- 1Dr. Neher’s Biophysics Laboratory for Innovative Drug Discovery, State Key Laboratory of Quality Research in Chinese Medicine, Macau Institute for Applied Research in Medicine and Health, Macau University of Science and Technology, Macao, Macao SAR, China
- 2Intervention and Cell Therapy Center, Peking University Shenzhen Hospital, Shenzhen, China
- 3Gynecology Department, Zhuhai Hospital of Integrated Traditional Chinese and Western Medicine, Zhuhai, China
With the COVID-19 pandemic, the importance of vaccines has been widely recognized and has led to increased research and development efforts. Vaccines also play a crucial role in cancer treatment by activating the immune system to target and destroy cancer cells. However, enhancing the efficacy of cancer vaccines remains a challenge. Adjuvants, which enhance the immune response to antigens and improve vaccine effectiveness, have faced limitations in recent years, resulting in few novel adjuvants being identified. The advancement of artificial intelligence (AI) technology in drug development has provided a foundation for adjuvant screening and application, leading to a diversification of adjuvants. This article reviews the significant role of tumor vaccines in basic research and clinical treatment and explores the use of AI technology to screen novel adjuvants from databases. The findings of this review offer valuable insights for the development of new adjuvants for next-generation vaccines.
1 Introduction
In the field of drug discovery and screening, artificial intelligence (AI) has become a dominant method in the community due to its unique advantages (1). Firstly, the AI-based process significantly reduces the time and resources required for the research because it can quickly screen, design, and optimize millions of compound structures with enhanced prediction accuracy (2, 3). Moreover, AI facilitates studying specific targets and discovering suitable target compounds, often called the “automation” process (4). Cancer vaccines that use tumor antigens and adjuvants to identify and eliminate cancer cells are becoming a promising approach (5, 6). As the main component of cancer vaccines, adjuvants mainly enhance the vaccine’s immune response speed, intensity, and persistence (7). At present, a variety of proteins/receptors are being studied as potential targets for vaccine adjuvant design, including Toll-like receptor (TLR) (8, 9), Stimulator of interferon genes (STING) (10, 11), Indoleamine 2, 3-dioxygenase (IDO) (12), Programmed Cell Death Protein 1 (PD-1)/Programmed Cell Death Ligand 1 (PD-L1) (13) and so on. Although many potential adjuvants have been evaluated in preclinical studies, the number of adjuvants successfully converted to approved vaccines is still minimal (14). With the researchers’ mastery of the immune system and vaccine technology, it has been found that the classical licensed adjuvants have limited applicability to cancer antigens, especially nucleic acid antigens, because of their inefficiency. It can no longer meet the requirements of current cancer immunotherapy. Therefore, the integration of AI technology and tools to promote the generation of novel adjuvants is required. The limitations of adjuvants have many causes, including the small number of currently approved adjuvants for clinical use and the significant barriers to translating new adjuvants from the laboratory to clinical applications. The high cost of clinical approval for new adjuvants and the preference of developers for existing adjuvants due to their lower regulatory risk are also contributing factors (15). To address this issue, the Adjuvant Development Program of the National Institutes of Health (NIH) has provided crucial funding and support for the development and application of new adjuvants in recent years (16, 17). Another pressing issue is the low response rate of adjuvants. Specifically, why do adjuvants added to vaccines fail to work effectively or meet expectations? In our literature review, we found that this problem is mainly because many adjuvants do not translate well from animal studies to clinical studies. For example, there are significant differences in TLRs (Toll-like receptors) between different species (18). Therefore, it is crucial to screen potential TLR agonists in appropriate animal models while considering species differences. AI and machine learning are transforming multiple fields, including vaccine adjuvant development. For instance, in the study of new human TLR9 ligands, A. Ahuja et al. developed a customized software program called the ‘Synthetic Chemist.’ Specifically, 1016 synthetic oligonucleotides were generated using a computer, and a second machine learning program, ‘Search Algorithms for Ligands,’ was used to screen these ligands to find the best pan-species TLR9 ligand (CPG55.2) (19, 20). Subsequently, CPG55.2 was shown to be active across multiple species and successfully used in the development of vaccines for influenza (21), malaria (22), herpes simplex virus type 2 infection (23), poliovirus (24), and COVID-19 (25, 26). This example illustrates the advantages of using AI to screen adjuvants and demonstrates how the developed software has played an important role in advancing new adjuvants in practical applications. This article summarizes prospective tactics and technology for generating cancer vaccine adjuvants, which can be helpful in the development of cancer vaccines.
2 Status and challenges of cancer vaccine adjuvants
Cancer vaccine adjuvants are substances used in cancer vaccines to enhance the immune system’s response to antigens. They play a crucial role in improving the efficacy of cancer vaccines by enhancing the body’s immune response to cancer cells. Like an experienced coach, they are gradually rewriting the rules of immunotherapy (27). Adjuvants can help present antigens to the immune system, stimulate the production of cytokines, and activate various immune cells, making them an essential component in the development of effective cancer vaccines (28).In recent years, scientists have been continuously exploring how to best utilize these “coaches” to enhance patients’ immune responses through precise immunological data and personalized treatment plans. To ensure safety and efficacy, ideal cancer vaccine adjuvants should possess several key characteristics: low or no toxicity, minimal side effects, the ability to enhance the immune response to vaccine antigens, specificity, stability, and ease of large-scale production. In a groundbreaking study, researchers developed a nanoparticle-based adjuvant that showed remarkable results in clinical trials, achieving unprecedented therapeutic outcomes by enhancing antigen presentation and stimulating immune cells (29, 30). Cancer vaccine adjuvants can be broadly classified based on their mechanisms and sources into the following categories: biological adjuvants, chemical adjuvants, particulate adjuvants, and combination adjuvants.
2.1 Biological adjuvants
Biological adjuvants are natural or synthetic biological molecules that enhance vaccine efficacy by modulating the immune system. These adjuvants can enhance immune responses through various mechanisms, including enhancing antigen presentation, activating immune cells, and promoting cytokine production.
2.1.1 Cytokines
GM-CSF (Granulocyte-Macrophage Colony-Stimulating Factor) is an important cytokine that enhances immune responses by promoting the maturation and function of dendritic cells and macrophages. It is widely used in cancer vaccines to enhance antigen presentation and T cell activation (31). Provenge (Sipuleucel-T), the first FDA-approved therapeutic cancer vaccine, uses GM-CSF as an adjuvant to enhance antigen presentation by dendritic cells, used for treating prostate cancer (32). DCVax®-L, a personalized dendritic cell vaccine for glioblastoma, combines the patient’s own tumor antigens with GM-CSF, significantly extending patient survival (33).
IL-12 (Interleukin-12) is another key cytokine that induces Th1 immune responses, promoting the activation of cytotoxic T cells and NK cells, thereby enhancing immune attacks on tumor cells. NKTR-214 (Bempegaldesleukin) is an IL-2 pathway agonist that enhances T cell and NK cell activity. It is used in combination with immune checkpoint inhibitors to treat various solid tumors and can provide long-lasting immune responses to prevent tumor recurrence when combined with anti-CTLA-4 antibodies (34).
2.1.2 Microbial components
BCG (Bacillus Calmette-Guérin) is an attenuated live vaccine derived from Mycobacterium bovis, widely used for bladder cancer immunotherapy (35). Initially developed to prevent tuberculosis, BCG is used as an immunoadjuvant in cancer immunotherapy due to its ability to stimulate the innate immune system, activate macrophages and dendritic cells, and promote antigen presentation and specific T cell responses (36).
CpG (Cytosine-phosphate-Guanine) oligodeoxynucleotides are synthetic molecules that activate TLR9, inducing strong Th1 immune responses, promoting dendritic cell maturation, and enhancing antigen presentation. Several new adjuvants, including TLR3, TLR7, and TLR9 agonists, are undergoing clinical trials to enhance the efficacy of immune checkpoint inhibitors and improve cancer vaccine responses (37).
2.1.3 Other biological molecules
Mitogens, such as superantigens and bacterial toxins, can strongly activate T cells and enhance immune responses. For example, Tetanus Toxoid Fragment C (TTFC) is used as an effective adjuvant to enhance the efficacy of various vaccines (38).
2.2 Chemical adjuvants
Chemical adjuvants are synthetically designed substances aimed at enhancing immune responses. They improve vaccine efficacy through various mechanisms, including enhancing antigen uptake, activating immune cells, and promoting inflammatory responses. The in-depth study and application of chemical adjuvants have significantly improved vaccine efficacy, providing more options and possibilities, especially in cancer vaccine development.
2.2.1 Aluminum salts (Alum)
Aluminum salts are among the earliest and most widely used vaccine adjuvants. They enhance immune responses by forming an antigen depot, prolonging antigen presence in the body, and promoting uptake by antigen-presenting cells (APCs) (39). Aluminum salts are widely used in various vaccines, including DTP (Diphtheria, Tetanus, Pertussis) vaccines (40), hepatitis B vaccines (41), and human papillomavirus (HPV) vaccines (42). In some cancer vaccines, aluminum salts are used as adjuvants to enhance immune responses. For instance, aluminum adjuvants in HPV vaccines such as Gardasil and Cervarix improve vaccine immunogenicity and effectively prevent HPV-related cancers (43).
2.2.2 Saponins
Saponins, such as Quillaja saponaria extract QS-21, are the only saponin adjuvant approved for clinical use in humans and have demonstrated strong immunostimulatory activity. Traditional extraction methods for QS-21 are time-consuming and yield low amounts (44). Recently, Liu et al. developed a novel method to synthesize QS-21 analogs, laying a rational foundation for effective vaccine adjuvants (45).
2.3 Particulate adjuvants
2.3.1 PLGA nanoparticles
PLGA (Polylactic-co-glycolic acid)) is a biodegradable polymer commonly used to prepare nanoparticles. PLGA nanoparticles enhance immune responses by controlling antigen release rates, increasing antigen stability, and promoting uptake by antigen-presenting cells (46). PLGA nanoparticles have been studied in various vaccines, including rabies and tuberculosis vaccines, showing significant immune-enhancing effects (47, 48). Additionally, PLGA is often used as a stabilizer for solid particle-stabilized emulsions (Pickering emulsions) (49). Due to its high stability, biocompatibility, and large loading capacity, Pickering emulsions have been widely used in biomedicine. PLGA-based Pickering emulsions can adhere to cell membranes, increasing contact areas (50).
2.3.2 Liposomes
Liposomes are tiny vesicles formed by phospholipid bilayers that can effectively encapsulate and deliver antigens and adjuvants. Liposomes enhance immune responses by improving antigen delivery, promoting uptake by antigen-presenting cells, and providing antigen protection (51). Liposomes are widely used in various vaccines, including influenza and COVID-19 vaccines. For example, mRNA vaccines (such as Pfizer-BioNTech’s COVID-19 vaccine) use liposomes as delivery systems, significantly improving vaccine immunogenicity and protective efficacy (52). Liposome adjuvants show promising applications in vaccine development. For instance, a study indicated that using liposomes as adjuvants in influenza vaccines significantly improved immune responses in elderly populations (53).
2.4 Combination adjuvants
Combination adjuvants use multiple adjuvants together to achieve synergistic effects and enhance vaccine immune responses. This strategy aims to utilize complementary mechanisms of different adjuvants to provide stronger and longer-lasting immune protection compared to single adjuvants.
2.4.1 Combination of cytokines and nanoparticles
Cytokines such as GM-CSF can enhance the function of antigen-presenting cells (APCs) and promote antigen presentation. Nanoparticles can efficiently deliver antigens and adjuvants to immune cells and provide sustained immune stimulation through controlled release mechanisms. A study combined GM-CSF with PLGA nanoparticles for melanoma vaccine development, showing that this combination significantly enhanced vaccine immunogenicity and anti-tumor efficacy (54).
2.4.2 Combination of multiple nanoparticles
Different types of nanoparticles (e.g., gold nanoparticles and PLGA nanoparticles) can enhance immune responses through different mechanisms. Gold nanoparticles can improve antigen stability and delivery efficiency, while PLGA nanoparticles provide sustained immune stimulation. Research indicated that combining gold nanoparticles and PLGA nanoparticles in liver cancer vaccines significantly enhanced antigen-specific T cell responses and anti-tumor activity (55).
2.4.3 Combination of TLR agonists and liposomes
TLR agonists (e.g., CpG oligodeoxynucleotides) can activate the innate immune system, promote antigen presentation, and T cell activation. Liposomes, as delivery systems, can effectively encapsulate and deliver antigens and adjuvants, protecting them from degradation. In HPV-related cancer vaccine research, combining TLR9 agonist CpG with liposomes significantly enhanced vaccine immune responses and protective efficacy. MPL, a TLR4 ligand derived from LPS, enhances the ability to prevent tuberculosis in mice and crab-eating monkeys when present in the Ag85B-ESAT-6—DDA liposome formulation (56).
Recent research has explored various novel adjuvants and delivery systems to enhance the efficacy of cancer vaccines. For instance, studies have shown that using bispecific antibodies (i.e., antibodies that target both tumor cells and immune cells simultaneously) can enhance immune responses against cancer. Bispecific antibodies have demonstrated significant potential in targeting tumor-associated antigens and activating immune cells, thereby improving therapeutic outcomes (57).
Despite the crucial role of adjuvants in vaccine development, their use and development still face many challenges. Safety issues have always been paramount in drug development, as many adjuvants can cause local or systemic reactions or autoimmune diseases. Therefore, it is necessary to develop new adjuvants that reduce side effects and increase safety. For example, optimizing dosage and administration methods, as well as conducting extensive clinical trials for validation, are important. Individual differences in immune responses also need to be considered. Due to differences in genetics (58), environment (59), age (60), sex (61), occupation (62), and other factors, individuals may exhibit varying intensities and types of immune responses when receiving the same vaccine or being exposed to the same pathogen.
Additionally, the production processes for adjuvants are complex, and quality control often presents significant challenges, with variations between different batches. Thus, it is crucial to establish and adhere to strict standardized procedures and employ advanced quality control techniques. Besides these issues, adjuvants also face challenges such as insufficient immune persistence, high costs, and inadequate regulation, all of which need to be addressed and improved.
3 Online available algorithms and databases of AI Drug Discovery
Recently, many algorithms and databases have been rapidly established for AIDD. For example, DeepChem, a Multi-Layer Perceptron (MLP) model primarily using Python AI system to search for suitable drug candidates in drug discovery (63), and DeepTox, a software capable of predicting the toxicity of 12,000 drugs (64). For the development of small molecule drugs, there is a database (https://smpdb.ca/) that includes 49,833 pathways that can be found in databases that are not accessible through other routes (65). Furthermore, Shtar and colleagues have established the continuously updated Continuous Drug Combination Database (CDCDB). In this database, they use various methods, including natural language processing, to improve the drug combination discovery process and ensure the database is applicable for predicting drug synergies (66). In sum, we have summarized other related databases and open-source tools in Table 1.
4 AIDD assists in the discovery of novel adjuvants
Depending on different technical routes and functions, AIDD could be divided into five subgroups, including De novo design, Virtual screening, Properties and Toxicity prediction, and Drug repurposing.
4.1 The “De novo design” in the discovery of adjuvant
The stages of drug design have always been a focal point in AI. “De novo design” is an indispensable aspect of using AI in drug development. Since the introduction of the first “De novo design” software, HSITE/2D Skeletons, which was developed in the 1980s (95–97), it has gradually replaced traditional methods in drug discovery design, encompassing scoring, assembly, and search strategies. It has been continuously used until today (98). Based on it, Popova et al. designed a reinforcement deep learning algorithm called ReLeaSE (https://github.com/isayev/ReLeaSE). This algorithm includes deep neural networks (DNN) for compound generation and prediction of compound properties (99). Open-sourced tools for finding the optimal pathways of target molecule synthesis were also available, called AiZynthFinder (https://github.com/MolecularAI/aizynthfinder) (100).
To focus on exploring small molecule adjuvants for cancer vaccines, a practical and highly efficient tool to find specific inhibitors or agonists is necessary. Zhavoronkov et al. designed a tool called Generative Tensor Reinforcement Learning (GENTRL) for small molecule “De novo design”. GENTRL optimizes synthesis feasibility, novelty, and biological activity. Using GENTRL, they discovered a potent inhibitor of Discoidin Domain Receptor 1 (DDR1), a kinase target associated with fibrosis and other diseases, within 21 days (101). Chemistry42 is a platform for de novo small molecule design and optimization. It combines AI and medicinal chemistry to generate novel structural molecules efficiently with in vitro and in vivo validation. These approaches have effectively validated for DDR1 and CDK20 (102). All these advancements provide significant assistance to AIDD or adjuvant identification.
4.2 Virtual screening
Virtual screening technology is widely applied to facilitate the screening of large compound libraries based on computer simulations and molecular modeling techniques (103), thus contributing to drug high-throughput screening (HTS) (104). It allows for assessing the biological activity of candidate compounds based on their chemical structures, thus predicting their interactions with specific targets (105). Therefore, it enables the rapid identification of potentially active compounds, saving time and resources during the early stages of drug development (106). Traditional HTS often faces high costs, time-consuming processes, and limited sample quantities (107). This fast and efficient screening provides crucial support to drug development and advances the progress of drug discovery (108).
There are two main technical routes for virtual screening, including Ligand-based or Structure-based virtual screening (LB/SBVS), which are all primarily attributed to the advancements in extensive databases of protein and chemical structures. With improved computational power and the accumulation of large compound libraries, virtual screening can more accurately predict the biological activity and efficacy of candidate compounds (109). This has led to successful discoveries of new bioactive molecules, particularly in drug repurposing (110).
4.2.1 Ligand-based virtual screening
LBVS is a computer-assisted drug discovery method that predicts compounds’ binding ability and affinity by simulating their interactions with protein targets (111). This screening approach is commonly used to identify potential drug candidates, particularly for drug discovery targeting known protein targets. It typically involves data preparation, ligand preprocessing, target preprocessing, molecular docking, and result analysis (112). For instance, Luca et al. recently utilized LBVS to identify an effective α-Syn amyloid formation inhibitor, MeSC-04 and demonstrated its binding mode. This work provided new insights for developing α-Syn amyloid inhibitors from synthetic sources (113). Zarei et al. employed LBVS on the RON receptor homology model to screen the ZINC database, identifying two compounds, TKI1 and TKI2, further evaluated in vitro. This study laid the foundation for novel RON inhibitors applicable in cancer therapy and targeting CXCL12 (114). This small pro-inflammatory chemokine plays a significant role in tumor formation by binding to the specific receptor CXCR4. Using LBVS, Haider et al. identified three potential anti-cancer CXCL12 inhibitors (115). The above examples illustrate that LBVS holds extensive prospects for application. Its emergence provides researchers with an efficient, rapid, and cost-effective approach to identifying potential candidate compounds, thus advancing the development of computer-assisted research in this field.
4.2.2 Structure-based virtual screening
SBVS approach relies on the three-dimensional structure of the target protein, often obtained through X-ray crystallography (116) or nuclear magnetic resonance (NMR) spectroscopy (117). SBVS is widely used to identify potential drug candidates and has proven to be an effective tool in the early-stage drug discovery process (118). It typically involves molecular docking, scoring, and filtering to prioritize compounds with high binding affinity and potential therapeutic activity against the target protein. Thus, SBVS significantly accelerates the drug discovery process by reducing the number of compounds that need to be experimentally tested (119). Here, we have presented numerous examples of drug screening based on SBVS, demonstrating its importance in enhancing drug screening efficiency and improving the accuracy of candidate drug selection. For instance, Mukherjee et al. discovered compound CID 88265020 with significant potential as a VGFR inhibitor among over 80 virtual screening compounds, which could be used for prospective future studies in ovarian cancer (120). Xie et al., based on the importance of FOXM1 in ovarian cancer treatment, selected XST-119 through SBVS and found that XST-119 exhibited apparent inhibitory activity in a xenograft mouse model, laying the foundation for further drug discovery (121). G9a, a lysine methyltransferase, was investigated by Bellver-Sanchis et al., who proposed a candidate G9a inhibitor, referred to as compound F., providing a lead for G9a inhibitor design and demonstrating their involvement in reducing Alzheimer’s disease (AD) (122). Guo et al. discovered novel Tropomyosin receptor kinases A (TrkA) allosteric inhibitors through SBVS and identified a promising hit (D5261, TrkA cell IC50 = 3.32 μM). Their results suggest that D5261 could be a starting point for developing TrkA allosteric inhibitors (123). Lin et al. reported a novel non-covalent Bruton tyrosine kinase (BTK) inhibitor that could potentially target malignant tumors, laying the foundation for developing effective BTK inhibitors for solid tumors (124). Luo et al. performed PD-L1 screening on 52,765 marine natural products and identified compound 51320 as a PD-L1 small molecule inhibitor through SBVS and other methods (125). Similarly, Ge et al. identified two compounds through SBVS that could serve as scaffolds for IDO1 inhibitors (126).
Furthermore, the SBVS process involves the interaction between molecules and proteins, making it indispensable for studying molecular mechanisms within living organisms. It contributes to uncovering the foundations of diseases and potential therapeutic pathways. In summary, with the continuous expansion of computer model training, SBVS is poised to become one of the critical tools in drug discovery.
4.3 Predicting the physicochemical properties and biological activities of compound
4.3.1 Quantitative Structure-Activity Relationship
QSAR modeling is one of the most popular computer-aided tools in drug chemistry for drug discovery and lead compound optimization. It involves four main steps: selecting appropriate molecules, model construction, model validation, and model application (127). Since its introduction in the 1960s, QSAR modeling has been continuously refined and updated (128). One of the most representative 3D QSAR models is Comparative Molecular Field Analysis (CoMFA), considered the most classical and widely used. However, its development has been limited by certain drawbacks (129, 130). To address these limitations, Wang and colleagues created the Cloud 3D-QSAR server, which can facilitate the development of robust QSAR models in drug discovery (131). With the advancement of QSAR techniques, applying 4D, 5D, 6D, and 7D models is becoming more prominent, as these methods can provide additional information on small molecules beyond traditional 3D-QSAR (132).
4.3.2 Predicting physical and chemical properties and biological activity
The physical and chemical properties of compounds determine their binding efficiency with targets, and predicting the physicochemical properties and biological activities of drugs can expedite the decision-making process.
Currently, numerous software programs are utilized for predicting the physicochemical properties and biological activities of drugs. JunctionTree VAE (Variational Autoencoder) is an open-source tool capable of predicting compound properties (133). Conv_qsar_fast is another open-source toolkit that uses CNN (Convolutional Neural Network) methods to predict molecular properties (134). Additionally, InnerOuterRNN utilizes internal and external recursive neural networks to predict physical, chemical, and biological properties (135). In conclusion, predicting the physicochemical properties and biological activities of drugs by AIDD can significantly enhance the efficiency and success rate of research and provide valuable tools and resources for scientists, accelerating the discovery and development of new medicines.
4.4 Prediction of compound toxicity
Toxicity testing is an essential but resource-consuming task for drug discovery (136), and utilizing AIDD in toxicity prediction can reduce the need for animal experiments (137, 138). To address this challenge, various tools have been developed. One of the most classic tools is Deep Tox, which employs deep learning methods to predict compound toxicity (139). Furthermore, eToxPred is a software capable of evaluating candidate compound toxicity based on machine learning methods (140). TargeTox can also predict drug safety related to toxicity (141). Therefore, compound toxicity prediction is crucial in drug development, contributing to improved research efficiency and risk reduction and providing robust support for drug safety assessment.
4.5 Drug repurposing
Drug repurposing, also known as drug repositioning (142), is categorized into early-stage and late-stage repurposing. The former involves the identification of potential lead compounds through large-scale screening of compound libraries (143); the latter is used to complete the preparation of lead compounds. For clinical testing and evaluation (144).
Drug repurposing has shown many successful examples: Aspirin was initially introduced by Bayer in 1899 as an analgesic and was first repositioned in the 1980s as an antiplatelet aggregation drug using low doses (145). With further research, scientists have revealed the therapeutic role of aspirin in the field of cancer, especially in colorectal cancer (146), where its anticancer effect is believed to result from the inhibition of COX-2, thereby blocking the anti-apoptotic effect of COX-2 in malignant cells and promoting their apoptosis (147). The second is Propranolol, originally a classic drug for treating angina and hypertension, which has recently been discovered to be effective in the treatment of osteoporosis and melanoma (148). As is well known, the chiral drug Sildenafil was once withdrawn from the market due to its teratogenic effects. However, a serendipitous discovery revealed that Sildenafil has a significant therapeutic impact on erythema nodosum leprosum (a type of autoimmune complication of leprosy) by inhibiting the synthesis of the pro-inflammatory cytokine tumor necrosis factor-alpha (TNF-α). Therefore, in 1998, it was repositioned by Celgene as an orphan drug for treating leprosy complications of leprosy (149). However, these cases are often based on serendipitous clinical discoveries and lack systematic patterns. Therefore, it is essential to utilize network-based approaches to discover the potential molecular mechanisms of drug actions (150).
Currently, there are several databases available for drug repurposing. For instance, the NCGC Pharmaceutical Collection (NPC) (http://tripod.nih.gov/npc/) is suitable for screening and research on numerous diseases, and it minimizes false negatives and false positives (151). DrugBank (www.drugbank.ca) is a network-based database that provides comprehensive molecular information about drugs, drug mechanisms, drug interactions, and their targets. It can offer crucial clues for drug repurposing (77). Moreover, Drugs@FDA and ClinicalTrial.gov provide the latest updates on drugs in clinical development, presenting new research directions and systematic applications in AIDD.
5 Big data improves the efficiency of adjuvant identification
The precision of AI-based drug screening is crucial to reduce the use of animal models. Big data technology and machine algorithms provide us with significant advantages. Optimizing AI models’ algorithms and parameters can improve predictive accuracy. Specifically, data preprocessing and standardization are essential (152). Standardizing and unifying data formats ensure comparability across different data sources. Additionally, addressing noise and missing values in the data can enhance its quality (153). On this foundation, model selection and extensive training are indispensable steps. Cross-validation and hyperparameter methods can improve model prediction accuracy (154, 155). Once we obtain raw data, converting it into features suitable for supervised learning, known as feature engineering, is necessary. This process includes feature creation, transformation, extraction, exploratory data analysis, and benchmarking, aimed at simplifying and accelerating data transformation while improving model accuracy (156). Finally, small-scale experimental validation of adjuvants generated by AI screening, followed by model adjustments based on the prediction results, can enhance screening precision. Currently, machine learning-based predictive models can leverage existing experimental and clinical data for training, thereby reducing the need for animal experiments (157).
6 Successful examples of different targets
AI has aided researchers in streamlining the intricate process of identifying candidate compounds on various targets. Leveraging AI, novel adjuvants have been developed for several diverse functional targets (Figure 1). We will highlight the research progress of adjuvants in some of the most valuable target categories. The upper part of the figure, from left to right, describes how adjuvants are designed, screened, quantitatively structured, and eventually repurposed and added to vaccines. When a vaccine containing AI-screened adjuvants is injected into a patient, it triggers a series of immune responses, stimulating immune cells to produce and release cytokines or target and attack tumors.
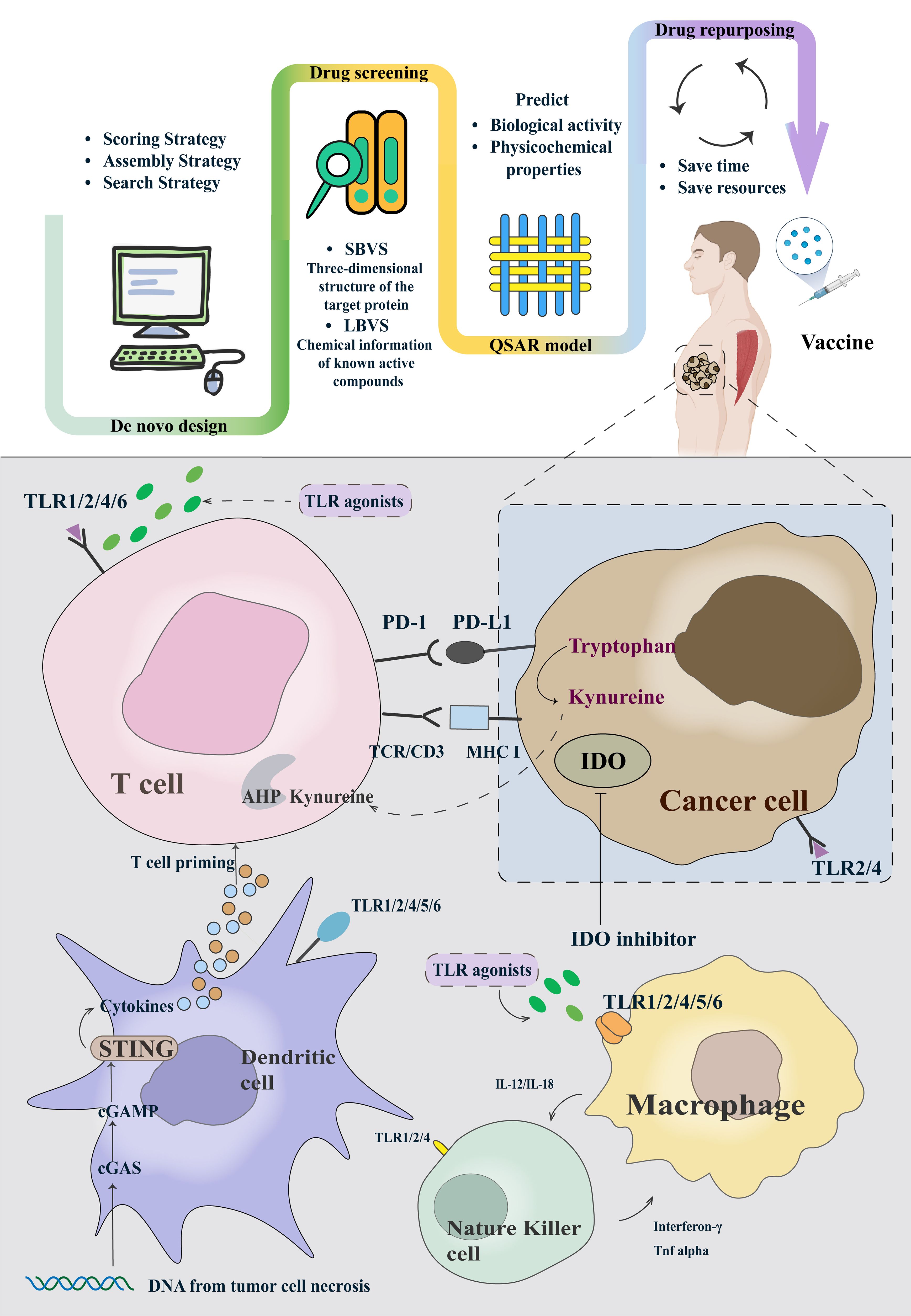
Figure 1. The framework of AIDD of adjuvants and typical examples of cancer vaccine adjuvants based on the clinical trials.
6.1 Toll-like receptor family
The TLR family plays a crucial role in the innate immune system, typically serving as a class of pattern recognition receptors (PRRs) capable of recognizing specific molecular patterns associated with pathogens such as bacteria, viruses, and fungi (158). Specifically, when TLRs recognize one of these patterns, they trigger an immune response to protect the body from infection (159).
Due to these characteristics of TLRs, their agonists are widely used as adjuvants in cancer vaccines to enhance the immune response (14). We have summarized some of the relevant clinical advancements of TLRs in Table 2. Depending on the recognized molecular patterns, they can be categorized into four classes: Recognizing Bacterial Molecules: TLR1, TLR2, TLR4, TLR5, TLR6; Recognizing Double-Stranded RNA (dsRNA): TLR3; Recognizing Single-Stranded RNA (ssRNA) from Viruses: TLR7, TLR8; Recognizing Unmethylated CpG DNA Motifs from Viral DNA: TLR9. Some representative agents are examined below.
6.1.1 TLR 3 agonist
TLR 3 is a critical recognition receptor primarily identifying dsRNA, a hallmark of viral infection. It aids in combating viral infections mainly by activating the host’s innate immune response and is widely expressed in dendritic cells, macrophages, and some epithelial cells (160). TLR 3 adjuvants have been extensively studied for their ability to induce robust type I interferon responses, thereby enhancing vaccine efficacy. Numerous studies have reported the application of TLR3 agonists. For instance, some research suggests that polyinosinic polycytidylic acid (Poly (I:C)) as a vaccine adjuvant significantly enhances immune responses and shows promising anti-tumor effects in preclinical studies (161). RECEPTOR.AI offers a TLR 3 custom library predicted by Alphafold for on-demand applications, leveraging state-of-the-art virtual screening and parameter assessment technologies. They maintain a large virtual library with over 600 billion molecules to provide compounds with increased potency, selectivity, and safety. [RECEPTOR.AI Platform] (https://www.receptor.ai/platform).
6.1.2 TLR 7/8 agonist
TLR7/8, expressed intracellularly, was first proposed in 2000 (162). They jointly recognize ssRNA, triggering the immune response to identify pathogens. TLR7 is primarily expressed in plasmacytoid dendritic cells (pDC), while TLR8 is mainly expressed in myeloid dendritic cells (163, 164). In recent years, TLR7/8 has gained popularity as an up-and-coming class of vaccine adjuvants due to their ability to directly activate antigen-presenting cells (APCs) and enhance cellular immunity.
Recent studies have utilized molecular dynamics simulations to investigate the selective mechanisms of structurally similar TLR7/8, which can be regarded as an advanced computational method (165). The integration of automation and data-driven design has further expanded the research parameters. By employing a combinatorial approach, TLR-7/8a binders that induce the desired T-cell immune responses and exhibit sufficient efficacy can be identified (166, 167). The Rapid Overlay of Chemical Structures software (vROCS version 3.1.1. OpenEye Scientific Software, Santa Fe, NM. [http://www.eyesopen.com]) is a tool used in computational chemistry and drug discovery. By comparing the shapes of millions of compounds, researchers can quickly narrow down potential candidates. Urban Švajger et al. employed the LVBS method to search for potential TLR7 ligands and discovered six new compounds, addressing the gap where only imidazoquinolines had been used as TLR7 modulators (168). To enhance the accuracy of flexible site binding, Duan et al. conducted enrichment evaluations of various virtual methods and proposed that a combination strategy can improve the effectiveness of virtual screening for TLR8 agonists (169).
However, a common drawback of TLR7/8 agonists is their reactogenicity. In recent years, many researchers have aimed to overcome this issue by encapsulating these small molecules in nanoparticles or covalently binding them to polymers. This approach can prevent harmful systemic reactions (170, 171). AI is also used to design nanomedicines that effectively deliver TLR7/8 agonists. Nanoparticle-based delivery systems can enhance the stability and targeting of these compounds, improving their efficacy and reducing side effects. This approach is particularly valuable in cancer therapy, where precise targeting of tumor cells is crucial. Research by Hyunjoon and colleagues reported that subcutaneous injection of biodegradable polymer, poly(d,l-lactide-co-glycolide) (PLGA), and nanoparticles containing TLR7/8 agonists resulted in a potent antigen-specific immune response. Their experiments validated these effects in various tumor models, demonstrating the potential of TLR7/8 as effective vaccine adjuvants for cancer immunotherapy (172).
Furthermore, studies have suggested that tethering small molecule TLR-7/8a to polymer scaffolds can enhance the immunogenicity of vaccines. The formed polymer-TLR-7/8a complexes can restrict the distribution of adjuvants and prolong their retention in lymph nodes, providing new insights for optimizing adjuvant design (172). All these findings underscore the potential therapeutic benefits of TLR7/8 as vaccine adjuvants.
6.1.3 TLR9
The innate immune system in humans can be activated by bacterial DNA (173). This discrimination is attributed to mammalian DNA having a low frequency of CpG dinucleotides, most of which are methylated (174). Recent research has shown that unmethylated CpG sequences with two purines at the 5’ end and two pyrimidines at the 3’ end are necessary for immune activation (175, 176). TLR9 was discovered to specifically recognize the unmethylated CpG and initiated host immunity (177). Based on their immune-stimulatory functions, CpGs can be divided into CpG-A, CpG-B, and CpG-C. CpG-A mainly exists in double-stranded aggregates and comprises single CpG motifs with partial phosphorothioate (PS modification) and phosphodiester main chain bases. It is primarily responsible for activating the STING pathway, inducing the production of IFN-α and IFN-β by stimulating pDC, and can indirectly activate NK cells (178). CpG-B is composed of multiple CpG motifs entirely modified with PS. It can activate the TLR9 pathway to generate pro-inflammatory cytokines. Unlike class A, class B contains B cell activators and stimulates pDC maturation. CpG-C typically consists of fully PS-modified double-stranded palindromic motifs, exhibiting immunostimulatory effects similar to the first two classes; it can induce robust IFN-α production, pDC maturation, and effective B cell stimulation (179).
Many studies have shown that CpG Oligodeoxynucleotides (ODNs) can expedite vaccine responses. For example, the hepatitis B vaccine HEPLISAV-B, approved by the FDA in 2017, was the first vaccine to use CpG-ODN 1018 as an adjuvant. Compared to the traditional aluminum hydroxide adjuvant in Engerix-B®, HEPLISAV-B induced a faster and more sustained response and significantly improved immunogenicity (180). Furthermore, CpG-ODNs can induce Th1 responses, promote the generation of cytotoxic T lymphocytes (CTLs), and enhance the secretion of IFN-γ (181). Sayami et al. found that K3 (CpG-ODN) generated more antigen-specific antibodies than other TLR ligands and induced Th1 polarization. Their report on Transcutaneous immunization also indicated that K3 could promote B cell activation and differentiation, demonstrating the promising potential of K3 as a vaccine adjuvant (182).
In sum, with the continuous advancements in the PRR field, the strategic design of PRR-targeted adjuvants has emerged as a prominent area of research. This encompasses the combination and fine-tuning of methods. Such efforts hold promise for advancing the development of both preventive and therapeutic vaccines.
6.2 STING agonists
In recent years, Stimulator of interferon genes (STING) agonist stimulators have garnered significant interest as potential vaccine adjuvants and cancer therapeutics. The STING pathway is critical for immune responses against viruses and bacteria and contributes to antitumor immunity (183). It is an endoplasmic reticulum adapter that plays an essential role in the immune system (184). Cytoplasmic DNA activates the cyclic GMP-AMP synthase (cGAS)-STING pathway in this mechanism, triggering an immune response (185). DNA binding to cGAS activates its enzymatic activity, creating conditions for the formation of cyclic dinucleotide, 2’3’-cGAMP (186). This cyclic dinucleotide (CDN) can indirectly activate transcription factors in the STING pathway, including interferon regulatory factor 3 (IRF3) and nuclear factor-kappa B (NF-κB) (187).
Most STING agonists are CDN compounds, widely proposed as adjuvants in vaccine administration and cancer therapy (188). Leveraging the characteristics of STING agonists, Fu et al. combined CDNs with a cancer vaccine called STINGVAX, finding it effective in multiple mouse tumor models (189). CDNs as adjuvants for developing infectious disease vaccines were initially validated in pneumococcus and Staphylococcus aureus infections (190, 191). Since then, many teams have explored CDN applications in contagious diseases (192). Furthermore, nano adjuvants and nanomaterials have demonstrated significant advantages in vaccine design. For instance, CDN chemical modifications, such as fluorination and thiophosphorylation on the same side, have been found to activate STING with higher stability, cellular uptake, and immunostimulatory capacity for antitumor therapy (193). Additionally, combinations of nanoscale adjuvants have shown promise in enhancing innate immunity. For example, combining TLR7/8 and STING agonists as vaccine adjuvants encapsulated in polymer nanoparticles can further improve their effectiveness, demonstrating additive effects of adjuvant combinations (194).
However, clinical outcomes of STING agonists, whether used as monotherapy or combined with immune checkpoint inhibitors, have been disappointing (195–197). This indicates the presence of unknown mechanisms leading to adverse reactions. To go further, James J’s team revealed that reversing the methylation silencing of STING in mouse melanoma cell lines using clinically available DNA methylation inhibitors can enhance agonist-induced STING activation and type I IFN induction. This, in turn, can induce tumor regression in syngeneic mice through a CD8+ T cell-dependent immune response. These findings shed light on the mechanisms of how functional impairments in STING signaling within tumor cells lead to compromised responses to STING agonist therapy (198). Moreover, the clinical application of CDN-like compounds still faces a series of inescapable challenges. Due to their damaging charge property, strong water solubility, and high polar surface area, these compounds exhibit poor membrane permeability, potentially diminishing the effectiveness of vaccines when used as adjuvants (199). In Table 3 we have also summarized the current clinical progress of STING as a vaccine adjuvant in clinical practice.
AI has made great progress in predicting and screening STING agonists as adjuvants. Ramanjulu et al. utilized high-throughput screening methods to discover ABZI (amidobenzimidazole). Intravenous injection of the STING agonist diABZI in immunocompetent mice with established syngeneic colon tumors resulted in complete tumor regression (200). Additionally, screening has shown that formulating STING agonists as nanoparticles can enhance drug stability and bioavailability (201).
6.3 Programmed Cell Death Protein 1/Programmed Death-Ligand 1 inhibitor
PD-1 and PD-L1 immune checkpoint molecules play a role in balancing immune responses in the immune system (202, 203). When excess PD-L1 is bound to PD-1 on T cells, it inhibits T cell activity, leading to a weakened immune response and immune escape (204, 205).
Typically, cancer vaccines stimulate the immune system to generate a specific response against the tumor (206). By introducing tumor-associated antigens, the vaccine can identify and target tumor cells. However, once a tumor has formed, the immune system is suppressed, limiting the therapeutic effectiveness of vaccines (207). In clinical practice, combining PD-1/PD-L1 inhibitors with tumor vaccines aims to enhance immunotherapy. PD-1/PD-L1 inhibitors as adjuvants relieve immune suppression, thereby strengthening the attack on tumors (208). There are examples in clinical trials that its antibodies and tumor vaccines have shown synergistic effects. For instance, Patrick A. et al. reported the first Phase Ib clinical trial (Clinicaltrials.gov: NCT02897765) using a neoantigen-based personalized vaccine called NEO-PV-01 in combination with PD-1 inhibitor Nivolumab to treat advanced melanoma non-small cell lung cancer, or bladder cancer (209). Compared to monoclonal antibodies, small molecules are cost-effective, have a shorter half-life, and can reduce sustained systemic side effects (210). Thus, they have become candidates for adjuvants. The design of small molecules targeting PD-1/PD-L1 typically involves disrupting protein-protein interactions (PPI) (211). Due to the lack of a prominent binding pocket, researchers have developed a series of biphenyl derivatives based on the mechanism of PD-1/PD-L1 interaction, including BMS-8, BMS-37, BMS-202, BMS-200, BMS-1001, BMS-1166 (212). PD-L1 dimerization is also a hot research area for small molecule PD-1/PD-L1 studies (213). For example, BMS’s small molecules tend to form stable dimers with PD-L1 monomers. BMS-1166 can specifically inhibit partial glycosylation of PD-L1, thereby blocking PD-L1 from moving to the Golgi apparatus from the endoplasmic reticulum, rendering it inactive (214). The first oral small molecule PD-L1 inhibitor that entered clinical trials is CA-170 (215). Its clinical trials have been conducted in lung cancer, head and neck cancer, and Hodgkin’s lymphoma (NCT02812875, clinicaltrials.gov) (CTRI/2017/12/011026, ctri.nic.in), which may mark a new era for small molecule vaccine adjuvants. In Table 4, we have summarized the clinical advancements of other PD-1/PD-L1 as small molecule adjuvants.
6.4 Indoleamine 2,3-dioxygenase inhibitor
IDO is an enzyme responsible for converting tryptophan into kynurenine. Within the immune system, the primary role of IDO is to suppress T-cell activity, helping to maintain immune balance and prevent excessive immune responses. In recent years, IDO inhibitors have drawn significant attention in tumor immunotherapy. As reported, it can delay tumor growth and enhance the effect of dendritic cell vaccines (216). They are often used with other immunotherapies, such as PD-1/PD-L1 inhibitors, to bolster the immune response. Yao et al. introduced an in situ multifunctional vaccine utilizing bacterial outer membrane vesicles (OMVs, 1-MT@OMV-Mal) designed to encapsulate IDO inhibitors internally. The in situ injection of 1-MT@OMV-Mal effectively overcomes the immune suppression induced by IDO on effector T cells infiltrating the tumor, resulting in significant inhibition of both primary and distant tumors (217). Su et al. used polymer-lipid hybrid nanovesicle (P/LNV)–based liposomes to deliver IDO inhibitors and TLR9, enhancing antigen immunogenicity and simultaneously blocking immune checkpoints. Mechanistically, this approach significantly increases the infiltration of CD8+ T cells in tumors and drains lymph nodes. Cationic liposomes delivered with tumor vaccines and IDO inhibitors provide a promising platform for cancer immunotherapy by provoking antitumor T-cell immunity and reversing the immunosuppressive tumor microenvironment (218). Troitskaya et al. demonstrated that IDO can enhance the efficacy of recombinant human milk peptide lactating (RL2) —treated cell vaccination. Their experimental results showed that additional IDO chemical inhibition exhibits better long-term antitumor responses than vaccination with RL2-treated cells alone (219).
Moreover, IDO as a cancer vaccine adjuvant also finds applications in the Human Papillomavirus (HPV) field. Recently, Pagni et al. developed a vaccine based on the genetic fusion of HSV-1 glycoprotein D (gD) with the HPV-16 E7 oncoprotein (gDE7 vaccine) for treating HPV-related tumors. They aimed to enhance the existing efficacy by incorporating an IDO inhibitor (206). In vivo experimental results showed that multitarget therapy improved the antitumor efficacy of the gDE7 protein vaccine, providing evidence for IDO as a therapeutic vaccine adjuvant for HPV tumors (220). Their earlier studies also indicated the significant role of IDO in HPV vaccines. They added two immune metabolic adjuvants, an IDO1 inhibitor, to the gDE7 vaccine and found that they enhanced the in vivo antitumor effect. In simple terms, combining IDO inhibitors with immunotherapy and reducing the negative impact of IDO1 expression on vaccine-induced protective immunity significantly increased the anticancer effect (221). Their experiments demonstrated that IDO1-targeted therapy could improve antitumor treatment by reprogramming inflammatory cells. These series of studies revealed a novel and promising approach to control HPV-related tumors and potentially other cancer types, providing substantial evidence to support further research.
Finally, a few IDO inhibitor has been tested in clinical. For example, Indoximod has been widely used in clinical settings. A phase I/II clinical trial (NCT01042535) combining indoximod with adenovirus p53-Dendritic Cells (DC) vaccine in the treatment of invasive breast cancer showed a maximum tolerated dose (MTD) of 1600 mg BID, and the two drugs demonstrated significant synergistic effects. This proves that IDO inhibitors can effectively enhance the efficacy of vaccines (222). Therefore, the strategy of using indoximod as an adjuvant for tumor vaccines to enhance anti-tumor treatment is worthy of further exploration in subsequent clinical trials. In sum, we summarized the clinical trials of potential IDO inhibitors as adjuvants for tumor vaccines currently underway and presented them in Table 5.
7 Conclusion
The development of new drugs is a lengthy and expensive process. The average cost of research and development is around $1.3 billion per drug (223). The average duration for developing oncology drugs is 13.1 years, with only 13.8% of all drug development projects ultimately gaining approval (224, 225). These challenges are often attributed to inappropriate patient selection, outdated equipment, and technological limitations. With the application of AI in drug development, it is possible to reduce these figures (226). For example, AI can use patient genetic exposure profiles to screen for potential drug target populations (226). Predictive machine learning and alternative deductive methods also contribute to forecasting lead compounds, thereby reducing development costs (226).
To reduce the costs associated with drug development, there is an increasingly close collaboration between pharmaceutical companies and the field of AI, especially in the realm of target discovery. We have compiled a summary of projects launched in partnership between select AI companies and pharmaceutical firms from 2020 to the present. These initiatives aim to develop and predict viable drugs, ultimately streamlining the process of bringing drugs into clinical trials (Figure 2). Current AI systems are mainly based on the deep learning method, the most popular machine learning method after the success of deep neural network (DNN) (227). Deep learning has been widely explored in various tasks (228–230), including drug design and molecular dynamics (MD) development (231). The MD development plays a crucial role in the small molecule adjuvant effect since it can explain how molecules interact at the atomic level during drug discovery (232). However, the MD process is known to be time-consuming and labor-intensive. The AI-based system can solve these issues and thus accelerate the MD process. For example, Drew Bennett et al. proposed an MD simulation that calculates the free energy of transferring 15,000 small molecules from water to cyclohexane (233). The research findings show that AI technology can speed up the MD simulations and enhance improvisation, however, at the cost of substantial model training, which is indispensable for deep learning-based AI systems.
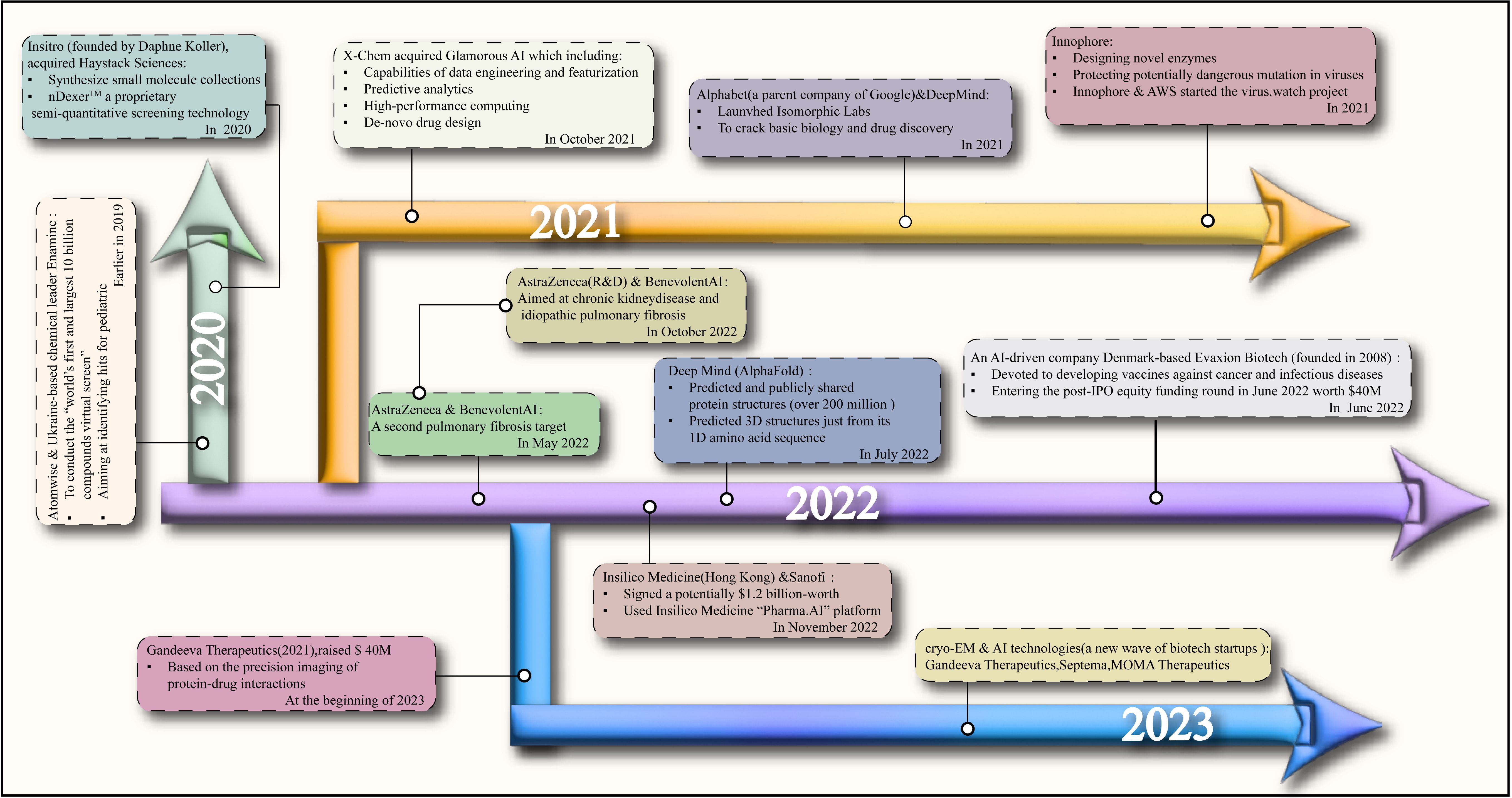
Figure 2. The streamlining of the process of bringing drugs into clinical trials and the collaboration between artificial intelligence companies and pharmaceutical companies based on the years.
The success of AI in developing adjuvants for cancer vaccines relies heavily on the availability of extensive data and well-trained models (2). Accessing various databases has introduced additional costs, and screening reliable and high-quality data is essential to ensure prediction accuracy. AI faces significant challenges in developing adjuvants for cancer vaccines and other drugs. These challenges include reducing the 2-3 year gap between prediction and drug development, a shortage of skilled personnel for AI-driven drug screening, ensuring the credibility of generated predictions, dealing with the “black box” phenomenon, and establishing reasonable regulations (234). While AI has been widely used in drug discovery and translational research over the past two decades, its progress in clinical operations and data analysis has been relatively slow.
While many challenges are associated with using AI in developing adjuvants for cancer vaccines, we anticipate that AI will become increasingly prevalent in this field, helping mitigate the risks associated with drug development (235, 236). With the development of personalized medicine, AI can also assist in selecting the most suitable adjuvants for patients to achieve personalized immunotherapy plans. This will contribute to the advancement of precision medicine. Overall, AI has accelerated the research process in developing tumor vaccine adjuvants, improving the efficiency and effectiveness of adjuvant design. It can bring more innovation and breakthroughs to the future of global vaccine research and immunotherapy.
Author contributions
WZ: Conceptualization, Data curation, Formal analysis, Investigation, Software, Visualization, Writing – original draft. XZ: Conceptualization, Data curation, Formal analysis, Investigation, Methodology, Writing – original draft. PC: Data curation, Formal analysis, Investigation, Writing – original draft. JC: Data curation, Formal Analysis, Investigation, Writing – original draft. BD: Conceptualization, Project administration, Supervision, Writing – review & editing. XF: Conceptualization, Funding acquisition, Project administration, Resources, Supervision, Visualization, Writing – review & editing.
Funding
The author(s) declare that financial support was received for the research, authorship, and/or publication of this article. This work was funded by FDCT project grant to Dr. Xing-Xing Fan (Grant no. 0058/2020/A2 and 0038/2023/RIB2).
Conflict of interest
The authors declare that the research was conducted in the absence of any commercial or financial relationships that could be construed as a potential conflict of interest.
Publisher’s note
All claims expressed in this article are solely those of the authors and do not necessarily represent those of their affiliated organizations, or those of the publisher, the editors and the reviewers. Any product that may be evaluated in this article, or claim that may be made by its manufacturer, is not guaranteed or endorsed by the publisher.
References
1. Xu W, Gao Z. Applying human-centered AI in developing effective human-AI teaming: A perspective of human-AI joint cognitive systems. ArXiv. (2023), abs/2307.03913. doi: 10.48550/arXiv.2307.03913
2. Smalley E. AI-powered drug discovery captures pharma interest. Nat Biotechnol. (2017) 35:604–5. doi: 10.1038/nbt0717-604
3. Askr H, Elgeldawi E, Ella HA, Elshaier Y, Gomaa M, Hassanien A, et al. Deep learning in drug discovery: an integrative review and future challenges. Artif Intell Rev. (2022) 56:5975–6037. doi: 10.1007/s10462-022-10306-1
4. Schneider G. Automating drug discovery. Nat Rev Drug Discovery. (2017) 17:97–113. doi: 10.1038/nrd.2017.232
5. Wolff Sagy Y, Zucker R, Hammerman A, Markovits H, Arieh NG, Ahmad WA, et al. Real-world effectiveness of a single dose of mpox vaccine in males. Nat Med. (2023) 29:748–52. doi: 10.1038/s41591-023-02229-3
6. Aguas R, Bharath A, White LJ, Gao B, Pollard A, Voysey M, et al. Potential global impacts of alternative dosing regimen and rollout options for the ChAdOx1 nCoV-19 vaccine. Nat Commun. (2021) 12:6370. doi: 10.1038/s41467-021-26449-8
7. Kozak M, Hu J. The integrated consideration of vaccine platforms, adjuvants, and delivery routes for successful vaccine development. Vaccines (Basel). (2023) 11:1–16. doi: 10.3390/vaccines11030695
8. Meneveau MO, Petroni G, Salerno EP, Lynch KT, Smolkin MMH, Woodson E, et al. Immunogenicity in humans of a transdermal multipeptide melanoma vaccine administered with or without a TLR7 agonist. J Immunother Cancer. (2021) 9:1–11. doi: 10.1136/jitc-2020-002214
9. Patel SP, Petroni G, Roszik J, Olson W, Wages N, Chianese-Bullock K, et al. Phase I/II trial of a long peptide vaccine (LPV7) plus toll-like receptor (TLR) agonists with or without incomplete Freund's adjuvant (IFA) for resected high-risk melanoma. J Immunother Cancer. (2021) 9:1–12. doi: 10.1136/jitc-2021-003220
10. Lv M, Chen M, Zhang R, Zhang W, Wang C, Zhang Y, et al. Manganese is critical for antitumor immune responses via cGAS-STING and improves the efficacy of clinical immunotherapy. Cell Res. (2020) 30:966–79. doi: 10.1038/s41422-020-00395-4
11. Wilson DR, Sen R, Sunshine J, Pardoll D, Green J, Kim Y, et al. Biodegradable STING agonist nanoparticles for enhanced cancer immunotherapy. Nanomedicine: Nanotechnology Biol Med. (2018) 14:237–46. doi: 10.1016/j.nano.2017.10.013
12. Prendergast GC, Smith CM, Thomas S, Mandik-Nayak L, Laury-Kleintop L, Metz R, et al. Indoleamine 2,3-dioxygenase pathways of pathogenic inflammation and immune escape in cancer. Cancer Immunol Immunother. (2014) 63:721–35. doi: 10.1007/s00262-014-1549-4
13. Gibney GT, Kudchadkar R, Deconti R, Thebeau M, Czupryn MP, Tetteh CL, et al. Safety, correlative markers, and clinical results of adjuvant nivolumab in combination with vaccine in resected high-risk metastatic melanoma. Clin Cancer Res. (2015) 21:712–20. doi: 10.1158/1078-0432.CCR-14-2468
14. Kaur A, Baldwin J, Brar DS, Salunke D, Petrovsky N. Toll-like receptor (TLR) agonists as a driving force behind next-generation vaccine adjuvants and cancer therapeutics. Curr Opin Chem Biol. (2022) 70:102172. doi: 10.1016/j.cbpa.2022.102172
15. Gnjatic S, Sawhney NB, Bhardwaj N. Toll-like receptor agonists: are they good adjuvants? Cancer J. (2010) 16:382–91. doi: 10.1097/PPO.0b013e3181eaca65
16. Tabarsi P, Anjidani N, Shahpari R, Mardani M, Sabzvari A, Yazdani B, et al. Safety and immunogenicity of SpikoGen®, an Advax-CpG55.2-adjuvanted SARS-CoV-2 spike protein vaccine: a phase 2 randomized placebo-controlled trial in both seropositive and seronegative populations. Clin Microbiol Infection. (2022) 28:1263–71. doi: 10.1016/j.cmi.2022.04.004
17. Ella R, Reddy S, Jogdand H, Sarangi V, Ganneru B, Prasad S, et al. Safety and immunogenicity of an inactivated SARS-CoV-2 vaccine, BBV152: interim results from a double-blind, randomised, multicentre, phase 2 trial, and 3-month follow-up of a double-blind, randomised phase 1 trial. Lancet Infect Dis. (2021) 21:950–61. doi: 10.1016/S1473-3099(21)00070-0
18. Zhang E, Ma Z, Lu M. Contribution of T- and B-cell intrinsic toll-like receptors to the adaptive immune response in viral infectious diseases. Cell Mol Life Sci. (2022) 79:1–15. doi: 10.1007/s00018-022-04582-x
19. Ahuja AS, Reddy VP, Marques O. Artificial intelligence and COVID-19: A multidisciplinary approach. Integr Med Res. (2020) 9:1–3. doi: 10.1016/j.imr.2020.100434
20. Thomas S, Abraham A, Baldwin J, Piplani S, Petrovsky N. Artificial intelligence in vaccine and drug design. Methods Mol Biol. (2021) 2410:131–46. doi: 10.1007/978-1-0716-1884-4_6
21. Sakala IG, Honda-Okubo Y, Li L, Baldwin J, Petrovsky N. A M2 protein-based universal influenza vaccine containing Advax-SM adjuvant provides newborn protection via maternal or neonatal immunization. Vaccine. (2021) 39:5162–72. doi: 10.1016/j.vaccine.2021.07.037
22. Jelinkova L, Jhun H, Eaton A, Petrovsky N, Zavala F, Chackerian B, et al. An epitope-based malaria vaccine targeting the junctional region of circumsporozoite protein. NPJ Vaccines. (2021) 6:1–10. doi: 10.1038/s41541-020-00274-4
23. Görander S, Honda-Okubo Y, Bäckström M, Baldwin J, Bergström T, Petrovsky N, et al. A truncated glycoprotein G vaccine formulated with Advax-CpG adjuvant provides protection of mice against genital herpes simplex virus 2 infection. Vaccine. (2021) 39:5866–75. doi: 10.1016/j.vaccine.2021.08.050
24. Honda-Okubo Y, Baldwin J, Petrovsky N. Advax-cpG adjuvant provides antigen dose-sparing and enhanced immunogenicity for inactivated poliomyelitis virus vaccines. Pathogens. (2021) 10:1–14. doi: 10.3390/pathogens10050500
25. Li L, Honda-Okubo Y, Huang Y, Jang H, Carlock M, Baldwin J, et al. Immunisation of ferrets and mice with recombinant SARS-CoV-2 spike protein formulated with Advax-SM adjuvant protects against COVID-19 infection. Vaccine. (2021) 39:5940–53. doi: 10.1016/j.vaccine.2021.07.087
26. Li L, Honda-Okubo Y, Baldwin J, Bowen R, Bielefeldt-Ohmann H, Petrovsky N, et al. Covax-19/Spikogen® vaccine based on recombinant spike protein extracellular domain with Advax-CpG55.2 adjuvant provides single dose protection against SARS-CoV-2 infection in hamsters. Vaccine. (2022) 40:3182–92. doi: 10.1016/j.vaccine.2022.04.041
27. Lin Y-J, Zimmermann JKB, Schülke S. Novel adjuvants in allergen-specific immunotherapy: where do we stand? Front Immunol. (2024) 15. doi: 10.3389/fimmu.2024.1348305
28. Pulendran B, Arunachalam PS, O’hagan DT. Emerging concepts in the science of vaccine adjuvants. Nat Rev Drug Discovery. (2021) 20:454–75. doi: 10.1038/s41573-021-00163-y
29. Sun Q, Melino G, Amelio I, Jiang J, Wang Y, Shi Y, et al. Recent advances in cancer immunotherapy. Discover Oncol. (2021) 12:1–9. doi: 10.1007/s12672-021-00422-9
30. Alaluf E, Shalamov MM, Sonnenblick A. Update on current and new potential immunotherapies in breast cancer, from bench to bedside. Front Immunol. (2024) 15. doi: 10.3389/fimmu.2024.1287824
31. Becher B, Tugues S, Greter M. GM-CSF: from growth factor to central mediator of tissue inflammation. Immunity. (2016) 45:963–73. doi: 10.1016/j.immuni.2016.10.026
32. Kantoff PW, Higano C, Shore N, Berger E, Small E, Penson D, et al. Sipuleucel-T immunotherapy for castration-resistant prostate cancer. New Engl J Med. (2010) 363:411–22. doi: 10.1056/NEJMoa1001294
33. Polyzoidis S, Ashkan K. DCVax®-L—Developed by northwest biotherapeutics. Hum Vaccines Immunotherapeutics. (2014) 10:3139–45. doi: 10.4161/hv.29276
34. Charych D, Hoch U, Langowski J, Lee SR, Addepalli M, Kirk P, et al. NKTR-214, an engineered cytokine with biased IL2 receptor binding, increased tumor exposure, and marked efficacy in mouse tumor models. Clin Cancer Res. (2016) 22:680–90. doi: 10.1158/1078-0432.CCR-15-1631
35. Pettenati C, Ingersoll MA. Mechanisms of BCG immunotherapy and its outlook for bladder cancer. Nat Rev Urol. (2018) 15:615–25. doi: 10.1038/s41585-018-0055-4
36. Sfakianos JP, Salomé B, Daza J, Farkas Á, Bhardwaj N, Horowitz A, et al. Bacillus Calmette-Guerin (BCG): Its fight against pathogens and cancer. Urologic Oncol. (2020) 39(2):121–9. doi: 10.1016/j.urolonc.2020.09.031
37. Zhang Z, Kuo JC, Yao S, Zhang C, Khan H, Lee RJ, et al. CpG oligodeoxynucleotides for anticancer monotherapy from preclinical stages to clinical trials. Pharmaceutics. (2021) 14:1–15. doi: 10.3390/pharmaceutics14010073
38. Bayart C, Mularoni A, Hemmani N, Kerachni S, Jose J, Gouet P, et al. Tetanus toxin fragment C: structure, drug discovery research and production. Pharmaceuticals. (2022) 15:1–28. doi: 10.3390/ph15060756
39. Sun B, Xia T. Nanomaterial-based vaccine adjuvants. J materials Chem B. (2016) 4 33:5496–509. doi: 10.1039/C6TB01131D
40. Prygiel M, Mosiej E, Górska P, Zasada A. Diphtheria-tetanus-pertussis vaccine: past, current & future. Future Microbiol. (2021) 17(3):185–97. doi: 10.2217/fmb-2021-0167
41. Pattyn J, Hendrickx G, Vorsters A, van Damme P. Hepatitis B vaccines. J Infect Dis. (2021) 224:S343–S351. doi: 10.1093/infdis/jiaa668
42. Williamson A-L. Recent developments in human papillomavirus (HPV) vaccinology. Viruses. (2023) 15:1–13. doi: 10.3390/v15071440
43. Roy V, Jung W, Linde C, Coates EE, Ledgerwood J, Costner PJM, et al. Differences in HPV-specific antibody Fc-effector functions following Gardasil® and Cervarix® vaccination. NPJ Vaccines. (2023) 8:1–11. doi: 10.1038/s41541-023-00628-8
44. Lövgren Bengtsson K, Morein B, Osterhaus ADME. ISCOM technology-based Matrix M™ adjuvant: success in future vaccines relies on formulation. Expert Rev Vaccines. (2011) 10:401–3. doi: 10.1586/erv.11.25
45. Liu Y, Zhao X, Gan F, Chen X, Deng K, Crowe SA, et al. Complete biosynthesis of QS-21 in engineered yeast. Nature. (2024) 629:937–44. doi: 10.1038/s41586-024-07345-9
46. Lü J, Wang X, Marin-Muller C, Wang H, Lin P, Yao Q, et al. Current advances in research and clinical applications of PLGA-based nanotechnology. Expert Rev Mol Diagnostics. (2009) 9:325–41. doi: 10.1586/erm.09.15
47. Sledge G, Loehrer P, Roth B, Einhorn L. Cisplatin as first-line therapy for metastatic breast cancer. J Clin Oncol. (1988) 6:1811–4. doi: 10.1200/JCO.1988.6.12.1811
48. Shao L, Shen S, Liu H. Recent advances in PLGA micro/nanoparticle delivery systems as novel therapeutic approach for drug-resistant tuberculosis. Front Bioengineering Biotechnol. (2022) 10. doi: 10.3389/fbioe.2022.941077
49. Liu Q, Chen X, Jia J, Zhang W, Yang T, Wang L, et al. pH-responsive poly(D,L-lactic-co-glycolic acid) nanoparticles with rapid antigen release behavior promote immune response. ACS nano. (2015) 9:4925–38. doi: 10.1021/nn5066793
50. Xia Y, Wu J, Wei W, Du Y, Wan T, Ma X, et al. Exploiting the pliability and lateral mobility of Pickering emulsion for enhanced vaccination. Nat materials. (2018) 17:187–94. doi: 10.1038/nmat5057
51. Shah SM, Dhawan V, Holm R, Nagarsenker M, Perrie Y, et al. Liposomes: Advancements and innovation in the manufacturing process. Advanced Drug delivery Rev. (2020) 154-155:102–22. doi: 10.1016/j.addr.2020.07.002
52. Meo S, Bukhari I, Akram J, Meo A, Klonoff D. COVID-19 vaccines: comparison of biological, pharmacological characteristics and adverse effects of Pfizer/BioNTech and Moderna Vaccines. Eur Rev Med Pharmacol Sci. (2021) 25:1663–9. doi: 10.26355/eurrev_202102_24877
53. Weinberger B. Adjuvant strategies to improve vaccination of the elderly population. Curr Opin Pharmacol. (2018) 41:34–41. doi: 10.1016/j.coph.2018.03.014
54. Goforth R, Salem A, Zhu X, Miles S, Zhang XQ, Lee JH, et al. Immune stimulatory antigen loaded particles combined with depletion of regulatory T-cells induce potent tumor specific immunity in a mouse model of melanoma. Cancer Immunology Immunotherapy. (2009) 58:517–30. doi: 10.1007/s00262-008-0574-6
55. Almeida JPM, Figueroa ER, Drezek RA. Gold nanoparticle mediated cancer immunotherapy. Nanomedicine: nanotechnology biology Med. (2014) 10:503–14. doi: 10.1016/j.nano.2013.09.011
56. Langermans JAM, Doherty T, Vervenne R, Laan TVD, Lyashchenko K, Greenwald R, et al. Protection of macaques against Mycobacterium tuberculosis infection by a subunit vaccine based on a fusion protein of antigen 85B and ESAT-6. Vaccine. (2005) 23:2740–50. doi: 10.1016/j.vaccine.2004.11.051
57. Moon D, Tae N, Park Y, Lee SW, Kim DH. Development of bispecific antibody for cancer immunotherapy: focus on T cell engaging antibody. Immune Network. (2022) 22:1–22. doi: 10.4110/in.2022.22.e4
58. Pagliuca S, Gurnari C, Rubio M, Visconte V, Lenz T. Individual HLA heterogeneity and its implications for cellular immune evasion in cancer and beyond. Front Immunol. (2022) 13. doi: 10.3389/fimmu.2022.944872
59. Macgillivray DM, Kollmann TR. The role of environmental factors in modulating immune responses in early life. Front Immunol. (2014) 5. doi: 10.3389/fimmu.2014.00434
60. Cisneros B, García-Aguirre I, Unzueta J, Arrieta-Cruz J, Gonzalez-Morales O, Domínguez-Larrieta JM, et al. Immune system modulation in aging: Molecular mechanisms and therapeutic targets. Front Immunol. (2022) 13. doi: 10.3389/fimmu.2022.1059173
61. Klein SL, Flanagan KL. Sex differences in immune responses. Nat Rev Immunol. (2016) 16:626–38. doi: 10.1038/nri.2016.90
62. Jordakieva G, Nowak D. Editorial: Occupational immunology: current knowledge and future perspectives. Front Med. (2023) 10. doi: 10.3389/fmed.2023.1322157
63. Zhu H. Big data and artificial intelligence modeling for drug discovery. Annu Rev Pharmacol Toxicol. (2020) 60:573–89. doi: 10.1146/annurev-pharmtox-010919-023324
64. Ciallella HL, Zhu H. Advancing computational toxicology in the big data era by artificial intelligence: data-driven and mechanism-driven modeling for chemical toxicity. Chem Res Toxicol. (2019) 32:536–47. doi: 10.1021/acs.chemrestox.8b00393
65. Frolkis A, Knox C, Lim E, Jewison T, Law V, Hau D, et al. SMPDB: the small molecule pathway database. Nucleic Acids Res. (2009) 38:D480–7. doi: 10.1093/nar/gkp1002
66. Shtar G, Azulay L, Nizri O, Rokach L, Shapira B. CDCDB: A large and continuously updated drug combination database. Sci Data. (2022) 9:1–11. doi: 10.1038/s41597-022-01360-z
67. Doms A, Schroeder M. GoPubMed: exploring pubMed with the gene ontology. Nucleic Acids Res. (2005) 33:W783–6. doi: 10.1093/nar/gki470
68. Corney DPA, Buxton B, Langdon W, Jones DT. BioRAT: extracting biological information from full-length papers. Bioinformatics. (2004) 20:3206–13. doi: 10.1093/bioinformatics/bth386
69. Chan HCS, Shan H, Dahoun T, Vogel H, Yuan S. Advancing drug discovery via artificial intelligence. Trends Pharmacol Sci. (2019) 40:592–604. doi: 10.1016/j.tips.2019.06.004
70. Yang Y, Adelstein SJ, Kassis AI. Target discovery from data mining approaches. Drug Discovery Today. (2009) 14:147–54. doi: 10.1016/j.drudis.2008.12.005
71. Feinberg EN, Sur D, Wu Z, Husic B, Mai H, Li Y, et al. PotentialNet for molecular property prediction. ACS Cent Sci. (2018) 4:1520–30. doi: 10.1021/acscentsci.8b00507
72. Brown N. In Silico Medicinal Chemistry: Computational Methods to Support Drug Design. Cambridge, UK: The Royal Society of Chemistry (2015).
73. Kumar R, Chaudhary K, Gupta S, Singh H, Kumar S, Gautam A, et al. CancerDR: cancer drug resistance database. Sci Rep. (2013) 3:1445. doi: 10.1038/srep01445
74. Kim S, Thiessen P, Bolton EE, Chen J, Fu G, Gindulyte A, et al. PubChem substance and compound databases. Nucleic Acids Res. (2015) 44:D1202–D1213. doi: 10.1093/nar/gkv951
75. Schomburg I, Chang A, Schomburg D. BRENDA, enzyme data and metabolic information. Nucleic Acids Res. (2002) 30:47–9. doi: 10.1093/nar/30.1.47
76. Chang A, Jeske L, Ulbrich S, Hofmann J, Koblitz J, Schomburg I, et al. BRENDA, the ELIXIR core data resource in 2021: new developments and updates. Nucleic Acids Res. (2020) 49:D498–508. doi: 10.1093/nar/gkaa1025
77. Wishart DS, Feunang YD, Guo A, Lo EJ, Marcu A, Grant J, et al. DrugBank 5.0: a major update to the DrugBank database for 2018. Nucleic Acids Res. (2017) 46:D1074–82. doi: 10.1093/nar/gkx1037
78. Mendez D, Gaulton A, Bento AP, Chambers J, Veij M, Felix E, et al. ChEMBL: towards direct deposition of bioassay data. Nucleic Acids Res. (2019) 47:D930–40. doi: 10.1093/nar/gky1075
79. Hastings J, Matos P, Dekker A, Ennis M, Harsha B, Kale N, et al. The ChEBI reference database and ontology for biologically relevant chemistry: enhancements for 2013. Nucleic Acids Res. (2012) 41:D456–63. doi: 10.1093/nar/gks1146
80. Irwin JJ, Tang KG, Young J, Dandarchuluun C, Wong BR, Khurelbaatar M, et al. ZINC20—A free ultralarge-scale chemical database for ligand discovery. J Chem Inf Modeling. (2020) 60:6065–73. doi: 10.1021/acs.jcim.0c00675
81. Steiner S, Wolf JB, Glatzel S, Andreou A, Granda J, Keenan G, et al. Organic synthesis in a modular robotic system driven by a chemical programming language. Science. (2019) 363:eaav2211. doi: 10.1126/science.aav2211
82. Gómez-Bombarelli R, Duvenaud D, Hernández-Lobato JM, Aguilera-Iparraguirre J, Hirzel TD, Adams RP, et al. Automatic chemical design using a data-driven continuous representation of molecules. ACS Cent Sci. (2018) 4:268–76. doi: 10.1021/acscentsci.7b00572
83. Tevosyan A, Khondkaryan L, Khachatrian H, Tadevosyan G, Apresyan L, Babayan N, et al. Improving VAE based molecular representations for compound property prediction. J Cheminformatics. (2022) 14:69. doi: 10.1186/s13321-022-00648-x
84. Li T, Zhao XM, Li L. (2022). Co-VAE: drug-target binding affinity prediction by co-regularized variational autoencoders, in: IEEE Transactions on Pattern Analysis and Machine Intelligence, (New York, USA: IEEE), Vol. 44. pp. 8861–73.
85. Wang Y-L, Wang F, Shi XX, Jia CY, Wu FX, Hao GF, et al. Cloud 3D-QSAR: a web tool for the development of quantitative structure–activity relationship models in drug discovery. Briefings Bioinf. (2021) 22:bbaa276. doi: 10.1093/bib/bbaa276
86. Duvenaud D, Maclaurin D, Aguilera-Iparraguirre J, Gómez-Bombarelli R, Hirzel T, Aspuru-Guzik A, et al. Convolutional networks on graphs for learning molecular fingerprints. Adv Neural Inf Process Syst. (2015) 28:2224–32. doi: 10.48550/arXiv.1509.09292
87. Gilson MK, Liu T, Baitaluk M, Nicola G, Hwang L, Chong J, et al. BindingDB in 2015: a public database for medicinal chemistry, computational chemistry and systems pharmacology. Nucleic Acids Res. (2016) 44:D1045–53. doi: 10.1093/nar/gkv1072
88. Zhou Y, Zhang Y, Lian X, Li F, Wang C, Zhu F, et al. Therapeutic target database update 2022: facilitating drug discovery with enriched comparative data of targeted agents. Nucleic Acids Res. (2021) 50:D1398–407. doi: 10.1093/nar/gkab953
89. Groom CR, Bruno IJ, Lightfoot MP, Ward SC. The Cambridge structural database. Acta Crystallographica Section B Struct Science Crystal Eng Materials. (2016) 72:171–9. doi: 10.1107/S2052520616003954
90. Allen FH. The Cambridge Structural Database: a quarter of a million crystal structures and rising. Acta crystallographica Section B Struct Sci. (2002) 58 Pt 3 Pt 1:380–8. doi: 10.1107/s0108768102003890
91. Nicklaus M. Downloadable structure files of nci open database compounds. Bethesda, Maryland, USA: national cancer institute (2016).
92. Veber DF, Johnson SR, Cheng HY, Smith B, Ward K, Kopple K, et al. Molecular properties that influence the oral bioavailability of drug candidates. J medicinal Chem. (2002) 45:2615–23. doi: 10.1021/jm020017n
93. Fu J, Wu L, Hu G, Shi Q, Wang R, Zhu L, et al. AMTDB: A comprehensive database of autophagic modulators for anti-tumor drug discovery. Front Pharmacol. (2022) 13. doi: 10.3389/fphar.2022.956501
94. Pence HE, Williams AJ. ChemSpider:: an online chemical information resource. J Chem Educ. (2010) 87:1123–4. doi: 10.1021/ed100697w
95. Danziger D, Dean PM. Automated site-directed drug design: a general algorithm for knowledge acquisition about hydrogen-bonding regions at protein surfaces. Proc R Soc London B Biol Sci. (1989) 236:101–13. doi: 10.1098/rspb.1989.0015
96. Lewis RA, Dean PM. Automated site-directed drug design: the concept of spacer skeletons for primary structure generation. Proc R Soc London B Biol Sci. (1989) 236:125–40. doi: 10.1098/rspb.1989.0017
97. Lewis RA, Dean PM. Automated site-directed drug design: the formation of molecular templates in primary structure generation. Proc R Soc London B Biol Sci. (1989) 236:141–62. doi: 10.1098/rspb.1989.0018
98. Hartenfeller M, Schneider G. Enabling future drug discovery by de novo design. Wiley Interdiscip Reviews: Comput Mol Sci. (2011) 1:742–59. doi: 10.1002/wcms.49
99. Popova M, Isayev O, Tropsha A. Deep reinforcement learning for de novo drug design. Sci Adv. (2017) 4:1–14. doi: 10.1126/sciadv.aap7885
100. Genheden S, Thakkar A, Chadimová V, Reymond J, Engkvist O, Bjerrum E, et al. AiZynthFinder: a fast, robust and flexible open-source software for retrosynthetic planning. J Cheminformatics. (2020) 12:1–9. doi: 10.1186/s13321-020-00472-1
101. Zhavoronkov A, Ivanenkov YA, Aliper A, Veselov MS, Aladinskiy VA, Aladinskaya AV, et al. Deep learning enables rapid identification of potent DDR1 kinase inhibitors. Nat Biotechnol. (2019) 37:1038–40. doi: 10.1038/s41587-019-0224-x
102. Goris A, Vandebergh M, McCauley J, Saarela J, Cotsapas C. Genetics of multiple sclerosis: lessons from polygenicity. Lancet Neurol. (2022) 21:830–42. doi: 10.1016/S1474-4422(22)00255-1
103. Sastry GM, Adzhigirey M, Day T, Annabhimoju R, Sherman W. Protein and ligand preparation: parameters, protocols, and influence on virtual screening enrichments. J Computer-Aided Mol Design. (2013) 27:221–34. doi: 10.1007/s10822-013-9644-8
104. Jin Z, Du X, Xu Y, Deng Y, Liu M, Zhao Y, et al. Structure-based drug design, virtual screening and high-throughput screening rapidly identify antiviral leads targeting COVID-19. bioRxiv. (2020) 582:289–93. doi: 10.1038/s41586-020-2223-y
105. Shen C, Zhang X, Deng Y, Gao J, Wang D, Xu L, et al. Boosting protein-ligand binding pose prediction and virtual screening based on residue-atom distance likelihood potential and graph transformer. J medicinal Chem. (2022) 65(15):10691–706. doi: 10.1021/acs.jmedchem.2c00991
106. Giordano D, Biancaniello C, Argenio MA, Facchiano A. Drug design by pharmacophore and virtual screening approach. Pharmaceuticals. (2022) 15:1–16. doi: 10.3390/ph15050646
107. Zhang J-H, Chung TDY, Oldenburg KR. A simple statistical parameter for use in evaluation and validation of high throughput screening assays. J Biomolecular Screening. (1999) 4:67–73. doi: 10.1177/108705719900400206
108. Kontoyianni M. Docking and virtual screening in drug discovery. Methods Mol Biol. (2017) 1647:255–66. doi: 10.1007/978-1-4939-7201-2_18
109. Park JW, Hong S-W. (2019). Ligand- and structure-based virtual screening studies for the discovery of selective inhibitors, in: 2019 IEEE International Conference on Bioinformatics and Biomedicine (BIBM), (New York, USA: IEEE). pp. 1235–6. doi: 10.1109/BIBM47256.2019.8983013
110. Bologa CG, Ursu O, Oprea TI. How to prepare a compound collection prior to virtual screening. Methods Mol Biol. (2019) 1939:119–38. doi: 10.1007/978-1-4939-9089-4_7
111. Banegas-Luna AJ, Cerón-Carrasco JP, Pérez-Sánchez H. A review of ligand-based virtual screening web tools and screening algorithms in large molecular databases in the age of big data. Future medicinal Chem. (2018) 10:2641–58. doi: 10.4155/fmc-2018-0076
112. Badrinarayan P, Sastry GN. Virtual high throughput screening in new lead identification. Combinatorial Chem High throughput screening. (2011) 14:840–60. doi: 10.2174/138620711797537102
113. De Luca L, Vittorio S, Peña-Díaz S, Pitasi G, Fornt-Suñé M, Bucolo F, et al. Ligand-based discovery of a small molecule as inhibitor of α-synuclein amyloid formation. Int J Mol Sci. (2022) 23:1–14. doi: 10.3390/ijms232314844
114. Zarei O, Faham N, Vankayalapati H, Raeppel S, Welm A, Hamzeh-Mivehroud M, et al. Ligand-based discovery of novel small molecule inhibitors of RON receptor tyrosine kinase. Mol Inf. (2020) 41:1–9. doi: 10.1002/minf.202000181
115. Haider S, Barakat A, Ul-Haq Z. Discovery of potential chemical probe as inhibitors of CXCL12 using ligand-based virtual screening and molecular dynamic simulation. Molecules. (2020) 25:1–13. doi: 10.3390/molecules25204829
116. Brunger AT. X-PLOR Version 3.1: A System for X-ray Crystallography and NMR. (1992) (New Haven, Connecticut, USA: Yale University Press).
117. Wüthrich K. Nuclear magnetic resonance (NMR) spectroscopy. Int Tables Crystallogr. (2012), 464–79. doi: 10.1107/97809553602060000704
118. Zhu H, Zhang Y, Li W, Huang N. A comprehensive survey of prospective structure-based virtual screening for early drug discovery in the past fifteen years. Int J Mol Sci. (2022) 23:1–18. doi: 10.3390/ijms232415961
119. Ferreira LLG, Santos RND, Oliva G, Andricopulo A. Molecular docking and structure-based drug design strategies. Molecules. (2015) 20:13384–421. doi: 10.3390/molecules200713384
120. Mukherjee S, Abdalla M, Yadav M, Madhavi M, Bhrdwaj A, Khandelwal R, et al. Structure-Based Virtual Screening, Molecular Docking, and Molecular Dynamics Simulation of VEGF inhibitors for the clinical treatment of Ovarian Cancer. J Mol Modeling. (2022) 28:1–21. doi: 10.1007/s00894-022-05081-3
121. Xie Z-S, Zhou Z, Sun L, Yi H, Xue ST, Li ZR, et al. Structure-based virtual screening towards the discovery of novel FOXM1 inhibitors. Future medicinal Chem. (2021). doi: 10.4155/fmc-2021-0282
122. Bellver-Sanchis A, Choudhary BS, Companys-Alemany J, Ávila-López SP, Rodríguez ALM, et al. Structure‐Based Virtual Screening and in vitro and in vivo Analyses Revealed Potent Methyltransferase G9a Inhibitors as Prospective Anti‐Alzheimer's Agents. Chemmedchem. (2022) 17:1–10. doi: 10.1002/cmdc.202200002
123. Guo J, Xiang S, Wang J, Zhou Y, Wang Z, Zhang Z, et al. Discovery of novel TrkA allosteric inhibitors: Structure-based virtual screening, biological evaluation and preliminary SAR studies. Eur J Medicinal Chem. (2021) 228:114022. doi: 10.1016/j.ejmech.2021.114022
124. Lin TE, Sung L, Chao MW, Li M, Zheng JH, Sung TY, et al. Structure-based virtual screening and biological evaluation of novel small-molecule BTK inhibitors. J Enzyme Inhibition Medicinal Chem. (2021) 37:226–35. doi: 10.1080/14756366.2021.1999237
125. Luo L, Zhong A, Wang Q, Zheng T. Structure-based pharmacophore modeling, virtual screening, molecular docking, ADMET, and molecular dynamics (MD) simulation of potential inhibitors of PD-L1 from the library of marine natural products. Mar Drugs. (2021) 20:1–18. doi: 10.3390/md20010029
126. Ge H-j, Mao L, Zhao J, Wang Y, Shi D, Yang X, et al. Discovery of novel IDO1 inhibitors via structure-based virtual screening and biological assays. J Computer-Aided Mol Design. (2021) 35:679–94. doi: 10.1007/s10822-021-00386-6
127. Wang T, Wu MB, Lin JP, Yang L. Quantitative structure–activity relationship: promising advances in drug discovery platforms. Expert Opin Drug Discovery. (2015) 10:1283–300. doi: 10.1517/17460441.2015.1083006
128. Hansch C, Fujita T. p-σ-π Analysis. A method for the correlation of biological activity and chemical structure. J Am Chem Soc. (1964) 86:1616–26. doi: 10.1021/ja01062a035
129. Hasegawa K, Arakawa M, Funatsu K. Rational choice of bioactive conformations through use of conformation analysis and 3-way partial least squares modeling. Chemometrics Intelligent Lab Syst. (2000) 50:253–61. doi: 10.1016/S0169-7439(99)00063-5
130. Podlogar BL, Ferguson DM. QSAR and CoMFA: a perspective on the practical application to drug discovery. Drug design Discovery. (2000) 17:4–12.
131. Wang Y-L, Wang F, Shi XX, Jia CY, Wu FX, Hao GF, et al. Cloud 3D-QSAR: a web tool for the development of quantitative structure-activity relationship models in drug discovery. Briefings Bioinf. (2020) 22(4):1–8. doi: 10.1093/bib/bbaa276
132. Damale MG, Harke S, Khan FAK, Shinde D, Sangshetti J. Recent advances in multidimensional QSAR (4D-6D): a critical review. Mini Rev medicinal Chem. (2014) 14:35–55. doi: 10.2174/13895575113136660104
133. Duvenaud DK, Maclaurin D, Aguilera-Iparraguirre J, Gómez-Bombarelli R, Hirzel TD, Aspuru-Guzik A, et al. Convolutional Networks on Graphs for Learning Molecular Fingerprints. Vancouver, Canada: NIPS (2015).
134. Coley CW, Barzilay R, Green W, Jaakkola T, Jensen K. Convolutional embedding of attributed molecular graphs for physical property prediction. J Chem Inf modeling. (2017) 57:1757–72. doi: 10.1021/acs.jcim.6b00601
135. Urban G, Subrahmanya NA, Baldi P. Inner and outer recursive neural networks for chemoinformatics applications. J Chem Inf modeling. (2018) 58:207–11. doi: 10.1021/acs.jcim.7b00384
136. Scialli AR, Daston G, Chen CL, Coder P, Euling S, Foreman JE, et al. Rethinking developmental toxicity testing: Evolution or revolution? Birth defects Res. (2018) 110:840–50. doi: 10.1002/bdr2.1212
137. Russell WMS, Burch RL. The principles of humane experimental technique. Med J Aust. (1960) 1–238.
138. Curzer HJ, Perry G, Wallace M, Perry D. The three rs of animal research: what they mean for the institutional animal care and use committee and why. Sci Eng Ethics. (2016) 22:549–65. doi: 10.1007/s11948-015-9659-8
139. Mayr A, Klambauer G, Unterthiner T, Hochreiter S. DeepTox: toxicity prediction using deep learning. Front Environ Sci. (2016) 3. doi: 10.3389/fenvs.2015.00080
140. Pu L, Naderi M, Liu T, Wu HC, Mukhopadhyay S, Brylinski M, et al. eToxPred: a machine learning-based approach to estimate the toxicity of drug candidates. BMC Pharmacol Toxicol. (2019) 20(1):1–15. doi: 10.1186/s40360-018-0282-6
141. Lysenko A, Sharma A, Boroevich KA, Tsunoda T. An integrative machine learning approach for prediction of toxicity-related drug safety. Life Sci Alliance. (2018) 1(6). doi: 10.1101/455667
142. Pushpakom SP, Iorio F, Eyers P, Escott KJ, Hopper S, Wells A, et al. Drug repurposing: progress, challenges and recommendations. Nat Rev Drug Discovery. (2018) 18:41–58. doi: 10.1038/nrd.2018.168
143. Martorana A, Perricone U, Lauria A. The repurposing of old drugs or unsuccessful lead compounds by in silico approaches: new advances and perspectives. Curr topics medicinal Chem. (2016) 16:2088–106. doi: 10.2174/1568026616666160216153457
144. Rumienczyk I, Kulecka M, Statkiewicz M, Ostrowski J, Mikuła M, et al. Oncology drug repurposing for sepsis treatment. Biomedicines. (2022) 10:921. doi: 10.3390/biomedicines10040921
145. Monneret C, Bohuon C. Fabuleux hasards: histoire de la découverte de médicaments. Les Ulis, France: L'Editeur: EDP Sciences (2009).
146. Rothwell PM, Fowkes GR, Belch J, Ogawa H, Warlow C, Meade T, et al. Effect of daily aspirin on long-term risk of death due to cancer: analysis of individual patient data from randomised trials. Lancet. (2011) 377:31–41. doi: 10.1016/S0140-6736(10)62110-1
147. Rüschoff J, Wallinger S, Dietmaier W, Bocker T, Brockhoff G, Hofstädter F, et al. Aspirin suppresses the mutator phenotype associated with hereditary nonpolyposis colorectal cancer by genetic selection. Proc Natl Acad Sci. (1998) 95:11301–6. doi: 10.1073/pnas.95.19.11301
148. Cavalla D, Singal C. Retrospective clinical analysis for drug rescue: for new indications or stratified patient groups. Drug Discovery Today. (2012) 17:104–9. doi: 10.1016/j.drudis.2011.09.019
149. Raje N, Anderson K. Thalidomide—a revival story. Mass Med Soc. (1999) 341(21):1606–9. doi: 10.1056/NEJM199911183412110
150. Liu R, Wei L, Zhang P. A deep learning framework for drug repurposing via emulating clinical trials on real-world patient data. Nat Mach Intell. (2021) 3:68–75. doi: 10.1038/s42256-020-00276-w
151. Huang R, Southall N, Wang Y, Yasgar A, Shinn P, Jadhav A, et al. The NCGC pharmaceutical collection: A comprehensive resource of clinically approved drugs enabling repurposing and chemical genomics. Sci Trans Med. (2011) 3:80ps16–80ps16. doi: 10.1126/scitranslmed.3001862
152. Finak G, Mayer BT, Fulp W, Obrecht P, Sato A, Chung E, et al. DataPackageR: Reproducible data preprocessing, standardization and sharing using R/Bioconductor for collaborative data analysis. Gates Open Res. (2018) 2:1–17. doi: 10.12688/gatesopenres
153. Uechi L, Galas DJ, Sakhanenko NA. Multivariate analysis of data sets with missing values: an information theory-based reliability function. J Comput Biol. (2019) 26:152–71. doi: 10.1089/cmb.2018.0179
154. AtChadé MN, Sokadjo YM, Moussa AD, Kurisheva S, Bochenina M, et al. Cross-validation comparison of COVID-19 forecast models. Sn Comput Sci. (2021) 2:1–9. doi: 10.1007/s42979-021-00699-1
155. Westerlund AM, Barge B, Mervin L, Genheden S. Data‐driven approaches for identifying hyperparameters in multi‐step retrosynthesis. Mol Inf. (2023) 42:1–9. doi: 10.1002/minf.202300128
156. Li L, Wang H, Zha L, Huang Q, Wu S, Chen G, et al. (2023). Learning a data-driven policy network for pre-training automated feature engineering, in: International Conference on Learning Representations.
157. Vamathevan J, Clark D, Czodrowski P, Dunham I, Ferran E, Lee G, et al. Applications of machine learning in drug discovery and development. Nat Rev Drug Discovery. (2019) 18:463–77. doi: 10.1038/s41573-019-0024-5
158. Brubaker SW, Bonham K, Zanoni I, Kagan JC. Innate immune pattern recognition: a cell biological perspective. Annu Rev Immunol. (2015) 33:257–90. doi: 10.1146/annurev-immunol-032414-112240
159. Duan T, Du Y, Xing C, Wang HY, Wang RF, et al. Toll-like receptor signaling and its role in cell-mediated immunity. Front Immunol. (2022) 13:812774. doi: 10.3389/fimmu.2022.812774
160. Veneziani I, Alicata C, Moretta L, Maggi E. The latest approach of immunotherapy with endosomal TLR agonists improving NK cell function: an overview. Biomedicines. (2022) 11:1–17. doi: 10.3390/biomedicines11010064
161. Chin AI, Miyahira AK, Covarrubias AJ, Teague J, Guo B, Dempsey P, et al. Toll-like receptor 3-mediated suppression of TRAMP prostate cancer shows the critical role of type I interferons in tumor immune surveillance. Cancer Res. (2010) 70:2595–603. doi: 10.1158/0008-5472.CAN-09-1162
162. Du X, Poltorak A, Wei Y, Beutler B. Three novel mammalian toll-like receptors: gene structure, expression, and evolution. Eur Cytokine Netw. (2000) 11:362–71.
163. Vasilakos JP, Tomai MA. The use of Toll-like receptor 7/8 agonists as vaccine adjuvants. Expert Rev Vaccines. (2013) 12:809–19. doi: 10.1586/14760584.2013.811208
164. Cervantes JL, Weinerman B, Basole CP, Salaza J. TLR8: the forgotten relative revindicated. Cell Mol Immunol. (2012) 9:434–8. doi: 10.1038/cmi.2012.38
165. Sun H, Li Y, Zhang P, Xing H, Zhao S, Song Y, et al. Targeting toll-like receptor 7/8 for immunotherapy: recent advances and prospectives. biomark Res. (2022) 10:1–19. doi: 10.1186/s40364-022-00436-7
166. Lynn GM, Chytil P, Francica J, Lagová A, Kueberuwa G, Ishizuka A, et al. Impact of polymer-TLR-7/8 agonist (Adjuvant) morphology on the potency and mechanism of CD8 T cell induction. Biomacromolecules. (2019) 20:854–70. doi: 10.1021/acs.biomac.8b01473
167. Lynn GM, Laga R, Darrah P, Ishizuka A, Balaci AJ, Dulcey AE, et al. In vivo characterization of the physicochemical properties of TLR agonist delivery that enhance vaccine immunogenicity. Nat Biotechnol. (2015) 33:1201–10. doi: 10.1038/nbt.3371
168. Švajger U, Horvat Ž, Knez D, Rožman P, Turk S, Gobec S, et al. New antagonists of toll-like receptor 7 discovered through 3D ligand-based virtual screening. Medicinal Chem Res. (2014) 24:362–71. doi: 10.1007/s00044-014-1127-5
169. Duan J, Sastry G, Dixon SL, Lowrie J, Sherman W, et al. Analysis and comparison of 2D fingerprints: insights into database screening performance using eight fingerprint methods. J Cheminformatics. (2010) 3:P1–1. doi: 10.1186/1758-2946-3-S1-P1
170. Auderset F, Belnoue E, Mastelic-Gavillet B, Lambert P, Siegrist C, et al. A TLR7/8 agonist-including DOEPC-based cationic liposome formulation mediates its adjuvanticity through the sustained recruitment of highly activated monocytes in a type I IFN-independent but NF-kappaB-dependent manner. Front Immunol. (2020) 11:580974. doi: 10.3389/fimmu.2020.580974
171. Liong CS, Smith AAA, Mann JL, Roth GA, Gale EC, Maikawa CL, et al. Enhanced humoral immune response by high density TLR agonist presentation on hyperbranched polymers. Advanced Ther. (2021) 4:1–7. doi: 10.1002/adtp.202000081
172. Kim H, Niu L, Larson PG, Kucaba T, Murphy K, James BR, et al. Polymeric nanoparticles encapsulating novel TLR7/8 agonists as immunostimulatory adjuvants for enhanced cancer immunotherapy. Biomaterials. (2018) 164:38–53. doi: 10.1016/j.biomaterials.2018.02.034
173. Messina JP, Gilkeson GS, Pisetsky DS. Stimulation of in vitro murine lymphocyte proliferation by bacterial DNA. J Immunol. (1991) 147:1759–64. doi: 10.4049/jimmunol.147.6.1759
174. Sparwasser T, Koch E, Vabulas R, Heeg K, Lipford G, Ellwart J, et al. Bacterial DNA and immunostimulatory CpG oligonucleotides trigger maturation and activation of murine dendritic cells. Eur J Immunol. (1998) 28:2045–54. doi: 10.1002/(ISSN)1521-4141
175. Krieg AM, Yi A, Matson S, Waldschmidt T, Bishop G, Teasdale R, et al. CpG motifs in bacterial DNA trigger direct B-cell activation. Nature. (1995) 374:546–9. doi: 10.1038/374546a0
176. Krieg AM. CpG motifs in bacterial DNA and their immune effects. Annu Rev Immunol. (2002) 20:709–60. doi: 10.1146/annurev.immunol.20.100301.064842
177. Ashkar AA, Rosenthal KL. Toll-like receptor 9, CpG DNA and innate immunity. Curr Mol Med. (2002) 2:545–56. doi: 10.2174/1566524023362159
178. Rothenfusser S, Hornung V, Ayyoub M, Britsch S, Towarowski A, Krug A, et al. CpG-A and CpG-B oligonucleotides differentially enhance human peptide-specific primary and memory CD8+ T-cell responses in vitro. Blood. (2004) 103:2162–9. doi: 10.1182/blood-2003-04-1091
179. Bode C, Poth J, Fox M, Schulz S, Klinman D, Latz E, et al. Cytosolic d‐type CpG‐oligonucleotides induce a type I interferon response by activating the cGAS‐STING signaling pathway. Eur J Immunol. (2021) 51:1686–97. doi: 10.1002/eji.202048810
180. Campbell JD. Development of the cpG adjuvant 1018: A case study. Methods Mol Biol. (2017) 1494:15–27. doi: 10.1007/978-1-4939-6445-1_2
181. Lipford GB, Sparwasser T, Zimmermann S, Heeg K, Wagner H. CpG-DNA-mediated transient lymphadenopathy is associated with a state of Th1 predisposition to antigen-driven responses. J Immunol. (2000) 165:1228–35. doi: 10.4049/jimmunol.165.3.1228
182. Ito S, Hirobe S, Kawakita T, Saito M, Quan Y, Kamiyama F, et al. Characteristic of K3 (CpG-ODN) as a transcutaneous vaccine formulation adjuvant. Pharmaceutics. (2020) 12:1–17. doi: 10.3390/pharmaceutics12030267
183. Woo SR, Fuertes M, Corrales L, Spranger S, Furdyna MJ, Leung M, et al. STING-dependent cytosolic DNA sensing mediates innate immune recognition of immunogenic tumors. Immunity. (2014) 41:830–42. doi: 10.1016/j.immuni.2014.10.017
184. Temizoz B, Kuroda E, Ishii KJ. Vaccine adjuvants as potential cancer immunotherapeutics. Int Immunol. (2016) 28:329–38. doi: 10.1093/intimm/dxw015
185. Li T, Chen ZJ. The cGAS-cGAMP-STING pathway connects DNA damage to inflammation, senescence, and cancer. J Exp Med. (2018) 215:1287–99. doi: 10.1084/jem.20180139
186. Sun L, Wu J, Du F, Chen X, Chen ZJ. Cyclic GMP-AMP synthase is a cytosolic DNA sensor that activates the type I interferon pathway. Science. (2013) 339:786–91. doi: 10.1126/science.1232458
187. Ishikawa H, Barber GN. STING is an endoplasmic reticulum adaptor that facilitates innate immune signalling. Nature. (2008) 455:674–8. doi: 10.1038/nature07317
188. Chen W, Kuolee R, Yan H. The potential of 3',5'-cyclic diguanylic acid (c-di-GMP) as an effective vaccine adjuvant. Vaccine. (2010) 28:3080–5. doi: 10.1016/j.vaccine.2010.02.081
189. Fu J, Kanne D, Leong M, Glickman L, McWhirter S, Lemmens E, et al. STING agonist formulated cancer vaccines can cure established tumors resistant to PD-1 blockade. Sci Transl Med. (2015) 7:283ra52. doi: 10.1126/scitranslmed.aaa4306
190. Karaolis DK, Means T, Yang D, Takahashi M, Yoshimura T, Muraille E, et al. Bacterial c-di-GMP is an immunostimulatory molecule. J Immunol. (2007) 178:2171–81. doi: 10.4049/jimmunol.178.4.2171
191. Ogunniyi AD, Paton J, Kirby A, McCullers J, Cook J, Hyodo M, et al. c-di-GMP is an effective immunomodulator and vaccine adjuvant against pneumococcal infection. Vaccine. (2008) 26:4676–85. doi: 10.1016/j.vaccine.2008.06.099
192. Yan H, Chen W. The promise and challenges of cyclic dinucleotides as molecular adjuvants for vaccine development. Vaccines (Basel). (2021) 9:1–31. doi: 10.3390/vaccines9080917
193. Li WH, Su JY, Li YM. Rational design of T-cell- and B-cell-based therapeutic cancer vaccines. Acc Chem Res. (2022) 55:2660–71. doi: 10.1021/acs.accounts.2c00360
194. Bhatnagar S, Revuri V, Shah MD, Larson PG, Ferguson D, Panyam J, et al. Combination of STING and TLR 7/8 agonists as vaccine adjuvants for cancer immunotherapy. Cancers (Basel). (2022) 14:1–18. doi: 10.3390/cancers14246091
195. Harrington KJ, Brody J, Ingham M, Strauss J, Cemerski S, Wang M, et al. Preliminary results of the first-in-human (FIH) study of MK-1454, an agonist of stimulator of interferon genes (STING), as monotherapy or in combination with pembrolizumab (pembro) in patients with advanced solid tumors or lymphomas. Ann Oncol. (2018) 29 Suppl 8:viii712. doi: 10.1093/annonc/mdy424.015
196. van der Zanden SY, Luimstra JJ, Neefjes J, Borst J, Ovaa H. Opportunities for small molecules in cancer immunotherapy. Trends Immunol. (2020) 41(6):493–511. doi: 10.1016/j.it.2020.04.004
197. Meric-Bernstam F, Sweis R, Hodi F, Messersmith W, Andtbacka R, Ingham M, et al. Phase I dose-escalation trial of MIW815 (ADU-S100), an intratumoral STING agonist, in patients with advanced/metastatic solid tumors or lymphomas. Clin Cancer Res. (2021) 677–88. doi: 10.1158/1078-0432.CCR-21-1963
198. Falahat R, Berglund A, Perez-Villarroel P, Putney RM, Hamaidi I, Kim S, et al. Epigenetic state determines the in vivo efficacy of STING agonist therapy. Nat Commun. (2023) 14:1–16. doi: 10.1038/s41467-023-37217-1
199. Leach DG, Dharmaraj N, Piotrowski S, Lopez-Silva T, Lei Y, Sikora A, et al. STINGel: Controlled release of a cyclic dinucleotide for enhanced cancer immunotherapy. Biomaterials. (2018) 163:67–75. doi: 10.1016/j.biomaterials.2018.01.035
200. Ramanjulu JM, Pesiridis GS, Yang J, Concha N, Singhaus RR, Zhang S, et al. Design of amidobenzimidazole STING receptor agonists with systemic activity. Nature. (2018) 564:439–43. doi: 10.1038/s41586-018-0705-y
201. Li Y, Wilson HL, Kiss-Toth E. Regulating STING in health and disease. J Inflammation (London England). (2017) 14:1–21. doi: 10.1186/s12950-017-0159-2
202. Ishida Y, Agata Y, Shibahara K, Honjo T. Induced expression of PD‐1, a novel member of the immunoglobulin gene superfamily, upon programmed cell death. EMBO J. (1992) 11:3887–95. doi: 10.1002/embj.1992.11.issue-11
203. Wu Y, Chen W, Xu Z, Gu W. PD-L1 distribution and perspective for cancer immunotherapy—Blockade, knockdown, or inhibition. Front Immunol. (2019) 10. doi: 10.3389/fimmu.2019.02022
204. Cha J-H, Chan LC, Li CW, Hsu J, Hung M. Mechanisms controlling PD-L1 expression in cancer. Mol Cell. (2019) 76(3):359–70. doi: 10.1016/j.molcel.2019.09.030
205. Mocellin S, Nitti D. Therapeutics targeting tumor immune escape: Towards the development of new generation anticancer vaccines. Medicinal Res Rev. (2008) 28:413–44. doi: 10.1002/med.20110
206. Morse MA, Gwin WR, Mitchell DA. Vaccine therapies for cancer: then and now. Targeted Oncol. (2021) 16:121–52. doi: 10.1007/s11523-020-00788-w
207. Igarashi Y, Sasada T. Cancer vaccines: toward the next breakthrough in cancer immunotherapy. J Immunol Res. (2020) 2020:1–13. doi: 10.1155/2020/5825401
208. Kuol N, Stojanovska L, Nurgali K, Apostolopoulos V. PD-1/PD-L1 in disease. Immunotherapy. (2018) 10:149–60. doi: 10.2217/imt-2017-0120
209. Ott PA, Hu-Lieskovan S, Chmielowski B, Govindan R, Naing A, Bhardwaj N, et al. A phase Ib trial of personalized neoantigen therapy plus anti-PD-1 in patients with advanced melanoma, non-small cell lung cancer, or bladder cancer. Cell. (2020) 183:347–62.e24. doi: 10.1016/j.cell.2020.08.053
210. Wu Y, Yang Z, Cheng K, Bi H, Chen J. Small molecule-based immunomodulators for cancer therapy. Acta Pharm Sinica. B. (2022) 12:4287–308. doi: 10.1016/j.apsb.2022.11.007
211. Wilson CG, Arkin MR. Probing structural adaptivity at PPI interfaces with small molecules. Drug Discovery Today Technol. (2013) 10:e501–8. doi: 10.1016/j.ddtec.2012.10.009
212. Zak KM, Grudnik P, Guzik K, Zięba B, Musielak B, Dömling A, et al. Structural basis for small molecule targeting of the programmed death ligand 1 (PD-L1). Oncotarget. (2016) 7:30323–35. doi: 10.18632/oncotarget.v7i21
213. Zak KM, Grudnik P, Guzik K, Zięba B, Musielak B, Dömling A, et al. Structural biology of the immune checkpoint receptor PD-1 and its ligands PD-L1/PD-L2. Structure. (2017) 25:1163–74. doi: 10.1016/j.str.2017.06.011
214. Bailly C, Vergoten G. Flurbiprofen as a biphenyl scaffold for the design of small molecules binding to PD-L1 protein dimer. Biochem Pharmacol. (2020) 178:114042. doi: 10.1016/j.bcp.2020.114042
215. Sasikumar PGN, Sudarshan N, Adurthi S, Ramachandra R, Samiulla D, Lakshminarasimhan A, et al. PD-1 derived CA-170 is an oral immune checkpoint inhibitor that exhibits preclinical anti-tumor efficacy. Commun Biol. (2021) 4:1–12. doi: 10.1038/s42003-021-02191-1
216. Soliman H, Mediavilla-Varela M, Antonia S. Indoleamine 2,3-dioxygenase: is it an immune suppressor? Cancer J. (2010) 16:354–9. doi: 10.1097/PPO.0b013e3181eb3343
217. Li Y, Zhang K, Wu Y, Yue Y, Cheng K, Feng Q, et al. Antigen capture and immune modulation by bacterial outer membrane vesicles as in situ vaccine for cancer immunotherapy post-photothermal therapy. Small. (2022) 18(14):e2107461. doi: 10.1002/smll.202107461
218. Su Q, Wang C, Song H, Zhang C, Liu J, Huang P, et al. Co-delivery of anionic epitope/CpG vaccine and IDO inhibitor by self-assembled cationic liposomes for combination melanoma immunotherapy. J materials Chem B. (2021) 9:3892–99. doi: 10.1039/D1TB00256B
219. Troitskaya O, Varlamov M, Nushtaeva A, Richter V, Koval O. Recombinant lactaptin induces immunogenic cell death and creates an antitumor vaccination effect in vivo with enhancement by an IDO inhibitor. Molecules. (2020) 25:1–17. doi: 10.3390/molecules25122804
220. Pagni RL, Souza PC, Pegoraro R, Porchia BF, Silva JR, Aps LR, et al. Interleukin-6 and indoleamine-2,3-dioxygenase as potential adjuvant targets for Papillomavirus-related tumors immunotherapy. Front Immunol. (2022) 13. doi: 10.3389/fimmu.2022.1005937
221. Moreno ACR, Porchia BF, Pagni R, Souza PC, Pegoraro R, Rodrigues KB, et al. The combined use of melatonin and an indoleamine 2,3-dioxygenase-1 inhibitor enhances vaccine-induced protective cellular immunity to HPV16-associated tumors. Front Immunol. (2018) 9. doi: 10.3389/fimmu.2018.01914
222. Soliman HH, Khambati F, Han H, Ismail-Khan R, Bui M, Sullivan D, et al. A phase-1/2 study of adenovirus-p53 transduced dendritic cell vaccine in combination with indoximod in metastatic solid tumors and invasive breast cancer. Oncotarget. (2015) 9:10110–7. doi: 10.18632/oncotarget.v9i11
223. DiMasi JA, Grabowski HG, Hansen RW. Innovation in the pharmaceutical industry: New estimates of R&D costs. J Health economics. (2016) 47:20–33. doi: 10.1016/j.jhealeco.2016.01.012
224. Wong CH, Siah KW, Lo AW. Estimation of clinical trial success rates and related parameters. Biostatistics (Oxford England). (2018) 20:273–86. doi: 10.1093/biostatistics/kxx069
225. Hay M, Thomas DW, Craighead JC, Economides C, Rosenthal J, et al. Clinical development success rates for investigational drugs. Nat Biotechnol. (2014) 32:40–51. doi: 10.1038/nbt.2786
226. Harrer S, Shah P, Antony B, Hu J. Artificial intelligence for clinical trial design. Trends Pharmacol Sci. (2019) 40(8):577–91. doi: 10.1109/SPMB50085.2020.9353614
227. Heaton J. Ian Goodfellow, Yoshua Bengio, and Aaron Courville: deep learning. Genet Programming Evolvable Machines. (2018) 19:305–7. doi: 10.1007/s10710-017-9314-z
228. Deng K, Woodland PC. (2023). Adaptable end-to-end ASR models using replaceable internal LMs and residual softmax, in: ICASSP 2023, New York, USA: IEEE. doi: 10.1109/ICASSP49357.2023.10095860
229. Dara S, Dhamercherla S, Jadav SS, Babu CM, Ahsan M. Machine learning in drug discovery: A review. Artif Intell Rev. (2021) 55:1947–99. doi: 10.1007/s10462-021-10058-4
230. He K, Zhang X, Ren S, Sun J. (2015). Deep residual learning for image recognition, in: 2016 IEEE Conference on Computer Vision and Pattern Recognition (CVPR), New York, USA: IEEE. pp. 770–8.
231. Lipinski CF, Maltarollo V, Oliveira PR, Silva ABF, Honório KM. Advances and perspectives in applying deep learning for drug design and discovery. Front Robotics AI. (2019) 6. doi: 10.3389/frobt.2019.00108
232. Gastegger M, McSloy A, Luya M, Schütt KT, Maure R. A deep neural network for molecular wave functions in quasi-atomic minimal basis representation. J Chem Phys. (2020) 153:044123. doi: 10.1063/5.0012911
233. Bennett WFD, He S, Bilodeau CL, Jones D, Sun D, Kim H. Predicting small molecule transfer free energies by combining molecular dynamics simulations and deep learning. J Chem Inf modeling. (2020) 60(11):5375–81. doi: 10.1021/acs.jcim.0c00318
234. Lamberti MJ, Wilkinson M, Donzanti BA, Wohlhieter GE, Parikh S, Wilkins RG, et al. A study on the application and use of artificial intelligence to support drug development. Clin Ther. (2019) 41(8):1414–26. doi: 10.1016/j.clinthera.2019.05.018
Keywords: AI drug discovery, vaccine adjuvant, cancer, immune agonist, small molecular
Citation: Zhang W-Y, Zheng X-L, Coghi PS, Chen J-H, Dong B-J and Fan X-X (2024) Revolutionizing adjuvant development: harnessing AI for next-generation cancer vaccines. Front. Immunol. 15:1438030. doi: 10.3389/fimmu.2024.1438030
Received: 24 May 2024; Accepted: 23 July 2024;
Published: 14 August 2024.
Edited by:
Srinivasa Reddy Bonam, University of Texas Medical Branch at Galveston, United StatesReviewed by:
Claudio Rhyner, Christine-Kühne-Center for Allergy Research and Education (CK-Care), SwitzerlandAmrita Das, Indian Institute of Chemical Biology (CSIR), India
Copyright © 2024 Zhang, Zheng, Coghi, Chen, Dong and Fan. This is an open-access article distributed under the terms of the Creative Commons Attribution License (CC BY). The use, distribution or reproduction in other forums is permitted, provided the original author(s) and the copyright owner(s) are credited and that the original publication in this journal is cited, in accordance with accepted academic practice. No use, distribution or reproduction is permitted which does not comply with these terms.
*Correspondence: Bing-Jun Dong, enh5ZG9uZ2JpbmdqdW5Aemh1aGFpLmdvdi5jbg==; Xing-Xing Fan, eHhmYW5AbXVzdC5lZHUubW8=
†These authors have contributed equally to this work