- 1First Clinical Medical College, Shanxi Medical University, Taiyuan, China
- 2Department of Neurosurgery, First Hospital of Shanxi Medical University, Taiyuan, China
- 3Department of Gastrointestinal Surgery, Harbin Medical University Cancer Hospital, Harbin, China
- 4Third Clinical Medical College, Harbin Medical University, Harbin, China
Background: A bibliometric and visual analysis of articles related to glioblastoma metabolism was conducted to reveal the dynamics of scientific development and to assist researchers in gaining a global perspective when exploring hotspots and trends.
Methods: The Web of Science Core Collection (WoSCC) was employed to search, screen, and download articles about glioblastoma metabolism published between 2014 and 2024. The relevant literature was analyzed using CiteSpace, VOSviewer and Microsoft Excel.
Results: A total of 729 articles were included for bibliometric analysis between 2014 and 2024, and the number of articles published each year showed an overall increasing trend, except for a decrease in the number of articles published in 2018 compared to 2017. Collaboration network analysis showed that the United States, Germany and China are influential countries in this field, with a high number of articles published, citations and collaborations with other countries. The journal with the largest number of published articles is the International Journal of Molecular Sciences. Mischel PS is the most prolific author with 14 articles, and Guo DL received the most citations with 104 citations. Keyword analysis of the literature showed that the “Warburg effect” achieved the highest burst intensity, and “central nervous system”, “classification” and “fatty acids” showed stronger citation bursts in 2024, indicating that they are still popular topics so far.
Conclusion: This article elucidates the research trends and focal points in the field of glioblastoma metabolism, furnishes invaluable insights into the historical and contemporary status of this field, and offers guidance for future research. Further research into glioblastoma metabolism will undoubtedly yield new insights that will inform the diagnosis and treatment of this disease.
1 Introduction
Glioblastoma (GBM) is a common malignant tumor in humans, with a high recurrence, mortality, aggressiveness, proliferation, and recurrence rate (1–3). Due to the critical function of the brain and the metastatic nature of GBM making surgical resection complex and challenging, coupled with patient resistance to temozolomide and the protective effect of the blood-brain barrier against tumors, even with the current standard of care, which consists of a combination of surgical resection, radiotherapy and chemotherapy with temozolomide, the outcome of patients with GBM is not satisfactory, with the median survival is only 8 months and a merely five-year survival rate of 6.9% (4–7).
More than 100 years ago, the German biochemist Otto Warburg discovered the metabolic differences between tumor cells and normal tissues and proposed the famous “Warburg effect” (8). This discovery was one of the major milestones in cancer research in the 20th century. Wahlberg observed that tumor cells tend to produce more energy through glycolysis than normal cells, even in the presence of sufficient oxygen (9). This discovery revealed the fundamental characteristics of tumor metabolism and provided important clues for subsequent cancer research and treatment.
As is the case with the majority of cancers, GBM cells undergo metabolic reprogramming for various growth- and survival-promoting functions, playing a critical role in GBM progression and recurrence (10). In GBM cells, common metabolic abnormalities include aberrations in lipid (11–14), amino acid (15–18) and nucleotide metabolism (19), as well as enhanced glycolysis (20–23). For example, the research team led by P. Chen discovered that the lactate dehydrogenase A (LDHA)-mediated extracellular signal-regulated kinase (ERK) pathway can activate the transcriptional coactivators YAP1 and STAT3 in GBM cells, thereby upregulating the expression of CCL2 and CCL7. GBM cells induce macrophages to infiltrate the tumor microenvironment by upregulating CCL2 and CCL7, and then macrophages promote tumor growth and survival by delivering LDHA to tumor cells (24). The present study proposes a novel strategy for the treatment of GBM, which targets the LDHA-mediated tumor-macrophage symbiosis. Given that these metabolic adaptations may be present in multiple genotypes, therapeutic strategies targeting aberrant metabolism may lead to new ideas for treating GBM in the clinic.
The intricate and diverse nature of the GBM immune microenvironment has historically posed a significant challenge in scientific research. Historically, the lack of effective tools for investigating TAMs in GBM has constrained our understanding of GBM heterogeneity (25, 26). However, with the ongoing advancement of artificial intelligence and machine learning technologies, coupled with the persistent efforts of scientific researchers, the study of tumor heterogeneity has made a significant advancement. Artificial intelligence can identify specific molecular changes in the process of tumor occurrence and development and predict the patient’s prognosis by processing a large amount of complex multi-omics data, thereby revealing the heterogeneous characteristics of the tumor microenvironment (27–29). It is anticipated that in the future, artificial intelligence will provide substantial technical support for the study of GBM metabolism and establish the foundation for future precision medicine and personalized treatment strategies.
Bibliometrics, which originated in the early 20th century, is a branch of scientometrics in informatics and refers to the interdisciplinary science of quantitative analysis of all knowledge carriers using mathematical and statistical methods (30). The application of bibliometrics can assist scientists and clinicians in identifying the research lineage and emerging trends within a specific research area (31–33). This enables a deeper understanding of the knowledge structure within the field of research. To date, several bibliometric articles about GBM have been published (34–37), yet there is no bibliometric study focused on GBM metabolism. The objective of this study is to provide a comprehensive analysis and visualization of research related to glioblastoma (GBM) metabolism, encompassing lipid, amino acid, nucleotide, and sugar metabolism, from 2014 to 2024. This analysis aims to evaluate the current research status in the field of GBM metabolism and identify potential future trends and research hotspots.
This study is based on WoSCC data and uses tools such as the Bibliometrics online analysis platform, CiteSpace, VOSviewer and Microsoft Excel to analyze the number of published articles on glioblastoma metabolism, countries, authors, institutions, journals, references, keywords, etc. To sort out the directions and hot spots of glioblastoma metabolism research and predict the direction of further research.
2 Materials and methods
2.1 Data acquisition
A data search was conducted on 1 May 2024 in the Web of Science Core Collection database (WoSCC) for relevant literature, including articles published between 1 January 2014 and 30 April 2024 related to glioblastoma metabolism. Wildcards were used systematically and scientifically to perform the search, and the specific search strategy is shown in Table 1. Only original research articles and review articles published in English-language journals were selected for analysis. Subsequently, two authors undertook an independent assessment of the relevance of each publication, based on an analysis of the title and abstract. Articles that were irrelevant to the study topic, incomplete, retracted, or duplicated were excluded. To mitigate the potential for bias in the analysis, dissenting articles were collectively deliberated upon and a final consensus was reached. The process of literature screening is illustrated in the accompanying Figure 1. All information from all the screened literature was saved in plain text file format.
2.2 Bibliometric analysis
The data was processed using Microsoft Office Excel 2016 (Microsoft Corporation, Redmond, WA, USA) to demonstrate the yearly growth trend of publications. CiteSpace (version 6.3.R2) is a citation visualization and analysis software that focuses on and analyses the fundamentals embedded in scientific analysis, developed in the context of scientometrics and data visualization. It is used to perform cluster analysis of keywords, references and other data sets, as well as the generation of timelines to illustrate the most significant citation outbreaks (38, 39). VOSviewer (version 1.6.20) is a computer program for building and viewing bibliometric maps (30, 40). It is employed to conduct co-occurrence analyses of countries/regions, institutions, authors and keywords in a variety of ways. The Bibliometric Analysis Online Platform (https://bibliometric.com/) is an online platform designed for bibliometric analysis. It is intended to assist researchers, academic institutions and policymakers in analyzing and visualizing citation networks and research trends in the academic literature. The platform is employed to illustrate the number of publications in the literature and the number of publications by country.
2.3 Ethics in research
The study did not require the consent of the Ethical Medical Council.
3 Results
3.1 Number and trend analysis of published papers
A comprehensive search of the WOScc database revealed a total of 2,258 research publications on GBM metabolism between 1 January 2014 and 30 April 2024. However, data from 2024 could not be fully included due to the search deadline. Following a series of screening processes, a total of 729 documents were ultimately selected for in-depth bibliometric analysis. Of the selected papers, 617 (84.6%) were articles and 112 (15.4%) were reviews. The results of the analyses revealed a clear trend: the number of research articles on GBM metabolism showed an overall increase during the period under review. Figure 2A illustrates the trend of publications, both in terms of annual and cumulative figures. Specifically, the number of related studies continued to increase between 2014 and 2017. Although there was a slight decline in 2018, academic interest in research in this field increased again from 2018 until 2022, reaching a peak in 2022, a total of 113 articles published that year. In 2023, 94 high-quality articles were published, although the number of publications declined from the previous year. The data provide ample evidence that GBM metabolism has received widespread academic attention and research, highlighting the importance and development potential of the field.
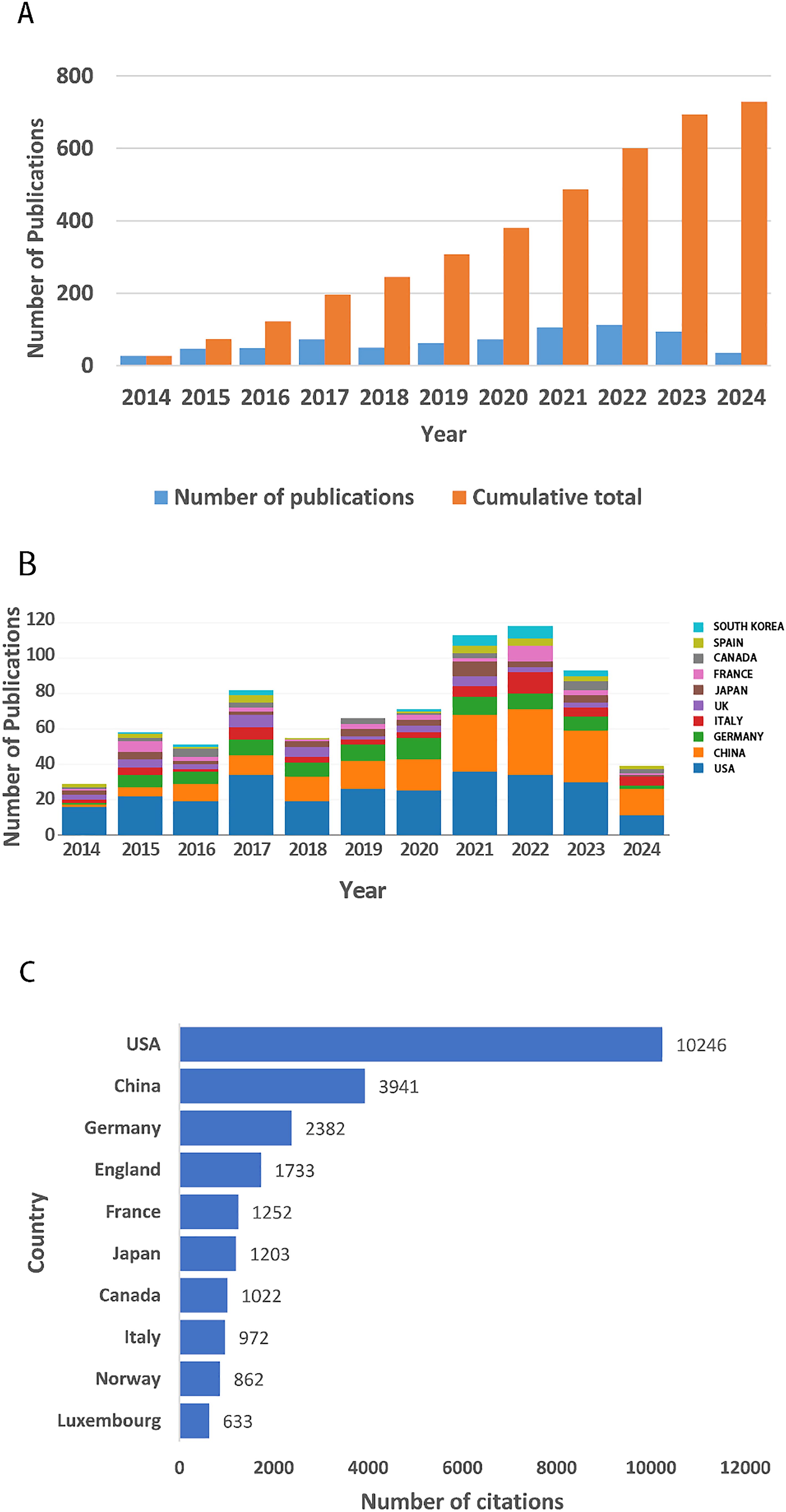
Figure 2. Number and trend of annual publications. (A) Annual publication volume and trends, including annual publication and cumulative publication. (B) Annual publication trends of the top 10 countries/regions. (C) Top 10 countries/regions with the highest total number of citations of publications.
3.2 Distribution of countries/areas and institutions
A total of 729 publications were obtained from 245 countries/regions and 3,314 institutions. As shown in Table 2, the countries with the highest number of publications were the USA (272, 37.3%) and China (181, 24.8%), followed by Germany (82, 11.2%), Italy (51, 7.0%) and the United Kingdom (38, 5.2%). The countries with the highest centrality in terms of publication are the USA (0.72), followed by Germany (0.33), Spain (0.18) and India (0.15). The United States leads the world in H-Index, with a total of 59, followed by China (37), Germany (31) and Japan (31). As evidenced by the relatively high number of publications and publication centrality, the United States and Germany have established a robust collaborative network with other countries in this field. Conversely, while Italy, the United Kingdom and China also occupy leading positions in terms of the number of publications, their relatively low centrality suggests that these regions engage in relatively limited cooperation with other countries. The H-Index of the United States and China is relatively high, indicating that the quality of articles published by these two countries is widely acknowledged by the academic community. The top 10 institutions in terms of number of published articles are shown in Table 3. The research institution with the highest number of publications was the University of California System (53), followed by the University of Texas System (37), Helmholtz Association (31) and German Cancer Research Center (DKFZ) (26). Among the top ten institutions in terms of number of publications, the University of California System (0.25) has the highest centrality, followed by Pennsylvania Commonwealth System of Higher Education (PCSHE) (0.23), Institut National de la Sante et de la Recherche Medicale (Inserm) (0.15) and University of Texas System (0.12). The University of California System has the highest H-Index, with a total of 25, followed by the University of Texas System (21) and the Helmholtz Association (19). Figure 3 shows a visual mapping of collaborative relationships between countries/regions and institutions. A node represents an institution. The size of the node is indicative of the number of publications and the institution’s centrality.
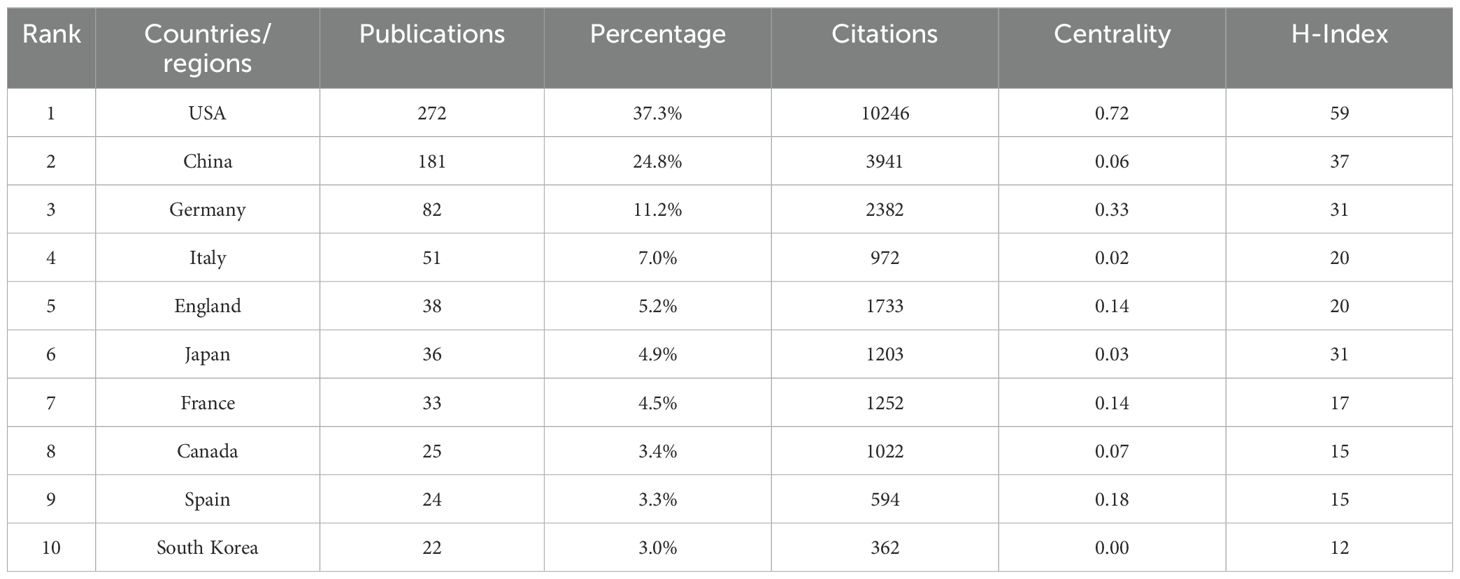
Table 2. Top 10 countries/regions regarding number of publications, the corresponding frequency of citations and centrality.
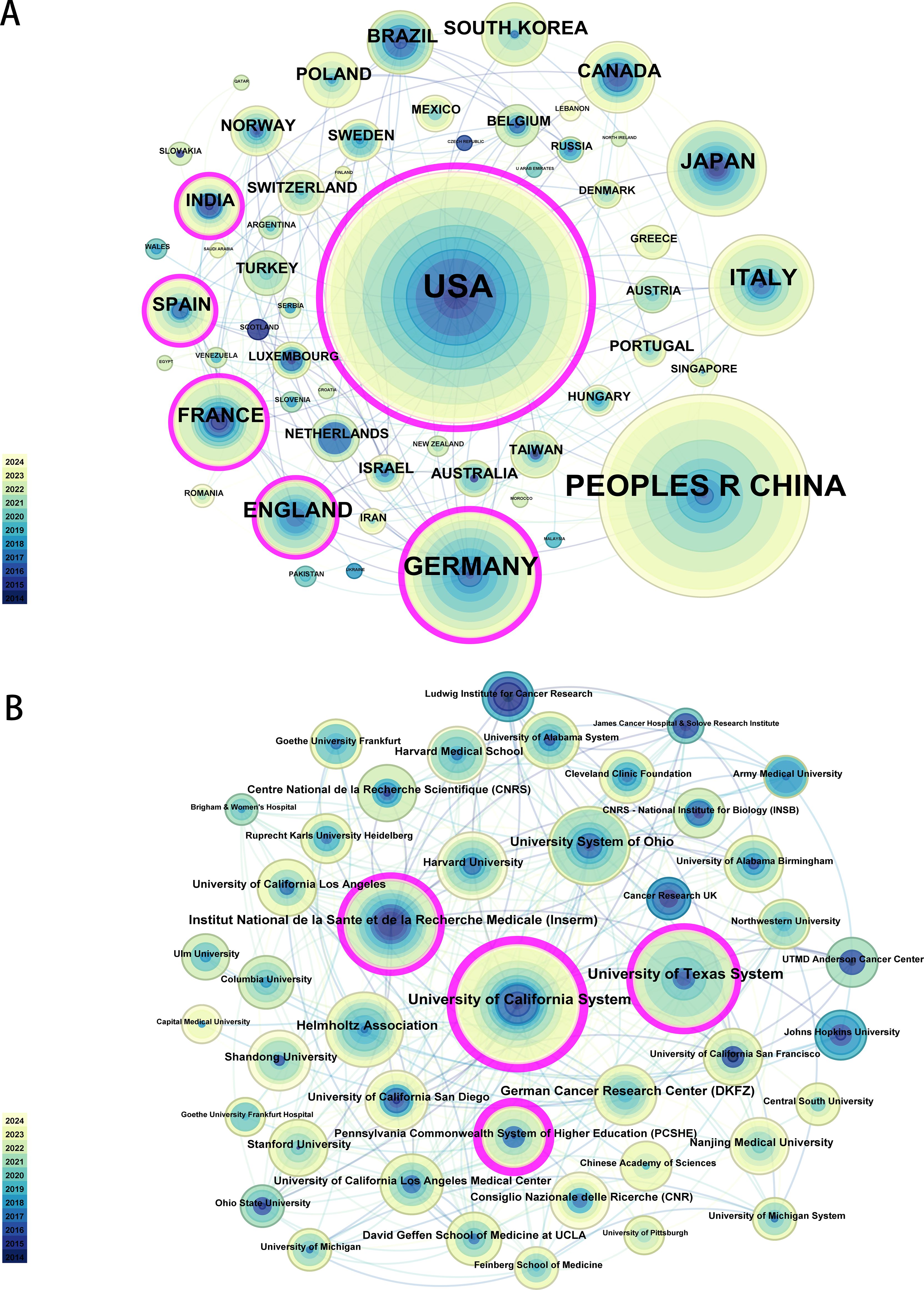
Figure 3. Visualization of collaboration between countries/regions and institutions (A) Collaboration between countries. (B) Collaboration between institutions. Each circle represents a country/region or institution. The size of the circle represents the publication output of the country/region or institution. The lines between the circles represent the collaboration between countries/regions or institutions. Nodes with high centrality are displayed as purple rings. The thickness of the purple rings represents the size of the betweenness centrality value.
3.3 Analysis of co-author networks and core author distribution
A total of 5420 authors contributed to 729 selected articles related to GBM metabolism. Among all authors, Mischel PS emerged as the most prolific author with 14 publications (1.92% of all publications), closely followed by Cloughesy TF (11, 1.50%) and Siegelin MD (11, 1.50%), with Chinnaiyan P (10, 1.37%), Karpel- Massler G (10, 1.37%) and Westhoff MA (10, 1.37%) tied for fourth. The top 10 authors with the most publications are shown in Table 4. The ten most prolific authors published 102 papers, representing 14.0% of the total number of papers. In addition, the H-Index of these authors is greater than or equal to 7.
In terms of citations, the authors with the highest number of citations are presented in Table 4. Guo DL received the highest number of citations (104), followed by Chakravarti A (100 citations), Stoll EA (93 citations), Geng F (91 citations) and Nakano I (90 citations). Except for Stoll EA (The author has only published two articles in this field), the H-Index of these authors is equal to or greater than 5. The successful completion of a research project often depends on the collaboration of several researchers. By analyzing in-depth collaborative networks between authors, we can identify key researchers in a given academic field and assess how closely they work together. Figure 4 presents a visual representation of the author collaboration network. Figure 4A focuses on the 266 researchers who have published more than three papers, while Figure 4B depicts the collaboration graph of 168 authors who have been cited more than 20 times. Node and font size are positively correlated with the number of published articles, and the thickness of the connecting lines represents the density of collaboration between authors. Our findings indicate that these prolific authors were primarily from countries or organizations that were highly prolific and had established close collaborative networks between them.
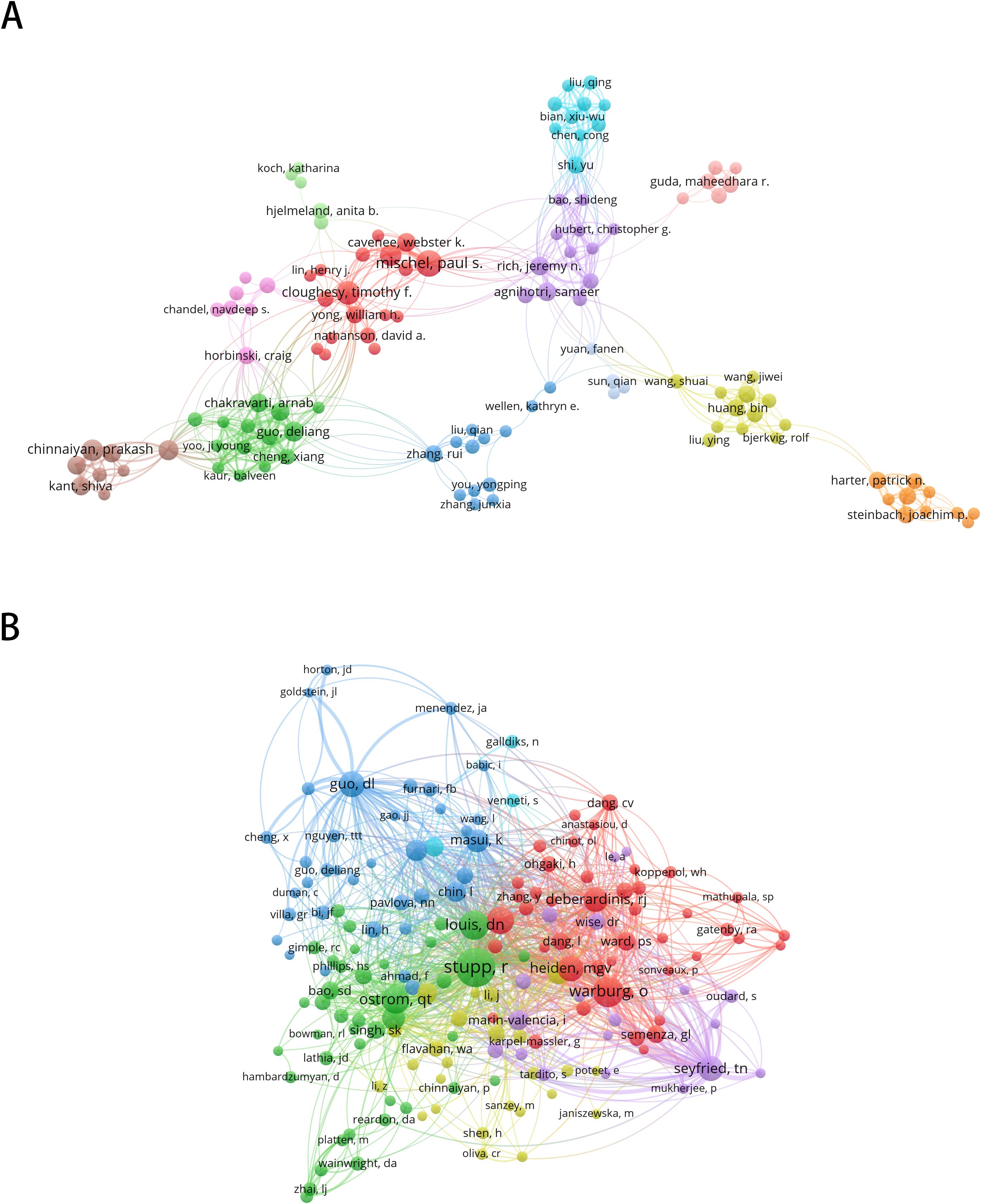
Figure 4. Visual map of the author collaboration network. (A) Collaboration graph of 266 authors who have published more than 3 papers. (B) Collaboration graph of 168 authors who have been cited more than 20 times. Node and font size are positively correlated with the number of published articles, and the thickness of the connecting line represents the density of collaboration between authors.
3.4 Number of journal publications and journal impact analysis
The value of journal research lies in the identification of research hotspots and disciplinary branches in a particular field, as well as in revealing the layout of key journals in the field, providing indispensable reference materials for academic researchers. The Impact Factor (IF) is a key quantitative metric employed to assess the academic importance of a journal and the quality of its papers. Furthermore, the Impact Factor is employed as a gauge of a journal’s practical applicability and as a means of demonstrating its academic impact. The concept was first proposed in 1972 by Eugene Garfield, the founder of the Institute for Scientific Information (ISI) in the United States, and has since become an important evaluation tool in international academic publishing. In this study, we conducted a meticulous selection and inclusion of 729 articles from 285 different journals. With the assistance of a bibliometric online analysis platform, we were able to provide insights into the impact of these journals. Specifically, Table 5 demonstrates the top ten journals in terms of the total number of articles published and their impact on academia, while Table 6 reveals the top ten journals in terms of the total number of citations and their impact on academia. The data presented here provide valuable insights into the academic standing and influence of the journals in question. The International Journal of Molecular Sciences was the journal with the highest number of articles published, with a total of 37 articles. This was followed by Cancers (34) and Oncotarget (31). In terms of the number of citations, Neuro-Oncology emerged as the most cited journal, with a total of 218 citations. This was followed by Oncotarget (85 citations) and Clinical Cancer Research (81 citations). Furthermore, the Cell journal had the highest average number of citations per article (51), followed by Nature Cell Biology (44), Embo Journal (21) and Nature Neuroscience (21). The H-Index of these journals is greater than or equal to 9. In our study, we identified several journals that have a high impact on the academic world. These journals demonstrated a high level of productivity, with a significant number of articles published and citations received. These results provide important insights into the status and influence of journals in academia.
3.5 Co-citation analysis of reference
A total of 31,358 references were cited in the 729 articles selected for this search. The 10 most frequently cited references have been listed in detail in Table 7. These highly cited articles were published in high-quality journals: New England Journal of Medicine (IF=96.2), Nature (IF=50.5), Cell (IF=45.5), Science (IF=44.7) and Lancet Oncology (IF=41.6) and Cancer Cell (IF=48.8). The 2005 publication by Roger Stupp’s team of “Radiotherapy plus concomitant and adjuvant temozolomide for glioblastoma” is the document most frequently cited in the field, with a total of 2,324 citations.
A network analysis of publication clusters for GBM metabolism-related research between 2014 and 2024 is presented in Figure 5. Figure 5A illustrates the CiteSpace visualization analysis of the clustered network of co-cited literature. The top 13 largest citation clusters are presented. The number of cluster labels is inversely proportional to the number of articles in each cluster. The greater the number of yellow clusters, the more frequently the references within them have been cited in recent years. The fields of immunotherapy and fatty acids have become a key focus in recent years. Figure 5B presents a timeline view of the co-cited references related to GBM metabolism. Each circle represents a major cited reference in the cluster, while the size of the circle on the timeline indicates the frequency of citations. Links indicate the co-citation relationship and the colors of the nodes and lines represent the different citation years. Cancer, tumor metabolism and morc2 appeared earlier in the field, while immunotherapy has been a hot research topic in recent years.
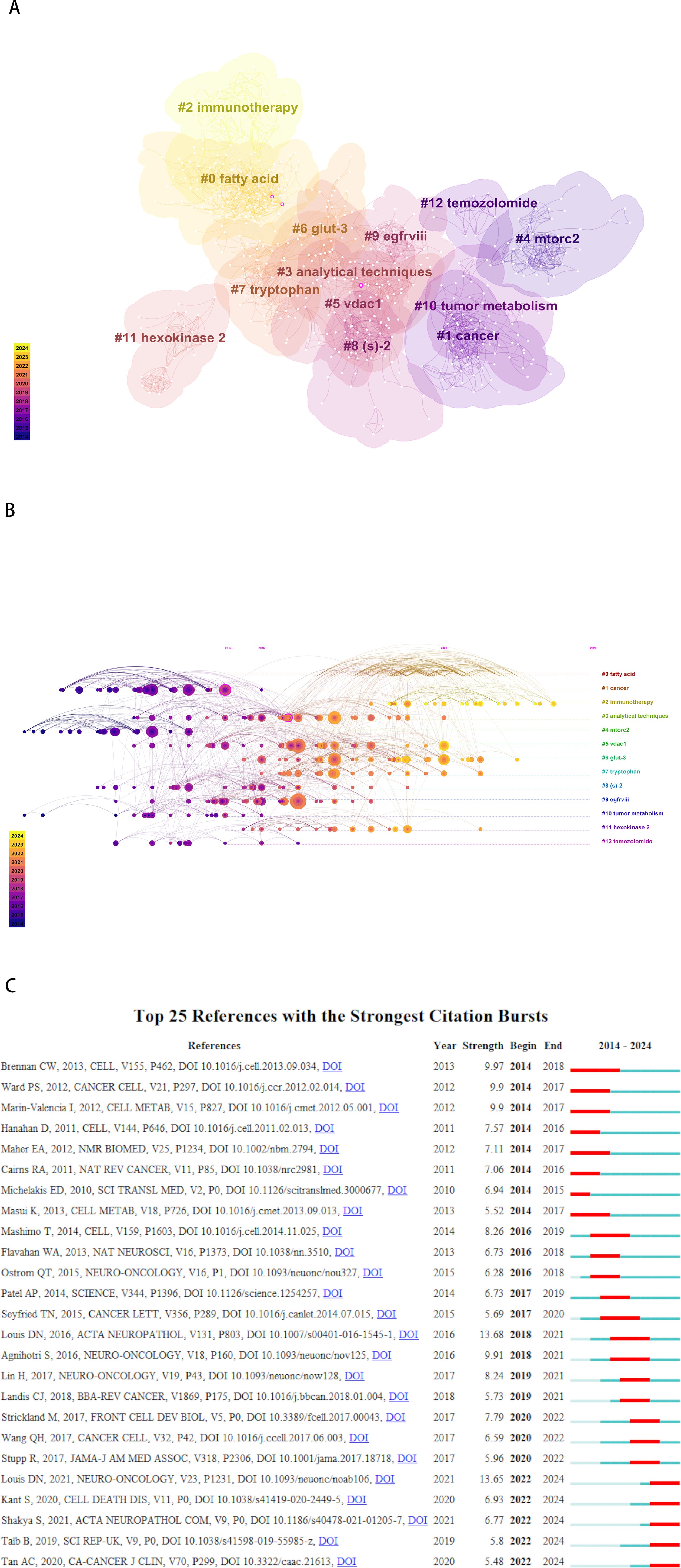
Figure 5. A cluster network analysis of GBM metabolism research publications between 2014 and 2024. (A) A visual analysis of the co-citation literature clustering network. The top 13 largest citation clusters are presented. The greater the yellow, the more frequently the references in these clusters have been cited in recent years. (B) Timeline view of co-citation references. Each horizontal line represents a cluster, and the smaller the label number is, the larger the cluster the node size reflects the co-citation frequency, while the links represent the co-citation relationship. The colors of the nodes and lines represent the different years of citation. (C) The top 25 most cited references.
A burst of citation frequency is defined as a sudden and significant increase in the number of citations a document receives over a period, which is higher than the average for that document. Such analysis can assist in comprehending the alterations in research focus over time and investigating the prevailing trends and research interests within the research domain. Figure 5C illustrates the 25 most cited references over the period between 2014 and 2024. The red bars represent high citation frequencies, while the blue bars represent lower citation frequencies. Among the most cited articles, the reference with the strongest citation burst intensity (intensity = 13.68, burst period = 2018-2021) is the article published by Louis DN et al. in 2016 in Acta Neuropathologica under the title ‘The 2016 World Health Organization Classification of Tumors of the Central Nervous System: a summary’, which ranked 8th among the most cited articles.
3.6 Keyword visual analysis
The co-occurring keyword network and overlay visualization are presented in Figure 6. The ten most frequent keywords were as follows: “glioblastoma”, “metabolism”, “glioma”, “glycolysis”, “glioblastoma multiforme”, “hypoxia”, “Warburg effect”, “tumor microenvironment”, “cancer” and “cancer metabolism”. Figure 6A shows the keywords divided into 13 clusters. The largest cluster, which is red, is related to the metabolic reprogramming of brain tumor cells, including “brain cancer”, “lipids”, “mass spectrometry metabolic reprogram metabolomic” and “oxidative stress”. The second major cluster is orange and is related to lipid metabolism in brain tumors, including “brain tumors”, “cholesterol metabolism”, “lipid metabolism” and “fatty acids”. The third cluster is yellow and is related to mitochondrial metabolism in glioblastoma stem cells. It includes the keywords “cancer stem cells”, “glioblastoma”, “mitochondrial metabolism” and “autophagy”.
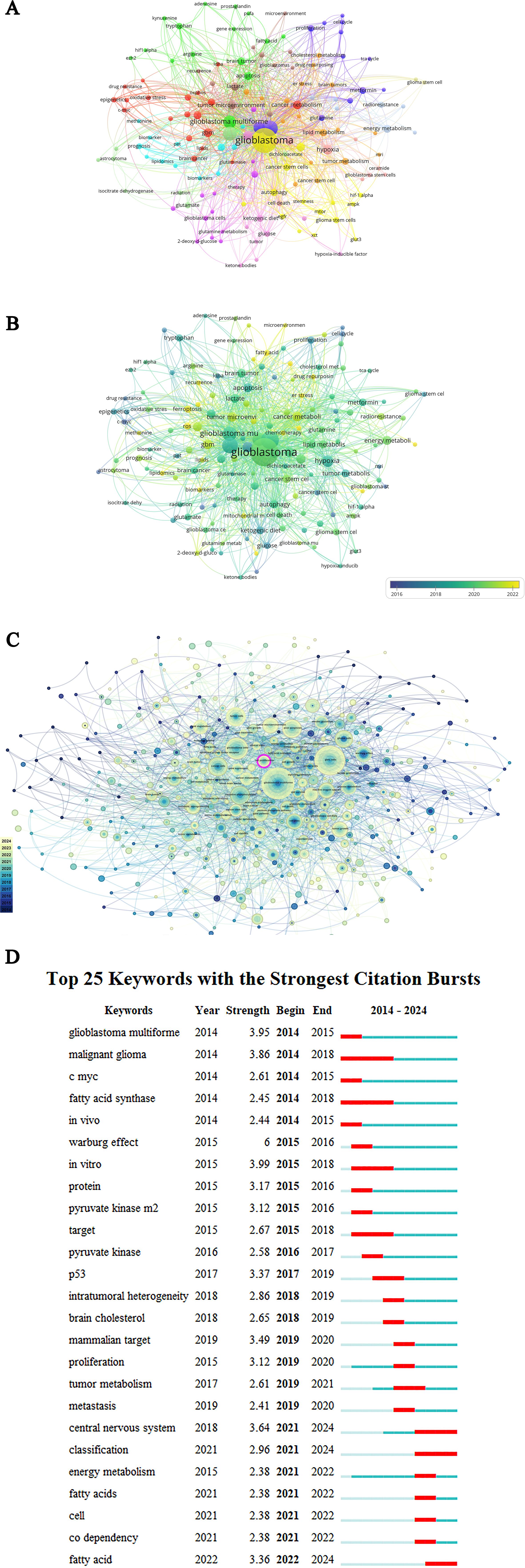
Figure 6. A network visualization of keyword/hot topics co-occurrence. (A) Network graph of keyword co-occurrence. (B) Time view of keywords. (C) Visualization of popular research topics. (D) The top 25 keywords with the highest citation burst rate.
Figure 6B also shows the evolution of the GBM metabolism research clusters over time. The size of the circle and the font represent the frequency of the word, and the lines indicate the correlation between them; the purple color indicates that the keyword appeared on average in 2016 or even earlier, while the yellow color indicates that the keyword appeared in the last three years after 2022. In GBM metabolism research (2014-2024), the research trend has shifted from the ketogenic diet and Warburg effect to fatty acid metabolism and iron metabolism. In addition, energy metabolism has been a hot direction of much attention in this field.
Keyword Burst refers to the frequency of occurrence of a keyword over a specified period, indicating an elevated level of interest or activity. By calculating the strength of a keyword burst, it is possible to gain insight into the current hotspots and future development trends within a given research field. Researchers can then use this information to inform their research direction and identify valuable sources of information. Figure 6D illustrates the top 25 keywords with the strongest citation bursts. The keywords marked with red bars indicate a sudden surge in the frequency of use of the keyword within that period, while the blue color indicates a relatively less cited time. The “Warburg effect” has the highest outburst intensity (6.00), followed by “in vitro” (3.99), “glioblastoma multiforme” (3.95), “malignant glioma” (3.86) and “central nervous system” (3.64). The central nervous system, classification and fatty acid show strong citation bursts in 2024. In conjunction with Figure 6C, glioblastoma, cell proliferation and fatty acids remain prominent in investigation within GBM metabolism.
3.7 Research discipline analysis
The dual map overlay of journals can be utilized to illustrate the distribution of subjects across academic journals, the evolution of citation trajectories and the relocation of research centers (41). Research on GBM metabolism encompasses a multitude of disciplines and fields. Figure 7 presents a dual-map overlay of journals on GBM metabolism. The clusters on the left represent the collection of citing journals, which serve to illustrate the current state of knowledge; the clusters on the right represent the collection of cited journals, which demonstrate the knowledge base that is currently being cited by the frontier of knowledge. The length of the ellipse is indicative of the number of authors, while the width is indicative of the number of publications. The curve connecting the two sides represents the connection between citations. The use of different colors represents the distinction between subject areas. The greater the thickness of the curve, the stronger the connection. As illustrated in the figure, the citing journals are predominantly distributed across subjects #2 (Medicine, Medical, Clinical) and #4 (Molecular, Biology, Immunology), while the cited journals are primarily concentrated in subject #8 (Molecular, Biology, Genetics). Additionally, a subset of the cited journals are also distributed across subjects #2 (Environmental, Toxicology, Nutrition), #4 (Chemistry, Materials, Physics), #5 (Health, Nursing, Medicine) and #7 (Psychology, Education, Social). The literature in the disciplines of Molecular, Biology and Immunology is significantly affected by the disciplines of Molecular, Biology and Genetics (z = 7.05, f = 9902).
4 Discussion
Data from 729 articles on GBM metabolism screened in Web of Science from 2014 to 2024 were analyzed using CiteSpace 6.3.R2 Advanced, VOSviewer 1.6.20, and Microsoft Office Excel 2016. Based on data, we assessed country/institution distribution, author contributions, core articles and research hotspots.
4.1 General distribution
The analysis of this study is based on 729 articles on glioblastoma metabolism from 245 countries, 3,314 institutions, and 5,420 authors, published between 1 January 2014 and 30 April 2024 in the Web of Science Core Collection (WoSCC) database. The rapid increase in the number of articles indicates that GBM metabolism is receiving more and more attention. In 1920, the German biologist Otto Warburg proposed the ‘Warburg effect’, which states that under normal, aerobic conditions, most healthy cells completely oxidize glucose in the mitochondria through the tricarboxylic acid cycle and the electron transport chain, thereby obtaining the maximum amount of energy (ATP). In contrast, cancer cells rely primarily on glycolysis for energy production even in the presence of sufficient oxygen (42–44). Since that time, the scientific community has initiated studies related to the metabolism of tumor cells. The number of studies related to GBM metabolism has increased steadily over the past decade. The year with the highest number of publications, 2022, will have approximately four times as many publications as 2014.
In terms of the most productive countries, the United States was the most prolific, followed by China, Germany, Italy, the United Kingdom, Japan and France, all of which published more than 30 articles (Figure 2). The top three countries with the highest number of published articles were the United States, China and Germany, which collectively published 535 articles, accounting for 73.39 percent of all articles. Furthermore, the top three countries with the highest frequency of collaboration were the United States, Germany and Spain. The countries with the highest number of article citations are the United States, China, Germany, the United Kingdom, France, Japan and Canada, all of which have been cited more than 1,000 times (Figure 2). The above findings demonstrate the significant contribution and leading position of the United States, China and Germany in the research of GBM metabolism. This may be attributed to their adequate research funding, advanced research infrastructure, and well-established academic publishing and evaluation system, which attracts outstanding scholars from all over the world to pursue further studies in the region. In terms of the most productive research institutions, the University of California System is at the pinnacle of the list, followed by the University of Texas System, the Helmholtz Association, the German Cancer Research Center (DKFZ), Institut National de la Sante et de la Recherche Medicale (Inserm) and the University System of Ohio, all of which have published more than 20 publications (Figure 2).
In terms of the most influential journals and authors, the International Journal of Molecular Sciences (37 articles) and Cancers, Oncotarget and Neuro-Oncology (all published more than 20 articles) are the most prominent (Table 5). The Oncology journal published 25 articles that received the most citations (218 citations), followed by Oncotarget (85 citations) and Clinical Cancer Research (81 citations). The Journal Citation Reports (JCR) is one of the most common metrics used to assess the impact of journals. It divides all journals into four quartiles (Q1-Q4) based on their impact factor. Of the top ten most cited journals, Q1 journals account for 50 percent (Table 6). As illustrated in Table 7, the majority of the most highly cited articles were published in journals with high impact factors. The high impact factor of a journal is often indicative of the quality of the research published therein, which has typically undergone rigorous peer review. This is a crucial factor in the results of our bibliometric analysis. Journals with high impact factors typically exert greater academic influence and can attract a larger readership and a greater number of citations, thereby exerting a more significant impact on the field of GBM metabolism-related research. To illustrate, the New England Journal of Medicine has an impact factor of 96.2, which evinces its preeminent status within the domain of biomedicine. Consequently, research findings about GBM metabolism published in this journal receive heightened attention and citations.
Mischel PS is one of the most prolific authors in the field, with 14 publications and 85 citations, which attests to his significant impact in the field of glioblastoma metabolism. Other distinguished authors include Cloughesy TF and Siegelin MD On the other hand, Guo DL ranks first in terms of citation rankings, having been cited 104 times. Other authors who have been cited more than 90 times include Chakravarti A., Stoll EA, Geng F and Nakano I. These authors and their teams have made significant contributions to the field of GBM metabolism. The results of their valuable research have provided us with important references for our future research in this field.
In 2005, physicist Jorge E. Hirsch proposed the concept of the H-index (45), which is a concise and effective indicator for measuring the quality and influence of the research results of scientists, academic institutions, or countries. It is based on the number of papers published by researchers and the number of citations of these papers, to comprehensively reflect the productivity and academic influence of researchers. At the national level, the United States, China, Germany and Japan all have relatively high H-Indexes, indicating that the scientific research results of these countries are not only substantial in number, but also exert a considerable academic influence on the scientific research field of GBM metabolism. In particular, they have exerted a powerful, autonomous, and unavoidable influence in high-quality, widely cited fields. At the institutional level, the H-Index of the University of California System, the University of Texas System and the University of Texas System are among the highest. Despite the relatively limited number of publications, Harvard University’s H-Index remains notably high, suggesting that the impact of its research output is concentrated in a small number of high-quality papers. The research conducted by Harvard University is often more refined, and its high-level scientific research results frequently exert an inevitable impact on core areas. At the author level, Mischel PS is one of the authors with the highest number of published papers and is also ranked highly among the most highly cited authors. The high H-Index reflects his sustained contributions to the field of GBM metabolism and the inevitable impact of these contributions within the literature network. While Guo DL’s number of published papers is not among the top ten, his relatively high H-Index suggests that the quality and citation rate of his papers in the field of GBM metabolism are comparatively high.
Concerning subject areas, the dual-map overlay of journals demonstrates the current state of knowledge flow and interdisciplinary collaboration. The most recent research findings on GBM metabolism are primarily disseminated in journals belonging to the following disciplines: medicine, clinical medicine, molecular science, biology, immunology and other related fields. Conversely, research findings published in molecular science, biology and genetics, as well as health, nursing, medicine, psychology, education and sociology provide a robust foundation for contemporary research endeavors. Research on GBM metabolism is inherently multidisciplinary and cross-disciplinary. The close communication and mutual influence between different disciplines not only introduce new ideas and new methods into this field but also provide impetus for in-depth research and breakthroughs. The advancement of fundamental science (such as molecular science, biology, genetics, etc.) furnishes theoretical substantiation for comprehending the pertinent mechanisms of GBM metabolism. Meanwhile, applied disciplines, such as medicine and clinical medicine, to a certain extent, are capable of transforming these fundamental research outcomes into clinical practice. It is this interdisciplinary collaboration and therapeutic integration that has driven the continuous advancement of GBM metabolic research and provided solutions for future targeted and precise treatments.
4.2 Hotspots and Frontiers
The selection of keywords is a crucial aspect of literature management and scholarly communication. These words are carefully chosen for their ease of retrieval and comprehension of the core content of a paper, and can succinctly reflect the core theme and discussion points of the paper. Through in-depth analysis of keywords, it is possible to summarize the research focus of a particular subject area, identify current research hotspots and possible future development trends, and assist academics in tracking the dynamics of the discipline and advancing knowledge. In our study, the keywords that appear more frequently are “glioblastoma”, “metabolism”, “glycolysis”, “hypoxia”, “Warburg effect”, “cancer metabolism”, “mitochondria”, “tumor microenvironment”, “energy metabolism” and “metabolic reprogramming”. These keywords are mainly related to GBM cellular glucose metabolism, suggesting that they are some of the more popular topics in the last decade. In recent years, several new keywords have emerged in the field of cancer research, including “Fatty acids”, “mitochondrial metabolism”, “iron metabolism”, “tumor microenvironment”, “metabolism”, “tumor heterogeneity”, “cancer metabolism”, “immunotherapy”, “arginine” and “cholesterol”, which are mainly focused on the metabolism of lipids and the heterogeneity of GBM cells. This suggests that the above keywords have become a new and popular topic in recent years.
As is the case with other types of tumor cells, GBM cells also demonstrate a significant Warburg effect. Even in an aerobic environment, they continue to obtain energy through glycolysis rather than through the more efficient oxidative phosphorylation pathway (13). This metabolic mode allows tumor cells to obtain the energy and metabolites necessary for growth rapidly. This significant difference in sugar metabolism between GBM cells and normal cells has initiated a new research direction for researchers. At present, a considerable number of scientific research teams have initiated research in this area, and have achieved notable scientific research results. For instance, the research team led by Zhenxing Zhang discovered that the protein DHHC9 stimulates glycolysis in GBM cells, thereby promoting their proliferation and tumorigenesis, which is strongly correlated with the patient’s prognosis, indicating that it has significant potential as a therapeutic target and prognostic indicator for abnormal glucose metabolism in GBM cells (21). Rui Yang and his team demonstrated that the transcription factor homeobox A3 (HOXA3) promotes aerobic glycolysis in GBM cells by activating the transcription of lysine-specific demethylase KDM6A, thereby promoting tumor growth (22).
In recent years, abnormalities in lipid and iron metabolism have emerged as a significant area of concern for researchers in the field of GBM research, with the potential to become new therapeutic targets. Compared with normal cells, GBM cells exhibit aberrant lipid metabolism, which enables them to meet the energy demands of tumor cells (14, 46, 47). Ferroptosis is a form of programmed cell death that arises from the accumulation of iron-dependent lipid peroxides within cells, which is involved in the malignant progression of glioblastoma (48). When intracellular iron levels increase, polyunsaturated fatty acids on the cell membrane are oxidized to produce lipid peroxides, which in turn leads to cell membrane rupture and cell death (49, 50). Jenna K Minami’s team found that knocking down CDKN2A can promote lipid peroxidation in GBM cells, selectively induce tumor ferroptosis, and thereby inhibit the malignant progression of glioblastoma (13). Yang Jiang and colleagues demonstrated that circLRFN5 can facilitate ferroptosis by binding to PRRX2 protein and inducing its degradation, thereby inhibiting the growth of glioblastoma (48). Xiang Cheng and colleagues demonstrated that elevated diacylglycerol acyltransferase 1 (DGAT1) facilitates the storage of surplus fatty acids as triglycerides and lipid droplets, thereby safeguarding GBM from oxidative stress and cell death. Consequently, it can be postulated that the inhibition of DGAT1 represents an efficacious strategy for the limitation of GBM development (11).
The field of glioblastoma metabolism is attracting increasing attention from scholars, which indicates that this field has considerable potential for the diagnosis and treatment of GBM. To achieve this, it is necessary to conduct further research into the mechanisms underlying the abnormal metabolism of glioblastoma cells, and it is expected to provide important references for the diagnosis and treatment of other malignant tumors.
4.3 Challenges and prospects
The field of GBM metabolic reprogramming has emerged as a prominent area of interest and a focal point for numerous researchers, suggesting that it holds significant promise in the diagnosis and treatment of GBM. Nevertheless, the process of studying tumor cell metabolic reprogramming presents several significant challenges. Firstly, as cancer progresses, the metabolic phenotype of cancer cells undergoes a corresponding change (51), leading to drug resistance during targeted drug therapy and consequently affecting the patient’s prognosis. Secondly, Secondly, The development of targeted drugs related to metabolism necessitates a robust foundation in medicinal chemistry, structural biology, pharmacokinetics and pharmacodynamics. This represents a significant challenge for researchers, necessitating close collaboration between researchers from diverse disciplines. Furthermore, species-specific differences may result in disparate outcomes when developing targeted drugs, from the animal testing stage to the clinical trial stage. This may, in turn, complicate the evaluation of the side effects of targeted drugs that are designed to target the metabolism of human GBM cells (52). Ultimately, the technical intricacy and high expense associated with metabolomics have largely constrained its extensive implementation in clinical research. Nevertheless, metabolic reprogramming is of vital importance in the field of cancer research. Its influence extends beyond the mere occurrence and development of tumors, offering new avenues for therapeutic intervention. In the future, methods for evaluating and quantifying the metabolic phenotype of human GBM will continue to evolve, including metabolomics, isotope tracing studies and metabolic imaging (28, 53, 54). Concurrently, the integration of multi-omics data and AI-assisted analysis technology, metabolic dynamic labeling and real-time metabolic monitoring technology, and the combination of metabolism and immunotherapy (27, 52, 55, 56) will facilitate a more profound comprehension of the mechanism of GBM metabolic reprogramming. This, in turn, will contribute to the development of more precise and efficacious treatment strategies, the incorporation of precision medicine into the comprehensive treatment regimen of GBM patients, and the provision of more targeted treatment options to GBM patients, while simultaneously reducing adverse effects and enhancing efficacy.
4.4 Limitations
It should be noted that our study is not without limitations. Firstly, the data used in this study were sourced exclusively from the WoSCC database. Despite the extensive and comprehensive nature of the WoSCC database, which is the most commonly used source of publications in bibliometric analyses, there may be relevant literature that is not included in it. Secondly, the articles included in the study were those included in WoSCC from 1 January 2014 to 31 April 2024. This may have resulted in the overlooking of important research that was published before or after this period. It should be noted that the database is continuously updated. Only articles published in English were selected, which may have resulted in the omission of research results in non-English languages. Furthermore, the bibliometric analyses may have been influenced by temporal bias. It is typical for papers published earlier to accumulate more citations. A variety of other factors, such as self-citations, may also have an impact on citation rates. Finally, the article screening process, in which the researchers manually screened for literature that was not relevant to the topic, is open to subjective interpretation and may lead to selection bias.
5 Conclusion
This article presents a comprehensive examination of the current status and development trends in the field of glioblastoma metabolism research through bibliometric analysis methods. The findings indicated that the metabolic mechanism of GBM plays a pivotal role in tumor occurrence and development, and has emerged as a significant research focus in recent years. Our analysis identified key areas and cutting-edge research directions in metabolic research, including tumor metabolic reprogramming, the tumor microenvironment, lipid metabolism, and the role of metabolic pathways in treatment resistance. As technology continues to evolve and our understanding of tumor metabolism deepens, it is anticipated that further breakthroughs will be made in the field of GBM metabolism research. It provides a valuable reference point for future GBM metabolic research and establishes the foundation for the development of new metabolic-targeted therapeutic strategies. By deepening our comprehension of GBM metabolism, we aspire to identify more efficacious treatments. Continued, comprehensive investigation of this field will foster new prospects for enhancing patient prognoses and treatment outcomes.
Data availability statement
The original contributions presented in the study are included in the article/supplementary material. Further inquiries can be directed to the corresponding author.
Author contributions
JD: Conceptualization, Data curation, Investigation, Methodology, Software, Writing – original draft, Writing – review & editing. SS: Conceptualization, Methodology, Software, Writing – review & editing. PC: Writing – review & editing. QH: Writing – review & editing. HD: Funding acquisition, Project administration, Resources, Supervision, Writing – review & editing.
Funding
The author(s) declare financial support was received for the research, authorship, and/or publication of this article. This work was supported by the National Clinical Key Specialty Project Foundation (2023011), Shanxi Provincial Key Research and Development Project (2022-188), International Science and Technology of Cooperation Program Shanxi Province (202204041101007) and Shanxi Provincial Basic Research Program (20210302124399, 20210302123247).
Conflict of interest
The authors declare that the research was conducted in the absence of any commercial or financial relationships that could be construed as a potential conflict of interest.
Publisher’s note
All claims expressed in this article are solely those of the authors and do not necessarily represent those of their affiliated organizations, or those of the publisher, the editors and the reviewers. Any product that may be evaluated in this article, or claim that may be made by its manufacturer, is not guaranteed or endorsed by the publisher.
References
1. Verdugo E, Puerto I, Medina MÁChecktae. An update on the molecular biology of glioblastoma, with clinical implications and progress in its treatment. Cancer Commun (Lond). (2022) 42:1083–111. doi: 10.1002/cac2.12361
2. Rybin MJ, Ivan ME, Ayad NG, Zeier Z. Organoid models of glioblastoma and their role in drug discovery. Front Cell Neurosci. (2021) 15:605255. doi: 10.3389/fncel.2021.605255
3. Sun X, Klingbeil O, Lu B, Wu C, Ballon C, Ouyang M, et al. BRD8 maintains glioblastoma by epigenetic reprogramming of the p53 network. Nature. (2023) 613:195–202. doi: 10.1038/s41586-022-05551-x
4. Yang E, Wang L, Jin W, Liu X, Wang Q, Wu Y, et al. PTRF/Cavin-1 enhances chemo-resistance and promotes temozolomide efflux through extracellular vesicles in glioblastoma. Theranostics. (2022) 12:4330–47. doi: 10.7150/thno.71763
5. Wu W, Klockow JL, Zhang M, Lafortune F, Chang E, Jin L, et al. Glioblastoma multiforme (GBM): An overview of current therapies and mechanisms of resistance. Pharmacol Res. (2021) :171:105780. doi: 10.1016/j.phrs.2021.105780
6. Kumthekar P, Ko CH, Paunesku T, Dixit K, Sonabend AM, Bloch O, et al. A first-in-human phase 0 clinical study of RNA interference-based spherical nucleic acids in patients with recurrent glioblastoma. Sci Transl Med. (2021) 13:eabb3945. doi: 10.1126/scitranslmed.abb3945
7. Ballestín A, Armocida D, Ribecco V, Seano G. Peritumoral brain zone in glioblastoma: biological, clinical and mechanical features. Front Immunol. (2024) 15:1347877. doi: 10.3389/fimmu.2024.1347877
8. Barba I, Carrillo-Bosch L, Seoane J. Targeting the warburg effect in cancer: where do we stand? Int J Mol Sci. (2024) 25:3142. doi: 10.3390/ijms25063142
9. Fukushi A, Kim HD, Chang YC, Kim CH. Revisited metabolic control and reprogramming cancers by means of the warburg effect in tumor cells. Int J Mol Sci. (2022) 23:10037. doi: 10.3390/ijms231710037
10. Cui X, Zhao J, Li G, Yang C, Yang S, Zhan Q, et al. Blockage of EGFR/AKT and mevalonate pathways synergize the antitumor effect of temozolomide by reprogramming energy metabolism in glioblastoma. Cancer Commun (Lond). (2023) 43:1326–53. doi: 10.1002/cac2.12502
11. Cheng X, Geng F, Pan M, Wu X, Zhong Y, Wang C, et al. Targeting DGAT1 ameliorates glioblastoma by increasing fat catabolism and oxidative stress. Cell Metab. (2020) 32:229–42. doi: 10.1016/j.cmet.2020.06.002
12. Wang S, Yan W, Kong L, Zuo S, Wu J, Zhu C, et al. Oncolytic viruses engineered to enforce cholesterol efflux restore tumor-associated macrophage phagocytosis and anti-tumor immunity in glioblastoma. Nat Commun. (2023) 14:4367. doi: 10.1038/s41467-023-39683-z
13. Minami JK, Morrow D, Bayley NA, Fernandez EG, Salinas JJ, Tse C, et al. CDKN2A deletion remodels lipid metabolism to prime glioblastoma for ferroptosis. Cancer Cell. (2023) 41:1048–1060.e9. doi: 10.1016/j.ccell.2023.05.001
14. Shakya S, Gromovsky AD, Hale JS, Knudsen AM, Prager B, Wallace LC, et al. Altered lipid metabolism marks glioblastoma stem and non-stem cells in separate tumor niches. Acta Neuropathol Commun. (2021) 9:101. doi: 10.1186/s40478-021-01205-7
15. Wu X, Yuan H, Wu Q, Gao Y, Duan T, Yang K, et al. Threonine fuels glioblastoma through YRDC-mediated codon-biased translational reprogramming. Nat Cancer. (2024) 5:1024–44. doi: 10.1038/s43018-024-00748-7
16. Yuan H, Wu X, Wu Q, Chatoff A, Megill E, Gao J, et al. Lysine catabolism reprograms tumour immunity through histone crotonylation. Nature. (2023) 617:818–26. doi: 10.1038/s41586-023-06061-0
17. Chisari A, Golán I, Campisano S, Gélabert C, Moustakas A, Sancho P, et al. Glucose and amino acid metabolic dependencies linked to stemness and metastasis in different aggressive cancer types. Front Pharmacol. (2021) 12:723798. doi: 10.3389/fphar.2021.723798
18. Sowers ML, Sowers LC. Glioblastoma and methionine addiction. Int J Mol Sci. (2022) 23:7156. doi: 10.3390/ijms23137156
19. Zhou W, Yao Y, Scott AJ, Wilder-Romans K, Dresser JJ, Werner CK, et al. Purine metabolism regulates DNA repair and therapy resistance in glioblastoma. Nat Commun. (2020) 11:3811. doi: 10.1038/s41467-020-17512-x
20. Guo D, Tong Y, Jiang X, Meng Y, Jiang H, Du L, et al. Aerobic glycolysis promotes tumor immune evasion by hexokinase2-mediated phosphorylation of IκBα. Cell Metab. (2022) 34:1312–24. doi: 10.1016/j.cmet.2022.08.002
21. Zhang Z, Li X, Yang F, Chen C, Liu P, Ren Y, et al. DHHC9-mediated GLUT1 S-palmitoylation promotes glioblastoma glycolysis and tumorigenesis. Nat Commun. (2021) 12:5872. doi: 10.1038/s41467-021-26180-4
22. Yang R, Zhang G, Dong Z, Wang S, Li Y, Lian F, et al. Homeobox A3 and KDM6A cooperate in transcriptional control of aerobic glycolysis and glioblastoma progression. Neuro Oncol. (2023) 25:635–47. doi: 10.1093/neuonc/noac231
23. Bailleul J, Ruan Y, Abdulrahman L, Scott AJ, Yazal T, Sung D, et al. M2 isoform of pyruvate kinase rewires glucose metabolism during radiation therapy to promote an antioxidant response and glioblastoma radioresistance. Neuro Oncol. (2023) 25:1989–2000. doi: 10.1093/neuonc/noad103
24. Khan F, Lin Y, Ali H, Pang L, Dunterman M, Hsu WH, et al. Lactate dehydrogenase A regulates tumor-macrophage symbiosis to promote glioblastoma progression. Nat Commun. (2024) 15:1987. doi: 10.1038/s41467-024-46193-z
25. Khan F, Pang L, Dunterman M, Lesniak MS, Heimberger AB, Chen P. Macrophages and microglia in glioblastoma: heterogeneity, plasticity, and therapy. J Clin Invest. (2023) 133:e163446. doi: 10.1172/JCI163446
26. Bikfalvi A, da Costa CA, Avril T, Barnier JV, Bauchet L, Brisson L, et al. Challenges in glioblastoma research: focus on the tumor microenvironment. Trends Cancer. (2023) 9:9–27. doi: 10.1016/j.trecan.2022.09.005
27. Pun FW, Leung GHD, Leung HW, Rice J, Schmauck-Medina T, Lautrup S, et al. A comprehensive AI-driven analysis of large-scale omic datasets reveals novel dual-purpose targets for the treatment of cancer and aging. Aging Cell. (2023) 22:e14017. doi: 10.1111/acel.14017
28. Liu Y, Zhou Q, Song S, Tang S. Integrating metabolic reprogramming and metabolic imaging to predict breast cancer therapeutic responses. Trends Endocrinol Metab. (2021) 32:762–75. doi: 10.1016/j.tem.2021.07.001
29. Adir O, Poley M, Chen G, Froim S, Krinsky N, Shklover J, et al. Integrating artificial intelligence and nanotechnology for precision cancer medicine. Adv Mater. (2020) 32:e1901989. doi: 10.1002/adma.201901989
30. Miao L, Zhang J, Zhang Z, Wang S, Tang F, Teng M, et al. A bibliometric and knowledge-map analysis of CAR-T cells from 2009 to 2021. Front Immunol. (2022) 13:840956. doi: 10.3389/fimmu.2022.840956
31. Xing Y, Yasinjan F, Cui J, Peng Y, He M, Liu W, et al. Advancements and current trends in tumor treating fields: a scientometric analysis. Int J Surg. (2024) 110:2978–91. doi: 10.1097/JS9.0000000000001151
32. Zhang HY, Yu HY, Zhao GX, Jiang XZ, Gao G, Wei BJ. Global research trends in immunotherapy for glioma: a comprehensive visualization and bibliometric analysis. Front Endocrinol (Lausanne). (2023) 14:1273634. doi: 10.3389/fendo.2023.1273634
33. Zhang H, Chen Y, Jiang X, Gu Q, Yao J, Wang X, et al. Unveiling the landscape of cytokine research in glioma immunotherapy: a scientometrics analysis. Front Pharmacol. (2023) 14:1333124. doi: 10.3389/fphar.2023.1333124
34. Montes-Escobar K, de la Hoz MJ, Castillo-Cordova P, Duran-Ospina JP, Bravo-Saltos RK, Lapo-Talledo GJ, et al. Glioblastoma: a comprehensive approach combining bibliometric analysis, Latent Dirichlet Allocation, and HJ-Biplot: Glioblastoma insights and trends: a 49-year bibliometric analysis. Neurosurg Rev. (2024) 47:209. doi: 10.1007/s10143-024-02440-x
35. Akmal M, Hasnain N, Rehan A, Iqbal U, Hashmi S, Fatima K, et al. Glioblastome multiforme: A bibliometric analysis. World Neurosurg. (2020) 136:270–82. doi: 10.1016/j.wneu.2020.01.027
36. Du X, Chen C, Xiao Y, Cui Y, Yang L, Li X, et al. Research on application of tumor treating fields in glioblastoma: A bibliometric and visual analysis. Front Oncol. (2022) 12:1055366. doi: 10.3389/fonc.2022.1055366
37. Łaba AE, Ziółkowski P. Trends in glioblastoma treatment research: an analysis of clinical trials and literature. Neurol Neurochir Pol. (2021) 55:269–80. doi: 10.5603/PJNNS.a2021.0024
38. Zhong D, Li Y, Huang Y, Hong X, Li J, Jin R. Molecular mechanisms of exercise on cancer: A bibliometrics study and visualization analysis via citeSpace. Front Mol Biosci. (2021) 8:797902. doi: 10.3389/fmolb.2021.797902
39. Liu S, Sun YP, Gao XL, Sui Y. Knowledge domain and emerging trends in Alzheimer’s disease: a scientometric review based on CiteSpace analysis. Neural Regener Res. (2019) 14:1643–50. doi: 10.4103/1673-5374.255995
40. van Eck NJ, Waltman L. Software survey: VOSviewer, a computer program for bibliometric mapping. Scientometrics. (2010) 84:523–38. doi: 10.1007/s11192-009-0146-3
41. Wan Y, Shen J, Ouyang J, Dong P, Hong Y, Liang L, et al. Bibliometric and visual analysis of neutrophil extracellular traps from 2004 to 2022. Front Immunol. (2022) 13:1025861. doi: 10.3389/fimmu.2022.1025861
42. Liberti MV, Locasale JW. The warburg effect: how does it benefit cancer cells? Trends Biochem Sci. (2016) 41:211–8. doi: 10.1016/j.tibs.2015.12.001
43. Martins Pinto M, Paumard P, Bouchez C, Ransac S, Duvezin-Caubet S, Mazat JP, et al. The Warburg effect and mitochondrial oxidative phosphorylation: Friends or foes? Biochim Biophys Acta Bioenerg. (2023) 1864:148931. doi: 10.1016/j.bbabio.2022.148931
44. Bhattacharya B, Mohd Omar MF, Soong R. The Warburg effect and drug resistance. Br J Pharmacol. (2016) 173:970–9. doi: 10.1111/bph.13422
45. Hirsch JE. An index to quantify an individual’s scientific research output. Proc Natl Acad Sci U S A. (2005) 102:16569–72. doi: 10.1073/pnas.0507655102
46. Lewis CA, Brault C, Peck B, Bensaad K, Griffiths B, Mitter R, et al. SREBP maintains lipid biosynthesis and viability of cancer cells under lipid- and oxygen-deprived conditions and defines a gene signature associated with poor survival in glioblastoma multiforme. Oncogene. (2015) 34:5128–40. doi: 10.1038/onc.2014.439
47. Taïb B, Aboussalah AM, Moniruzzaman M, Chen S, Haughey NJ, Kim SF, et al. Lipid accumulation and oxidation in glioblastoma multiforme. Sci Rep. (2019) 9:19593. doi: 10.1038/s41598-019-55985-z
48. Jiang Y, Zhao J, Li R, Liu Y, Zhou L, Wang C, et al. CircLRFN5 inhibits the progression of glioblastoma via PRRX2/GCH1 mediated ferroptosis. J Exp Clin Cancer Res. (2022) 41:307. doi: 10.1186/s13046-022-02518-8
49. Stockwell BR, Friedmann Angeli JP, Bayir H, Bush AI, Conrad M, Dixon SJ, et al. Ferroptosis: A regulated cell death nexus linking metabolism, redox biology, and disease. Cell. (2017) 171:273–85. doi: 10.1016/j.cell.2017.09.021
50. Dixon SJ, Lemberg KM, Lamprecht MR, Skouta R, Zaitsev EM, Gleason CE, et al. Ferroptosis: an iron-dependent form of nonapoptotic cell death. Cell. (2012) 149:1060–72. doi: 10.1016/j.cell.2012.03.042
51. Faubert B, Solmonson A, DeBerardinis RJ. Metabolic reprogramming and cancer progression. Science. (2020) 368:eaaw5473. doi: 10.1126/science.aaw5473
52. Stine ZE, Schug ZT, Salvino JM, Dang CV. Targeting cancer metabolism in the era of precision oncology. Nat Rev Drug Discovery. (2022) 21:141–62. doi: 10.1038/s41573-021-00339-6
53. Zhu Z, Cai J, Hou W, Xu K, Wu X, Song Y, et al. Microbiome and spatially resolved metabolomics analysis reveal the anticancer role of gut Akkermansia muciniphila by crosstalk with intratumoral microbiota and reprogramming tumoral metabolism in mice. Gut Microbes. (2023) 15:2166700. doi: 10.1080/19490976.2023.2166700
54. You X, Jiang W, Lu W, Zhang H, Yu T, Tian J, et al. Metabolic reprogramming and redox adaptation in sorafenib-resistant leukemia cells: detected by untargeted metabolomics and stable isotope tracing analysis. Cancer Commun (Lond). (2019) 39:17. doi: 10.1186/s40880-019-0362-z
55. Yang F, Xiao Y, Ding JH, Jin X, Ma D, Li DQ, et al. Ferroptosis heterogeneity in triple-negative breast cancer reveals an innovative immunotherapy combination strategy. Cell Metab. (2023) 35:84–100. doi: 10.1016/j.cmet.2022.09.021
Keywords: glioblastoma, metabolize, metabolic reprogramming, tumor microenvironment, bibliometric analysis, hotspots
Citation: Dai J, Song S, Chen P, Huang Q and Duan H (2024) Analyzing research trends in glioblastoma metabolism: a bibliometric review. Front. Immunol. 15:1444305. doi: 10.3389/fimmu.2024.1444305
Received: 12 June 2024; Accepted: 01 October 2024;
Published: 18 October 2024.
Edited by:
Paula Lam, Independent Researcher, Singapore, SingaporeReviewed by:
Mirza Pojskic, University Hospital of Giessen and Marburg, GermanyFatima Khan, Cleveland Clinic, United States
Copyright © 2024 Dai, Song, Chen, Huang and Duan. This is an open-access article distributed under the terms of the Creative Commons Attribution License (CC BY). The use, distribution or reproduction in other forums is permitted, provided the original author(s) and the copyright owner(s) are credited and that the original publication in this journal is cited, in accordance with accepted academic practice. No use, distribution or reproduction is permitted which does not comply with these terms.
*Correspondence: Hubin Duan, aHViaW5kdWFuNjhAMTYzLmNvbQ==