleles from all populations into a single test. These SNPs would need to be determined from and vetted in unique populations, underscoring the value of continuing to study classical HLA in even small populations with low T1D prevalence.
5 Future directions in T1D risk assessment
5.1 HLA expression
Initial studies of HLA association with T1D reported data from serologic typing. The assays were based on using antibodies with known specificities, taken from multi-parous women and standardized among HLA typing laboratories. To see a positive typing result, a particular HLA was required to be present. The advent of DNA-based typing technology meant that measurement of the actual antigen was not required; the presence of a given HLA was inferred from the presence of the gene(s) encoding it. Since then, almost every HLA disease association study has been reported with DNA-based data. As new alleles were discovered, only some inferences about expression levels could be made. If an allele changed an encoded amino acid to a stop codon, it received the designation “N,” meaning no expression of the allele was expected. Very few studies have been reported on the actual expression level of HLA on the cell surface. With six classical HLA antigen types, multiple cell types on which they might be expressed, and the extreme number of alleles for each locus, expression studies are much more cumbersome and expensive to perform than are genotyping assays. Collecting cells from patients for functional studies is much more difficult than collecting DNA to genotype.
DNA-based HLA genotyping determines which alleles are present in an individual sample but, except in rare case (e.g., alleles containing a stop codon), provides no information about differences among alleles at either the transcription or translation level of expression. A limited number of studies have been performed to date attempting to unravel the role of cell-surface expression levels on disease risk. In one such study, a cell surface expression assay was engineered to determine amounts of cell-surface expression as a proxy for the stability of the DQ heterodimeric antigen (54). The authors concluded that T1D association for DQ antigens is inversely correlated with the level of cell-surface protein density, including for heterodimeric proteins encoded in trans, such as that formed by the products of DQA1*05:01 and DQB1*03:02 alleles present in a typical high-risk European DR3/DR4 genotype (Figure 2A) (53, 54). Given the extent of polymorphism of HLA alleles, testing of every observed HLA antigen is clearly not feasible, but expression testing of select antigens of interest, particularly those HLA class II antigens encoded in trans, should be possible.
Now that HLA genotyping technology has improved to the point that whole genes, and even the entire HLA region, can be sequenced with relative ease, the possibility of inferring expression from sequence data has increased. One example of the utility of HLA expression data can be found in the field of transplantation. A SNP in the 3’ untranslated region of DPB1, rs9277534, was found to correlate with low versus high expression levels of DPB1 expression, and high expression of DP is known to be associated with graft versus host disease (123). More recently, LD of that SNP with DPB1 exon 2 sequence was shown to be adequate for prediction of the SNP without direct SNP testing, thereby aiding in transplant donor selection (124). The field of HLA expression and its relationship with disease risk is still in early stages; however, a haplotype of three SNPs in a short region of exon 1 of the DRA gene was shown to be associated with T1D in individuals homozygous for the DRB1*03:01~DQA1*05:01~DQB1*02:01 haplotype (125). Based on eQTL evidence, one proposed mechanism for the effect of this “tri-SNP” haplotype is that it changes, directly or indirectly, expression levels of HLA class II loci. Now that sequencing an entire HLA locus, including untranslated sequences, is becoming routine, non-coding sequences can lead to hypotheses that can be tested in direct functional analyses.
5.2 HLA in innate immunity
Studies of T1D to date have focused mainly on the classic role of HLA in presenting peptides to the adaptive immune system. Clearly, HLA plays a major role in initiating the immune response in T1D (class II) and in driving progression of disease via β cell destruction (class I). However, individual allele association studies have not explained all of the risk conferred by the HLA region. The innate immune system also appears to play a role in T1D pathogenesis. Many reports have implicated viruses, inflammation, and interferons as predecessors of disease onset (126–129). Natural killer (NK) cells are key players in the innate immune system and express many activating and inhibitory receptors on their surface, including the widely studied killer-cell inhibitory receptors (KIR) (130, 131). KIR can be either inflammatory or stimulatory depending upon the receptor structure. Loci encoding KIR are found in the leukocyte receptor complex (LRC) at chromosomal position 19q3.4. Like HLA, KIR genes are polymorphic; unlike HLA, much of the polymorphism is attributable to the presence or absence of given genes and their variegated expression, that is, finding a KIR-encoding locus in an individual does not necessarily indicate that its product will be expressed on the cell surface. HLA class I molecules act as ligands for KIR (132). Over the past 20 years, a limited number of studies in several populations have reported attempts to elucidate an association for KIR with T1D (133–156). Not surprisingly, results have been largely inconsistent. A KIR effect would stem from the interaction of a particular KIR with its cognate ligand, requiring that both receptor and ligand be expressed in the same individual, confounding analysis of either molecule alone. Awareness of the extent of KIR polymorphism is in early stages; KIR genotyping studies have evolved from simple “presence or absence” genotyping and copy number variation to genotyping of a growing number of alleles for each locus. Thus, examination of effects of interaction of products of multiple highly polymorphic loci is likely to require extremely large data sets.
LILR genes are also located in the LRC and are largely conserved among primates (157, 158). They have structural similarity to KIR and, like KIR, function to maintain immune homeostasis. LILR are primarily expressed in cells of the myeloid lineage, and their ligands include class I HLA. LILR are reported to be important in infection, transplantation, cancer, and autoimmune diseases, including systemic lupus erythematosus (SLE), ankylosing spondylitis, Crohn’s disease, rheumatoid arthritis (RA), and multiple sclerosis (MS) (157, 159–161). T1D is noticeably absent from this list, and a PubMed search for T1D and LILR returns no results. As sample sets increase and analysis strategies evolve, LILR will undoubtedly be examined for T1D association, perhaps focusing on patients who do not have traditional high-risk HLA alleles.
HLA-C association with T1D is weaker than for HLA-A and HLA-B and is inconsistent among studies. The lack of consistency in the results of HLA-C association analyses with T1D may reflect that the locus is not associated with T1D, and any individual observations of disease association may be spurious or may be attributable to LD with other loci that are T1D associated. An alternate explanation may be that HLA-C influences T1D susceptibility through a mechanism involving the innate immune system, where HLA-C antigens serve as ligands for KIR. A recent study in subjects from Mali demonstrated no locus association with T1D for HLA-C at the allele level; however, analysis at the amino acid level revealed polymorphic amino acid positions that appear to be associated with T1D. One of these was position 309, which is in the transmembrane region and might not be expected to affect T1D risk. However, in a series of transfection-based experiments, the presence of a cysteine at position 309 of HLA-C was shown to inhibit the cytotoxicity of NK cells, suggesting a role for HLA-C in innate immunity (162). The mechanism of such an effect, which might include influencing folding or stability of the protein or its motion in the membrane, remains to be determined.
Association of genes encoding the HLA class II DP antigen also tends to vary widely among studies and among populations (see section 3.5). Recent reports demonstrate that, in addition to classical antigen presenting functions, a subset of HLA-DP molecules can serve as ligands for NKp44, a receptor found on NK cells that can activate them upon ligand binding (16, 163). The interaction of DPB1 with NKp44 has been reported as a risk factor for both primary sclerosing cholangitis, an immune-mediated liver disease, and ulcerative colitis, a form of inflammatory bowel disease (164, 165). Given its apparent role in other immune-mediated diseases, binding of NKp44 to DPB represents a reasonable hypothesis to test for T1D association as well.
6 Discussion
More than 50 years have elapsed since the first reports of HLA association with T1D. Those original positive associations of A8 and B15 (now recognized as representing LD with class II DR3 and DR4 haplotypes), negative association of Dw2 (now recognized as DRB1*15 haplotypes), and particularly strong positive association for the Dw3/Dw4 heterozygous genotype, remain valid. They represent, in many cases, the primary contribution of HLA to T1D risk. Does that mean that 50 years of research and more than 5,000 studies were wasted? Not really. Many lessons have been learned from those studies, including (1) HLA-associated alleles should not be considered in isolation but should be analyzed in haplotypic and genotypic context as well; (2) genotyping should be performed minimally at two field resolution, since alleles within a serogroup can have opposite effects on T1D risk; (3) T1D protection is dominant, suggesting that the mechanisms for susceptibility and protection are different; (4) T1D-associated HLA risk can be quite different in different populations, even when they are geographically proximal, due to differences in population allele frequencies and haplotypic combinations. Analysis at the allele level has not yet been sufficient to fully understand how HLA-associated T1D risk works. Full elucidation may require different and more detailed analyses, for example, of gene features and individual amino acid positions, may require very large sample sets, and may require sophisticated techniques to combine old and new data as well as account for population stratification. Still, those original associations with DR3, DR4, DR2 (protection), and the DR3/DR4 genotype remain.
The availability of highly sophisticated genotyping techniques has increased the ability to see exactly what is in the genome of an individual but decreased the ability to determine whether or not the encoded antigens are actually expressed on the surface of the cell. What drives the expression of an allele, on which cells it is expressed, and at what level is it expressed are questions that are just beginning to be addressed. The full extent of the peptide repertoire, both native and hybrid, is currently under investigation and should provide additional clues to the many possible pathways to autoimmunity in patients, perhaps leading to more individualized interventions. Continued refinement of predictive tools, like the GRS, should help identify future patients before overt disease to aid in the efficacy of current (teplizumab) and future intervention strategies.
Adaptive immunity provided by the classical HLA is undoubtedly the major basis for HLA-associated T1D susceptibility; however, the interaction of HLA with the innate immune system represents an intriguing and exciting path to study going forward. Given the solid base of understanding to date and the tools available for continuing study, a thorough and complete understanding of the complexity of HLA-associated T1D risk is unlikely to take another 50 years.
Author contributions
JN: Conceptualization, Data curation, Writing – original draft, Writing - review & editing.
Funding
The author(s) declare that no financial support was received for the research, authorship, and/or publication of this article.
Conflict of interest
The author declares that the research was conducted in the absence of any commercial or financial relationships that could be construed as a potential conflict of interest.
Publisher’s note
All claims expressed in this article are solely those of the authors and do not necessarily represent those of their affiliated organizations, or those of the publisher, the editors and the reviewers. Any product that may be evaluated in this article, or claim that may be made by its manufacturer, is not guaranteed or endorsed by the publisher.
Author disclaimer
No artificial intelligence systems were applied in the creation of the manuscript.
Abbreviations
HLA, Human leukocyte antigen; LD, Linkage disequilibrium; T1D, Type 1 diabetes; KIR, Killer-cell immunoglobulin-like receptors; LILR, Leukocyte Immunoglobulin-like receptor.
References
1. Redondo MJ, Onengut-Gumuscu S, Gaulton KJ. Genetics of Type 1 Diabetes. Lawrence JM, Casagrande SS, Herman WH, Wexler DJ, Cefalu WT, editors. eds. Bethesda: Diabetes in America (2023).
2. Noble JA. Immunogenetics of type 1 diabetes: A comprehensive review. J Autoimmun. (2015) 64:101–12. doi: 10.1016/j.jaut.2015.07.014
3. Singal DP, Blajchman MA. Histocompatibility (HL-A) antigens, lymphocytotoxic antibodies and tissue antibodies in patients with diabetes mellitus. Diabetes. (1973) 22:429–32. doi: 10.2337/diab.22.6.429
4. Finkelstein S, Zeller E, Walford RL. No relation between HL-A and juvenile diabetes. Tissue Antigens. (1972) 2:74–7. doi: 10.1111/j.1399-0039.1972.tb00120.x
5. Marx V. Method of the year: long-read sequencing. Nat Methods Jan. (2023) 20:6–11. doi: 10.1038/s41592-022-01730-w
6. Ashraf MT, Ahmed Rizvi SH, Kashif MAB, Shakeel Khan MK, Ahmed SH, Asghar MS. Efficacy of anti-CD3 monoclonal antibodies in delaying the progression of recent-onset type 1 diabetes mellitus: A systematic review, meta-analyses and meta-regression. Diabetes Obes Metab Nov. (2023) 25:3377–89. doi: 10.1111/dom.15237
7. Hirsch JS. FDA approves teplizumab: a milestone in type 1 diabetes. Lancet Diabetes Endocrinol. (2023) 11:18. doi: 10.1016/S2213-8587(22)00351-5
8. Misra S, Shukla AK. Teplizumab: type 1 diabetes mellitus preventable? Eur J Clin Pharmacol. (2023) 79:609–16. doi: 10.1007/s00228-023-03474-8
9. Sharma N, Das DD, Chawla PA. Journey of teplizumab: A promising drug in the treatment of type 1 diabetes mellitus. Curr Diabetes Rev. (2024). doi: 10.2174/0115733998261825231026060241
10. Barker DJ, Maccari G, Georgiou X, Cooper MA, Flicek P, Robinson J, et al. The IPD-IMGT/HLA database. Nucleic Acids Res. (2023) 51:D1053–60. doi: 10.1093/nar/gkac1011
11. Zhou Y, Song L, Li H. Full resolution HLA and KIR genes annotation for human genome assemblies. bioRxiv. (2024). doi: 10.1101/2024.01.20.576452
12. Marsh SG, Albert ED, Bodmer WF, Bontrop RE, Dupont B, Erlich HA, et al. Nomenclature for factors of the HLA system, 2010. Tissue Antigens. (2010) 75:291–455. doi: 10.1111/j.1399-0039.2010.01466.x
13. Hurley CK. Naming HLA diversity: A review of HLA nomenclature. Hum Immunol. (2021) 82:457–65. doi: 10.1016/j.humimm.2020.03.005
14. Osoegawa K, Montero-Martin G, Mallempati KC, Bauer M, Milius RP, Maiers M, et al. Challenges for the standardized reporting of NGS HLA genotyping: Surveying gaps between clinical and research laboratories. Hum Immunol Nov. (2021) 82:820–8. doi: 10.1016/j.humimm.2021.08.011
15. Varney MD, Valdes AM, Carlson JA, Noble JA, Tait BD, Bonella P, et al. HLA DPA1, DPB1 alleles and haplotypes contribute to the risk associated with type 1 diabetes: analysis of the type 1 diabetes genetics consortium families. Diabetes. Aug. (2010) 59:2055–62. doi: 10.2337/db09-0680
16. Niehrs A, Garcia-Beltran WF, Norman PJ, Watson GM, Holzemer A, Chapel A, et al. A subset of HLA-DP molecules serve as ligands for the natural cytotoxicity receptor NKp44. Nat Immunol Sep. (2019) 20:1129–37. doi: 10.1038/s41590-019-0448-4
17. Padoan B, Casar C, Krause J, Schultheiss C, Baumdick ME, Niehrs A, et al. NKp44/HLA-DP-dependent regulation of CD8 effector T cells by NK cells. Cell Rep. (2024) 43:114089. doi: 10.1016/j.celrep.2024.114089
18. Gragert L, Madbouly A, Freeman J, Maiers M. Six-locus high resolution HLA haplotype frequencies derived from mixed-resolution DNA typing for the entire US donor registry. Hum Immunol. (2013) 74:1313–20. doi: 10.1016/j.humimm.2013.06.025
19. Cudworth AG, Woodrow JC. Letter: HL-A antigens and diabetes mellitus. Lancet. (1974) 2:1153. doi: 10.1016/s0140-6736(74)90930-1
20. Nerup J, Platz P, Andersen OO, Christy M, Lyngsoe J, Poulsen JE, et al. HL-A antigens and diabetes mellitus. Lancet. (1974) 2:864–6. doi: 10.1016/s0140-6736(74)91201-x
21. Barbosa J, Chern MM, Anderson VE, Noreen H, Johnson S, Reinsmoen N, et al. Linkage analysis between the major histocompatibility system and insulin-dependent diabetes in families with patients in two consecutive generations. J Clin Invest. Mar. (1980) 65:592–601. doi: 10.1172/JCI109704
22. Bilbao JR, Calvo B, Aransay AM, Martin-Pagola A, Perez de Nanclares G, Aly TA, et al. Conserved extended haplotypes discriminate HLA-DR3-homozygous Basque patients with type 1 diabetes mellitus and celiac disease. Genes Immun. (2006) 7:550–4. doi: 10.1038/sj.gene.6364328
23. Robinson WP, Barbosa J, Rich SS, Thomson G. Homozygous parent affected sib pair method for detecting disease predisposing variants: application to insulin dependent diabetes mellitus. Genet Epidemiol. (1993) 10:273–88. doi: 10.1002/gepi.1370100502
24. Thomson G, Robinson WP, Kuhner MK, Joe S, MacDonald MJ, Gottschall JL, et al. Genetic heterogeneity, modes of inheritance, and risk estimates for a joint study of Caucasians with insulin-dependent diabetes mellitus. Am J Hum Genet Dec. (1988) 43:799–816.
25. Baschal EE, Aly TA, Jasinski JM, Steck AK, Johnson KN, Noble JA, et al. The frequent and conserved DR3-B8-A1 extended haplotype confers less diabetes risk than other DR3 haplotypes. Diabetes Obes Metab. (2009) 11 Suppl 1:25–30. doi: 10.1111/j.1463-1326.2008.01000.x
26. Noble JA, Martin A, Valdes AM, Lane JA, Galgani A, Petrone A, et al. Type 1 diabetes risk for human leukocyte antigen (HLA)-DR3 haplotypes depends on genotypic context: association of DPB1 and HLA class I loci among DR3- and DR4-matched Italian patients and controls. Hum Immunol. (2008) 69:291–300. doi: 10.1016/j.humimm.2008.02.003
27. Hawkins BR, Lam KS, Ma JT, Low LC, Cheung PT, Serjeantson SW, et al. Strong association of HLA-DR3/DRw9 heterozygosity with early-onset insulin-dependent diabetes mellitus in Chinese. Diabetes. (1987) 36:1297–300. doi: 10.2337/diab.36.11.1297
28. Kumar N, Kaur G, Tandon N, Kanga U, Mehra NK. Genomic evaluation of HLA-DR3+ haplotypes associated with type 1 diabetes. Ann N Y Acad Sci. (2013) 1283:91–6. doi: 10.1111/nyas.12019
29. Erlich H, Valdes AM, Noble J, Carlson JA, Varney M, Concannon P, et al. HLA DR-DQ haplotypes and genotypes and type 1 diabetes risk: analysis of the type 1 diabetes genetics consortium families. Diabetes. (2008) 57:1084–92. doi: 10.2337/db07-1331
30. Noble JA, Valdes AM, Cook M, Klitz W, Thomson G, Erlich HA. The role of HLA class II genes in insulin-dependent diabetes mellitus: molecular analysis of 180 Caucasian, multiplex families. Am J Hum Genet. (1996) 59:1134–48.
31. McGrail C, Chiou J, Elgamal R, Luckett AM, Oram RA, Benaglio P, et al. Genetic discovery and risk prediction for type 1 diabetes in individuals without high-risk HLA-DR3/DR4 haplotypes. medRxiv. (2023). doi: 10.1101/2023.11.11.23298405
32. Mirel DB, Valdes AM, Lazzeroni LC, Reynolds RL, Erlich HA, Noble JA. Association of IL4R haplotypes with type 1 diabetes. Diabetes. (2002) 51:3336–41. doi: 10.2337/diabetes.51.11.3336
33. Noble JA, Valdes AM, Bugawan TL, Apple RJ, Thomson G, Erlich HA. The HLA class I A locus affects susceptibility to type 1 diabetes. Hum Immunol. (2002) 63:657–64. doi: 10.1016/s0198-8859(02)00421-4
34. Noble JA, Valdes AM, Thomson G, Erlich HA. The HLA class II locus DPB1 can influence susceptibility to type 1 diabetes. Diabetes. (2000) 49:121–5. doi: 10.2337/diabetes.49.1.121
35. Noble JA, White AM, Lazzeroni LC, Valdes AM, Mirel DB, Reynolds R, et al. A polymorphism in the TCF7 gene, C883A, is associated with type 1 diabetes. Diabetes. (2003) 52:1579–82. doi: 10.2337/diabetes.52.6.1579
36. Noble JA, Johnson J, Lane JA, Valdes AM. HLA class II genotyping of African American type 1 diabetic patients reveals associations unique to African haplotypes. Diabetes. (2013) 62:3292–9. doi: 10.2337/db13-0094
37. Chow IT, Gates TJ, Papadopoulos GK, Moustakas AK, Kolawole EM, Notturno RJ, et al. Discriminative T cell recognition of cross-reactive islet-antigens is associated with HLA-DQ8 transdimer-mediated autoimmune diabetes. Sci Adv. (2019) 5:eaaw9336. doi: 10.1126/sciadv.aaw9336
38. Thomson G, Valdes AM, Noble JA, Kockum I, Grote MN, Najman J, et al. Relative predispositional effects of HLA class II DRB1-DQB1 haplotypes and genotypes on type 1 diabetes: a meta-analysis. Tissue Antigens. (2007) 70:110–27. doi: 10.1111/j.1399-0039.2007.00867.x
39. Thomson G. HLA DR antigens and susceptibility to insulin-dependent diabetes mellitus. Am J Hum Genet. (1984) 36:1309–17.
40. Mychaleckyj JC, Noble JA, Moonsamy PV, Carlson JA, Varney MD, Post J, et al. HLA genotyping in the international Type 1 Diabetes Genetics Consortium. Clin Trials. (2010) 7:S75–87. doi: 10.1177/1740774510373494
41. Rich SS, Akolkar B, Concannon P, Erlich H, Hilner JE, Julier C, et al. Overview of the type I diabetes genetics consortium. Genes Immun Dec. (2009) 10 Suppl 1:S1–4. doi: 10.1038/gene.2009.84
42. Noble JA, Valdes AM, Varney MD, Carlson JA, Moonsamy P, Fear AL, et al. HLA class I and genetic susceptibility to type 1 diabetes: results from the Type 1 Diabetes Genetics Consortium. Diabetes. (2010) 59:2972–9. doi: 10.2337/db10-0699
43. Sang Y, Yan C, Zhu C, Ni G. Relationship between HLA-DRB1 and DQ alleles and the genetic susceptibility to type 1 diabetes. Chin Med J (Engl). (2001) 114:407–9.
44. Kawa A, Nakazawa M, Sakaguchi S, Nakamura S, Kono Y. HLA system in Japnaese patients with diabetes mellitus. Diabetes. (1977) 26:591–5. doi: 10.2337/diab.26.6.591
45. Ikegami H, Kawaguchi Y, Yamato E, Kuwata S, Tokunaga K, Noma Y, et al. Analysis by the polymerase chain reaction of histocompatibility leucocyte antigen-DR9-linked susceptibility to insulin-dependent diabetes mellitus. J Clin Endocrinol Metab. (1992) 75:1381–5. doi: 10.1210/jcem.75.5.1358911
46. Ronningen KS, Gjertsen HA, Iwe T, Spurkland A, Hansen T, Thorsby E. Particular HLA-DQ alpha beta heterodimer associated with IDDM susceptibility in both DR4-DQw4 Japanese and DR4-DQw8/DRw8-DQw4 whites. Diabetes. (1991) 40:759–63. doi: 10.2337/diab.40.6.759
47. Ahmadov GA, Govender D, Atkinson MA, Sultanova RA, Eubova AA, Wasserfall CH, et al. Epidemiology of childhood-onset type 1 diabetes in Azerbaijan: Incidence, clinical features, biochemistry, and HLA-DRB1 status. Diabetes Res Clin Pract. (2018) 144:252–9. doi: 10.1016/j.diabres.2018.09.009
48. Noble JA, Erlich HA. Genetics of type 1 diabetes. Cold Spring Harb Perspect Med. (2012) 2:a007732. doi: 10.1101/cshperspect.a007732
49. Horn GT, Bugawan TL, Long CM, Manos MM, Erlich HA. Sequence analysis of HLA class II genes from insulin-dependent diabetic individuals. Hum Immunol Apr. (1988) 21:249–63. doi: 10.1016/0198-8859(88)90034-1
50. Todd JA, Bell JI, McDevitt HO. HLA-DQ beta gene contributes to susceptibility and resistance to insulin-dependent diabetes mellitus. Nature. (1987) 329:599–604. doi: 10.1038/329599a0
51. van Lummel M, Buis DTP, Ringeling C, de Ru AH, Pool J, Papadopoulos GK, et al. Epitope stealing as a mechanism of dominant protection by HLA-DQ6 in type 1 diabetes. Diabetes. (2019) 68:787–95. doi: 10.2337/db18-0501
52. Simmons KM, Mitchell AM, Alkanani AA, McDaniel KA, Baschal EE, Armstrong T, et al. Failed genetic protection: type 1 diabetes in the presence of HLA-DQB1*06:02. Diabetes. (2020) 69:1763–9. doi: 10.2337/db20-0038
53. Miyadera H, Tokunaga K. Associations of human leukocyte antigens with autoimmune diseases: challenges in identifying the mechanism. J Hum Genet. (2015) 60:697–702. doi: 10.1038/jhg.2015.100
54. Miyadera H, Ohashi J, Lernmark A, Kitamura T, Tokunaga K. Cell-surface MHC density profiling reveals instability of autoimmunity-associated HLA. J Clin Invest. (2015) 125:275–91. doi: 10.1172/JCI74961
55. Ettinger RA, Liu AW, Nepom GT, Kwok WW. Exceptional stability of the HLA-DQA1*0102/DQB1*0602 alpha beta protein dimer, the class II MHC molecule associated with protection from insulin-dependent diabetes mellitus. J Immunol. (1998) 161:6439–45. doi: 10.4049/jimmunol.161.11.6439
56. Ettinger RA, Liu AW, Nepom GT, Kwok WW. Beta 57-Asp plays an essential role in the unique SDS stability of HLA-DQA1*0102/DQB1*0602 alpha beta protein dimer, the class II MHC allele associated with protection from insulin-dependent diabetes mellitus. J Immunol. (2000) 165:3232–8. doi: 10.4049/jimmunol.165.6.3232
57. Pugliese A, Boulware D, Yu L, Babu S, Steck AK, Becker D, et al. HLA-DRB1*15:01-DQA1*01:02-DQB1*06:02 haplotype protects autoantibody-positive relatives from type 1 diabetes throughout the stages of disease progression. Diabetes. (2016) 65:1109–19. doi: 10.2337/db15-1105
58. Mijovic CH, Barnett AH, Todd JA. Genetics of diabetes. Trans-racial gene mapping studies. Baillieres Clin Endocrinol Metab. (1991) 5:321–40. doi: 10.1016/s0950-351x(05)80130-2
59. Noble JA, Johnson J, Lane JA, Valdes AM. Race-specific type 1 diabetes risk of HLA-DR7 haplotypes. Tissue Antigens. (2011) 78:348–51. doi: 10.1111/j.1399-0039.2011.01772.x
60. Baschal EE, Aly TA, Babu SR, Fernando MS, Yu L, Miao D, et al. HLA-DPB1*0402 protects against type 1A diabetes autoimmunity in the highest risk DR3-DQB1*0201/DR4-DQB1*0302 DAISY population. Diabetes. (2007) 56:2405–9. doi: 10.2337/db07-0029
61. Cruz TD, Valdes AM, Santiago A, Frazer de Llado T, Raffel LJ, Zeidler A, et al. DPB1 alleles are associated with type 1 diabetes susceptibility in multiple ethnic groups. Diabetes. (2004) 53:2158–63. doi: 10.2337/diabetes.53.8.2158
62. Cucca F, Dudbridge F, Loddo M, Mulargia AP, Lampis R, Angius E, et al. The HLA-DPB1–associated component of the IDDM1 and its relationship to the major loci HLA-DQB1, -DQA1, and -DRB1. Diabetes. (2001) 50:1200–5. doi: 10.2337/diabetes.50.5.1200
63. Erlich HA, Rotter JI, Chang JD, Shaw SJ, Raffel LJ, Klitz W, et al. Association of HLA-DPB1*0301 with IDDM in mexican-americans. Diabetes. (1996) 45:610–4. doi: 10.2337/diab.45.5.610
64. Lie BA, Akselsen HE, Joner G, Dahl-Jorgensen K, Ronningen KS, Thorsby E, et al. HLA associations in insulin-dependent diabetes mellitus: no independent association to particular DP genes. Hum Immunol. (1997) 55:170–5. doi: 10.1016/s0198-8859(97)00095-5
65. Balducci-Silano PL, Layrisse ZE. HLA-DP and susceptibility to insulin-dependent diabetes mellitus in an ethnically mixed population. Associations with other HLA-alleles. J Autoimmun. (1995) 8:425–37. doi: 10.1006/jaut.1995.0034
66. Noble JA, Besancon S, Sidibe AT, Rozemuller EH, Rijkers M, Dadkhodaie F, et al. Complete HLA genotyping of type 1 diabetes patients and controls from Mali reveals both expected and novel disease associations. HLA. (2024) 103:e15319. doi: 10.1111/tan.15319
67. Valdes AM, Erlich HA, Noble JA. Human leukocyte antigen class I B and C loci contribute to Type 1 Diabetes (T1D) susceptibility and age at T1D onset. Hum Immunol. (2005) 66:301–13. doi: 10.1016/j.humimm.2004.12.001
68. Howson JM, Walker NM, Clayton D, Todd JA, Type 1 Diabetes Genetics C. Confirmation of HLA class II independent type 1 diabetes associations in the major histocompatibility complex including HLA-B and HLA-A. Diabetes Obes Metab Feb. (2009) 11 Suppl 1:31–45. doi: 10.1111/j.1463-1326.2008.01001.x
69. Nejentsev S, Howson JM, Walker NM, Szeszko J, Field SF, Stevens HE, et al. Localization of type 1 diabetes susceptibility to the MHC class I genes HLA-B and HLA-A. Nature. (2007) 450:887–92. doi: 10.1038/nature06406
70. Yeo L, Pujol-Autonell I, Baptista R, Eichmann M, Kronenberg-Versteeg D, Heck S, et al. Circulating beta cell-specific CD8(+) T cells restricted by high-risk HLA class I molecules show antigen experience in children with and at risk of type 1 diabetes. Clin Exp Immunol. (2020) 199:263–77. doi: 10.1111/cei.13391
71. Nakanishi K, Kobayashi T, Murase T, Nakatsuji T, Inoko H, Tsuji K, et al. Association of HLA-A24 with complete beta-cell destruction in IDDM. Diabetes. (1993) 42:1086–93. doi: 10.2337/diab.42.7.1086
72. Nakanishi K, Kobayashi T, Murase T, Naruse T, Nose Y, Inoko H. Human leukocyte antigen-A24 and -DQA1*0301 in Japanese insulin-dependent diabetes mellitus: independent contributions to susceptibility to the disease and additive contributions to acceleration of beta-cell destruction. J Clin Endocrinol Metab. (1999) 84:3721–5. doi: 10.1210/jcem.84.10.6045
73. Kobayashi T, Tamemoto K, Nakanishi K, Kato N, Okubo M, Kajio H, et al. Immunogenetic and clinical characterization of slowly progressive IDDM. Diabetes Care. (1993) 16:780–8. doi: 10.2337/diacare.16.5.780
74. Ye J, Long AE, Pearson JA, Taylor H, Bingley PJ, Williams AJ, et al. Attenuated humoral responses in HLA-A*24-positive individuals at risk of type 1 diabetes. Diabetologia. (2015) 58:2284–7. doi: 10.1007/s00125-015-3702-9
75. Mikk ML, Heikkinen T, El-Amir MI, Kiviniemi M, Laine AP, Harkonen T, et al. The association of the HLA-A*24:02, B*39:01 and B*39:06 alleles with type 1 diabetes is restricted to specific HLA-DR/DQ haplotypes in Finns. HLA. (2017) 89:215–24. doi: 10.1111/tan.12967
76. Schloss J, Ali R, Racine JJ, Chapman HD, Serreze DV, DiLorenzo TP. HLA-B*39:06 efficiently mediates type 1 diabetes in a mouse model incorporating reduced thymic insulin expression. J Immunol. (2018) 200:3353–63. doi: 10.4049/jimmunol.1701652
77. Omar MA, Hammond MG, Asmal AC. HLA-A, B, C and DR antigens in young South African blacks with Type 1 (insulin-dependent) diabetes mellitus. Diabetologia. (1984) 26:20–3. doi: 10.1007/BF00252257
78. Alshiekh S, Maziarz M, Geraghty DE, Larsson HE, Agardh D. High-resolution genotyping indicates that children with type 1 diabetes and celiac disease share three HLA class II loci in DRB3, DRB4 and DRB5 genes. HLA. (2021) 97:44–51. doi: 10.1111/tan.14105
79. Erlich HA, Valdes AM, McDevitt SL, Simen BB, Blake LA, McGowan KR, et al. Next generation sequencing reveals the association of DRB3*02:02 with type 1 diabetes. Diabetes. (2013) 62:2618–22. doi: 10.2337/db12-1387
80. Zhao LP, Alshiekh S, Zhao M, Carlsson A, Larsson HE, Forsander G, et al. Next-generation sequencing reveals that HLA-DRB3, -DRB4, and -DRB5 may be associated with islet autoantibodies and risk for childhood type 1 diabetes. Diabetes. (2016) 65:710–8. doi: 10.2337/db15-1115
81. Zhao LP, Papadopoulos GK, Kwok WW, Xu B, Kong M, Moustakas AK, et al. Eleven amino acids of HLA-DRB1 and fifteen amino acids of HLA-DRB3, 4, and 5 include potentially causal residues responsible for the risk of childhood type 1 diabetes. Diabetes. (2019) 68:1692–704. doi: 10.2337/db19-0273
82. Zabeen B, Govender D, Hassan Z, Noble JA, Lane JA, Mack SJ, et al. Clinical features, biochemistry and HLA-DRB1 status in children and adolescents with diabetes in Dhaka, Bangladesh. Diabetes Res Clin Pract. (2019) 158:107894. doi: 10.1016/j.diabres.2019.107894
83. Fawwad A, Govender D, Ahmedani MY, Basit A, Lane JA, Mack SJ, et al. Clinical features, biochemistry and HLA-DRB1 status in youth-onset type 1 diabetes in Pakistan. Diabetes Res Clin Pract. (2019) 149:9–17. doi: 10.1016/j.diabres.2019.01.023
84. Sunni M, Noble JA, Yu L, Mahamed Z, Lane JA, Dhunkal AM, et al. Predominance of DR3 in Somali children with type 1 diabetes in the twin cities, Minnesota. Pediatr Diabetes. (2017) 18:136–42. doi: 10.1111/pedi.12369
86. Lin L, Houwink API, van Dieren JM, Wolthuis EK, van Thienen JV, van der Heijden MS, et al. Treatment patterns and survival outcomes of patients admitted to the intensive care unit due to immune-related adverse events of immune checkpoint inhibitors. Cancer Med. (2024) 13:e7302. doi: 10.1002/cam4.7302
87. Beran D, Hojlund K, Besancon S, Mundt ML, Ogle GD, Ramaiya K, et al. A plan to improve global type 1 diabetes epidemiology data. Lancet Diabetes Endocrinol. (2023) 11:154–5. doi: 10.1016/S2213-8587(23)00029-3
88. Leete P, Oram RA, McDonald TJ, Shields BM, Ziller C, Team Ts, et al. Studies of insulin and proinsulin in pancreas and serum support the existence of aetiopathological endotypes of type 1 diabetes associated with age at diagnosis. Diabetologia. (2020) 63:1258–67. doi: 10.1007/s00125-020-05115-6
89. Battaglia M, Ahmed S, Anderson MS, Atkinson MA, Becker D, Bingley PJ, et al. Introducing the endotype concept to address the challenge of disease heterogeneity in type 1 diabetes. Diabetes Care. (2020) 43:5–12. doi: 10.2337/dc19-0880
90. Krischer JP, Lynch KF, Schatz DA, Ilonen J, Lernmark A, Hagopian WA, et al. The 6 year incidence of diabetes-associated autoantibodies in genetically at-risk children: the TEDDY study. Diabetologia. (2015) 58:980–7. doi: 10.1007/s00125-015-3514-y
91. Mohan JF, Petzold SJ, Unanue ER. Register shifting of an insulin peptide-MHC complex allows diabetogenic T cells to escape thymic deletion. J Exp Med. (2011) 208:2375–83. doi: 10.1084/jem.20111502
92. Crawford F, Stadinski B, Jin N, Michels A, Nakayama M, Pratt P, et al. Specificity and detection of insulin-reactive CD4+ T cells in type 1 diabetes in the nonobese diabetic (NOD) mouse. Proc Natl Acad Sci U S A. (2011) 108:16729–34. doi: 10.1073/pnas.1113954108
93. Arribas-Layton D, Guyer P, Delong T, Dang M, Chow IT, Speake C, et al. Hybrid insulin peptides are recognized by human T cells in the context of DRB1*04:01. Diabetes. (2020) 69:1492–502. doi: 10.2337/db19-0620
94. Babon JA, DeNicola ME, Blodgett DM, Crevecoeur I, Buttrick TS, Maehr R, et al. Analysis of self-antigen specificity of islet-infiltrating T cells from human donors with type 1 diabetes. Nat Med. (2016) 22:1482–7. doi: 10.1038/nm.4203
95. Baker RL, Rihanek M, Hohenstein AC, Nakayama M, Michels A, Gottlieb PA, et al. Hybrid insulin peptides are autoantigens in type 1 diabetes. Diabetes. (2019) 68:1830–40. doi: 10.2337/db19-0128
96. Callebaut A, Guyer P, Baker RL, Gallegos JB, Hohenstein AC, Gottlieb PA, et al. An Insulin-Chromogranin A Hybrid Peptide activates DR11 restricted T cells in human type 1 diabetes. Diabetes. (2024). doi: 10.2337/db23-0622
97. Crawford SA, Groegler J, Dang M, Michel C, Powell RL, Hohenstein AC, et al. Hybrid insulin peptide isomers spontaneously form in pancreatic beta-cells from an aspartic anhydride intermediate. J Biol Chem. (2023) 299:105264. doi: 10.1016/j.jbc.2023.105264
98. Crawford SA, Wiles TA, Wenzlau JM, Powell RL, Barbour G, Dang M, et al. Cathepsin D drives the formation of hybrid insulin peptides relevant to the pathogenesis of type 1 diabetes. Diabetes. (2022) 71:2793–803. doi: 10.2337/db22-0303
99. Delong T, Wiles TA, Baker RL, Bradley B, Barbour G, Reisdorph R, et al. Pathogenic CD4 T cells in type 1 diabetes recognize epitopes formed by peptide fusion. Science. (2016) 351:711–4. doi: 10.1126/science.aad2791
100. Jamison BL, DiLisio JE, Beard KS, Neef T, Bradley B, Goodman J, et al. Tolerogenic delivery of a hybrid insulin peptide markedly prolongs islet graft survival in the NOD mouse. Diabetes. (2022) 71:483–96. doi: 10.2337/db20-1170
101. Jamison BL, Neef T, Goodspeed A, Bradley B, Baker RL, Miller SD, et al. Nanoparticles containing an insulin-chgA hybrid peptide protect from transfer of autoimmune diabetes by shifting the balance between effector T cells and regulatory T cells. J Immunol. (2019) 203:48–57. doi: 10.4049/jimmunol.1900127
102. Wenzlau JM, DiLisio JE, Barbour G, Dang M, Hohenstein AC, Nakayama M, et al. Insulin B-chain hybrid peptides are agonists for T cells reactive to insulin B:9-23 in autoimmune diabetes. Front Immunol. (2022) 13:926650. doi: 10.3389/fimmu.2022.926650
103. Wenzlau JM, Gu Y, Michels A, Rewers M, Haskins K, Yu L. Identification of autoantibodies to a hybrid insulin peptide in type 1 diabetes. Diagnostics (Basel). (2023) 13(17). doi: 10.3390/diagnostics13172859
104. Wiles TA, Delong T, Baker RL, Bradley B, Barbour G, Powell RL, et al. An insulin-IAPP hybrid peptide is an endogenous antigen for CD4 T cells in the non-obese diabetic mouse. J Autoimmun. (2017) 78:11–8. doi: 10.1016/j.jaut.2016.10.007
105. Wiles TA, Hohenstein A, Landry LG, Dang M, Powell R, Guyer P, et al. Characterization of human CD4 T cells specific for a C-peptide/C-peptide hybrid insulin peptide. Front Immunol. (2021) 12:668680. doi: 10.3389/fimmu.2021.668680
106. Wiles TA, Powell R, Michel R, Beard KS, Hohenstein A, Bradley B, et al. Identification of hybrid insulin peptides (HIPs) in mouse and human islets by mass spectrometry. J Proteome Res Mar 1. (2019) 18:814–25. doi: 10.1021/acs.jproteome.8b00875
107. Hu X, Deutsch AJ, Lenz TL, Onengut-Gumuscu S, Han B, Chen WM, et al. Additive and interaction effects at three amino acid positions in HLA-DQ and HLA-DR molecules drive type 1 diabetes risk. Nat Genet Aug. (2015) 47:898–905. doi: 10.1038/ng.3353
108. Dashti M, Nizam R, Jacob S, Al-Kandari H, Al Ozairi E, Thanaraj TA, et al. Association between alleles, haplotypes, and amino acid variations in HLA class II genes and type 1 diabetes in Kuwaiti children. Front Immunol. (2023) 14:1238269. doi: 10.3389/fimmu.2023.1238269
109. Pappas DJ, Marin W, Hollenbach JA, Mack SJ. Bridging ImmunoGenomic Data Analysis Workflow Gaps (BIGDAWG): An integrated case-control analysis pipeline. Hum Immunol. (2016) 77:283–7. doi: 10.1016/j.humimm.2015.12.006
110. Pappas DJ, Lizee A, Paunic V, Beutner KR, Motyer A, Vukcevic D, et al. Significant variation between SNP-based HLA imputations in diverse populations: the last mile is the hardest. Pharmacogenomics J. (2018) 18:367–76. doi: 10.1038/tpj.2017.7
111. Redondo MJ, Oram RA, Steck AK. Genetic risk scores for type 1 diabetes prediction and diagnosis. Curr Diabetes Rep. (2017) 17:129. doi: 10.1007/s11892-017-0961-5
112. Johnson MB, Patel KA, De Franco E, Houghton JAL, McDonald TJ, Ellard S, et al. A type 1 diabetes genetic risk score can discriminate monogenic autoimmunity with diabetes from early-onset clustering of polygenic autoimmunity with diabetes. Diabetologia. (2018) 61:862–9. doi: 10.1007/s00125-018-4551-0
113. Redondo MJ, Geyer S, Steck AK, Sharp S, Wentworth JM, Weedon MN, et al. A type 1 diabetes genetic risk score predicts progression of islet autoimmunity and development of type 1 diabetes in individuals at risk. Diabetes Care. (2018) 41:1887–94. doi: 10.2337/dc18-0087
114. Sharp SA, Weedon MN, Hagopian WA, Oram RA. Clinical and research uses of genetic risk scores in type 1 diabetes. Curr Opin Genet Dev. (2018) 50:96–102. doi: 10.1016/j.gde.2018.03.009
115. Perry DJ, Wasserfall CH, Oram RA, Williams MD, Posgai A, Muir AB, et al. Application of a genetic risk score to racially diverse type 1 diabetes populations demonstrates the need for diversity in risk-modeling. Sci Rep. (2018) 8:4529. doi: 10.1038/s41598-018-22574-5
116. Onengut-Gumuscu S, Chen WM, Robertson CC, Bonnie JK, Farber E, Zhu Z, et al. Type 1 diabetes risk in african-ancestry participants and utility of an ancestry-specific genetic risk score. Diabetes Care. (2019) 42:406–15. doi: 10.2337/dc18-1727
117. Harrison JW, Tallapragada DSP, Baptist A, Sharp SA, Bhaskar S, Jog KS, et al. Type 1 diabetes genetic risk score is discriminative of diabetes in non-Europeans: evidence from a study in India. Sci Rep. (2020) 10:9450. doi: 10.1038/s41598-020-65317-1
118. Luckett AM, Weedon MN, Hawkes G, Leslie RD, Oram RA, Grant SFA. Utility of genetic risk scores in type 1 diabetes. Diabetologia. (2023) 66:1589–600. doi: 10.1007/s00125-023-05955-y
119. Sharp SA, Rich SS, Wood AR, Jones SE, Beaumont RN, Harrison JW, et al. Development and standardization of an improved type 1 diabetes genetic risk score for use in newborn screening and incident diagnosis. Diabetes Care. (2019) 42:200–7. doi: 10.2337/dc18-1785
120. Redondo MJ, Gignoux CR, Dabelea D, Hagopian WA, Onengut-Gumuscu S, Oram RA, et al. Type 1 diabetes in diverse ancestries and the use of genetic risk scores. Lancet Diabetes Endocrinol. (2022) 10:597–608. doi: 10.1016/S2213-8587(22)00159-0
121. Guertin KA, Repaske DR, Taylor JF, Williams ES, Onengut-Gumuscu S, Chen WM, et al. Implementation of type 1 diabetes genetic risk screening in children in diverse communities: the Virginia PrIMeD project. Genome Med. (2024) 16:31. doi: 10.1186/s13073-024-01305-8
122. Davis TME, Peters KE, Davis W. Use of a type 1 genetic risk score for classification of diabetes type in young Australian adults: the Fremantle Diabetes Study Phase II. Intern Med J. (2024). doi: 10.1111/imj.16328
123. Petersdorf EW, Malkki M, O’HUigin C, Carrington M, Gooley T, Haagenson MD, et al. High HLA-DP expression and graft-versus-host disease. N Engl J Med. (2015) 373:599–609. doi: 10.1056/NEJMoa1500140
124. Schone B, Bergmann S, Lang K, Wagner I, Schmidt AH, Petersdorf EW, et al. Predicting an HLA-DPB1 expression marker based on standard DPB1 genotyping: Linkage analysis of over 32,000 samples. Hum Immunol. (2018) 79:20–7. doi: 10.1016/j.humimm.2017.11.001
125. Aydemir O, Noble JA, Bailey JA, Lernmark A, Marsh P, Andersson Svard A, et al. Genetic variation within the HLA-DRA1 gene modulates susceptibility to type 1 diabetes in HLA-DR3 homozygotes. Diabetes. (2019) 68:1523–7. doi: 10.2337/db18-1128
126. Smyth DJ, Cooper JD, Bailey R, Field S, Burren O, Smink LJ, et al. A genome-wide association study of nonsynonymous SNPs identifies a type 1 diabetes locus in the interferon-induced helicase (IFIH1) region. Nat Genet. (2006) 38:617–9. doi: 10.1038/ng1800
127. Ferreira RC, Guo H, Coulson RM, Smyth DJ, Pekalski ML, Burren OS, et al. A type I interferon transcriptional signature precedes autoimmunity in children genetically at risk for type 1 diabetes. Diabetes. (2014) 63:2538–50. doi: 10.2337/db13-1777
128. Nigi L, Laiho JE, Hyoty H, Dotta F. Editorial: The contribution of viruses and innate immune system in the pathogenesis of type 1 diabetes. Front Endocrinol (Lausanne). (2023) 14:1335716. doi: 10.3389/fendo.2023.1335716
129. Guo M, Guo H, Zhu J, Wang F, Chen J, Wan C, et al. A novel subpopulation of monocytes with a strong interferon signature indicated by SIGLEC-1 is present in patients with in recent-onset type 1 diabetes. Diabetologia. (2024). doi: 10.1007/s00125-024-06098-4
130. Quatrini L, Della Chiesa M, Sivori S, Mingari MC, Pende D, Moretta L. Human NK cells, their receptors and function. Eur J Immunol. (2021) 51:1566–79. doi: 10.1002/eji.202049028
131. Pende D, Falco M, Vitale M, Cantoni C, Vitale C, Munari E, et al. Killer ig-like receptors (KIRs): their role in NK cell modulation and developments leading to their clinical exploitation. Front Immunol. (2019) 10:1179. doi: 10.3389/fimmu.2019.01179
132. Pollock NR, Harrison GF, Norman PJ. Immunogenomics of killer cell immunoglobulin-like receptor (KIR) and HLA class I: coevolution and consequences for human health. J Allergy Clin Immunol Pract. (2022) 10:1763–75. doi: 10.1016/j.jaip.2022.04.036
133. Correction to “Autoimmune diabetes from chilhood to adulthood: the role of pancreatic autoantibodies and HLA-DRB1 genotype. J Clin Endocrinol Metab. (2024) 109:e879. doi: 10.1210/clinem/dgad609
134. Akesson C, Uvebrant K, Oderup C, Lynch K, Harris RA, Lernmark A, et al. Altered natural killer (NK) cell frequency and phenotype in latent autoimmune diabetes in adults (LADA) prior to insulin deficiency. Clin Exp Immunol. (2010) 161:48–56. doi: 10.1111/j.1365-2249.2010.04114.x
135. Gunavathy N, Asirvatham A, Chitra A, Jayalakshmi M. Association of killer cell immunoglobulin-like receptors and their HLA-ligands with type 1 diabetes among south Indian population. Immunol Invest. (2023) 52:270–85. doi: 10.1080/08820139.2023.2165940
136. Jobim M, Chagastelles P, Salim PH, Portela P, Wilson TJ, Curti AG, et al. Association of killer cell immunoglobulin-like receptors and human leukocyte antigen-C genotypes in South Brazilian with type 1 diabetes. Hum Immunol. (2010) 71:799–803. doi: 10.1016/j.humimm.2010.05.014
137. Liu SL, Zheng AJ, Ding L. Association between KIR gene polymorphisms and type 1 diabetes mellitus (T1DM) susceptibility: A PRISMA-compliant meta-analysis. Med (Baltimore). (2017) 96:e9439. doi: 10.1097/MD.0000000000009439
138. Mehers KL, Long AE, van der Slik AR, Aitken RJ, Nathwani V, Wong FS, et al. An increased frequency of NK cell receptor and HLA-C group 1 combinations in early-onset type 1 diabetes. Diabetologia. (2011) 54:3062–70. doi: 10.1007/s00125-011-2299-x
139. Middleton D, Halfpenny I, Meenagh A, Williams F, Sivula J, Tuomilehto-Wolf E. Investigation of KIR gene frequencies in type 1 diabetes mellitus. Hum Immunol. (2006) 67:986–90. doi: 10.1016/j.humimm.2006.08.295
140. Mogami S, Hasegawa G, Nakayama I, Asano M, Hosoda H, Kadono M, et al. Killer cell immunoglobulin-like receptor genotypes in Japanese patients with type 1 diabetes. Tissue Antigens. (2007) 70:506–10. doi: 10.1111/j.1399-0039.2007.00956.x
141. Nikitina-Zake L, Rajalingham R, Rumba I, Sanjeevi CB. Killer cell immunoglobulin-like receptor genes in Latvian patients with type 1 diabetes mellitus and healthy controls. Ann N Y Acad Sci. (2004) 1037:161–9. doi: 10.1196/annals.1337.027
142. Osman AE, Eltayeb EN, Mubasher M, Al Harthi H, Alharbi S, Hamza MA, et al. Investigation of activating and inhibitory killer cell immunoglobulin-like receptors and their putative ligands in type 1 diabetes (T1D). Hum Immunol. (2016) 77:110–4. doi: 10.1016/j.humimm.2015.10.019
143. Park Y, Choi H, Park H, Park S, Yoo EK, Kim D, et al. Predominance of the group A killer Ig-like receptor haplotypes in Korean patients with T1D. Ann N Y Acad Sci. (2006) 1079:240–50. doi: 10.1196/annals.1375.037
144. Ramos-Lopez E, Scholten F, Aminkeng F, Wild C, Kalhes H, Seidl C, et al. Association of KIR2DL2 polymorphism rs2756923 with type 1 diabetes and preliminary evidence for lack of inhibition through HLA-C1 ligand binding. Tissue Antigens. (2009) 73:599–603. doi: 10.1111/j.1399-0039.2009.01252.x
145. Sanjeevi S, Sun C, Kanungo A, Sanjeevi CB. Killer immunoglobulin receptor genes and their HLA-C ligand are associated with Type 1 diabetes in an Eastern Indian population. Diabetes Med. (2016) 33:91–6. doi: 10.1111/dme.12815
146. Santin I, de Nanclares GP, Calvo B, Gaafar A, Castano L, Group G-N, et al. Killer cell immunoglobulin-like receptor (KIR) genes in the Basque population: association study of KIR gene contents with type 1 diabetes mellitus. Hum Immunol. (2006) 67:118–24. doi: 10.1016/j.humimm.2006.02.036
147. Shastry A, Sedimbi SK, Rajalingam R, Nikitina-Zake L, Rumba I, Wigzell H, et al. Combination of KIR 2DL2 and HLA-C1 (Asn 80) confers susceptibility to type 1 diabetes in Latvians. Int J Immunogenet. (2008) 35:439–46. doi: 10.1111/j.1744-313X.2008.00804.x
148. Shastry A, Sedimbi SK, Rajalingam R, Rumba I, Kanungo A, Sanjeevi CB. Different KIRs confer susceptibility and protection to adults with latent autoimmune diabetes in Latvian and Asian Indian populations. Ann N Y Acad Sci. (2008) 1150:133–8. doi: 10.1196/annals.1447.058
149. Smigoc Schweiger D, Mendez A, Kunilo Jamnik S, Bratanic N, Bratina N, Battelino T, et al. Genetic risk for co-occurrence of type 1 diabetes and celiac disease is modified by HLA-C and killer immunoglobulin-like receptors. Tissue Antigens. (2014) 84:471–8. doi: 10.1111/tan.12450
150. Soltani S, Mostafaei S, Aslani S, Farhadi E, Mahmoudi M. Association of KIR gene polymorphisms with Type 1 Diabetes: a meta-analysis. J Diabetes Metab Disord. (2020) 19:1777–86. doi: 10.1007/s40200-020-00569-2
151. Sun C, Sanjeevi S, Luo F, Zhi D, Sanjeevi CB. Interactions between maternal killer cell immunoglobulin receptor genes and foetal HLA ligand genes contribute to type 1 diabetes susceptibility in Han Chinese. Int J Immunogenet. (2016) 43:125–30. doi: 10.1111/iji.12257
152. Tan YY, Lin J, Huang G, Tang WL, Sedimbi SK, Sanjeevi CB, et al. Killer cell immunoglobin-like receptor and its ligand gene polymorphisms in Hunan Han patients with type 1 diabetes. Zhonghua Yi Xue Za Zhi. (2010) 90:236–40.
153. Traherne JA, Jiang W, Valdes AM, Hollenbach JA, Jayaraman J, Lane JA, et al. KIR haplotypes are associated with late-onset type 1 diabetes in European-American families. Genes Immun. (2016) 17:8–12. doi: 10.1038/gene.2015.44
154. van der Slik AR, Alizadeh BZ, Koeleman BP, Roep BO, Giphart MJ. Modelling KIR-HLA genotype disparities in type 1 diabetes. Tissue Antigens. (2007) 69 Suppl 1:101–5. doi: 10.1111/j.1399-0039.2006.762_5.x
155. van der Slik AR, Koeleman BP, Verduijn W, Bruining GJ, Roep BO, Giphart MJ. KIR in type 1 diabetes: disparate distribution of activating and inhibitory natural killer cell receptors in patients versus HLA-matched control subjects. Diabetes. (2003) 52:2639–42. doi: 10.2337/diabetes.52.10.2639
156. Zhi D, Sun C, Sedimbi SK, Luo F, Shen S, Sanjeevi CB. Killer cell immunoglobulin-like receptor along with HLA-C ligand genes are associated with type 1 diabetes in Chinese Han population. Diabetes Metab Res Rev. (2011) 27:872–7. doi: 10.1002/dmrr.1264
157. Redondo-Garcia S, Barritt C, Papagregoriou C, Yeboah M, Frendeus B, Cragg MS, et al. Human leukocyte immunoglobulin-like receptors in health and disease. Front Immunol. (2023) 14:1282874. doi: 10.3389/fimmu.2023.1282874
158. Storm L, Bruijnesteijn J, de Groot NG, Bontrop RE. The genomic organization of the LILR region remained largely conserved throughout primate evolution: implications for health and disease. Front Immunol. (2021) 12:716289. doi: 10.3389/fimmu.2021.716289
159. Kim K, Oh SJ, Lee J, Kwon A, Yu CY, Kim S, et al. Regulatory variants on the leukocyte immunoglobulin-like receptor gene cluster are associated with crohn’s disease and interact with regulatory variants for TAP2. J Crohns Colitis. (2024) 18:47–53. doi: 10.1093/ecco-jcc/jjad127
160. Wang H, Wang Y, Tang Y, Ye H, Zhang X, Zhou G, et al. Frequencies of the LILRA3 6.7-kb deletion are highly differentiated among han Chinese subpopulations and involved in ankylosing spondylitis predisposition. Front Genet. (2019) 10:869. doi: 10.3389/fgene.2019.00869
161. Kuroki K, Tsuchiya N, Shiroishi M, Rasubala L, Yamashita Y, Matsuta K, et al. Extensive polymorphisms of LILRB1 (ILT2, LIR1) and their association with HLA-DRB1 shared epitope negative rheumatoid arthritis. Hum Mol Genet. (2005) 14:2469–80. doi: 10.1093/hmg/ddi247
162. Davis DM, Mandelboim O, Luque I, Baba E, Boyson J, Strominger JL. The transmembrane sequence of human histocompatibility leukocyte antigen (HLA)-C as a determinant in inhibition of a subset of natural killer cells. J Exp Med. (1999) 189:1265–74. doi: 10.1084/jem.189.8.1265
163. Niehrs A, Altfeld M. Regulation of NK-cell function by HLA class II. Front Cell Infect Microbiol. (2020) 10:55. doi: 10.3389/fcimb.2020.00055
164. Baumdick ME, Niehrs A, Degenhardt F, Schwerk M, Hinrichs O, Jordan-Paiz A, et al. HLA-DP on epithelial cells enables tissue damage by NKp44(+) natural killer cells in ulcerative colitis. Gastroenterology. (2023) 165:946–962.e13. doi: 10.1053/j.gastro.2023.06.034
165. Zecher BF, Ellinghaus D, Schloer S, Niehrs A, Padoan B, Baumdick ME, et al. HLA-DPA1*02:01~B1*01:01 is a risk haplotype for primary sclerosing cholangitis mediating activation of NKp44+ NK cells. Gut. (2024) 73:325–37. doi: 10.1136/gutjnl-2023-329524
166. Michalek DA, Tern C, Zhou W, Robertson CC, Farber E, Campolieto P, et al. A multi-ancestry genome-wide association study in type 1 diabetes. Hum Mol Genet. (2024) 33:958–68. doi: 10.1093/hmg/ddae024
167. Al Naqbi H, Mawart A, Alshamsi J, Al Safar H, Tay GK. Major histocompatibility complex (MHC) associations with diseases in ethnic groups of the Arabian Peninsula. Immunogenetics. (2021) 73:131–52. doi: 10.1007/s00251-021-01204-x
168. Hamzeh AR, Nair P, Al Ali MT. The profile of HLA-DRB1 alleles in Arabs with type 1 diabetes; meta-analyses. HLA. (2016) 87:25–30. doi: 10.1111/tan.12717
169. Fagbemi KA, Medehouenou TCM, Azonbakin S, Adjagba M, Osseni R, Ahoueya J, et al. HLA class II allele, haplotype, and genotype associations with type 1 diabetes in Benin: A pilot study. J Diabetes Res. (2017) 2017:6053764. doi: 10.1155/2017/6053764
170. Gomes KF, Santos AS, Semzezem C, Correia MR, Brito LA, Ruiz MO, et al. The influence of population stratification on genetic markers associated with type 1 diabetes. Sci Rep. (2017) 7:43513. doi: 10.1038/srep43513
171. Gomes MB, Rodrigues V, Santos DC, Boas PRV, Silva DA, de Sousa Azulay RS, et al. Association between HLA class II alleles/haplotypes and genomic ancestry in Brazilian patients with type 1 diabetes: A nationwide exploratory study. Genes (Basel). (2023) 14(5). doi: 10.3390/genes14050991
172. Azulay RSS, Porto LC, Silva DA, Tavares MDG, Reis R, Nascimento GC, et al. Genetic ancestry inferred from autosomal and Y chromosome markers and HLA genotypes in Type 1 Diabetes from an admixed Brazilian population. Sci Rep. (2021) 11:14157. doi: 10.1038/s41598-021-93691-x
173. Santos DC, Porto LC, Oliveira RV, Secco D, Hanhoerderster L, Pizarro MH, et al. HLA class II genotyping of admixed Brazilian patients with type 1 diabetes according to self-reported color/race in a nationwide study. Sci Rep. (2020) 10:6628. doi: 10.1038/s41598-020-63322-y
174. Ding Y, Zhang P, Deng T, Yan X, Zhang M, Xie Z, et al. Association of human leukocyte antigen (HLA) footprints with the comorbidity of latent autoimmune diabetes in adults (LADA) and hepatitis C virus (HCV) infection: A multicenter cross-sectional study. Diabetes Metab Syndr. (2024) 18:102939. doi: 10.1016/j.dsx.2023.102939
175. Xia Y, Chen Y, Li X, Luo S, Lin J, Huang G, et al. HLA class I association with autoimmune diabetes in Chinese people: distinct implications in classic type 1 diabetes and LADA. J Clin Endocrinol Metab. (2023) 108:e404–14. doi: 10.1210/clinem/dgad006
176. Chen Y, Xia Y, Xie Z, Zhong T, Tang R, Li X, et al. The unfavorable impact of DR9/DR9 genotype on the frequency and quality of partial remission in type 1 diabetes. J Clin Endocrinol Metab. (2022) 107:e293–302. doi: 10.1210/clinem/dgab589
177. Xia Y, Li X, Huang G, Lin J, Luo S, Xie Z, et al. The association of HLA-DP loci with autoimmune diabetes in Chinese. Diabetes Res Clin Pract. (2021) 173:108582. doi: 10.1016/j.diabres.2020.108582
178. Jiang Z, Ren W, Liang H, Yan J, Yang D, Luo S, et al. HLA class I genes modulate disease risk and age at onset together with DR-DQ in Chinese patients with insulin-requiring type 1 diabetes. Diabetologia. (2021) 64:2026–36. doi: 10.1007/s00125-021-05476-6
179. Ren W, Yang D, Jiang Z, Xian Y, Huang Q, Luo S, et al. Adult-onset type 1 diabetic patients with less severe clinical manifestation have less risk DR-DQ genotypes than childhood-onset patients. Diabetes Metab Res Rev. (2021) 37:e3357. doi: 10.1002/dmrr.3357
180. Yin NN, Luo SM, Lin J, Xie ZG, Huang G, Li X, et al. Identification of HLA class II susceptible alleles and genotypes in latent autoimmune diabetes in adults. Zhonghua Yi Xue Za Zhi. (2017) 97:581–6. doi: 10.3760/cma.j.issn.0376-2491.2017.08.005
181. Gomez-Lopera N, Alfaro JM, Leal SM, Pineda-Trujillo N. Type 1 diabetes loci display a variety of native American and African ancestries in diseased individuals from Northwest Colombia. World J Diabetes. (2019) 10:534–45. doi: 10.4239/wjd.v10.i11.534
182. Sarrazola DC, Rodriguez AM, Toro M, Velez A, Garcia-Ramirez J, Lopera MV, et al. Classical HLA alleles tag SNP in families from Antioquia with type 1 diabetes mellitus. Biomedica. (2018) 38:329–37. doi: 10.7705/biomedica.v38i3.3768
183. Grubic Z, Rojnic Putarek N, Maskalan M, Zunec R, Stingl Jankovic K, Burek Kamenaric M, et al. Human Leukocyte Antigen class II polymorphisms among Croatian patients with type 1 diabetes and autoimmune polyglandular syndrome type 3 variant. Gene. (2018) 674:93–7. doi: 10.1016/j.gene.2018.06.083
184. Gerasimou P, Nicolaidou V, Skordis N, Picolos M, Monos D, Costeas PA. Combined effect of glutamine at position 70 of HLA-DRB1 and alanine at position 57 of HLA-DQB1 in type 1 diabetes: An epitope analysis. PloS One. (2018) 13:e0193684. doi: 10.1371/journal.pone.0193684
185. El-Amir MI, El-Feky MA, ELAbd A, El-Melegy TT, J I. HLA-B*08 carry a risk for type 1 diabetes among cow’s milk exposed Egyptian infants and unmarked linkage disequilibrium with DR3-DQA1*05-DQB1*02 haplotype. Egypt J Immunol. (2019) 26:113–20.
186. Balcha SA, Demisse AG, Mishra R, Vartak T, Cousminer DL, Hodge KM, et al. Type 1 diabetes in Africa: an immunogenetic study in the Amhara of North-West Ethiopia. Diabetologia. (2020) 63:2158–68. doi: 10.1007/s00125-020-05229-x
187. Zhao LP, Papadopoulos GK, Moustakas AK, Bondinas GP, Carlsson A, Larsson HE, et al. Nine residues in HLA-DQ molecules determine with susceptibility and resistance to type 1 diabetes among young children in Sweden. Sci Rep. (2021) 11:8821. doi: 10.1038/s41598-021-86229-8
188. Hommel A, Haupt F, Delivani P, Winkler C, Stopsack M, Wimberger P, et al. Screening for type 1 diabetes risk in newborns: the freder1k pilot study in saxony. Horm Metab Res. (2018) 50:44–9. doi: 10.1055/s-0043-120921
189. Singh GC, Ahmed M, Zaid M, Hasnain S. Biochemical, serological, and genetic aspects related to gene HLA-DQB1 and its association with type 1 diabetes mellitus (T1DM). Mol Genet Genomic Med. (2020) 8:e1147. doi: 10.1002/mgg3.1147
190. Kumar N, Mehra NK, Kanga U, Kaur G, Tandon N, Chuzho N, et al. Diverse human leukocyte antigen association of type 1 diabetes in north India. J Diabetes. (2019) 11:719–28. doi: 10.1111/1753-0407.12898
191. Kaur N, Singh J, Minz RW, Anand S, Saikia B, Bhadada SK, et al. Shared and distinct genetics of pure type 1 diabetes and type 1 diabetes with celiac disease, homology in their auto-antigens and immune dysregulation states: a study from North India. Acta Diabetol. (2024) 61:791–805. doi: 10.1007/s00592-024-02258-5
192. Chuzho N, Kumar N, Mishra N, Tandon N, Kanga U, Kaur G, et al. Differential HLA association of GAD65 and IA2 autoantibodies in north Indian type 1 diabetes patients. J Diabetes Res. (2021) 2021:4012893. doi: 10.1155/2021/4012893
193. Padma-Malini R, Rathika C, Ramgopal S, Murali V, Dharmarajan P, Pushkala S, et al. Associations of CTLA4 + 49 A/G dimorphism and HLA-DRB1*/DQB1* Alleles with type 1 diabetes from south India. Biochem Genet. (2018) 56:489–505. doi: 10.1007/s10528-018-9856-7
194. Solgi H, Badamchi A, Shahcheraghi F, Badmasti F, Akbari M, Behzadfar M. A comparative evaluation of five phenotypic methods for identification of carbapenemase-producing Enterobacteriaceae: a modified carbapenemase detection test. Microbiol Spectr. (2024):e0038624. doi: 10.1128/spectrum.00386-24
195. Ricci S, Perugia F, Piccini B, Lodi L, Pegoraro F, Giovannini M, et al. DR4/DQ2 haplotype confers susceptibility to T1DM with early clinical disease onset: A retrospective analysis in a tertiary-care hospital in Italy. PloS One. (2022) 17:e0276896. doi: 10.1371/journal.pone.0276896
196. Yamada E, Kajita R, Takahashi H, Horiguchi K, Yoshino S, Matsumoto S, et al. Exploring potential correlations between HLA class II and the risk of microvascular complications in Japanese patients with type 1 diabetes. J Diabetes Complications. (2024) 38:108763. doi: 10.1016/j.jdiacomp.2024.108763
197. Chujo D, Imagawa A, Yasuda K, Abiru N, Awata T, Fukui T, et al. Japanese Type 1 Diabetes Database Study (TIDE-J): rationale and study design. Diabetol Int. (2022) 13:288–94. doi: 10.1007/s13340-021-00541-2
198. Katahira M, Tsunekawa T, Mizoguchi A, Yamaguchi M, Tsuru K, Takashima H, et al. Critical amino acid variants in HLA-DRB1 and -DQB1 allotypes in the development of classical type 1 diabetes and latent autoimmune diabetes in adults in the Japanese population. Curr Issues Mol Biol. (2021) 43:107–15. doi: 10.3390/cimb43010009
199. Khdair SI, Jarrar W, Jarrar YB, Bataineh S, Al-Khaldi O. Association of HLA-DRB1 and -DQ alleles and haplotypes with type 1 diabetes in Jordanians. Endocr Metab Immune Disord Drug Targets. (2020) 20:895–902. doi: 10.2174/1871530319666191119114031
200. Haider MZ, Al Rushood M, Alsharhan H, Rasoul MA, Al-Mahdi M, Al-Kandari H. Association of interleukin-4, interleukin-13 gene polymorphisms, HLA-DQ and DR genotypes with genetic susceptibility of type-1 Diabetes Mellitus in Kuwaiti children. Front Pediatr. (2023) 11:1118137. doi: 10.3389/fped.2023.1118137
201. Jahromi M, Al-Ozairi E. Human leukocyte antigen (HLA) and islet autoantibodies are tools to characterize type 1 diabetes in Arab countries: emphasis on Kuwait. Dis Markers. (2019) 2019:9786078. doi: 10.1155/2019/9786078
202. Haider MZ, Rasoul MA, Al-Mahdi M, Al-Kandari H, Dhaunsi GS. Association of protein tyrosine phosphatase non-receptor type 22 gene functional variant C1858T, HLA-DQ/DR genotypes and autoantibodies with susceptibility to type-1 diabetes mellitus in Kuwaiti Arabs. PloS One. (2018) 13:e0198652. doi: 10.1371/journal.pone.0198652
203. Spinola H, Lemos A, Couto AR, Parreira B, Soares M, Dutra I, et al. Human leucocyte antigens class II allele and haplotype association with Type 1 Diabetes in Madeira Island (Portugal). Int J Immunogenet. Dec. (2017) 44:305–13. doi: 10.1111/iji.12335
204. Gomez-Diaz RA, Nishimura-Meguro E, Garrido-Magana E, Lizarraga-Paulin L, Aguilar-Herrera BE, Bekker-Mendez C, et al. HLA risk haplotype: insulin deficiency in pediatric type 1 diabetes. Rev Invest Clin May-Jun. (2016) 68:128–36.
205. Willis J, Cunningham-Tisdall C, Griffin C, Scott R, Darlow BA, Owens N, et al. Type 1 diabetes diagnosed before age 15 years in Canterbury, New Zealand: A 50 year record of increasing incidence. Pediatr Diabetes. May. (2022) 23:301–9. doi: 10.1111/pedi.13305
206. Stordal K, Tapia G, Lund-Blix NA, Stene LC. Genotypes predisposing for celiac disease and autoimmune diabetes and risk of infections in early childhood. J Pediatr Gastroenterol Nutr. (2024) 78:295–303. doi: 10.1002/jpn3.12078
207. Al-Balushi M, Al-Badi S, Al-Yaarubi S, Al-Riyami H, Al-Shidhani A, Al-Hinai S, et al. The association of human leukocyte antigens complex with type 1 diabetes in the Omani population. Sultan Qaboos Univ Med J. (2023) 23:68–75. doi: 10.18295/squmj.2.2022.016
208. Caramalho I, Matoso P, Ligeiro D, Paixao T, Sobral D, Fitas AL, et al. The rare DRB1*04:08-DQ8 haplotype is the main HLA class II genetic driver and discriminative factor of Early-onset Type 1 diabetes in the Portuguese population. Front Immunol. (2023) 14:1299609. doi: 10.3389/fimmu.2023.1299609
209. Haris B, Abdellatief A, Afyouni H, Abdel-Karim T, Mohammed S, Khalifa A, et al. The prevalence, immune profile, and clinical characteristics of children with celiac disease and type 1 diabetes mellitus in the state of Qatar. J Pediatr Endocrinol Metab. (2021) 34:1457–61. doi: 10.1515/jpem-2021-0452
210. Haris B, Ahmed I, Syed N, Almabrazi H, Saraswathi S, Al-Khawaga S, et al. Clinical features, epidemiology, autoantibody status, HLA haplotypes and genetic mechanisms of type 1 diabetes mellitus among children in Qatar. Sci Rep. (2021) 11:18887. doi: 10.1038/s41598-021-98460-4
211. Arhire AI, Ioacara S, Papuc T, Chiper MS, Dutescu IM, Moise A, et al. Association of HLA haplotypes with autoimmune pathogenesis in newly diagnosed type 1 Romanian diabetic children: A pilot, single-center cross-sectional study. Life (Basel). (2024) 14(6). doi: 10.3390/life14060781
212. Incani M, Serafini C, Satta C, Perra L, Scano F, Frongia P, et al. High prevalence of diabetes-specific autoimmunity in first-degree relatives of Sardinian patients with type 1 diabetes. Diabetes Metab Res Rev. (2017) 33(3). doi: 10.1002/dmrr.2864
213. Schirru E, Rossino R, Diana D, Jores RD, Baldera D, Muntoni S, et al. HLA genotyping in children with celiac disease allows to establish the risk of developing type 1 diabetes. Clin Transl Gastroenterol. (2024). doi: 10.14309/ctg.0000000000000710
214. Eltayeb-Elsheikh N, Khalil E, Mubasher M, AlJurayyan A, AlHarthi H, Omer WH, et al. Association of HLA-DR-DQ alleles, haplotypes, and diplotypes with type 1 diabetes in Saudis. Diabetes Metab Res Rev. (2020) 36:e3345. doi: 10.1002/dmrr.3345
215. Ali AA, Aalto M, Jonasson J, Osman A. Genome-wide analyses disclose the distinctive HLA architecture and the pharmacogenetic landscape of the Somali population. Sci Rep. (2020) 10:5652. doi: 10.1038/s41598-020-62645-0
216. Gandini A, Wyk NV, Maayer T, Gededzha MP, Mayne E. Coeliac disease high-risk human leukocyte antigen alleles in a South African type one diabetic population. Clin Lab. (2023) 69(8). doi: 10.7754/Clin.Lab.2023.220819
217. Urrutia I, Martinez R, Rica I, Martinez de LaPiscina I, Garcia-Castano A, Aguayo A, et al. Negative autoimmunity in a Spanish pediatric cohort suspected of type 1 diabetes, could it be monogenic diabetes? PloS One. (2019) 14:e0220634. doi: 10.1371/journal.pone.0220634
218. Katte JC, McDonald TJ, Sobngwi E, Jones AG. The phenotype of type 1 diabetes in sub-Saharan Africa. Front Public Health. (2023) 11:1014626. doi: 10.3389/fpubh.2023.1014626
219. Ibaid I, Hussien M, Kaukinen K, Sabir O, Elmekki M, Musa A, et al. Role of HLA-DQ typing and antitissue transglutaminase antibody titres in diagnosing coeliac disease among Sudanese children with type 1 diabetes mellitus. BMJ Open Gastroenterol. (2022) 9(1). doi: 10.1136/bmjgast-2021-000735
220. Ibrahim TAM, Govender D, Abdullah MA, Noble JA, Hussien MO, Lane JA, et al. Clinical features, biochemistry, and HLA-DRB1 status in youth-onset type 1 diabetes in Sudan. Pediatr Diabetes. (2021) 22:749–57. doi: 10.1111/pedi.13209
221. Alshiekh S, Geraghty DE, Agardh D. High-resolution genotyping of HLA class I loci in children with type 1 diabetes and celiac disease. HLA. (2021) 97:505–11. doi: 10.1111/tan.14280
222. Liao WL, Huang YN, Chang YW, Liu TY, Lu HF, Tiao ZY, et al. Combining polygenic risk scores and human leukocyte antigen variants for personalized risk assessment of type 1 diabetes in the Taiwanese population. Diabetes Obes Metab. (2023) 25:2928–36. doi: 10.1111/dom.15187
223. Tung YC, Fann CS, Chang CC, Chu CC, Yang WS, Hwu WL, et al. Comprehensive human leukocyte antigen genotyping of patients with type 1 diabetes mellitus in Taiwan. Pediatr Diabetes. (2018) 19:699–706. doi: 10.1111/pedi.12645
224. Hajjej A, Almawi WY, Stayoussef M, Arnaiz-Villena A, Hattab L, Hmida S. Association of HLA-DRB1 and -DQB1 alleles with type 1 (autoimmune) diabetes in African Arabs: systematic review and meta-analysis. Immunol Invest. (2019) 48:130–46. doi: 10.1080/08820139.2018.1493498
225. Al Yafei Z, Mack SJ, Alvares M, Ali BR, Afandi B, Beshyah SA, et al. HLA-DRB1 and -DQB1 alleles, haplotypes and genotypes in emirati patients with type 1 diabetes underscores the benefits of evaluating understudied populations. Front Genet. (2022) 13:841879. doi: 10.3389/fgene.2022.841879
226. Tay GK, Al Naqbi H, Mawart A, Baalfaqih Z, Almaazmi A, Deeb A, et al. Segregation analysis of genotyped and family-phased, long range MHC classical class I and class II haplotypes in 5 families with type 1 diabetes proband in the United Arab Emirates. Front Genet. (2021) 12:670844. doi: 10.3389/fgene.2021.670844
Keywords: Type 1 diabetes, autoimmunity, HLA, immune polymorphism, disease association, diversity,
Citation: Noble JA (2024) Fifty years of HLA-associated type 1 diabetes risk: history, current knowledge, and future directions. Front. Immunol. 15:1457213. doi: 10.3389/fimmu.2024.1457213
Received: 30 June 2024; Accepted: 16 August 2024;
Published: 12 September 2024.
Edited by:
Liping Yu, University of Colorado Anschutz Medical Campus, United StatesReviewed by:
Maki Nakayama, University of Colorado Denver, United StatesStuart Ian Mannering, University of Melbourne, Australia
Copyright © 2024 Noble. This is an open-access article distributed under the terms of the Creative Commons Attribution License (CC BY). The use, distribution or reproduction in other forums is permitted, provided the original author(s) and the copyright owner(s) are credited and that the original publication in this journal is cited, in accordance with accepted academic practice. No use, distribution or reproduction is permitted which does not comply with these terms.
*Correspondence: Janelle A. Noble, jnoble58@berkeley.edu