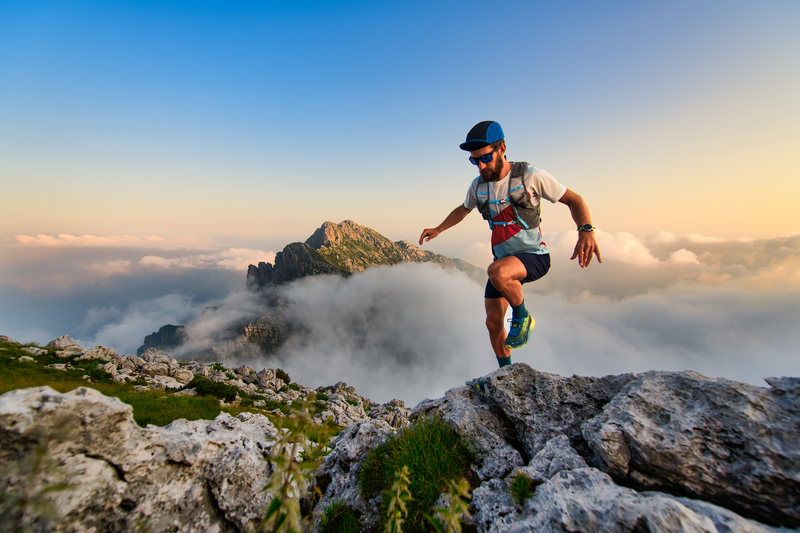
95% of researchers rate our articles as excellent or good
Learn more about the work of our research integrity team to safeguard the quality of each article we publish.
Find out more
ORIGINAL RESEARCH article
Front. Immunol. , 09 September 2024
Sec. Microbial Immunology
Volume 15 - 2024 | https://doi.org/10.3389/fimmu.2024.1438645
This article is part of the Research Topic Biomarkers, Mechanisms, and Treatment Strategies for Epilepsy - Volume II View all 5 articles
Background: Previous studies have suggested that gut microbiota (GM) may be involved in the pathogenesis of epilepsy through the microbiota-gut-brain axis (MGBA). However, the causal relationship between GM and different epilepsy subtypes and whether circulating inflammatory proteins act as mediators to participate in epileptogenesis through the MGBA remain unclear. Therefore, it is necessary to identify specific GM associated with epilepsy and its subtypes and explore their underlying inflammatory mechanisms for risk prediction, personalized treatment, and prognostic monitoring of epilepsy.
Methods: We hypothesized the existence of a pathway GM-inflammatory proteins-epilepsy. We found genetic variants strongly associated with GM, circulating inflammatory proteins, epilepsy and its subtypes, including generalized and partial seizures, from large-scale genome-wide association studies (GWAS) summary data and used Multivariate Mendelian Randomization to explore the causal relationship between the three and whether circulating inflammatory proteins play a mediating role in the pathway from GM to epilepsy, with inverse variance weighted (IVW) method as the primary statistical method, supplemented by four methods: MR-Egger, weighted median estimator (WME), Weighted mode and Simple mode.
Results: 16 positive and three negative causal associations were found between the genetic liability of GM and epilepsy and its subtypes. There were nine positive and nine negative causal associations between inflammatory proteins and epilepsy and its subtypes. Furthermore, we found that C-X-C motif chemokine 11 (CXCL11) levels mediated the causal association between Genus Family XIII AD3011 group and epilepsy.
Conclusion: Our study highlights the possible causal role of specific GM and specific inflammatory proteins in the development of epilepsy and suggests that circulating inflammatory proteins may mediate epileptogenesis through the MGBA.
Epilepsy, a chronic disorder characterized by the presence of at least one non-provoked epileptic seizure with high recurrence risk, is one of the most common neurological disorders (1), with more than 50 million cases of epilepsy globally, accounting for more than 0.5% of the global economic burden of the disease (2). According to the classification proposed by the International League Against Epilepsy (ILAE) in 2017, epilepsy can be classified into four categories: Generalized Epilepsy (GE), Focal Epilepsy (FE), Combined Generalized and Focal Epilepsy, and Unknown Epilepsy (3). Antiepileptic drugs are the primary and effective means of epilepsy treatment, and there are more than 25 different types of antiepileptic drugs available. However, one-third of epileptic patients still fail to achieve long-term remission with the application of drugs (4). Therefore, it is necessary to explore the pathogenic mechanisms of epilepsy further to provide new therapeutic approaches for the treatment of drug-resistant epilepsy (DRE).
Gut microbiota (GM) is a complex community of bacteria residing in the intestinal tract. There are about 800 species of human GM, mainly composed of the phylum of Firmicutes and Bacteroides (about 94%), of which Clostridia dominate the phylum Firmicutes (about 95%) (5). As an essential regulator of host disease susceptibility, the GM plays a role in basic neurogenic processes such as blood-brain barrier (BBB) formation, myelin sheath formation, neurogenesis, and microglia maturation (6). In recent years, several studies have shown that there is a statistical difference in the fecal microbiota composition between epileptic patients and healthy populations and that modulating the composition of GM in epileptic patients or epilepsy model rats using the ketogenic diet (KD), in turn, improves their epileptic symptoms (7–12). These studies have preliminarily investigated the association between GM and epilepsy, suggesting that GM may regulate neurological functions and behaviors and participate in the pathogenesis of various neurological disorders, including epilepsy, through the microbiota-gut-brain axis (MGBA). However, population-based trials are limited mainly by sample size, and the changes in the abundance of GM associated with epilepsy presented in different trials are inconsistent (13). Also, the effects of and specific mechanisms of various GM taxa on epilepsy have not been clarified.
Inflammatory proteins as essential mediators of inflammatory response, especially pro-inflammatory cytokines in microglia and astrocytes, including interleukin-1β (IL-1β), interleukin-6 (IL-6), tumor necrosis factor-α (TNF-α), and other cytotoxic factors, are closely associated with the occurrence of epilepsy (14). Studies have shown that epileptogenesis is associated with an increased, intense, and persistent inflammatory state in the microenvironment of neuro-tissues (15). However, circulating inflammatory proteins can also enter the central nervous system (CNS) by disrupting the BBB, leading to an inflammatory response that promotes neuronal overexcitation and seizure activity (16). Similarly, circulating inflammatory proteins are closely associated with GM. Recent studies have suggested that GM plays a role in inflammatory regulation by influencing the differentiation of inflammatory cell types, cytokine production, and hematopoiesis. Altering the composition of GM and increasing intestinal permeability can result in the leakage of endotoxins into the circulation, contributing to systemic inflammation (17). Based on the above, we hypothesized that circulating inflammatory proteins may be involved in the pathogenesis of epilepsy through the MGBA.
However, few studies have explored the causal association and mechanism of interaction between specific GM and epilepsy and its subtypes, as well as the potential mediators in the chain of causality between GM and epilepsy. Moreover, the complexity of the human intestinal environment, the numerous factors affecting it, and the difficulty of adequately measuring and controlling confounding factors make it costly, lengthy, and challenging to carry out randomized controlled experiments on large populations. Therefore, we used a novel epidemiological method, Mendelian randomization (MR) analysis, to overcome these limitations.
MR analysis detects and quantifies the causal association between exposure and outcome using genetic variants as instrumental variables (IVs), usually single nucleotide polymorphisms (SNPs) (18). This approach reduces confounding due to environmental factors and avoids bias due to reverse causation (19). Genome-wide association studies (GWAS) have identified thousands of genetic variants associated with various complex diseases, pushing the widespread use of MR to a higher stage (20). Several studies have been performed using MR analysis to explore the association between GM and CNS diseases such as stroke (21), Parkinson’s disease (22), cerebral hemorrhage (23), and Alzheimer’s disease (24). We used multivariate MR analysis on this foundation to investigate the causal relationship between GM and epilepsy, its subtypes, and possible mediating variables in their causal chains.
This study consists of two main components, as shown in Figure 1. We first performed univariate Mendelian randomization (UVMR) analyses to assess the causal effects of the GM-inflammatory proteins-epilepsy pathway. We evaluated the causal associations of (i) 211 GM with epilepsy and its subtypes, (ii) 91 inflammatory proteins with epilepsy and its subtypes, and (iii) GM and inflammatory proteins significantly associated with epilepsy. We then analyzed the potential mediating role in the causal relationship between GM and epilepsy by using the significantly associated inflammatory proteins obtained from Step 1, shown in Figure 1, as mediating variables and including them in multivariate Mendelian randomization. As in Step 2-2 of Figure 1, this design fulfills three basic assumptions of MR, which ensures valid IVs: (i) genetic variants as IVs must be significantly associated with exposure factors; (ii) genetic variants must be independent of any confounding factors affecting exposure and outcome; and (iii) genetic variants can only affect outcome through exposure and not through other pathways (25). This study used the STROBE-MR as the referenced reporting specification (26).
Figure 1. The overall flowchart of this study Step 1-1A represents the causal associations of gut microbiota on epilepsy and subtypes. Step 1-1B represents the reverse causal associations between gut microbiota and epilepsy and its subtypes. Step 1-2A represents the causal associations of inflammatory proteins on epilepsy and subtypes. Step 1-2B represents the reverse causal associations between inflammatory proteins and epilepsy and its subtypes. Step 1-3 represents the causal relationship between gut microbiota significantly associated with epilepsy and its subtypes and inflammatory proteins significantly associated with epilepsy and its subtypes. Each Univariate MR performs the procedure in the dashed box on the right. Step 2-1 represents incorporating positive microbiota and positive proteins obtained from Step 1 into Multivariate MR. Step 2-2 represents the detailed design of this Multivariate MR study of the causal associations of gut microbiota with the risk of epilepsy and its subtypes. MR, Mendelian randomization; GWAS, genome-wide association studies; WME, weighted median estimator; SNP, single nucleotide polymorphism; IVs, instrumental variables.
The GWAS summary statistics used for statistical analyses are listed in Table 1. The human GM GWAS data used in this study was derived from a study by the MiBioGen International Consortium, analyzing genome-wide genotypes and 16S fecal microbiome data from 18,340 participants in 24 cohorts from multiple countries and conducting a large-scale, multi-ancestry, genome-wide meta-analysis of associations between human autosomal genetic variants and GM, with the majority of participants being of European ancestry (16 cohorts, N = 13266). After adjusting for age, sex, technical covariates, and genetic principal components, the results of the study’s microbiome quantitative trait loci (mbQTLs) analyses yielded 211 microbial taxonomically relevant GWAS summary statistics for nine phyla, 16 classes, 20 orders, 35 families, and 131 genera (27).
Table 1. Characteristics of the GWAS summary-level data included in this Mendelian randomization analysis.
The GWAS summary data for circulating inflammatory proteins comes from a recent study that conducted a genome-wide protein quantitative trait locus (pQTL) study of 91 plasma proteins in 14,824 participants from 11 cohorts and combined these data with disease GWAS to characterize the functional effects of disease-associated variants (28).
The GWAS summary data for epilepsy in this study were obtained from the FinnGen Consortium R10 release, which involves 12891 epilepsy patients and 312803 control subjects and is the most recent genome-wide large-scale analysis available. In addition, we also downloaded summary GWAS data for GE and FE (29). FinnGen’s diagnosis of epilepsy is based on G40 in the 10th edition of the International Classification of Diseases (ICD). Under a strict definition, GE and FE are narrower endpoints for epilepsy.
In this study, P < 5 × 10-8 was pre-set as the significance threshold to screen highly correlated SNPs in the GWAS data of GM and inflammatory proteins. Since no SNPs or fewer than 3 SNPs were extracted for specific GM or inflammatory proteins, based on the convention of previously published articles, this study relaxed the threshold requirement by using P < 1 × 10-5 and P < 5 × 10-6 as the significance thresholds for selecting IVs associated with GM and inflammatory proteins, respectively (24). The selection of all IVs utilized additional criteria to ensure the robustness and accuracy of outcomes: (i) Independent SNPs were clustered according to Linkage disequilibrium (LD) analysis to obtain exposure, with a clustering threshold of R2 value < 0.001 and allelic distance > 10000 kb, to ensure that the IVs were independent of each other. If some SNPs were not included in the outcome GWAS, the 1000 Genomes Project’s European sample data was used as the reference standard to find alternative SNPs with R2 value > 0.8 under the LD criterion, and if no alternative SNP could be found, this SNP was excluded (30, 31); (ii) Exclusion of palindromic SNPs was performed to prevent alleles from affecting causal outcomes; (iii) Calculation of the F statistic for each IV according to the formula:
where R2, N, and K mean the estimated exposure variance explained by IVs, sample size, and the number of IVs, respectively; R2 is calculated as
where EAF is the effect allele frequency, β is the allele effect value, and SNPs with F value < 10 were excluded to avoid bias caused by weak IVs (19, 32).
We first performed three UVMR analyses to assess the causal association of the GM-inflammatory proteins-epilepsy pathway (Figure 1). We used the Wald ratio to evaluate causal effects for data in which a single SNP was used as an instrumental variable (32). The Wald ratio, which uses a single IV to estimate the causal effect of exposure (X) on outcome (Y), is the basis for all MR analyses. For data with 2 SNPs as IVs, MR analysis was performed using the inverse variance weighted (IVW) method (33). The IVW method is characterized by regressions that do not take into account the presence of an intercept term and are fitted with the inverse of the variance of the outcome as a weight. Thus, if there is no evidence of horizontal pleiotropy in the IVs, the results of IVW will be determined to be the most reliable. For data with multiple SNPs as instrumental variables, we used IVW, Mendelian randomization of Egger regression (MR-Egger), weighted median estimator (WME), Weighted mode, and Simple mode for MR analysis. The size of the regression intercept of MR-Egger can detect and adjust genetic pleiotropy. The larger the intercept, the greater the possibility of genetic pleiotropy. If the P>0.05 of the pleiotropy test, it is considered that there is no genetic pleiotropy, and the influence of the pleiotropy on the estimation of causal effect can be ignored, but the efficacy of the MR-Egger method is lower than that of the other methods (34). The weighted median method gives unbiased estimates based on the assumption that at least 50% of the IVs are valid (35). Under the assumption that all SNPs are valid instrumental variables, The IVW method provides the most precise effect estimates and is more efficacious than other methods. Therefore, the IVW was used in this study as the primary method to analyze the causal associations. The results of MR were expressed as the odds ratios (ORs) and the corresponding 95% confidence intervals (CIs). We determined the results to be statistically significant when the p-value of IVW was < 0.05 and the IVW was in the same direction as the MR-Egger.
We included GM and inflammatory proteins significantly causally associated with epilepsy and its subtypes in mediation analyses and used MVMR methods to validate the potential mediating role of inflammatory proteins in the causal pathway between GM and epilepsy. MVMR is an extension of UVMR that can decompose the total effect between exposure and outcome into direct and indirect effects through mediation. In our study, UVMR was used to estimate the total causal relationship between exposure and outcome, whereas MVMR analyses were used to assess the direct effect of exposure on outcome after adjusting for mediation (36, 37).
To determine the direction of causal associations and to exclude the influence of reverse causal associations on the results, we performed reverse MR analyses using epilepsy and its subtypes as exposures and significant GM and proteins identified in the forward MR analyses as outcomes, respectively. The conditions for screening IVs and statistical analysis methods were kept consistent with the forward direction to obtain more rigorous results of reverse MR analysis. When the forward MR analysis was statistically significant, but the reverse MR analysis was not, the direction of the causal effect could be further confirmed.
In order to further test the stability and reliability of the findings and to control the quality of the results, this study conducted heterogeneity tests, horizontal pleiotropy tests, and sensitivity analyses. First, Cochran’s Q test was used to assess the heterogeneity among the effect estimates of IVs, and it was concluded that if the P value was higher than 0.05 and there was no evidence of heterogeneity, the fixed-effects IVW method was the primary method, if the P<0.05, it could be assumed that there was a significant heterogeneity among the effect estimates of IVs, and the random-effects IVW method was used (38). Second, MR-Egger intercept test and Mendelian Randomization Pleiotropy RESidual Sum and Outlier (MR-PRESSO) were used to assess the pleiotropic relationship between IVs and other potential confounders, ensuring that the selected IVs did not affect outcome variables through pathways other than exposure factors. The MR-Egger intercept estimates genetic pleiotropy by analyzing the size of the regression intercept and calculating a p-value, which indicates horizontal pleiotropy in the set of IVs if the p-value is < 0. 05. MR-PRESSO consists of three parts: MR-PRESSO global test, MR-PRESSO outlier test, and MR-PRESSO distortion test. While identifying and eliminating SNPs with horizontal pleiotropic outliers, MR-PRESSO can re-estimate the effect of the IVs set and conduct a difference test between the effect estimates of the IVs set with and without removed outliers to determine whether the outlier SNPs have significantly affected the effect estimates of the original IVs set (39). In addition, we performed sensitivity analyses of the findings using the leave-one-out method, which calculates the results of all remaining IVs by eliminating SNPs from the set of IVs one by one. When there is no statistically significant difference between a particular MR result and the total result, it means that any single SNP cannot drive causality.
Multiple hypothesis tests were conducted in this study. To avoid increasing the probability of one type of error, we used the q-value procedure for False Discovery Rate (FDR) correction to control the false-positive rate of multiple comparisons. In this study, results with FDR q-value < 0.1 were defined as significant; results with P < 0.05 but FDR q-value > 0.1 were defined as nominally significant (40, 41).
All MR analyses were performed using R software (version 4.3.1) under the “TwoSample MR” package (version 0.5.6 https://mrcieu.github.io/TwoSampleMR). The main R packages used were also MR-PRESSO (version 1.0), q-value (version 2.34.0), Forestploter (version 1.1.1), and circlize (version 0.4.15). MR analyses were considered to be statistically significant at P < 0.05.
According to the screening criteria for IVs, 195 bacterial traits and 2559 SNPs were obtained in this study, containing 125 in 9 phyla, 224 in 16 classes, 280 in 20 orders, 434 in 31 families, and 1496 in 119 genera, respectively. (Of these, 15 GM were excluded due to unknown information, and 1 GM was excluded due to no eligible SNPs.) The F-statistics for all included variables were greater than 10, indicating no weak instrumental variable bias. (Min = 16.91, Max = 88.42). Detailed information on each GM trait SNP (e.g. effect allele, other allele, beta, standard error, and p-value) is available in Supplementary Table S1. We then identified 1820 SNPs associated with 91 inflammatory proteins at a level of P < 5 × 10-6, and similarly, the F-statistics of the included variables were all greater than 10. (Min = 20.84, Max = 2740.70) (Supplementary Table S2).
Figure 2A shows the effect of changes in abundance of 192 GM taxa on the risk of epilepsy. The results of our study suggested that a total of eleven GM (including two classes, one order, one family, and seven genera) were associated with epilepsy based on the IVW method, and detailed information on 118 SNPs associated with these eleven GM is shown in Supplementary Table S3. The causal associations between GM and epilepsy were also analyzed using MR-Egger, WME, Weighted mode, and Simple mode (Supplementary Table S4). Finally, nine epilepsy-associated GM were included, as shown in Figure 3 (Genus Ruminococcaceae UCG009 was excluded due to the opposite direction of effect value between the IVW method and MR-Egger method, and Genus Bifidobacterium was excluded due to unsatisfied pleiotropy). Class Betaproteobacteria (OR=1.2393, 95%CI =1.0643~ 1.4431, P=0.0057), Class Gammaproteobacteria (OR=1.2354, 95%CI =1.0051~ 1.5184, P=0.0446), Family Streptococcaceae (OR=1.146, 95%CI =1.0066~ 1.3046, P=0.0394), Genus Anaerotruncus (OR=1.1705, 95%CI =1.0236~ 1.3385, P=0.0214), Genus Eubacterium nodatum group (OR=1.0789, 95%CI =1.0085~ 1.1542, P=0.0274), Genus Family XIII AD3011 group (OR=1.1331, 95%CI =1.0001~ 1.2838, P=0.0498), Genus Marvinbryantia (OR=1.2082, 95%CI =1.0554~ 1.3831, P=0.0061), and Order Burkholderiales (OR=1.2236, 95%CI =1.053~ 1.4218, P=0.0084), a total of eight GM were genetically predicted to be associated with an increased risk of epilepsy. Genetic prediction of Genus Phascolarctobacterium (OR=0.8483, 95%CI =0.7389~ 0.9738, P=0.0194) was probably associated with a decreased risk of epilepsy.
Figure 2. Cyclic heat map of causality associated with epilepsy (A-C) represent causal associations of GM with epilepsy and its subtypes, (D-F) represent causal associations of inflammatory proteins with epilepsy and its subtypes, and the innermost circle represents the OR value of the IVW method, the outermost abbreviation of (A-C) consists of the initial capitalization of taxa names of GM plus the number of GM, and the outermost abbreviations of (A-F) are shown in Supplementary Table S10. IVW, inverse variance weighted; MR-Egger, Mendelian randomization of Egger regression; WM, weighted median estimator; Q, Cochran’s Q test; GE, Generalized Epilepsy; FE, Focal Epilepsy.
Figure 3. IVW method results on causal associations between GM and epilepsy and its subtypes. Nsnps, number of single nucleotide polymorphisms; OR, odds ratio; CI, confidence intervals.
Figure 2D presents the effect of changes in 91 circulating inflammatory proteins on the risk of epilepsy. Five inflammatory proteins, including 111 SNPs, were initially screened after the IVW method (Supplementary Table S5). The results of Mendelian randomization for the five methods are shown in Supplementary Table S6. After a series of sensitivity analyses, Leukemia inhibitory factor receptor (LIFR) levels were excluded due to non-satisfaction of pleiotropy, and thus four inflammatory proteins were causally associated with epilepsy onset at the genetical level (Figure 4). Two inflammatory proteins, namely, C-X-C motif chemokine 11(CXCL11) levels (OR=1.1078, 95%CI =1.0255~ 1.1967, P=0.0093) and Vascular endothelial growth factor A (VEGFA) levels (OR=1.0721, 95%CI =1.0219~ 1.1247, P=0.0044) were positively associated with epilepsy. Tumor necrosis factor-β (TNF-β) levels (OR=0.9558, 95%CI =0.9151~ 0.9983, P=0.0417) and Tumor necrosis factor ligand superfamily member 12 (TNFSF12) levels (OR=0.9049, 95%CI =0.8443~ 0.9698, P=0.0047) were negatively associated with epilepsy.
Figure 4. IVW method results on causal associations between circulating inflammatory proteins and epilepsy and its subtypes. Nsnps, number of single nucleotide polymorphisms; OR, odds ratio; CI, confidence intervals; CXCL11, C-X-C motif chemokine 11; TNF-β, Tumor necrosis factor-β; TNFSF12, Tumor necrosis factor ligand superfamily member 12; VEGF-A, Vascular endothelial growth factor A; CXCL1, C-X-C motif chemokine 1; CXCL9, C-X-C motif chemokine 9; IL-15RA, Interleukin-15 receptor subunit alpha; IL-6, interleukin-6; LIF, Leukemia inhibitory factor; TRAIL, TNF-related apoptosis-inducing ligand; CCL25, C-C motif chemokine 25; EIF4EBP1, Eukaryotic translation initiation factor 4E-binding protein 1; FGF21, Fibroblast growth factor 21; IL-20RA, Interleukin- 20 receptor subunit alpha; TNFSF14, Tumor necrosis factor ligand superfamily member 14; VEGF-A, Vascular endothelial growth factor A.
Figure 2B presents the effect of abundance variation of 192 GM taxa on FE risk. As shown in Figure 3, the genetic prediction of 1 GM, including 12 SNPs, was identified as being associated with FE after IVW screening. Details of the 12 SNPs are shown in Supplementary Table S3. The results suggested that Genetic prediction of Genus Phascolarctobacterium per unit increase was associated with a 15.17% reduction in the risk of FE (OR=0.8483, 95%CI =0.7389~ 0.9738, P=0.0194).
Figure 2E shows the effect of changes in 91 circulating inflammatory proteins on FE risk. A total of ten inflammatory proteins were associated with FE, and specific information on 176 SNPs is available in Supplementary Table S5. After screening by sensitivity test, a total of eight inflammatory proteins were finally included, shown in Figure 4 (CXCL11 levels was excluded due to the opposite direction of effect value between the IVW method and MR-Egger method, and Leukemia inhibitory factor receptor levels was excluded because it did not satisfy pleiotropy). C-X-C motif chemokine 1 (CXCL1) levels (OR=1.1626, 95%CI =1.0407~ 1.2986, P=0.0076), C-X-C motif chemokine 9 (CXCL9) levels (OR=1.1234, 95%CI =1.007~ 1.2531, P=0.037), IL-6 levels (OR=1.188, 95%CI =1.019~ 1.385, P=0.0277), TNF-related apoptosis-inducing ligand (TRAIL) levels (OR=1.0664, 95%CI =1.0009~ 1.1363, P=0.0469), and VEGFA levels (OR=1.0678, 95%CI =1.0007~ 1.1394, P=0.0476) were associated with an increased risk of FE. While Interleukin-15 receptor subunit alpha (IL15RA) levels (OR=0.9186, 95%CI =0.8534~ 0.9888, P=0.0238), Leukemia inhibitory factor (LIF) levels (OR=0.8393, 95%CI =0.7151~ 0.9851, P=0.0321), and TNFSF12 levels (OR=0.8694, 95%CI =0.7916~ 0.9549, P=0.0034) may decrease the risk of FE.
Figure 2C shows the effect of changes in the abundance of 192 GM taxa on GE risk. The same method was used to explore the causal association between GM and GE, and results showed that 95 SNPs in nine GM were associated with GE (Supplementary Table S3). Among them, seven GM, Genus Bifidobacterium (OR=1.2275, 95%CI =1.0567~ 1.4259, P=0.0073), Class Verrucomicrobiae (OR=1.8325, 95%CI =1.0332~ 3.2502, P=0.0383), Family Verrucomicrobiaceae (OR=1.8321, 95%CI =1.0326~ 3.2505, P=0.0385), Genus Akkermansia (OR=1.8318, 95%CI =1.0327~ 3.2493, P=0.0384), Genus Marvinbryantia (OR=2.1091, 95%CI =1.2092~ 3.6788, P=0.0086), Order Gastranaerophilales (OR=1.5522, 95%CI =1.0393~ 2.3182, P=0.0317), Order Verrucomicrobiales (OR=1.8325, 95%CI =1.0332~ 3.2502, P=0.0383) and Phylum Cyanobacteria (OR=1.7151, 95%CI =1.0868~ 2.7065, P=0.0205) may be associated with an increased risk of GE. Two GM, Genus Romboutsia (OR=0.5719, 95%CI =0.3466~ 0.9436, P=0.0287) and Genus Ruminiclostrium5 (OR=0.4414, 95%CI =0.2289~ 0.8514, P=0.0147) were protective factors for GE.
Figure 2F presents the effect of changes in 91 circulating inflammatory proteins on the risk of GE. Genetic prediction of 6 inflammatory proteins, a total of 109 SNPs (Supplementary Table S5), was associated with the risk of GE. Four inflammatory proteins, namely C-C motif chemokine 25 (CCL25) levels (OR=0.8358, 95%CI =0.7129~ 0.9799, P=0.0271), Eukaryotic translation initiation factor 4E-binding protein 1 (EIF4EBP1) levels (OR=0.4794, 95%CI =0.3104~0.7405, P=0.0009), Fibroblast growth factor 21 (FGF21) levels (OR=0.7067, 95%CI =0.5006~ 0.9979, P=0.0486), and Interleukin- 20 receptor subunit alpha (IL20RA) levels (OR=0.5907, 95%CI =0.3591~ 0.9717, P=0.0382) significantly reduced the risk of GE. Tumor necrosis factor ligand superfamily member 14 (TNFSF14) levels (OR=1.3814, 95%CI =1.0634~ 1.7946, P=0.0155) and VEGFA levels (OR=1.2505, 95%CI =1.0257~ 1.5246, P=0.027) were associated with an increased risk of GE.
Cochran’s Q test suggested that the results were not statistically significant, indicating that it was not necessary to consider the effect of heterogeneity and genetic pleiotropy on the results, demonstrating the robustness of our findings. Three sets of causal relationships (Genus Bifidobacterium on epilepsy, LIFR on epilepsy, and LIFR on FE) were excluded due to unsatisfied pleiotropy. The regression intercepts of MR-Egger for the remaining data after exclusion did not significantly deviate from 0, with p-values >0.05, indicating no horizontal pleiotropy and suggesting that the relationship between the IVs and the outcome is not affected by potential confounders. In addition, the significance of the MR-PRESSO global test (P > 0.05) revalidated the non-existence of horizontal pleiotropy, and no instrumental variables were identified as potential outliers by the MR-PRESSO outlier test (Supplementary Table S7). Leave-one-out analyses showed that after removing SNPs one by one, it turned out that the effect sizes of the including IVs and the total effect sizes were found to be relatively similar, and no SNPs were found yet to have a significant impact on the causal association estimates, see Additional file 1. The funnel plot results indicate that when SNPs are used as IVs on a case-by-case basis, the scatter of causal association effects is essentially symmetrically distributed, and there is no potential bias in the results.
In the reverse MR analysis of GM and epilepsy and its subtypes, the genome-wide significance level was set at P < 1 × 10-5, following the principle of consistency with the parameters of the forward MR analysis. Snps were extracted for reverse MR analysis after clustering (R2 = 0.001, kb = 10,000) and harmonization. The IVW method (Supplementary Table S8) showed no reverse causal association between GM and epilepsy and its subtypes. Also, following the same method and parameter settings as for forward MR (genomic significance level set to P < 5 × 10-6, removal of chain disequilibrium parameter set to R2 = 0.001, kb = 10,000), there was likewise no reverse causal effect between inflammatory proteins and epilepsy and its subtypes.
The causal effect of epilepsy-associated GM on epilepsy-associated inflammatory proteins is demonstrated in Supplementary Table S9, in which seven pairs of potential mediator proteins associated with GM and epilepsy were initially screened. Genus Family XIII AD3011 group was positively associated with CXCL11 levels (OR=1.1398, 95%CI =1.0168~ 1.2776, P=0.0247) among the epilepsy-associated GM and inflammatory proteins. In GM and inflammatory proteins associated with FE, no causal association was found. Among GM and inflammatory proteins associated with GE, a total of five GM, namely, Class Verrucomicrobiae (OR=1.1780, 95%CI =1.0557~ 1.3145, P=0.0034), Family Verrucomicrobiaceae (OR=1.1781, 95%CI =1.0558 ~1.3146, P=0.0034), Genus Akkermansia (OR=1.1782, 95%CI =1.0559~ 1.3146, P=0.0034), Order Gastranaerophilales (OR=1.1215, 95%CI =1.0312~ 1.2196, P=0.0074), and Order Verrucomicrobiales (OR=1.178, 95%CI =1.0557~1.3145, P=0.0034) were positively correlated with CCL25 levels, besides, Phylum Cyanobacteria was positively correlated with FGF21 levels (OR=1.1404, 95%CI =1.0312~ 1.2613, P=0.0106).
We sequentially incorporated seven pairs of GM and inflammatory proteins associated with epilepsy into the mediation analysis using the MVMR method to calculate the indirect effects and proportions mediated by these inflammatory proteins. As shown in Table 2, the effect of CXCL11 levels on epilepsy remained significant after adjustment for Genus Family XIII AD3011 group. In contrast, the effect of Genus Family XIII AD3011 group on epilepsy was not significant after adjusting for CXCL11. Therefore, we conclude that CXCL11 levels indirectly affect the association between Genus Family XIII AD3011 group and epilepsy. The mediation percentage was 11.64% (P=0.02126).
Table 2. Multivariable MR analyses of the causal associations between gut microbiota, inflammatory proteins, and epilepsy and its subtypes.
The GM regulates the CNS through metabolites, neurotransmitters, and inflammatory factors, while the CNS regulates the GM through the sympathetic and parasympathetic branches of the autonomic nervous system or hormonal axes (42). The bi-directional regulatory axis of functional interactions between GM and CNS is known as the MGBA (43, 44). Existing studies have shown that the MGBA plays a vital role in the regulation of a variety of neurological disorders, including Parkinson’s disease, Alzheimer’s disease, depression, and multiple sclerosis (45–48).
Our study comprehensively investigated the causal effects of 211 GM (from phylum to genus) on epilepsy and its subtypes using bidirectional Mendelian randomization, and the results suggested that 3 GM were associated with a reduced risk of epilepsy and its subtypes and 16 GM were associated with an increased risk of epilepsy and its subtypes. These suggest a possible relationship between structural and functional changes in the GM of epileptic patients and their pathogenesis. Existing studies have summarised that GM may be involved in the pathogenesis of epilepsy through a variety of pathways, such as regulating autoimmune and inflammatory responses, synthesizing enteroendocrine signaling and microbial metabolites, influencing the electrical activity of autonomic nerve cells, and improving the activity of the hypothalamic-pituitary-adrenal axis and the endocannabinoid system (13, 42, 49). Research on the mechanisms between epilepsy and GM, as well as the differences in GM between patients with different types of epilepsy and healthy individuals, have made it possible that changes in GM abundance may be a potential biomarker for epilepsy risk prediction, prognostic monitoring, and pharmacodynamics (50).
Our findings suggest that most of the GM associated with an increased risk of epilepsy and its subtypes are from Firmicutes, Proteobacteria, and Verrucomicrobia, with 12 species. A systematic review pooling three human studies and three animal experiments found increases in Firmicutes, Proteobacteria, Verrucomicrobia, and Fusobacteria and decreases in Bacteroidetes and Actinobacteria in epileptic subjects compared to normal controls (51), which is in line with our results, further demonstrating that epilepsy causes dysbiosis of the GM in research subjects. The increased ratio of Firmicutes/Bacteroidetes in patients with epilepsy suggests that this indicator may be potentially valuable for risk prediction in epilepsy, especially DRE.
Our studies have found that different GM has essentially different effects on different epilepsy types. However, we can see that Marvinbryantia is not only a risk factor for epilepsy but also FE, suggesting that Marvinbryantia may be involved in the pathogenesis of different epilepsies through multiple mechanisms. An experiment to evaluate changes in the composition of gut metagenome as well in the fecal metabolomic profile in rats before and after being submitted to status epilepticus (SE)-induced temporal lobe epilepsy (TLE) showed that Desulfovibrio and Marvinbryantia are significantly enriched and positively correlate with excitatory neurotransmitters in microbiota-host metabolism correlation analyses in rats after TLE (52). Research has also shown that the abundance of Marvinbryantia is positively correlated with the levels of inflammatory factors in the serum and intestinal mucosa and negatively correlated with the antioxidant capacity in the serum and the concentration of organic acids in the intestinal contents (53). The results of these studies are similar to ours, and all provide evidence for the pathway by which Marvinbryantia leads to an increased risk of seizures through neuroinflammatory pathways.
Our study showed that three GM, Phascolarctobacterium, Romboutsia, and Ruminiclostrium5, have protective effects against epilepsy and subtypes. An experiment comparing changes in the GM of WAG/Rij(a well-recognized genetic model of absence epilepsy) rats before and after epileptic seizures showed that, At four months of age, the microbiota distribution of WAG/Rij rats was different from that of the control rats, with a significant reorganization of bacterial genera of Firmicutes, of which Clostridiales, Clostridiaceae, and Lachnospiraceae were more abundant and Lactobacillus and Phascolarctobacterium were prominently less abundant (54). This suggested that Phascolarctobacterium may play a partially protective role against the onset of epilepsy in rats during this period. Seizure symptoms became increasingly severe in the WAG/Rij rat group. At the same time, Phascolarctobacterium abundance increased from being significantly lower than that of control rats at one month of age to return to normal control levels by eight months of age, suggesting that GM may be related to pathological aggravation of seizures and demonstrating Phascolarctobacterium may be used as a prognostic biomarker in clinical practice (54). Currently, there are relatively few studies on the effects of Romboutsia and Ruminiclostrium5 on epilepsy, and further studies are still needed to explore their relationship.
Peng et al. (7) found that the abundance of some rare phylum and genera of bacteria, such as Verrucomicrobia and Phascolarctobacterium, was abnormally increased in the DRE group compared with the sensitive group. In a trial comparing the GM composition of infantile spasms patients with and without response to adrenocorticotrophic hormone, the responder group had a decrease in the abundance of Odoribacter, Phascolarctobacterium, and others, as well as an increase in the abundance of Bifidobacterium (55), which provided a basis for the use of Phascolarctobacterium in assessing the pharmacodynamics of epilepsy-related drugs or as a prognostic biomarker. In the future, as research goes further, GM may serve as biomarkers and potential targets for the diagnosis and treatment of epileptic patients, as well as for assessing the efficacy of epilepsy medications.
Previous studies have shown that regardless of the integrity of the BBB, increased and sustained inflammatory states in the microenvironment of CNS neural tissues have been identified as a significant factor in the physiopathologically induced vicious circle of seizures (56, 57). Circulating inflammatory proteins, critical factors in communicating between the GM and its metabolites and host defense, may also play a role in epilepsy and the induction of a vicious circle through the MGBA (58). Among them, disruption of the BBB and diminished anti-inflammatory effects of short-chain fatty acids (SCFA) may be two main drivers of the vicious cycle of epilepsy (6).
Under a steady-state condition, the GM creates an ultra-low activation of the immune system with stimulation of several types of T cells and macrophages to secrete pro-inflammatory cytokines such as IL-1β and TNF-α. This chronic state of intestinal immune activation can eventually involve the entire body without consequences on health (59, 60). Once the epithelial barrier is damaged by aging, injury, infection, and other factors, it could lead to dysbiosis of the GM and the infiltration of its metabolites, triggering the systemic inflammatory immune response (61). Inflammatory proteins such as TNF-α, IL-6, and IL-1β in serum will increase dramatically in the systemic inflammatory immune state and bind to their special receptors (16), destroying the tight junctions and transendothelial electrical resistance of the BBB (62). At the same time, brain pericytes (a component of the BBB) can also respond to circulating inflammatory signals, such as IL-1β and TNF-α, and transmit these messages to pericytes, aggravating the inflammatory response (63). On the one hand, pericytes may secrete chemokines and regulate leukocyte migration across the brain microvascular endothelial cell barrier by upregulating intercellular adhesion molecule 1 (ICAM-1) and vascular cell adhesion molecule one on endothelial cells (64, 65), on the other hand, pericytes can activate microglia by releasing IL-6 through the Janus kinase-signal transducer and activator of transcriptions (JAK-STAT3) pathway, resulting in breakdown of the BBB and further aggravating the intracranial inflammatory storm, leading to epileptogenesis and a vicious cycle (66).
Some phyla of GM, primarily Bacteroidetes, can ferment insoluble dietary fiber to produce SCFA, including acetate, propionate, and butyrate, which are known to exert anti-inflammatory activity through a variety of mechanisms, thereby reducing the risk of seizures (67). Oxidative stress and neuroinflammation are often co-existing in the brain of patients with epilepsy (68). It has been shown that SCFA, especially butyrate, induces the expression of antioxidant genes, activates cellular antioxidant mechanisms, increases the level of antioxidant enzymes, and thus inhibits the production of pro-inflammatory mediators through histone deacetylase (HDAC) inhibition and nuclear factor erythroid 2-related factor 2 (Nrf2) nuclear translocation (69). SCFA can cross the BBB and activate free fatty acid receptor 2/3 (FFAR2/3) expressed by peripheral immune cells on microglia to exert anti-inflammatory effects, blocking the positive feedback regulation between microglia and pro-inflammatory factors and protecting neurons and glial cells from inflammatory damage (67). In addition, SCFA can directly reduce the expression of pro-inflammatory factors such as IL-6 and TNF-α by disrupting MAPK and NF-κB signaling (70). Once dysbiosis of the GM affects the production of short-chain fatty acids, it may lead to the up-regulation of the inflammatory response and eventually to the development of epilepsy.
Our study used UVMR to explore the causal associations between 91 circulating inflammatory proteins and epilepsy and its subtypes and showed that 18 circulating inflammatory proteins were associated with epilepsy and its subtypes. CXCL11 levels and VEGFA levels increased the risk of epilepsy, CXCL1 levels, CXCL9 levels, IL-6 levels, TRAIL levels, and VEGFA levels were associated with an increased risk of FE and TTNFSF14 levels, VEGFA levels were associated with an increased risk of GE.
We observed that VEGFA promotes increased risk in epilepsy, FE, and GE. In a clinical trial, IL-1β, IL-6, and TNF-α, as well as transforming growth factor-β1 (TGF-β1) and vascular endothelial growth factor (VEGF) mRNA expression were upregulated in the hippocampus after seizures (71, 72). Another clinical study demonstrated that febrile convulsions increase the levels of cytokines IL-1β, IL-6, and TNF-α in cerebrospinal fluid (73). These are similar to our results and further suggest the reliability of subsequent studies in which we investigated the pathway of controlling the inflammatory response to reduce CNS excitability and thus prevent epileptogenesis.
Our results suggest that the effect of CXCL11 levels in increasing the risk of epilepsy remains valuable after adjusting for the effect of GM on epilepsy. CXCL11, also known as interferon inducible T cell α-chemoattractant, belongs to the chemokine CXC subfamily, a member of the non-ELR group, and shares a common C-X-C motif receptor 3 (CXCR3) with CXCL9 and CXCL10 (74). C XCL11 plays a role in immune and inflammatory regulation by recruiting and aggregating immune cells to the site of injury or inflammation through binding to the CXCR3 receptor (75). Previous studies have shown that CXCL11/CXCR3 expression is significantly upregulated in many CNS diseases, such as cerebral ischemic stroke and multiple sclerosis (76, 77). Relatively few studies have examined the relationship between CXCL11/CXCR3 and epilepsy. A study showed that pentylenetetrazole (PTZ)-induced seizure rat models upregulated CXCR3 expression compared to control rats, while pretreatment with sitagliptin reduced CXCL4/CXCR3 expression in vivo and in vitro (78). These suggest that CXCL11/CXCR3 may be involved in seizure and provide a rationale for targeting the down-regulation of the CXCL11/CXCR3 axis for the treatment of epilepsy.
Neuroinflammation itself interacts with epilepsy in a loop (79). Changes in the proportions of specific GM only affect specific cytokine responses (58). Based on this specific GM-Cytokine-disease interaction pattern, treatments targeting modulation of GM to improve neuroinflammation show possible outcomes in epilepsy, especially in DRE patients (79). Currently, new approaches to treating the disease by reversing the ecological dysregulation of GM include KD, Probiotics, and Fecal microbiota transplantation (FMT) (51).
The KD is a pattern of eating a very low-carbohydrate, high-fat diet (80). Several studies have shown that KD can regulate the composition of GM in people with epilepsy and improve epileptic symptoms (8, 9, 11, 12). These beneficial effects may be related to an increased abundance of beneficial bacteria producing short-chain fatty acids, such as Akkermansia muciniphila and Lactobacillus, and a decreased abundance of pro-inflammatory microorganisms, such as Desulfovibrio and Turicibacter (81).
Probiotics are defined as live microorganisms that are beneficial to the host’s health when administered in sufficient amounts. The most common probiotics are Lactobacillus and Bifidobacterium (82). An animal study suggests that a mixture of Lactobacillus rhamnosus, Lactobacillus reuteri, and Bifidobacterium infantis attenuates the severity of PTZ seizures by increasing GABA levels, decreasing nitric oxide production and increasing the antioxidant/oxidant ratio (83). A clinical study that included 70 subjects showed that probiotics had a positive impact on seizure control and improved anxiety, depression and quality of life in TLE patients (84). In an open-label pre-trial, approximately 28.9% of patients with refractory epilepsy had a >50% reduction in seizure frequency after four months of administering a probiotic mixture of 8 bacteria (85). These studies suggest the potential value of altering GM environment through the direct addition of probiotics to control seizure, pending additional trials to validate which bacteria, or mixtures of those bacteria, can maximize the effectiveness of seizure control through altering GM.
FMT is a method of transferring feces from a healthy provider into the gut of a recipient to alter the recipient’s GM directly (86). A FMT from KD-treated mice into control diet mice was sufficient for seizure protection in animal experiments (10). There are case reports that FMT has efficacy in preventing seizure recurrence after withdrawal of antiepileptic drugs (87). In the future, FMT could be used as a promising treatment for epilepsy if purified colony samples highly correlated with elevated epileptic thresholds are available.
In this study, we included GM and inflammatory proteins associated with epilepsy and its subtypes in the mediation analysis and used the MVMR method to explore the causal associations between GM, inflammatory proteins, and epilepsy, and finally obtained the mediating effect of CXCL11 in the causal association between Genus Family XIII AD3011 group and epilepsy. Few studies have examined the relationship between Family XIII AD3011 group and inflammatory proteins. An animal study showed that elevation of the Family XIII AD3011 group at the genus level was strongly associated with hematopoietic toxicity and IL-5 in a mouse model of benzene-induced hematopoietic toxicity (88). In model mice of colitis, Family XIII AD3011 group was positively correlated with the expression level of Aconitate decarboxylase 1 (ACOD1), which is highly expressed in colonic tissues in inflammatory diseases, whose expression is positively correlated with the severity of intestinal inflammation (89). Multiple population trials demonstrated that Family XIII AD3011 group abundance associated with inflammation is elevated in patients with high levels of fatigue, chronic schizophrenia, colitis, and colon cancer (90–92). All of this evidence suggests that the increased abundance of Family XIII AD3011 group causes inflammation, which is generally consistent with our findings. No relevant studies exploring the relationship between Family XIII AD3011 group and epilepsy have been reported. However, our study suggests that CXCL11 and Genus Family XIII AD3011 group may serve as a new target for antiepileptic therapy and also indicates a chain of causality: Genus Family XIII AD3011 leads to epileptogenesis through CXCL11. In the future, animal experiments or large-scale clinical trials can be conducted to investigate the relationship and mechanism of action between the three.
This study is the first large MR study to investigate the mediating role of circulating inflammatory proteins in the causal chain of GM with epilepsy and its subtypes. This study has some limitations: firstly, our study only analyzed European populations. Future studies should consider the use of independent datasets using data from different populations and different research contexts that can help confirm whether the causal relationships we found are generalizable and robust. Secondly, the abundance of GM may also be affected by factors such as diet, gender, medication, and sampling time, and the variance explained by genes may be reduced. Although we explored the role of circulating inflammatory proteins in mediating the relationship between different GM abundances and epilepsy, the mechanisms of how GM affect seizures remain to be investigated, given the complexity of the co-action between GM and inflammatory proteins.
This study is the first to investigate the causal relationship between GM, circulating inflammatory proteins, and epilepsy and its subtypes. This study showed 16 positive and three negative causal associations between the genetic prediction of GM and epilepsy and its subtypes and nine positive and nine negative correlations between the genetic prediction of inflammatory protein epilepsy and its subtypes. Furthermore, we found that CXCL11 mediates the association between Genus Family XIII AD3011 group and epilepsy. These findings support the role of inflammatory proteins in the pathogenesis of epilepsy through MGBA and provide solid scientific evidence for GM and inflammatory proteins as potential targets for epilepsy in prevention, diagnosis, and treatment. Nonetheless, future studies need to dissect the pathophysiological role of specific GM in epilepsy and its subtypes and further reveal the optimal GM composition to provide a potential approach for the treatment of DRE.
Publicly available datasets were analyzed in this study. This data can be found here: GWAS summary data for GM genera from the MiBioGen repository was available at https://www.mibiogen.org/. GWAS datasets for 91 circulating inflammatory proteins are available from the EBI GWAS Catalog (https://www.ebi.ac.uk/gwas/downloads/summary-statistics accession numbers GCST90274758 to GCST90274848). GWAS data for epilepsy and its subtypes from the FinnGen consortium were available at https://r10.finngen.fi/.
HY: Conceptualization, Formal analysis, Methodology, Writing – original draft, Writing – review & editing. WL: Conceptualization, Data curation, Writing – original draft. TG: Data curation, Formal analysis, Visualization, Writing – original draft. QL: Formal analysis, Methodology, Writing – original draft, Data curation. MZ: Data curation, Software, Writing – original draft. YL: Data curation, Software, Writing – original draft. XM: Formal analysis, Software, Writing – original draft. NZ: Formal analysis, Visualization, Writing – original draft. KS: Formal analysis, Visualization, Writing – original draft. MD: Formal analysis, Software, Writing – original draft. SM: Software, Writing – original draft. XZ: Methodology, Software, Writing – original draft. YC: Supervision, Validation, Writing – review & editing. HQ: Supervision, Writing – review & editing. MC: Supervision, Validation, Writing – review & editing. SZ: Conceptualization, Funding acquisition, Supervision, Validation, Writing – review & editing.
The author(s) declare financial support was received for the research, authorship, and/or publication of this article. The study was supported by the National Natural Science Foundation of China (grant number 81070999) and the Foundation of Shaanxi health research project (grant number 2022A006).
We want to thank the participants and researchers in the FinnGen study and the MiBioGen consortium, as well as researchers such as Zhao JH and Stacey D, for sharing the GWAS summary statistics.
The authors declare that the research was conducted in the absence of any commercial or financial relationships that could be construed as a potential conflict of interest.
All claims expressed in this article are solely those of the authors and do not necessarily represent those of their affiliated organizations, or those of the publisher, the editors and the reviewers. Any product that may be evaluated in this article, or claim that may be made by its manufacturer, is not guaranteed or endorsed by the publisher.
The Supplementary Material for this article can be found online at: https://www.frontiersin.org/articles/10.3389/fimmu.2024.1438645/full#supplementary-material
SUPPLEMENTARY TABLE S1 | 2559 SNPs for the 195 gut microbiota taxa.
SUPPLEMENTARY TABLE S2 | 1820 SNPs for the 91 circulating inflammatory proteins.
SUPPLEMENTARY TABLE S3 | The characteristics of SNPs analyzing the causal effects of gut microbiota on epilepsy and its subtypes.
SUPPLEMENTARY TABLE S4 | The causal effects of gut microbiota on epilepsy and its subtypes.
SUPPLEMENTARY TABLE S5 | The characteristics of SNPs analyzing the causal effects of circulating inflammatory proteins on epilepsy and its subtypes.
SUPPLEMENTARY TABLE S6 | The causal effects of circulating inflammatory proteins on epilepsy and its subtypes.
SUPPLEMENTARY TABLE S7 | Mendelian randomization Sensitivity analysis.
SUPPLEMENTARY TABLE S8 | Reverse causal effects of epilepsy and its subtypes on the gut microbiota and circulating inflammatory proteins.
SUPPLEMENTARY TABLE S9 | Causal effects of gut microbiota associated with epilepsy and its subtypes on circulating inflammatory proteins associated with epilepsy and its subtypes.
SUPPLEMENTARY TABLE S10 | Table of abbreviations shown in Figure 2.
ADDITIONAL FILE 1 | The plots of MR analysis results.
ILAE: International League Against Epilepsy
GE: Generalized Epilepsy
FE: Focal Epilepsy
DRE: drug-resistant epilepsy
GM: gut microbiota
BBB: blood-brain barrier
KD: ketogenic diet
MGBA: microbiota gut-brain axis
IL-1β: interleukin-1β
IL-6: interleukin-6
TNF-α: tumor necrosis factor-α
MR: Mendelian randomization
IVs: instrumental variables
SNPs: single nucleotide polymorphisms
GWAS: genome-wide association studies
UVMR: Univariable Mendelian randomisation
mbQTLs: microbiome quantitative trait loci
pQTL: protein quantitative trait locus
ICD: International Classification of Diseases
LD: Linkage disequilibrium
IVW: inverse variance weighted
MR-Egger: Mendelian randomization of Egger regression
WME: weighted median estimator
ORs: odds ratios
CIs: confidence intervals
MVMR: Multivariable Mendelian randomization
MR-PRESSO: Mendelian Randomization Pleiotropy RESidual Sum and Outlier
FDR: False Discovery Rate
LIFR: Leukemia inhibitory factor receptor
CXCL11: C-X-C motif chemokine 11
VEGFA: Vascular endothelial growth factor A
TNF-β: Tumor necrosis factor-β
TNFSF12: Tumor necrosis factor ligand superfamily member 12
CXCL1: C-X-C motif chemokine 1
CXCL9: C-X-C motif chemokine 9
CXCR3: C-X-C motif receptor 3
TRAIL: TNF-related apoptosis-inducing ligand
IL15RA: Interleukin-15 receptor subunit alpha
LIF: Leukemia inhibitory factor
CCL25: C-C motif chemokine 25
EIF4EBP1: Eukaryotic translation initiation factor 4E-binding protein 1
FGF21: Fibroblast growth factor 21
IL20RA: Interleukin- 20 receptor subunit alpha
TNFSF14: Tumor necrosis factor ligand superfamily member 14
SE: status epilepticus
TLE: temporal lobe epilepsy
WAG/Rij: a well-recognized genetic model of absence epilepsy
CNS: central nervous system
HPA: hypothalamic pituitary adrenal
ICAM-1: intercellular adhesion molecule 1
JAK-STAT: Janus kinase-signal transducer and activator of transcriptions
SCFA: short-chain fatty acids
HDAC: histone deacetylase
Nrf2: nuclear factor erythroid 2-related factor 2
FFAR2/3: free fatty acid receptor 2/3
MAPK: mitogen-activated protein kinases
NF-κB: nuclear factor kappa B
TGF-β1: transforming growth factor-β1
VEGF: vascular endothelial growth factor
PTZ: pentylenetetrazole
FMT: Fecal microbiota transplantation
ACOD1: Aconitate decarboxylase 1
1. Fisher RS, Acevedo C, Arzimanoglou A, Bogacz A, Cross JH, Elger CE, et al. ILAE official report: a practical clinical definition of epilepsy. Epilepsia. (2014) 55:475–82. doi: 10.1111/epi.12550
2. Thijs RD, Surges R, O’Brien TJ, Sander JW. Epilepsy in adults. Lancet. (2019) 393:689–701. doi: 10.1016/S0140-6736(18)32596-0
3. Scheffer IE, Berkovic S, Capovilla G, Connolly MB, French J, Guilhoto L, et al. ILAE classification of the epilepsies: Position paper of the ILAE Commission for Classification and Terminology. Epilepsia. (2017) 58:512–21. doi: 10.1111/epi.13709
4. Duncan JS, Sander JW, Sisodiya SM, Walker MC. Adult epilepsy. Lancet. (2006) 367:1087–100. doi: 10.1016/S0140-6736(06)68477-8
5. Richard ML, Sokol H. The gut mycobiota: insights into analysis, environmental interactions and role in gastrointestinal diseases. Nat Rev Gastroenterol Hepatol. (2019) 16:331–45. doi: 10.1038/s41575-019-0121-2
6. De Caro C, Iannone LF, Citraro R, Striano P, De Sarro G, Constanti A, et al. Can we ‘seize’ the gut microbiota to treat epilepsy? Neurosci Biobehav Rev. (2019) 107:750–64. doi: 10.1016/j.neubiorev.2019.10.002
7. Peng A, Qiu X, Lai W, Li W, Zhang L, Zhu X, et al. Altered composition of the gut microbiome in patients with drug-resistant epilepsy. Epilepsy Res. (2018) 147:102–7. doi: 10.1016/j.eplepsyres.2018.09.013
8. Zhang Y, Zhou S, Zhou Y, Yu L, Zhang L, Wang Y. Altered gut microbiome composition in children with refractory epilepsy after ketogenic diet. Epilepsy Res. (2018) 145:163–8. doi: 10.1016/j.eplepsyres.2018.06.015
9. Gong X, Cai Q, Liu X, An D, Zhou D, Luo R, et al. Gut flora and metabolism are altered in epilepsy and partially restored after ketogenic diets. Microb Pathog. (2021) 155:104899. doi: 10.1016/j.micpath.2021.104899
10. Olson CA, Vuong HE, Yano JM, Liang QY, Nusbaum DJ, Hsiao EY. The gut microbiota mediates the anti-seizure effects of the ketogenic diet. Cell. (2018) 173:1728–41.e13. doi: 10.1016/j.cell.2018.04.027
11. Lindefeldt M, Eng A, Darban H, Bjerkner A, Zetterström CK, Allander T, et al. The ketogenic diet influences taxonomic and functional composition of the gut microbiota in children with severe epilepsy. NPJ Biofilms Microbiomes. (. (2019) 5:5. doi: 10.1038/s41522-018-0073-2
12. Xie G, Zhou Q, Qiu C-Z, Dai W-K, Wang H-P, Li Y-H, et al. Ketogenic diet poses a significant effect on imbalanced gut microbiota in infants with refractory epilepsy. World J Gastroenterol. (2017) 23:6164–71. doi: 10.3748/wjg.v23.i33.6164
13. Ding M, Lang Y, Shu H, Shao J, Cui L. Microbiota-gut-brain axis and epilepsy: A review on mechanisms and potential therapeutics. Front Immunol. (2021) 12:742449. doi: 10.3389/fimmu.2021.742449
14. Dong X, Fan J, Lin D, Wang X, Kuang H, Gong L, et al. Captopril alleviates epilepsy and cognitive impairment by attenuation of C3-mediated inflammation and synaptic phagocytosis. J Neuroinflamm. (2022) 19:226. doi: 10.1186/s12974-022-02587-8
15. Rana A, Musto AE. The role of inflammation in the development of epilepsy. J Neuroinflamm. (2018) 15:144. doi: 10.1186/s12974-018-1192-7
16. Yang J, Ran M, Li H, Lin Y, Ma K, Yang Y, et al. New insight into neurological degeneration: Inflammatory cytokines and blood-brain barrier. Front Mol Neurosci. (2022) 15:1013933. doi: 10.3389/fnmol.2022.1013933
17. Brandsma E, Kloosterhuis NJ, Koster M, Dekker DC, Gijbels MJJ, van der Velden S, et al. A proinflammatory gut microbiota increases systemic inflammation and A ccelerates atherosclerosis. Circ Res. (2019) 124:94–100. doi: 10.1161/CIRCRESAHA.118.313234
18. Lawlor DA, Harbord RM, Sterne JAC, Timpson N, Davey Smith G. Mendelian randomization: using genes as instruments for making causal inferences in epidemiology. Stat Med. (2008) 27:1133–63. doi: 10.1002/sim.3034
19. Burgess S, Small DS, Thompson SG. A review of instrumental variable estimators for Mendelian randomization. Stat Methods Med Res. (2017) 26:2333–55. doi: 10.1177/0962280215597579
20. Visscher PM, Wray NR, Zhang Q, Sklar P, McCarthy MI, Brown MA, et al. 10 years of GWAS discovery: biology, function, and translation. Am J Hum Genet. (2017) 101:5–22. doi: 10.1016/j.ajhg.2017.06.005
21. Meng C, Deng P, Miao R, Tang H, Li Y, Wang J, et al. Gut microbiome and risk of ischaemic stroke: a comprehensive Mendelian randomization study. Eur J Prev Cardiol. (2023) 30:613–20. doi: 10.1093/eurjpc/zwad052
22. Bottigliengo D, Foco L, Seibler P, Klein C, König IR, Del Greco MF. A Mendelian randomization study investigating the causal role of inflammation on Parkinson’s disease. Brain. (2022) 145:3444–53. doi: 10.1093/brain/awac193
23. Lin D, Zhu Y, Tian Z, Tian Y, Liang C, Peng X, et al. Causal associations between gut microbiota, gut microbiota-derived metabolites, and cerebrovascular diseases: a multivariable Mendelian randomization study. Front Cell Infect Microbiol. (2023) 13:1269414. doi: 10.3389/fcimb.2023.1269414
24. Ji D, Chen W-Z, Zhang L, Zhang Z-H, Chen L-J. Gut microbiota, circulating cytokines and dementia: a Mendelian randomization study. J Neuroinflamm. (2024) 21:2. doi: 10.1186/s12974-023-02999-0
25. Bowden J, Holmes MV. Meta-analysis and Mendelian randomization: A review. Res Synth Methods. (2019) 10:486–96. doi: 10.1002/jrsm.1346
26. Skrivankova VW, Richmond RC, Woolf BAR, Yarmolinsky J, Davies NM, Swanson SA, et al. Strengthening the reporting of observational studies in epidemiology U sing mendelian randomization: the STROBE-MR statement. JAMA. (2021) 326:1614–21. doi: 10.1001/jama.2021.18236
27. Kurilshikov A, Medina-Gomez C, Bacigalupe R, Radjabzadeh D, Wang J, Demirkan A, et al. Large-scale association analyses identify host factors influencing human gut microbiome composition. Nat Genet. (2021) 53:156–65. doi: 10.1038/s41588-020-00763-1
28. Zhao JH, Stacey D, Eriksson N, Macdonald-Dunlop E, Hedman ÅK, Kalnapenkis A, et al. Genetics of circulating inflammatory proteins identifies drivers of immune-mediated disease risk and therapeutic targets. Nat Immunol. (2023) 24:1540–51. doi: 10.1038/s41590-023-01588-w
29. Kurki MI, Karjalainen J, Palta P, Sipilä TP, Kristiansson K, Donner KM, et al. FinnGen provides genetic insights from a well-phenotyped isolated population. Nature. (2023) 613:508–18. doi: 10.1038/s41586-022-05473-8
30. Machiela MJ, Chanock SJ. LDlink: a web-based application for exploring population-specific haplotype structure and linking correlated alleles of possible functional variants. Bioinformatics. (2015) 31:3555–7. doi: 10.1093/bioinformatics/btv402
31. Myers TA, Chanock SJ, Machiela MJ. LDlinkR: an R package for rapidly calculating linkage disequilibrium statistics in diverse populations. Front Genet. (2020) 11:157. doi: 10.3389/fgene.2020.00157
32. Burgess S, Butterworth A, Thompson SG. Mendelian randomization analysis with multiple genetic variants using summarized data. Genet Epidemiol. (2013) 37:658–65. doi: 10.1002/gepi.21758
33. Pagoni P, Dimou NL, Murphy N, Stergiakouli E. Using Mendelian randomisation to assess causality in observational studies. Evid Based Ment Health. (2019) 22:67–71. doi: 10.1136/ebmental-2019-300085
34. Rees JMB, Wood AM, Burgess S. Extending the MR-Egger method for multivariable Mendelian randomization to correct for both measured and unmeasured pleiotropy. Stat Med. (2017) 36:4705–18. doi: 10.1002/sim.7492
35. Bowden J, Davey Smith G, Haycock PC, Burgess S. Consistent estimation in mendelian randomization with some invalid ins truments using a weighted median estimator. Genet Epidemiol. (2016) 40:304–14. doi: 10.1002/gepi.21965
36. Burgess S, Thompson DJ, Rees JMB, Day FR, Perry JR, Ong KK. Dissecting causal pathways using mendelian randomization with summarized genetic data: application to age at menarche and risk of breast cancer. Genetics. (2017) 207:481–7. doi: 10.1534/genetics.117.300191
37. Carter AR, Sanderson E, Hammerton G, Richmond RC, Davey Smith G, Heron J, et al. Mendelian randomisation for mediation analysis: current methods and challenges for implementation. Eur J Epidemiol. (2021) 36:465–78. doi: 10.1007/s10654-021-00757-1
38. Cohen JF, Chalumeau M, Cohen R, Korevaar DA, Khoshnood B, Bossuyt PMM. Cochran’s Q test was useful to assess heterogeneity in likelihood ratios in studies of diagnostic accuracy. J Clin Epidemiol. (2015) 68:299–306. doi: 10.1016/j.jclinepi.2014.09.005
39. Verbanck M, Chen C-Y, Neale B, Do R. Detection of widespread horizontal pleiotropy in causal relationships inferred from Mendelian randomization between complex traits and diseases. Nat Genet. (2018) 50:693–8. doi: 10.1038/s41588-018-0099-7
40. Thompson JR, Minelli C, Abrams KR, Tobin MD, Riley RD. Meta-analysis of genetic studies using Mendelian randomization–a multivariate approach. Stat Med. (2005) 24:2241–54. doi: 10.1002/(ISSN)1097-0258
41. Storey JD, Tibshirani R. Statistical significance for genomewide studies. Proc Natl Acad Sci U.S.A. (2003) 100:9440–5. doi: 10.1073/pnas.1530509100
42. Mitrea L, Nemeş SA, Szabo K, Teleky BE, Vodnar DC. Guts imbalance imbalances the brain: A review of gut microbiota association with neurological and psychiatric disorders. Front Med (Lausanne). (2022) 9:813204. doi: 10.3389/fmed.2022.813204
43. Burokas A, Arboleya S, Moloney RD, Peterson VL, Murphy K, Clarke G, et al. Targeting the microbiota-gut-brain axis: prebiotics have anxiolytic and antidepressant-like effects and reverse the impact of chronic stress in mice. Biol Psychiatry. (2017) 82:472–87. doi: 10.1016/j.biopsych.2016.12.031
44. Johnson KVA, Foster KR. Why does the microbiome affect behaviour? Nat Rev Microbiol. (2018) 16:647–55. doi: 10.1038/s41579-018-0014-3
45. Morais LH, Schreiber H, Mazmanian SK. The gut microbiota-brain axis in behaviour and brain disorders. Nat Rev Microbiol. (2021) 19:241–55. doi: 10.1038/s41579-020-00460-0
46. Ullah H, Arbab S, Tian Y, Liu C-Q, Chen Y, Qijie L, et al. The gut microbiota-brain axis in neurological disorder. Front Neurosci. (2023) 17:1225875. doi: 10.3389/fnins.2023.1225875
47. Hashimoto K. Gut-microbiota-brain axis by bile acids in depression. Psychiatry Clin Neurosci. (2022) 76:281. doi: 10.1111/pcn.13370
48. Quigley EMM. Microbiota-brain-gut axis and neurodegenerative diseases. Curr Neurol Neurosci Rep. (2017) 17:94. doi: 10.1007/s11910-017-0802-6
49. Wang H-X, Wang Y-P. Gut microbiota-brain axis. Chin Med J (Engl). (2016) 129:2373–80. doi: 10.4103/0366-6999.190667
50. Russo E. The gut microbiota as a biomarker in epilepsy. Neurobiol Dis. (2022) 163:105598. doi: 10.1016/j.nbd.2021.105598
51. Arulsamy A, Tan QY, Balasubramaniam V, O’Brien TJ, Shaikh MF. Gut microbiota and epilepsy: A systematic review on their relationship and possible therapeutics. ACS Chem Neurosci. (2020) 11:3488–98. doi: 10.1021/acschemneuro.0c00431
52. Oliveira MET, Paulino GVB, Dos Santos Júnior ED, da Silva Oliveira FA, Melo VMM, Ursulino JS, et al. Multi-omic analysis of the gut microbiome in rats with lithium-pilocar pine-induced temporal lobe epilepsy. Mol Neurobiol. (2022) 59:6429–46. doi: 10.1007/s12035-022-02984-3
53. Wang Y, Xie Q, Sun S, Huang B, Zhang Y, Xu Y, et al. Probiotics-fermented Massa Medicata Fermentata ameliorates weaning stress in piglets related to improving intestinal homeostasis. Appl Microbiol Biotechnol. (2018) 102:10713–27. doi: 10.1007/s00253-018-9438-y
54. Citraro R, Lembo F, De Caro C, Tallarico M, Coretti L, Iannone LF, et al. First evidence of altered microbiota and intestinal damage and their link to absence epilepsy in a genetic animal model, the WAG/Rij rat. Epilepsia. (2021) 62:529–41. doi: 10.1111/epi.16813
55. Wan L, Yang G, Zhang S, Sun Y, Li Z, Wang J, et al. Investigation of the association between imbalance of the intestinal flora and infantile spasms: a pilot case-control study. Transl Pediatr. (2021) 10:819–33. doi: 10.21037/tp
56. Devinsky O, Vezzani A, Najjar S, De Lanerolle NC, Rogawski MA. Glia and epilepsy: excitability and inflammation. Trends Neurosci. (2013) 36:174–84. doi: 10.1016/j.tins.2012.11.008
57. Vezzani A, French J, Bartfai T, Baram TZ. The role of inflammation in epilepsy. Nat Rev Neurol. (2011) 7:31–40. doi: 10.1038/nrneurol.2010.178
58. Schirmer M, Smeekens SP, Vlamakis H, Jaeger M, Oosting M, Franzosa EA, et al. Linking the human gut microbiome to inflammatory cytokine production capacity. Cell. (2016) 167:1125–36.e8. doi: 10.1016/j.cell.2016.10.020
59. Blander JM, Longman RS, Iliev ID, Sonnenberg GF, Artis D. Regulation of inflammation by microbiota interactions with the host. Nat Immunol. (2017) 18:851–60. doi: 10.1038/ni.3780
60. Belkaid Y, Hand TW. Role of the microbiota in immunity and inflammation. Cell. (2014) 157:121–41. doi: 10.1016/j.cell.2014.03.011
61. Zhao H-W, Yue Y-H, Han H, Chen X-L, Lu Y-G, Zheng J-M, et al. Effect of toll-like receptor 3 agonist poly I: C on intestinal mucosa and epithelial barrier function in mouse models of acute colitis. World J Gastroenterol. (2017) 23:999–1009. doi: 10.3748/wjg.v23.i6.999
62. Labus J, Häckel S, Lucka L, Danker K. Interleukin-1β induces an inflammatory response and the breakdown of t he endothelial cell layer in an improved human THBMEC-based in vitro b lood-brain barrier model. J Neurosci Methods. (2014) 228:35–45. doi: 10.1016/j.jneumeth.2014.03.002
63. Rustenhoven J, Jansson D, Smyth LC, Dragunow M. Brain pericytes as mediators of neuroinflammation. Trends Pharmacol Sci. (2017) 38:291–304. doi: 10.1016/j.tips.2016.12.001
64. Armulik A, Genové G, Mäe M, Nisancioglu MH, Wallgard E, Niaudet C, et al. Pericytes regulate the blood-brain barrier. Nature. (2010) 468:557–61. doi: 10.1038/nature09522
65. Jansson D, Rustenhoven J, Feng S, Hurley D, Oldfield RL, Bergin PS, et al. A role for human brain pericytes in neuroinflammation. J Neuroinflamm. (2014) 11:104. doi: 10.1186/1742-2094-11-104
66. Takata F, Dohgu S, Sakaguchi S, Sakai K, Yamanaka G, Iwao T, et al. Oncostatin-M-reactive pericytes aggravate blood-brain barrier dysfunct ion by activating JAK/STAT3 signaling in vitro. Neuroscience. (2019) 422:12–20. doi: 10.1016/j.neuroscience.2019.10.014
67. Salminen S, Bouley C, Boutron-Ruault MC, Cummings JH, Franck A, Gibson GR, et al. Functional food science and gastrointestinal physiology and function. Br J Nutr. (1998) 80 Suppl 1:S147–71. doi: 10.1079/BJN19980108
68. Borowicz-Reutt KK, Czuczwar SJ. Role of oxidative stress in epileptogenesis and potential implications for therapy. Pharmacol Rep. (2020) 72:1218–26. doi: 10.1007/s43440-020-00143-w
69. González-Bosch C, Boorman E, Zunszain PA, Mann GE. Short-chain fatty acids as modulators of redox signaling in health and disease. Redox Biol. (2021) 47:102165. doi: 10.1016/j.redox.2021.102165
70. Dalile B, Van Oudenhove L, Vervliet B, Verbeke K. The role of short-chain fatty acids in microbiota-gut-brain communication. Nat Rev Gastroenterol Hepatol. (2019) 16:461–78. doi: 10.1038/s41575-019-0157-3
71. Han T, Qin Y, Mou C, Wang M, Jiang M, Liu B. Seizure induced synaptic plasticity alteration in hippocampus is mediated by IL-1β receptor through PI3K/Akt pathway. Am J Transl Res. (2016) 8:4499–509.
72. Scorza CA, Marques MJG, Gomes da Silva S, Naffah-Mazzacoratti M, Scorza FA, Cavalheiro EA. Status epilepticus does not induce acute brain inflammatory response in the Amazon rodent Proechimys, an animal model resistant to epileptogenesis. Neurosci Lett. (2018) 668:169–73. doi: 10.1016/j.neulet.2017.02.049
73. Ichiyama T, Nishikawa M, Yoshitomi T, Hayashi T, Furukawa S. Tumor necrosis factor-alpha, interleukin-1 beta, and interleukin-6 in cerebrospinal fluid from children with prolonged febrile seizures. Comparison with acute encephalitis/encephalopathy. Neurology. (1998) 50:407–11. doi: 10.1212/WNL.50.2.407
74. Müller M, Carter S, Hofer MJ, Campbell IL. Review: The chemokine receptor CXCR3 and its ligands CXCL9, CXCL10 and CXCL11 in neuroimmunity–a tale of conflict and conundrum. Neuropathol Appl Neurobiol. (2010) 36:368–87. doi: 10.1111/j.1365-2990.2010.01089.x
75. Clark-Lewis I, Mattioli I, Gong J-H, Loetscher P. Structure-function relationship between the human chemokine receptor C XCR3 and its ligands. J Biol Chem. (2003) 278:289–95. doi: 10.1074/jbc.M209470200
76. Sandu RE, Uzoni A, Coman C, Popa-Wagner A. Cerebral ischemia in the aged. Limited anti-inflammatory efficacy of the indomethacin treatment. Rom J Morphol Embryol. (2015) 56:1111–7.
77. Kivisäkk P, Trebst C, Liu Z, Tucky BH, Sørensen TL, Rudick RA, et al. T-cells in the cerebrospinal fluid express a similar repertoire of inflammatory chemokine receptors in the absence or presence of CNS inflammation: implications for CNS trafficking. Clin Exp Immunol. (2002) 129:510–8. doi: 10.1046/j.1365-2249.2002.01947.x
78. Liu Y, Hou B, Zhang Y, Fan Y, Peng B, Liu W, et al. Anticonvulsant agent DPP4 inhibitor sitagliptin downregulates CXCR3/RA. Exp Neurol. (2018) 307:90–8. doi: 10.1016/j.expneurol.2018.06.004
79. Pracucci E, Pillai V, Lamers D, Parra R, Landi S. Neuroinflammation: A signature or a cause of epilepsy? Int J Mol Sci. (2021) 22:6981. doi: 10.3390/ijms22136981
80. Wheless JW. History of the ketogenic diet. Epilepsia. (2008) 49 Suppl 8:3–5. doi: 10.1111/j.1528-1167.2008.01821.x
81. Zhu H, Bi D, Zhang Y, Kong C, Du J, Wu X, et al. Ketogenic diet for human diseases: the underlying mechanisms and potential for clinical implementations. Signal Transduct Target Ther. (2022) 7:11. doi: 10.1038/s41392-021-00831-w
82. Conlon MA, Bird AR. The impact of diet and lifestyle on gut microbiota and human health. Nutrients. (2014) 7:17–44. doi: 10.3390/nu7010017
83. Bagheri S, Heydari A, Alinaghipour A, Salami M. Effect of probiotic supplementation on seizure activity and cognitive performance in PTZ-induced chemical kindling. Epilepsy Behav. (2019) 95:43–50. doi: 10.1016/j.yebeh.2019.03.038
84. Wang X, Ma R, Liu X, Zhang Y. Effects of long-term supplementation of probiotics on cognitive function and emotion in temporal lobe epilepsy. Front Neurol. (2022) 13:948599. doi: 10.3389/fneur.2022.948599
85. Gómez-Eguílaz M, Ramón-Trapero JL, Pérez-Martínez L, Blanco JR. The beneficial effect of probiotics as a supplementary treatment in drug-resistant epilepsy: a pilot study. Benef Microbes. (2018) 9:875–81. doi: 10.3920/BM2018.0018
86. Vindigni SM, Surawicz CM. Fecal microbiota transplantation. Gastroenterol Clin North Am. (2017) 46:171–85. doi: 10.1016/j.gtc.2016.09.012
87. He Z, Cui B-T, Zhang T, Li P, Long C-Y, Ji G-Z, et al. Fecal microbiota transplantation cured epilepsy in a case with Crohn’s disease: The first report. World J Gastroenterol. (2017) 23:3565–8. doi: 10.3748/wjg.v23.i19.3565
88. Zhang L, Jing J, Han L, Wang J, Zhang W, Liu Z, et al. Characterization of gut microbiota, metabolism and cytokines in benzene-induced hematopoietic damage. Ecotoxicol Environ Saf. (2021) 228:112956. doi: 10.1016/j.ecoenv.2021.112956
89. Zhang M-N, Xie R, Wang H-G, Wen X, Wang J-Y, He L, et al. Cepharanthine alleviates DSS-induced ulcerative colitis via regulating aconitate decarboxylase 1 expression and macrophage infiltration. Molecules. (2023) 28:1060. doi: 10.3390/molecules28031060
90. Misiak B, Pawlak E, Rembacz K, Kotas M, Żebrowska-Różańska P, Kujawa D, et al. Associations of gut microbiota alterations with clinical, metabolic, and immune-inflammatory characteristics of chronic schizophrenia. J Psychiatr Res. (2024) 171:152–60. doi: 10.1016/j.jpsychires.2024.01.036
91. Zhang Y, Wang X-L, Zhou M, Kang C, Lang H-D, Chen M-T, et al. Crosstalk between gut microbiota and Sirtuin-3 in colonic inflammation and tumorigenesis. Exp Mol Med. (2018) 50:1–11. doi: 10.1038/s12276-017-0002-0
Keywords: gut microbiota, epilepsy, inflammatory proteins, Mendelian randomization, microbiota-gut-brain-axis
Citation: Yang H, Liu W, Gao T, Liu Q, Zhang M, Liu Y, Ma X, Zhang N, Shi K, Duan M, Ma S, Zhang X, Cheng Y, Qu H, Chen M and Zhan S (2024) Causal associations between gut microbiota, circulating inflammatory proteins, and epilepsy: a multivariable Mendelian randomization study. Front. Immunol. 15:1438645. doi: 10.3389/fimmu.2024.1438645
Received: 26 May 2024; Accepted: 22 August 2024;
Published: 09 September 2024.
Edited by:
Jiajia Song, Southwest University, ChinaReviewed by:
Laura Mitrea, University of Agricultural Sciences and Veterinary Medicine of Cluj-Napoca, RomaniaCopyright © 2024 Yang, Liu, Gao, Liu, Zhang, Liu, Ma, Zhang, Shi, Duan, Ma, Zhang, Cheng, Qu, Chen and Zhan. This is an open-access article distributed under the terms of the Creative Commons Attribution License (CC BY). The use, distribution or reproduction in other forums is permitted, provided the original author(s) and the copyright owner(s) are credited and that the original publication in this journal is cited, in accordance with accepted academic practice. No use, distribution or reproduction is permitted which does not comply with these terms.
*Correspondence: Shuqin Zhan, c3F6aGFuQG1haWwueGp0dS5lZHUuY24=
Disclaimer: All claims expressed in this article are solely those of the authors and do not necessarily represent those of their affiliated organizations, or those of the publisher, the editors and the reviewers. Any product that may be evaluated in this article or claim that may be made by its manufacturer is not guaranteed or endorsed by the publisher.
Research integrity at Frontiers
Learn more about the work of our research integrity team to safeguard the quality of each article we publish.