- 1Department of Gastroenterology and Hepatology, Tianjin Medical University General Hospital, Tianjin Institute of Digestive Diseases, Tianjin Key Laboratory of Digestive Diseases, Tianjin, China
- 2The Second Hospital of Tianjin Medical University, Tianjin, China
- 3Department of Orthopedic, Tianjin Medical University General Hospital, Tianjin, China
Objective: This study sought to identify circulating proteins causally linked to Inflammatory Bowel Disease (IBD) traits through a Mendelian Randomization (MR) analytical framework.
Methods: Using a large-scale, two-sample MR approach, we estimated the genetic links of numerous plasma proteins with IBD and its subtypes, leveraging information from the Inflammatory Bowel Disease Genetics Consortium. To assess the robustness of MR findings, methods like Bayesian colocalization, and Steiger filtering analysis, evaluation of protein-altering variants. Further insights into IBD’s underlying mechanisms and therapeutic targets were gleaned from single-cell sequencing analyses, protein-protein interaction assessments, pathway enrichment analyses, and evaluation of drug targets.
Results: By cis-only MR analysis, we identified 83 protein-phenotype associations involving 27 different proteins associated with at least one IBD subtype. Among these proteins, DAG1, IL10, IL12B, IL23R, MST1, STAT3 and TNFRSF6B showed overlapping positive or negative associations in all IBD phenotypes. Extending to cis + trans MR analysis, we further identified 117 protein-feature associations, including 44 unique proteins, most of which were not detected in the cis-only analysis. In addition, by performing co-localization analysis and Steiger filtering analysis on the prioritized associations, we further confirmed the causal relationship between these proteins and the IBD phenotype and verified the exact causal direction from the protein to the IBD-related feature.
Conclusion: MR analysis facilitated the identification of numerous circulating proteins associated with IBD traits, unveiling protein-mediated mechanisms and promising therapeutic targets.
1 Introduction
Inflammatory Bowel Disease (IBD), comprising Crohn’s Disease (CD) and Ulcerative Colitis (UC), is marked by idiopathic, non-specific inflammatory intestinal disorders. IBD is characterized by a chronic, intractable course, necessitating extended periods of treatment and attentive care (1). The combination of endoscopy and histological analysis is universally acknowledged as the gold standard for diagnosing and assessing IBD. However, it is costly and invasive, potentially causing discomfort and pain for the patient (2). C-reactive protein (CRP) is a viable serum biomarker for monitoring IBD treatment. Although CRP is effective, it is not exclusively specific for IBD because any inflammation can lead to elevated CRP levels (3). Anti-inflammatory medicines and biologics are currently used to treat IBD. Anti-inflammatory medicines, such as 5-Aminosalicylates (5-ASA) and corticosteroids, are the initial step in IBD treatment (4), whereas biologics are mainly employed when there is no improvement after 5-ASA and steroid treatment (5). Nevertheless, some patients are insensitive to biologics. Hence, it is imperative to explore more efficient and non-invasive biomarkers for monitoring IBD treatment, alongside implementing more effective treatment approaches to reduce the physical and financial strain on individuals, families, and society.
Circulating plasma proteins, as key regulatory factors in most molecular pathways within the organism, hold immense potential in disease diagnosis and treatment. Earlier research indicates the potential involvement of circulating plasma proteins in the development and advancement of IBD, along with their potential therapeutic benefits. A case-control study involving 98 UC patients and 105 CD patients revealed the involvement of immune-mediated interleukin (IL)-22 in the development of IBD (6). A prospective study revealed that α-2 macroglobulin (LRG), interleukin (IL)-6, prealbumin (pre-Alb), high-sensitivity CRP (hs-CRP), CRP, and fecal calprotectin (FC) are associated with changes in UC disease activity, with LRG also reflecting disease activity during anti-tumor necrosis factor (TNF) antibody treatment (7). Genomics and proteomics are essential scientific research tools for studying the composition, structure, function, interactions, and regulatory mechanisms of genes and proteins within organisms. Genomic research provides foundational data for proteomics, while proteomics offers more comprehensive bioinformatics data for genomics. The amalgamation of genomic and proteomic data enables the examination of genetic differences linked to protein expression levels, also known as protein quantitative trait loci (pQTL). pQTL research can help understand the relationship between genotype and protein expression, revealing the regulatory mechanisms of genotype on protein expression, and providing a comprehensive and crucial information base for understanding the mechanisms of disease onset and progression, prevention, and precision treatment (8).
Mendelian Randomization (MR) functions as a statistical method in epidemiological studies to evaluate the causal association between exposure factors and outcomes, employing genetic variations highly correlated with the exposure factors as instrumental variables (9). By combining MR and pQTL, the causal relationship between specific genotypes and protein quantities can be evaluated, allowing for the more accurate identification of pQTL affecting protein quantities. For example, in a recent two-sample MR analysis utilizing pQTL data from seven large-scale proteomic studies, 13 protein biomarkers linked to a higher risk of colorectal cancer were identified (10). Another two-sample MR combined with colocalization analysis utilizing data on 4907 levels of circulating plasma proteins, identified links between these proteins and IBD and its subtypes. The amalgamation of drug databases data led to the deduction that circulating plasma proteins MST1, HGFAC, STAT3, ITPKA, and CXCL5 could be viable drug targets for IBD or UC (11). The objective of this study is to identify circulating plasma proteins associated with IBD and its subtypes (CD and UC) through MR analysis, delving into protein-mediated mechanisms and potential therapeutic targets.
This study (Figure 1) aims to explore the association between genetically predicted circulating proteins and IBD and its subtypes. Using the MR method, we constructed MR tools based on 10 proteomic GWAS datasets, focusing on evaluating cis- and trans-acting serum pQTLs related to circulating proteins. Through a comprehensive statistical analysis of the data provided by IIBDGC on IBD, which includes MR analysis, Steiger filtering, and colocalization analysis, we have effectively identified the causal relationship between circulating proteins and IBD. Additionally, for proteins identified through MR analysis, we further evaluated their potential for drug targeting in pharmaceutical development. This comprehensive approach provides new perspectives on the involvement of circulating proteins in the pathogenesis of IBD and highlights potential targets for therapy, establishing a solid foundation for future treatment strategies for IBD.
2 Method and material
2.1 Exposure
The exposure factors in this study are genetically predicted circulating proteins, for which we utilized nine different cohort pQTL (protein Quantitative Trait Loci) data provided by Zhang’s study (12). Additionally, we supplemented this with the latest pQTL data (13) based on their selection criteria, enriching the research value. The MR tools for circulating proteins were constructed from ten proteomics GWAS (Genome-Wide Association Studies) (13–22). The details of these ten studies are presented in Supplementary Table 1. The selection of candidate instrumental variables, serum pQTLs, was conducted as follows: initially, single nucleotide polymorphisms (SNPs) associated with any protein were chosen based on the recommended p-value thresholds in the respective studies (Supplementary Table 2). Following this, SNPs situated in the major histocompatibility complex (MHC) region (chr6: 26 Mb to 34 Mb), recognized for its intricate linkage disequilibrium (LD) formations, were omitted. The third step involved LD clumping, where a threshold of r2 > 0.01 and a distance of <5000kb upstream/downstream were applied to identify independent pQTLs for each protein. To further ensure the robustness of our MR analysis, we specifically excluded weak instrumental variables, i.e., those with F-statistic values less than or equal to 10. Ultimately, instruments linked to five or more proteins were omitted owing to their significant pleiotropy. In this study, tools were categorized into cis-pQTLs, situated within a 500kb segment of the protein-coding sequence, and trans-pQTLs, positioned beyond the 500kb segment of the protein-coding gene.
2.2 Outcomes
To delve into the genetic underpinnings of IBD and its subtypes, CD and UC, the study employed aggregated data from the International IBD Genetics Consortium (IIBDGC, https://www.ibdgenetics.org/). The International IBD Genetics Consortium dataset comprises 12,882 IBD cases, including 5,956 CD cases and 6,968 UC cases (23), all of European ancestry to maintain genetic homogeneity (Table 1). These cases were diagnosed according to strict clinical criteria, encompassing radiological, endoscopic, and histopathological assessments. Genetic association analyses were adjusted for age, sex, and up to 20 principal genetic components to control for potential confounding factors, ensuring the accuracy and reliability of the analysis results and providing in-depth insights into the genetic characteristics of IBD and its subtypes.
2.3 MR analysis
In the MR analysis, genetically predicted proteins were considered the exposure factor, with IBD and its subtypes (CD and UC) as the outcomes. MR analysis tools were constructed utilizing both cis-pQTLs and all pQTLs (cis + trans). The MR effect was assessed utilizing the Wald ratio estimator for a single pQTL, and the inverse variance weighted method for two or more available pQTLs. Given that our selection strategy might provide instrumental variables linked with up to four proteins, which could display pleiotropy, proteins-traits associations supported by MR evidence were scrutinized for their instruments if related with more than one protein. MR analyses were conducted, potential pleiotropic instruments were excluded, and results were presented. Additional sensitivity assessments were conducted to examine the solidity of the results and to tackle possible diversity and lateral pleiotropy across the instruments. In cases where Cochran’s Q test revealed variability across various instruments, the weighted median technique was utilized, permitting as much as 50% of the instruments to be invalid. Likewise, the MR-Egger technique was utilized in cases where horizontal pleiotropy was identified via the MR-Egger method, given its ability to adjust for instrument pleiotropy. The ‘TwoSampleMR’ R package (http://github.com/MRCIEU/TwoSampleMR) was utilized for MR analysis. For MR analysis, the threshold for the Bonferroni-adjusted p-value was established at 0.05, divided by the total proteins examined.
2.4 Steiger filtering analysis
The Steiger filtering technique, implemented via the ‘TwoSampleMR’ R package, was employed on MR associations passing the multiplicity-adjusted threshold to examine for potential distortions from reverse causation. For simpler understanding, results were categorized: ‘true’ when the exposure’s impact on the outcome was notable at p<0.05; ‘false’ when it was contrary at p<0.05; and ‘uncertain’ when p was 0.05 or more.
2.5 Colocalization analysis
Results from the surviving MR analysis were assessed through Bayesian colocalization analysis to calculate the likelihood of a single variant at each site impacting both protein and IBD-related characteristics, as opposed to coincidental variant sharing due to LD association. The ‘coloc’ R package (http://cran.r-project.org/web/packages/coloc) was utilized to assess colocalization. The ‘coloc’ R package offered posterior probabilities for five theories about the presence of a common variant in two traits, with the fourth hypothesis suggesting a link between both traits and the identical genetic variant in that area. Hypothesis 4’s posterior probability exceeding 0.8 was identified as robust proof of colocalization.
2.6 Single-cell type expression analysis
To understand the specificity of genetically predicted circulating proteins across different cell types, we opted to use the scIBD platform (http://scibd.cn/) (24), a cutting-edge tool specifically designed for single-cell meta-analysis in IBD. scIBD aggregates approximately 1.14 million single-cell data from various developmental stages (including fetal, child, and adult) and tissues from multiple anatomical regions, such as blood, small intestine, and colon, derived from 12 different datasets, reflecting different disease states including healthy, inflamed UC, and inflamed CD. Utilizing the scIBD platform, we were able to intricately analyze the transcriptomic features of 9 major cell subtypes (e.g., myeloid cells, CD4 T cells, CD8 T cells, etc.) and 101 cell subtypes. This was particularly important for our study as it allowed us to explore the expression and regulation of circulating proteins at the single-cell level, revealing their specificity across different cell types. Additionally, the interactive visualization tools provided by scIBD enabled us to more precisely analyze the expression patterns and gene regulatory networks of a given gene set in each cell subset. This in-depth single-cell analysis allowed us to more accurately identify key circulating proteins related to the pathogenesis of IBD, thereby revealing their specific roles in disease progression.
2.7 Protein-protein interaction and functional enrichment analysis
For exploring the connections between proteins ranked by MR, a protein-protein interaction (PPI) network was developed utilizing the Search Tool for the Retrieval of Interacting Genes (STRING, version 11.5, https://string-db.org/). Furthermore, the ‘ClusterProfiler’ R package (https://bioconductor.org/packages/release/bioc/html/clusterProfiler.html) was utilized for analyzing Gene Ontology (GO) and Kyoto Encyclopedia of Genes and Genomes (KEGG) pathways, aiming to investigate the possible biological roles and pathways linked to these proteins. For the enrichment analysis, the selection parameters were set thus: a p-value less than 0.05 signified notable enrichment outcomes. Gene ID conversion was performed using the ‘org.Hs.eg.db’ package, while the clusterProfiler package facilitated GO functional enrichment analysis, encompassing Biological Process (BP), Cellular Component (CC), and Molecular Function (MF), as well as KEGG pathway enrichment analysis to decipher the biological pathways engaged by DEGs (Differentially Expressed Genes) (25).
2.8 Identification of druggable proteins
To assess the druggability of identified proteins, this study queried the DGIdb database (https://old.dgidb.org/) and previously researched lists of druggable genes. Proteins identified in DGIdb, along with their associated drug names and development information, were documented. DGIdb aggregates information from various public databases and publications, including DrugBank, PharmGKB, ChEMBL, Drug Target Commons, among others, and updates it using specialized knowledge and text mining methods. Presently, the database houses in excess of 10,000 genes and 15,000 medications, encompassing over 50,000 interactions between drugs and genes, spanning 43 possible categories of drugs. DGIdb, as a tool for research, is crucial for investigating interactions between drugs and genes, as well as the genome that can be targeted for drug development, especially in clinical sequencing and tailored medical practices, serving as key sources for disease management and pharmaceutical advancement.
3 Results
3.1 Calculating the impact of plasma proteins on IBD through the application of cis-only MR
Considering that cis-pQTLs are assumed to have a higher a priori likelihood of specific biological effects compared to trans-pQTLs, cis-pQTLs were initially used as genetic instruments for MR analysis to methodically evaluate the proof of causal effects of causative effects of plasma proteins on IBD and its subtypes (Figure 2).
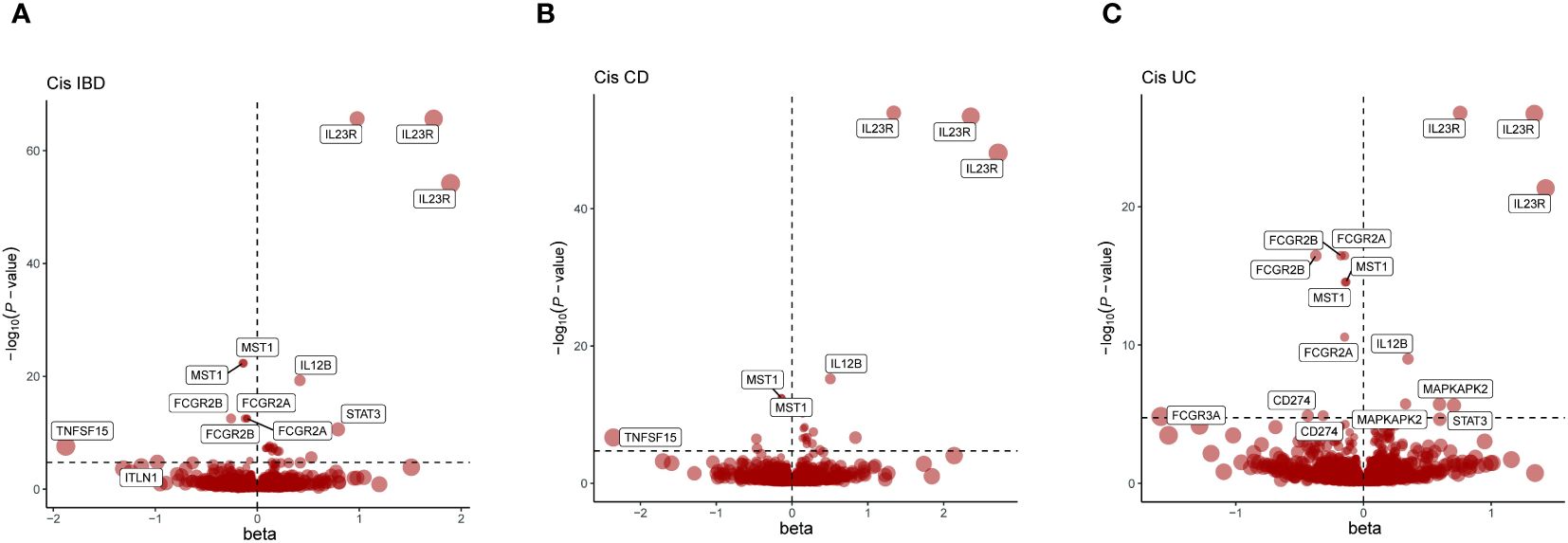
Figure 2 Presents a series of volcano plots from MR analyses, depicting the associations between genetically predicted levels of circulating proteins and IBD as well as its subtypes. Highlighted genes represent those that remained significant after Bonferroni correction in MR results (p<0.05/number of proteins tested). The x-axis beta values greater than zero indicate a positive influence of circulating proteins on the outcome, while values less than zero suggest a negative impact. (A) Volcano plot for IBD-related Cis-Only MR analysis; (B) Volcano plot for CD-related Cis-Only MR analysis; (C) Volcano plot for UC-related Cis-Only MR analysis.
In summary, using the Bonferroni adjustment threshold, with IBD p<0.05/4705, CD p<0.05/4704, UC p<0.05/4703 (Supplementary Table 2), 83 protein-phenotype association were identified, among which 27 distinct proteins were linked to at least one of the IBD subtypes (Figure 2, Table 2, Supplementary Table 4).
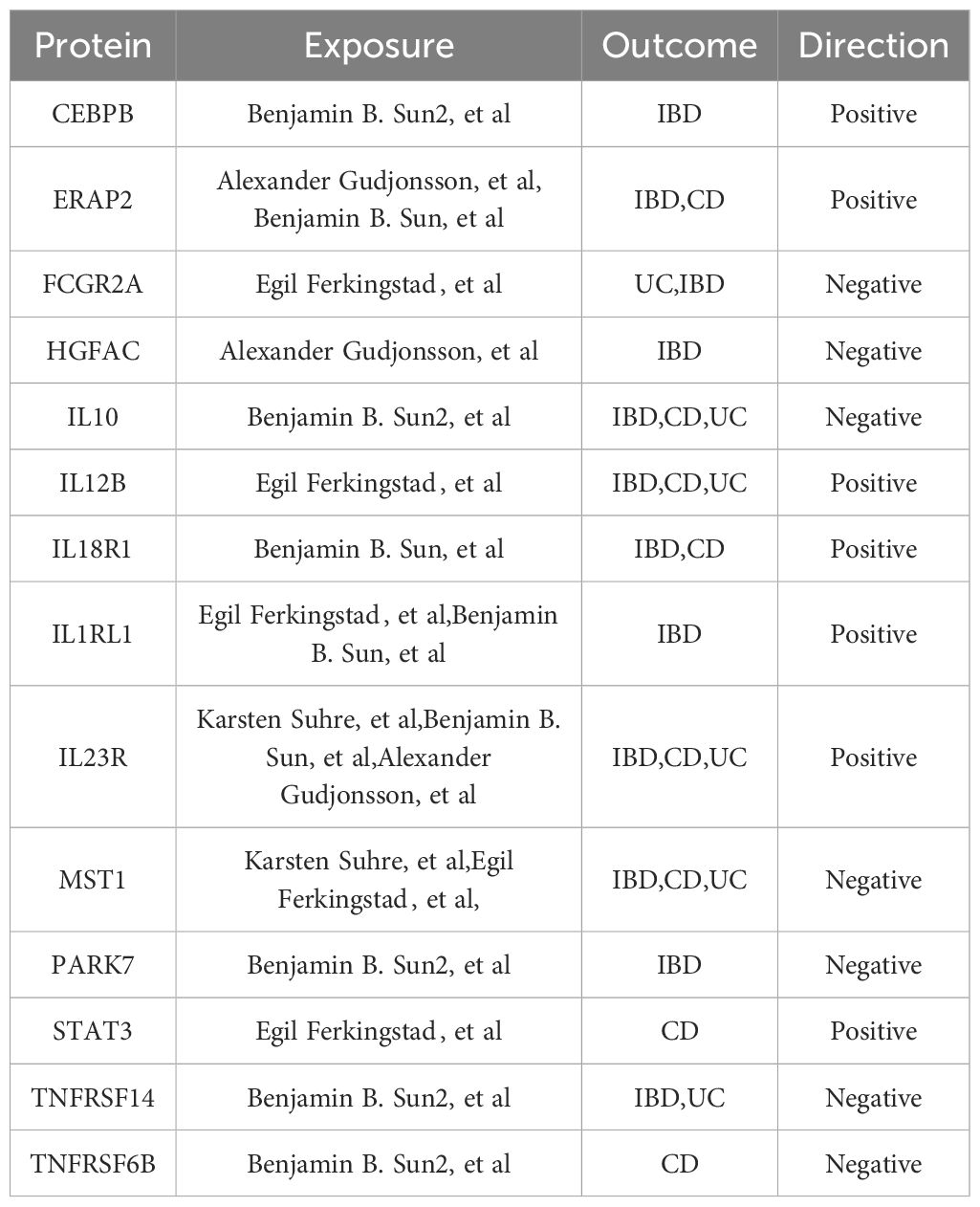
Table 2 Cis-pQTL-Based MR, Colocalization, and Steiger Filter Analysis: Final Protein Selections Associated with IBD and Its Subtypes.
Proteins like DAG1, IL10, IL12B, IL23R, MST1, STAT3, and TNFRSF6B showed overlapping positive associations across IBD, CD, and UC phenotypes (Supplementary Table 5), maintaining the same direction of association. For instance, the latest data from Benjamin B. Sun et al. indicate a negative correlation between genetically predicted IL10 and IBD, CD, and UC phenotypes (Figure 2, Table 2), suggesting a possible common pathogenesis mechanism among these phenotypes. Specifically, the beta coefficients of IL10 in IBD, CD and UC were -1.50822738, -1.258632705 and -1.670350144, respectively, suggesting that increased levels of IL10 are associated with a decreased risk of these disorders. DAG1 showed significant positive associations with IBD and its subtypes, with beta coefficients in IBD, CD and UC 2.72190224, 2.814379709, and 2.605902159, respectively. This suggests that an increase in DAG1 may be associated with an increased risk of IBD. The β coefficients of MST1 in IBD and its subtypes showed a negative correlation, which suggests that an increase in MST1 may be associated with a reduced risk of IBD. This provides clues to MST1 as a potential therapeutic target. Additionally, in the cis-pQTL MR sensitivity analysis, the newly identified ENTR1 protein was found to reduce the risk of IBD and CD incidence. The β coefficients of ENTR1 in CD and IBD were -0.519010561 and -0.331279952, respectively. This new finding emphasizes the potential role of ENTR1 in the pathogenesis of IBD (Supplementary Table 6).
3.2 Calculating the impact of plasma proteins on IBD through the application of cis + trans MR
Incorporating trans-pQTLs into the MR analysis potentially enhances the dependability of protein-phenotype associations. Consequently, our MR analysis was broadened to encompass all pQTLs (cis + trans) (Supplementary Table 3). In these analyses, a total of 117 protein-feature associations demonstrated MR evidence, including 44 distinct proteins, the majority of which remained undetected in the cis-pQTL analysis (Figure 3, Table 3, Supplementary Tables 7, 8). Within the newly identified proteins across all pQTLs, STOM, FASLG, ADH1B, IRF9, CYB5R1, TMPRSS11D, TENC1, PRDM1, and KLRF1 stood out as positive proteins that intersect in the IBD, CD, and UC phenotypes.
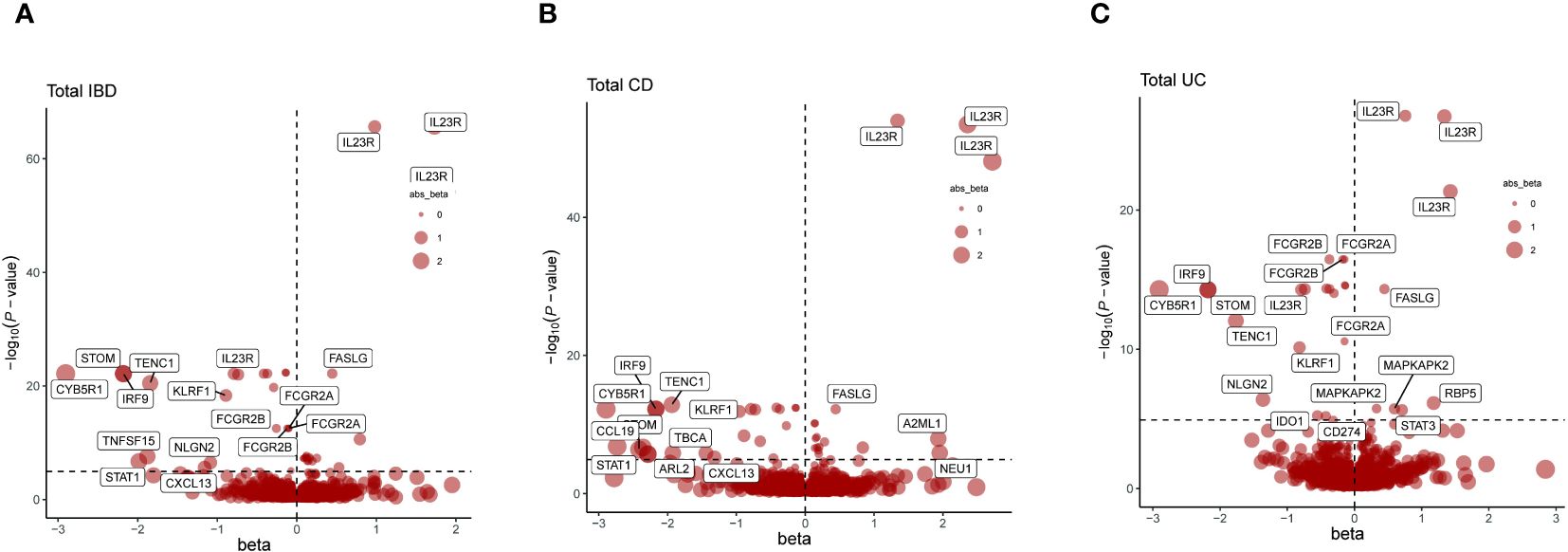
Figure 3 Presents a series of volcano plots from MR analyses, depicting the associations between genetically predicted levels of circulating proteins and IBD as well as its subtypes. Highlighted genes represent those that remained significant after Bonferroni correction in MR results (p<0.05/number of proteins tested). The x-axis beta values greater than zero indicate a positive influence of circulating proteins on the outcome, while values less than zero suggest a negative impact. (A) Volcano plot for the IBD-related cis + trans MR analysis. (B) Volcano plot for the CD-related cis + trans MR analysis. (C) Volcano plot for the UC-related cis + trans MR analysis.
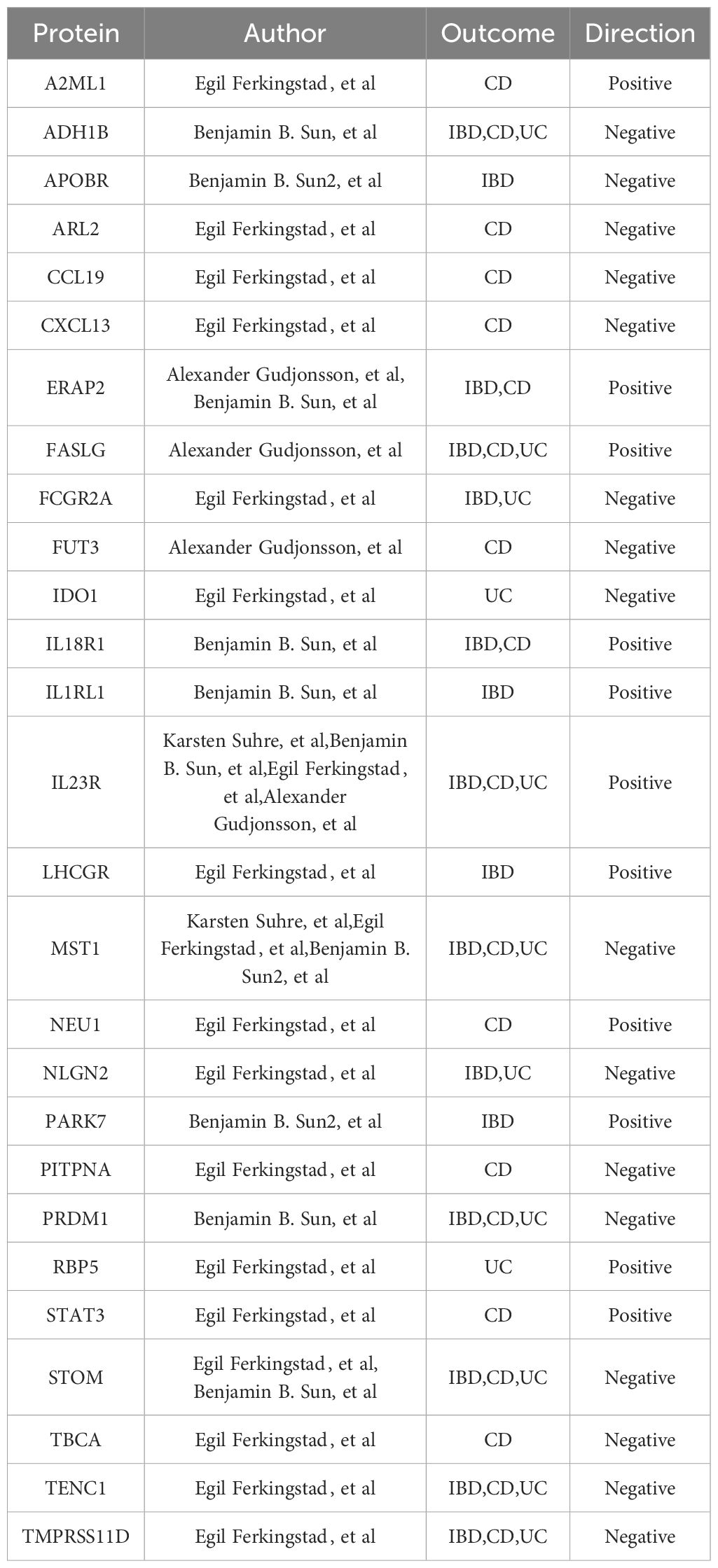
Table 3 cis + trans pQTL-Based MR, Colocalization, and Steiger Filter Analysis: Final Protein Selections Associated with IBD and Its Subtypes.
Sensitivity analyses confirmed the consistency of all significant associations detected and added 33 associations with MR evidence, encompassing 22 distinct proteins (Supplementary Tables 9, 10).
3.3 Co-localization of pQTLs with IBD risk loci
A colocalization analysis was performed to reduce the possible misleading effects of LD on MR- prioritized associations, aiming to determine the likelihood that genetic connections with proteins and phenotypes originated from identical causal variants. Colocalization was performed for proteins with singular instrumental variables and accessible summary-level GWAS data. Among the associations prioritized by cis-MR, 83 were subjected to colocalization analysis, and 40 showed significant signs of colocalization (Supplementary Table 11). Furthermore, among the associations identified by MR using all pQTLs, 78 out of 117 analyzed associations demonstrated substantial evidence of colocalization (Supplementary Table 12).
3.4 Testing causal direction through Steiger filtering analysis
Recognizing the potential of inverse causality in the anticipated associations between proteins and phenotypes, a directional examination, namely the Steiger filtering analysis, was performed to verify if the associations identified by MR truly evolved from proteins to IBD-related traits. The results indicated that the majority of associations identified by MR, including those identified via sensitivity analyses, mainly conformed to the accurate causal direction from proteins to IBD-related traits (Supplementary Tables 13-15).
3.5 Single-cell analysis
The scIBD encompasses 9 primary subtypes (myeloid, CD4 T cells, CD8 T cells, Innate Lymphoid Cells(ILC), B/plasma cells, epithelial cells, mesenchymal cells, endothelial cells, and neuronal cells) and 101 cell subtypes (Figure 4A). To understand the specificity of genetically predicted circulating proteins identified by MR analysis across different cell types and disease states, Gene Enrichment Analysis was employed, illustrating the variability among cell subtypes as shown in Figures 4B, D. It was observed that among the Cis-Only MR positive proteins, STAT3, CEBPB, PARK7, TNFRSF14, and ERAP2 were expressed at significantly higher levels compared to other proteins across all cell types. Other proteins demonstrated cell-specific expression, such as IL1RL1 and IL18R1 in endothelial cells, IL23R in Innate Lymphoid Cells (ILC), MST1 in epithelial cells, and IL10 and FCGR2A in myeloid cells (Figure 4B). Within the cis + trans MR positive proteins, IL1RL1 was highly expressed in endothelial cells, A2ML1 in mesenchymal cells, IDO1 in myeloid cells, and CXCL13 and CCL19 were co-expressed in mesenchymal cells (Figure 4D). Furthermore, the differential expression of these proteins across various health and disease states was investigated, revealing that PARK7 was highly expressed in both CD inflamed and CD non-inflamed conditions (Figures 4C, E).
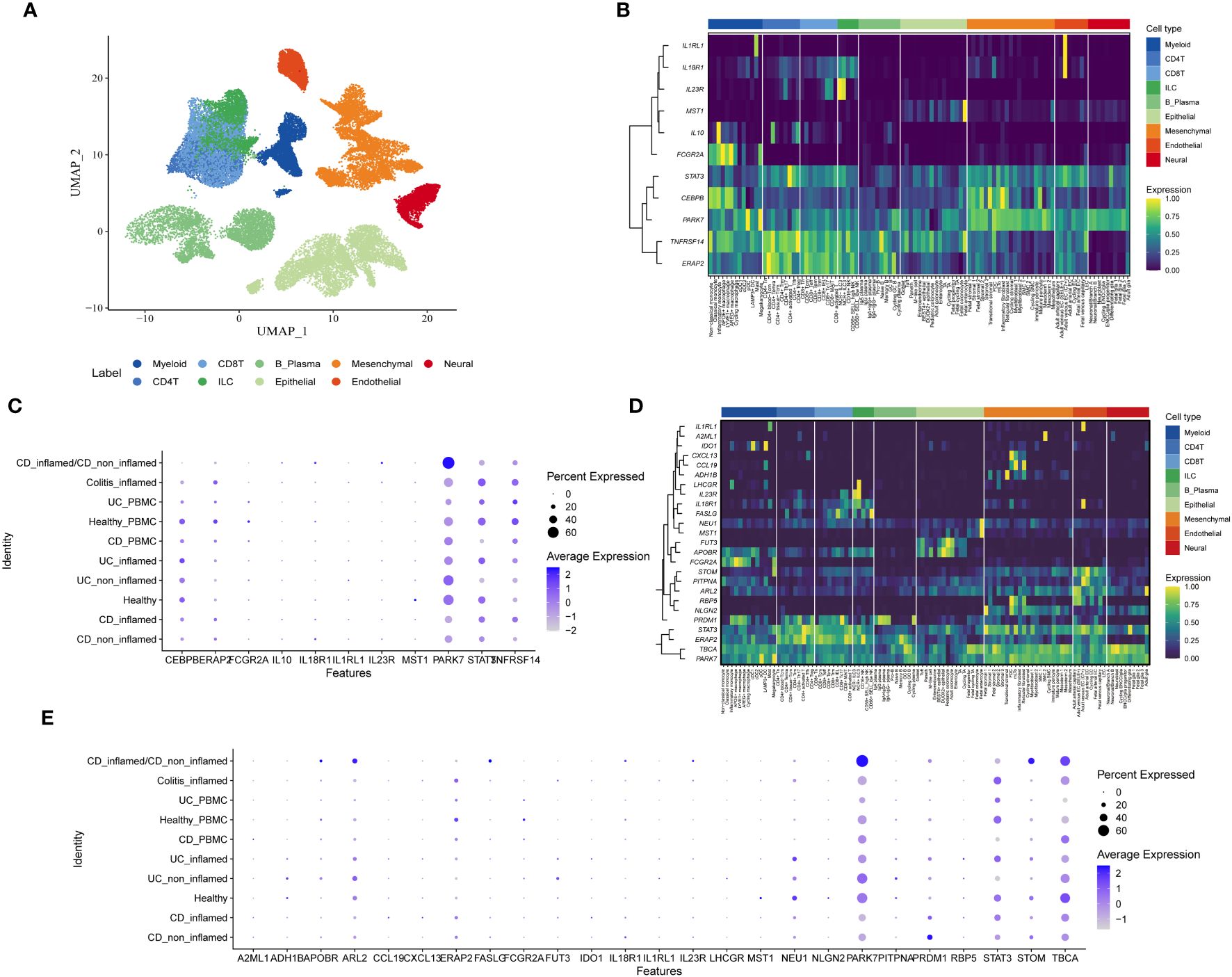
Figure 4 Single-cell type expression of protein-coding genes identified by whole proteome MR in colon tissues. (A) Umap showing 9 cell types. (B) Heatmap of expression distribution of Cis-Only MR positive proteins in different cell types. (C) Bubble chart plot of expression distribution of Cis-Only MR positive proteins in different disease states. (D) Heatmap of the expression distribution of cis + trans MR positive proteins in different cell types. (E) Bubble chart plot of expression distribution of cis + trans MR positive proteins in different disease states.
3.6 Protein-protein interaction network analysis
To deepen our comprehension of the relationship between MR-identified proteins and their enriched functions, thereby deepening our insight into the pathogenesis of IBD and its subtypes, PPI and pathway analyses were undertaken. By employing MR-prioritized proteins identified through cis-pQTLs, the PPI network consisted 14 nodes and formed 14 edges (with an expected number of edges being 3), guided by an interaction score threshold of 0.4 (medium confidence). This result indicates that this network has a notably higher number of interactions than a similarly sized collection drawn at random from the genome (enrichment p-value: 5.73×10^-7) (Supplementary Figure 1A). Regarding the proteins identified through cis and trans MR, the PPI network consisted 27 nodes and formed 14 edges, significantly surpassing the expected 4 edges (enrichment p-value: 5.15×10^-5) (Supplementary Figure 1B).
3.7 Functional enrichment analysis results
The GO pathway enrichment analysis revealed a multitude of biological pathways that were notably enriched and had a strong correlation with IBD. These pathways, enriched among the cis-MR prioritized proteins (Supplementary Table 17, Supplementary Figure 2A), encompass the generation of interferon-gamma, positive regulation of cytokine production, regulation of adaptive immune responses, modulation of interferon-gamma production, and regulation of Type 1 T helper cell immune responses. The results of this enrichment analysis highlight the critical role of immune regulatory mechanisms in IBD, particularly in the regulation of cytokine production and immune responses. Similar enrichments were observed among the proteins prioritized by cis + trans MR (Supplementary Table 18, Supplementary Figure 2B).
The KEGG pathway enrichment analysis also revealed significant pathway enrichments related to IBD. Among the cis-MR prioritized proteins, enriched pathways (Supplementary Table 19, Supplementary Figure 2C) include cytokine-cytokine receptor interaction, IBD, JAK-STAT signaling pathway, Leishmaniasis, and Tuberculosis. These enriched pathways further emphasize the central role of cytokine-mediated cellular signaling in the pathogenesis of IBD, while also revealing potential connections with other inflammatory and immune-mediated diseases. Enrichments were also found among proteins prioritized by cis + trans MR (Supplementary Table 20, Supplementary Figure 2D).
The results of these enrichment analysis provide new insights into comprehending the pathological processes of IBD, particularly regarding immune responses and cellular signaling. These insights contribute to revealing the intricate network of the development of IBD and present prospective focal points for upcoming treatment approaches.
3.8 Evaluating drug targets for MR-preferred proteins
Given the critical role of human proteins as therapeutic targets, we assessed whether proteins supported by MR evidence could be considered drug targets or have druggable properties. Through a search in the DGIdb database, we identified 15 out of 33 proteins that are considered druggable targets, covering a wide range of potential therapeutic strategies. In particular, we reassessed the potential role of these proteins in inflammatory bowel disease (IBD) therapy and immunomodulation. We found, for example, that IDO1 and IL10 genes interact with a variety of drugs, suggesting their importance in regulating immune responses and inflammatory processes. For example, the IDO1 gene demonstrated significant interactions with a variety of unapproved drugs, such as an interaction score of 8.426 with CHEMBL: CHEMBL1224312, highlighting the potential importance of IDO1 in drug development, particularly in the areas of immune regulation and anti-inflammatory therapy. Similarly, the interaction of the IL10 gene with a variety of drugs, including sirolimus, which is approved for the treatment of other diseases, as well as the unapproved PEGILODECAKIN and ILODECAKIN, further emphasizes the critical role of IL10 in the regulation of immune responses and inflammatory processes. These findings not only shed light on the underlying mechanisms of gene-drug interactions, but also provide valuable insights for future drug development and therapeutic strategies, especially in the field of IBD treatment and immunomodulation (Supplementary Table 21).
4 Discussion
The cause of Inflammatory Bowel Disease (IBD), which includes Crohn’s Disease (CD) and Ulcerative Colitis (UC), continues to be a mystery, with its origins not completely understood. There is a broad consensus that the pathogenesis of this condition is influenced by a multifaceted mix of genetic factors, immune system imbalance, environmental elements, and changes in the gut microbiome (26). Irregular immune reactions are crucial in the emergence and advancement of IBD, marked by an overabundance of immune cells and inflammatory agents, resulting in damage to intestinal tissues, imbalance, and prolonged inflammation (27, 28).
Circulating plasma proteins, including immunoglobulins (antibodies), complement proteins, and other immune modulators, play critical roles in the immune system. They are essential for maintaining immune homeostasis and modulating inflammatory responses (29). Abnormal levels or functionality of these plasma proteins can lead to immune dysregulation and exacerbate inflammatory conditions (30). Leveraging pQTL data from nine different cohorts provided by Zhang et al., supplemented with the latest pQTL data, we constructed MR tools using 10 proteomic GWAS. Sensitivity analyses were conducted on protein-trait associations supported by MR evidence, further validated through Steiger filtering and Bayesian colocalization analyses. Fourteen cis proteins were identified, including CEBPB, ERAP2, FCGR2A, HGFAC, IL10, IL12B, IL18R1, IL1RL1, IL23R, MST1, PARK7, STAT3, TNFRSF14, and TNFRSF6B. Single-cell expression analyses were undertaken to elucidate the specificity of genetically predicted circulating proteins across different cell types. PPI and functional enrichment analyses were performed using the STRING database. GO and KEGG pathway enrichment analyses performed with the ‘ClusterProfiler’ R package revealed key immunoregulatory and cellular signaling pathways associated with IBD. GO analyses highlighted the importance of the regulation of interferon-gamma production and cytokine production, while KEGG analyses highlighted the role of cytokine-cytokine receptor interactions and the JAK-STAT signaling pathway in the central role in the pathogenesis of IBD. These findings reveal a complex network of immune responses and cell signaling regulation in the development of IBD, providing new perspectives for future therapeutic strategies. Finally, the druggability of identified proteins was evaluated in the DGIdb database, along with exploring development information related to potential drugs. This study not only unveiled potential associations between circulating proteins and IBD subtypes but also provided valuable insights for future therapeutic target discovery and drug development through comprehensive bioinformatic analyses.
CEBPB, located downstream of IBD risk loci, is overexpressed in UC mucosa and strategically positioned at the interface of bone marrow and epithelial inflammatory clusters, suggesting its involvement in IBD pathogenesis (31–33). Research by Sudhakar et al. identified CEBPB’s role in CD4+ and CD14+ cells of CD patients (34), while Betal drugs modulate CRP responses to IL-1 via CEBPB (35). Dysregulation of transcription factors, including CEBPB, underscores its potential role in IBD, highlighted by whole-blood transcriptome analyses showing its significant upregulation in IBD (36). Furthermore, CEBPB affects IBD progression by regulating the expression of SCFAs, highlighting the central role of CEBPB in IBD and its potential as a therapeutic target (37).. Tamura et al. demonstrated CEBPB’s key role in monocyte/macrophage regulation, potentially affecting IBD immune responses by upregulating Csf1r expression (38), indicating CEBPB’s core function in IBD pathology and its potential as a therapeutic target.
ERAP2 gene polymorphisms, linked to resistance against lethal infections and susceptibility to autoimmune diseases including IBD (39–41), affect T-cell immune responses by processing antigen peptides (42). The involvement of ERAP2 in immune responses and inflammatory regulation may relate to its role in IBD pathogenesis. Further research is needed to understand the mechanisms linking ERAP2 with IBD and explore the potential of ERAP2 inhibitors as therapeutic interventions.
FCGR2A gene, associated with risks of various autoimmune diseases (43), has a protective effect against UC and CD through its functional variant FCGR2A*519G. Studies by Tomas Castro-Dopico and Kouichi Asano revealed the gene’s significant association with UC susceptibility and its pronounced effect in females (44–46), emphasizing FCGR2A’s importance in IBD pathogenesis and its therapeutic potential.
HGFAC, involved in cell proliferation, migration, and inflammation through activating HGF and MSP, plays roles in metabolic regulation and inflammatory responses (47–49). Its involvement in tissue repair and plasma activation post-injury suggests a role in IBD pathology, potentially influencing intestinal healing in IBD patients, making HGFAC a focus for new therapeutic approaches in IBD management.
IL-10, a key anti-inflammatory cytokine, is crucial for intestinal mucosal homeostasis. Deficiencies in IL-10 or its receptors lead to severe intestinal inflammation in mice and humans (50, 51). IL-10 polymorphism rs3024505 is associated with IBD, particularly in Europeans, highlighting IL-10’s role in UC pathophysiology and its importance in regulating mucosal immune homeostasis (52, 53).
IL12B gene, encoding the shared subunit IL-12p40 of IL-12 and IL-23, is key in IBD pathogenesis by affecting Th1 and Th17 cell differentiation and activation (54). Genetic variations in IL12B are linked to increased IBD susceptibility and disease severity (55, 56) with research indicating the potential of IL12/23p40-targeted immunotherapy for diagnosis and management, reducing invasive procedures like colonoscopy.
IL-23R, the receptor for IL-23, is implicated in IBD development through its role in Th17 cell differentiation and immune response (57, 58). Polymorphisms in IL-23R are associated with IBD risk, with specific mutations leading to abnormal IL-23R signaling and increased disease susceptibility (59, 60). Therapies targeting IL-23, such as ustekinumab, have shown effectiveness in treating moderate to severe CD and UC, underscoring the importance of ongoing research in understanding this pathway and optimizing treatments (61, 62).
IL18 and its receptor IL18R1 play significant roles in IBD pathogenesis. Genetic studies link IL18R1 to IBD susceptibility, with mutations affecting IL-18 signaling and intestinal inflammation (63, 64). Clinical trials exploring IL-12/IL-23 inhibitors’ effects in IBD treatment, alongside the causal relationship between elevated IL18 levels and IBD susceptibility, suggest repositioning existing drugs targeting IL18 signaling for IBD treatment, highlighting the therapeutic potential of targeting the IL18/IL18R1 pathway (61, 65).
IL-1RL1 (also known as IL-33R or ST2), involved in initiating inflammatory responses through IL-33 binding, is associated with IBD risk through gene polymorphisms in IL-33 and IL1RL1 (66, 67). This highlights the potential of the IL-33/IL1RL1 axis in modulating IBD susceptibility and the shared inflammatory pathways between IBD and respiratory diseases like asthma (68, 69), suggesting personalized treatment options for IBD patients resistant to certain therapies.
MST1, involved in various biological processes including cell proliferation and inflammation, is yet to be clearly defined in IBD. However, gene-gene interaction analysis shows its association with CD through polymorphisms in genes like NOD2 and ATG16L1 (70), providing new directions for understanding MST1’s role in IBD.
PARK7 (DJ-1), initially recognized for its role in neurodegenerative diseases, has been identified in the colonic mucosa of IBD children, suggesting its involvement in intestinal inflammation (71, 72). Further research is needed to clarify PARK7’s mechanisms in the gut and the efficacy of PARK7-based therapies in IBD treatment.
STAT3 plays a critical role in IBD pathogenesis, especially in inflammation and fibrosis processes. Its regulation by IL-6 family factors and the role in cell proliferation and chronic inflammation highlight the JAK/STAT3 pathway’s importance in intestinal immunity and IBD (73, 74), suggesting precise STAT3 modulation as a novel IBD treatment strategy.
TNFRSF14 is a member of the tumor necrosis factor receptor superfamily and plays a crucial role in regulating immune responses. Studies have shown that TNFRSF14 expression by innate immune cells has an important role in preventing intestinal inflammation in a T cell transfer model of colitis (75). In a Tnfsf14(-/-) mouse model, it was observed that these mice developed more severe colitis compared to control mice, indicating the protective role of TNFRSF14 in colitis (76).
TNFRSF6B (DcR3), by competitively binding with FasL, TL1A, or LIGHT, plays a role in regulating apoptosis and immune surveillance, impacting intestinal inflammation and CD development (77, 78). Further research is essential to understand the intricate mechanisms of TNFRSF6B/FasR in gut immune regulation and its potential as a therapeutic target in IBD management.
This study possesses significant strengths and limitations. First, the data integration was extensive, covering pQTL data from nine different cohorts and ten proteomics GWAS data, which enhanced the reliability and breadth of the study. The multiple advanced statistical techniques employed, such as Mendelian randomization analysis, Steiger filtering, co-localization analysis, and protein-protein interaction network analysis, ensured a comprehensive examination of the data and minimized bias. In addition, by using data from European populations, the study reduced potential confounders due to population stratification and improved the accuracy of the identified gene associations. Single-cell expression analysis High-resolution single-cell transcriptome analysis using the scIBD platform provided insight into the specific expression of circulating proteins in different cell types, providing valuable insights into the cellular mechanisms of IBD. However, there are some limitations of the study. First, population specificity limits the generalizability of the results to other populations and requires further studies in diverse populations. Despite the exclusion of polytomous instrumental variables, there may still be a degree of polytomousness that confounds the results of MR analyses. The study relied on publicly available datasets, and data quality and completeness may vary, affecting results. In addition, the cross-sectional design limited time-dynamic analysis to draw conclusions about dynamic changes in protein levels and their impact on disease progression. Taken together, the present study not only adds to existing scientific knowledge but also provides important guidance for future research and clinical practice. Future research should focus on validating findings in independent cohorts and diverse populations, exploring the functional mechanisms of proteins in IBD, and designing clinical trials to test the efficacy and safety of new therapeutic targets to advance the understanding and management of IBD.
5 Conclusion
This study, through the integration of multi-cohort pQTL data from Zhang et al. and the latest proteomics GWAS, established a MR analytical framework for circulating proteins, identifying 14 cis proteins associated with IBD, including CEBPB and ERAP2. The reliability of protein-trait associations was corroborated through sensitivity analysis, Steiger filtering, and Bayesian colocalization analysis. Single-cell expression analysis and bioinformatics approaches elucidated the specificity and biological functions of these proteins across different cell types. Furthermore, the druggability of these proteins was explored via the DGIdb database, offering fresh insights into therapeutic target discovery and drug development. This lays a foundational groundwork for future research and the formulation of treatment strategies for IBD.
Data availability statement
The datasets presented in this study can be found in online repositories. The names of the repository/repositories and accession number(s) can be found in the article/Supplementary Material.
Author contributions
BL: Writing – review & editing, Data curation, Formal analysis, Investigation, Methodology, Software, Writing – original draft. PH: Data curation, Software, Writing – original draft, Formal analysis, Writing – review & editing, Methodology, Validation. HL: Data curation, Formal analysis, Writing – original draft, Writing – review & editing, Investigation, Resources, Validation. XZ: Validation, Writing – review & editing, Project administration, Supervision. AZ: Supervision, Validation, Writing – review & editing, Visualization. YX: Visualization, Writing – review & editing, Project administration. BZ: Writing – review & editing, Resources, Supervision. JZ: Supervision, Writing – review & editing, Conceptualization.
Funding
The author(s) declare financial support was received for the research, authorship, and/or publication of this article. This research was supported by the National Natural Science Foundation of China (grant 81800531 to XZ.).
Conflict of interest
The authors declare that the research was conducted in the absence of any commercial or financial relationships that could be construed as a potential conflict of interest.
Publisher’s note
All claims expressed in this article are solely those of the authors and do not necessarily represent those of their affiliated organizations, or those of the publisher, the editors and the reviewers. Any product that may be evaluated in this article, or claim that may be made by its manufacturer, is not guaranteed or endorsed by the publisher.
Supplementary material
The Supplementary Material for this article can be found online at: https://www.frontiersin.org/articles/10.3389/fimmu.2024.1434369/full#supplementary-material
Abbreviations
IBD, Inflammatory Bowel Disease; CD, Crohn’s Disease; UC, Ulcerative Colitis; MR, Mendelian Randomization; eQTL, expression quantitative trait loci; pQTL, protein quantitative trait loci; GWAS, Genome-Wide Association Studies; SNPs, single nucleotide polymorphisms; MHC, major histocompatibility complex; LD, linkage disequilibrium; GO, Gene Ontology; KEGG, Kyoto Encyclopedia of Genes and Genomes; BP, Biological Process; CC, Cellular Component; MF, Molecular Function; DEGs, Differentially Expressed Genes; PPI, Protein-protein interaction; SCFAs, Short-Chain Fatty Acids; HGF, Hepatocyte Growth Factor; MSP, Macrophage Stimulating Protein.
References
2. American Society for Gastrointestinal Endoscopy Standards of Practice C, Shergill AK, Lightdale JR, Bruining DH, Acosta RD, Chandrasekhara V, et al. The role of endoscopy in inflammatory bowel disease. Gastrointest Endosc. (2015) 81:1101–21 e1-13. doi: 10.1016/j.gie.2014.10.030
3. Henriksen M, Jahnsen J, Lygren I, Stray N, Sauar J, Vatn MH, et al. C-reactive protein: a predictive factor and marker of inflammation in inflammatory bowel disease. Results from a prospective population-based study. Gut. (2008) 57:1518–23. doi: 10.1136/gut.2007.146357
4. Dubois-Camacho K, Ottum PA, Franco-Munoz D, de la Fuente M, Torres-Riquelme A, Diaz-Jimenez D, et al. Glucocorticosteroid therapy in inflammatory bowel diseases: From clinical practice to molecular biology. World J Gastroenterol. (2017) 23:6628–38. doi: 10.3748/wjg.v23.i36.6628
5. Zenlea T, Peppercorn MA. Immunosuppressive therapies for inflammatory bowel disease. World J Gastroenterol. (2014) 20:3146–52. doi: 10.3748/wjg.v20.i12.3146
6. Sakemi R, Mitsuyama K, Morita M, Yoshioka S, Kuwaki K, Tokuyasu H, et al. Altered serum profile of the interleukin-22 system in inflammatory bowel disease. Cytokine. (2020) 136:155264. doi: 10.1016/j.cyto.2020.155264
7. Tatsumi Y, Kakimoto K, Hara A, Mizuta N, Numa K, Kinoshita N, et al. Biomarkers for monitoring of changes in disease activity in ulcerative colitis. J Clin Med. (2023) 12. doi: 10.3390/jcm12227165
8. Zhao JH, Stacey D, Eriksson N, Macdonald-Dunlop E, Hedman AK, Kalnapenkis A, et al. Genetics of circulating inflammatory proteins identifies drivers of immune-mediated disease risk and therapeutic targets. Nat Immunol. (2023) 24:1540–51. doi: 10.1038/s41590-023-01588-w
9. Sekula P, Del Greco MF, Pattaro C, Kottgen A. Mendelian randomization as an approach to assess causality using observational data. J Am Soc Nephrol. (2016) 27:3253–65. doi: 10.1681/ASN.2016010098
10. Sun J, Zhao J, Jiang F, Wang L, Xiao Q, Han F, et al. Identification of novel protein biomarkers and drug targets for colorectal cancer by integrating human plasma proteome with genome. Genome Med. (2023) 15:75. doi: 10.1186/s13073-023-01229-9
11. Chen J, Xu F, Ruan X, Sun J, Zhang Y, Zhang H, et al. Therapeutic targets for inflammatory bowel disease: proteome-wide Mendelian randomization and colocalization analyses. EBioMedicine. (2023) 89:104494. doi: 10.1016/j.ebiom.2023.104494
12. Zhang Y, Xie J, Wen S, Cao P, Xiao W, Zhu J, et al. Evaluating the causal effect of circulating proteome on the risk of osteoarthritis-related traits. Ann rheumatic diseases. (2023) 82:1606–17. doi: 10.1136/ard-2023-224459
13. Sun BB, Chiou J, Traylor M, Benner C, Hsu YH, Richardson TG, et al. Plasma proteomic associations with genetics and health in the UK Biobank. Nature. (2023) 622:329–38. doi: 10.1038/s41586-023-06592-6
14. Suhre K, Arnold M, Bhagwat AM, Cotton RJ, Engelke R, Raffler J, et al. Connecting genetic risk to disease end points through the human blood plasma proteome. Nat Commun. (2017) 8:14357. doi: 10.1038/ncomms14357
15. Hillary RF, McCartney DL, Harris SE, Stevenson AJ, Seeboth A, Zhang Q, et al. Genome and epigenome wide studies of neurological protein biomarkers in the Lothian Birth Cohort 1936. Nat Commun. (2019) 10:3160. doi: 10.1038/s41467-019-11177-x
16. Pietzner M, Wheeler E, Carrasco-Zanini J, Raffler J, Kerrison ND, Oerton E, et al. Genetic architecture of host proteins involved in SARS-CoV-2 infection. Nat Commun. (2020) 11:6397. doi: 10.1038/s41467-020-19996-z
17. Sun BB, Maranville JC, Peters JE, Stacey D, Staley JR, Blackshaw J, et al. Genomic atlas of the human plasma proteome. Nature. (2018) 558:73–9. doi: 10.1038/s41586-018-0175-2
18. Yao C, Chen G, Song C, Keefe J, Mendelson M, Huan T, et al. Genome-wide mapping of plasma protein QTLs identifies putatively causal genes and pathways for cardiovascular disease. Nat Commun. (2018) 9:3268. doi: 10.1038/s41467-018-05512-x
19. Ferkingstad E, Sulem P, Atlason BA, Sveinbjornsson G, Magnusson MI, Styrmisdottir EL, et al. Large-scale integration of the plasma proteome with genetics and disease. Nat Genet. (2021) 53:1712–21. doi: 10.1038/s41588-021-00978-w
20. Folkersen L, Fauman E, Sabater-Lleal M, Strawbridge RJ, Franberg M, Sennblad B, et al. Mapping of 79 loci for 83 plasma protein biomarkers in cardiovascular disease. PloS Genet. (2017) 13:e1006706. doi: 10.1371/journal.pgen.1006706
21. Gilly A, Park YC, Png G, Barysenka A, Fischer I, Bjornland T, et al. Whole-genome sequencing analysis of the cardiometabolic proteome. Nat Commun. (2020) 11:6336. doi: 10.1038/s41467-020-20079-2
22. Gudjonsson A, Gudmundsdottir V, Axelsson GT, Gudmundsson EF, Jonsson BG, Launer LJ, et al. A genome-wide association study of serum proteins reveals shared loci with common diseases. Nat Commun. (2022) 13:480. doi: 10.1038/s41467-021-27850-z
23. Liu JZ, van Sommeren S, Huang H, Ng SC, Alberts R, Takahashi A, et al. Association analyses identify 38 susceptibility loci for inflammatory bowel disease and highlight shared genetic risk across populations. Nat Genet. (2015) 47:979–86. doi: 10.1038/ng.3359
24. Nie H, Lin P, Zhang Y, Wan Y, Li J, Yin C, et al. Single-cell meta-analysis of inflammatory bowel disease with scIBD. Nat Comput Sci. (2023) 3:522–31. doi: 10.1038/s43588-023-00464-9
25. Yu G, Wang LG, Han Y, He QY. clusterProfiler: an R package for comparing biological themes among gene clusters. OMICS. (2012) 16:284–7. doi: 10.1089/omi.2011.0118
26. Zhang YZ, Li YY. Inflammatory bowel disease: pathogenesis. World J Gastroenterol. (2014) 20:91–9. doi: 10.3748/wjg.v20.i1.91
27. Matsuoka K, Kanai T. The gut microbiota and inflammatory bowel disease. Semin Immunopathol. (2015) 37:47–55. doi: 10.1007/s00281-014-0454-4
28. Lee SH, Kwon JE, Cho ML. Immunological pathogenesis of inflammatory bowel disease. Intest Res. (2018) 16:26–42. doi: 10.5217/ir.2018.16.1.26
29. Chidlow JH Jr., Shukla D, Grisham MB, Kevil CG. Pathogenic angiogenesis in IBD and experimental colitis: new ideas and therapeutic avenues. Am J Physiol Gastrointest Liver Physiol. (2007) 293:G5–G18. doi: 10.1152/ajpgi.00107.2007
30. Eberhardson M, Hedin CRH, Carlson M, Tarnawski L, Levine YA, Olofsson PS. Towards improved control of inflammatory bowel disease. Scand J Immunol. (2019) 89:e12745. doi: 10.1111/sji.12745
31. Jostins L, Ripke S, Weersma RK, Duerr RH, McGovern DP, Hui KY, et al. Host-microbe interactions have shaped the genetic architecture of inflammatory bowel disease. Nature. (2012) 491:119–24. doi: 10.1038/nature11582
32. Boyd M, Thodberg M, Vitezic M, Bornholdt J, Vitting-Seerup K, Chen Y, et al. Characterization of the enhancer and promoter landscape of inflammatory bowel disease from human colon biopsies. Nat Commun. (2018) 9:1661. doi: 10.1038/s41467-018-03766-z
33. Ufer M, Hasler R, Jacobs G, Haenisch S, Lachelt S, Faltraco F, et al. Decreased sigmoidal ABCB1 (P-glycoprotein) expression in ulcerative colitis is associated with disease activity. Pharmacogenomics. (2009) 10:1941–53. doi: 10.2217/pgs.09.128
34. Sudhakar P, Verstockt B, Cremer J, Verstockt S, Sabino J, Ferrante M, et al. Understanding the molecular drivers of disease heterogeneity in crohn's disease using multi-omic data integration and network analysis. Inflammation Bowel Dis. (2021) 27:870–86. doi: 10.1093/ibd/izaa281
35. Kleemann R, Gervois PP, Verschuren L, Staels B, Princen HM, Kooistra T. Fibrates down-regulate IL-1-stimulated C-reactive protein gene expression in hepatocytes by reducing nuclear p50-NFkappa B-C/EBP-beta complex formation. Blood. (2003) 101:545–51. doi: 10.1182/blood-2002-06-1762
36. Nowak JK, Adams AT, Kalla R, Lindstrom JC, Vatn S, Bergemalm D, et al. Characterisation of the circulating transcriptomic landscape in inflammatory bowel disease provides evidence for dysregulation of multiple transcription factors including NFE2, SPI1, CEBPB, and IRF2. J Crohns Colitis. (2022) 16:1255–68. doi: 10.1093/ecco-jcc/jjac033
37. Liu Q, Peng Z, Zhou L, Peng R, Li X, Zuo W, et al. Short-chain fatty acid decreases the expression of CEBPB to inhibit miR-145-mediated DUSP6 and thus further suppresses intestinal inflammation. Inflammation. (2022) 45:372–86. doi: 10.1007/s10753-021-01552-6
38. Tamura A, Hirai H, Yokota A, Kamio N, Sato A, Shoji T, et al. C/EBPbeta is required for survival of Ly6C(-) monocytes. Blood. (2017) 130:1809–18. doi: 10.1182/blood-2017-03-772962
39. Hamilton F, Mentzer AJ, Parks T, Baillie JK, Smith GD, Ghazal P, et al. Variation in ERAP2 has opposing effects on severe respiratory infection and autoimmune disease. Am J Hum Genet. (2023) 110:691–702. doi: 10.1016/j.ajhg.2023.02.008
40. Yao Y, Liu N, Zhou Z, Shi L. Influence of ERAP1 and ERAP2 gene polymorphisms on disease susceptibility in different populations. Hum Immunol. (2019) 80:325–34. doi: 10.1016/j.humimm.2019.02.011
41. Hulur I, Gamazon ER, Skol AD, Xicola RM, Llor X, Onel K, et al. Enrichment of inflammatory bowel disease and colorectal cancer risk variants in colon expression quantitative trait loci. BMC Genomics. (2015) 16:138. doi: 10.1186/s12864-015-1292-z
42. Raja A, Kuiper JJW. Evolutionary immuno-genetics of endoplasmic reticulum aminopeptidase II (ERAP2). Genes Immun. (2023) 24:295–302. doi: 10.1038/s41435-023-00225-8
43. Weersma RK, Crusius JB, Roberts RL, Koeleman BP, Palomino-Morales R, Wolfkamp S, et al. Association of FcgR2a, but not FcgR3a, with inflammatory bowel diseases across three Caucasian populations. Inflammation Bowel Dis. (2010) 16:2080–9. doi: 10.1002/ibd.21342
44. Asano K, Matsushita T, Umeno J, Hosono N, Takahashi A, Kawaguchi T, et al. A genome-wide association study identifies three new susceptibility loci for ulcerative colitis in the Japanese population. Nat Genet. (2009) 41:1325–9. doi: 10.1038/ng.482
45. Asano K, Matsumoto T, Umeno J, Hirano A, Esaki M, Hosono N, et al. Impact of allele copy number of polymorphisms in FCGR3A and FCGR3B genes on susceptibility to ulcerative colitis. Inflammation Bowel Dis. (2013) 19:2061–8. doi: 10.1097/MIB.0b013e318298118e
46. Yang SK, Jung Y, Kim H, Hong M, Ye BD, Song K. Association of FCGR2A, JAK2 or HNF4A variants with ulcerative colitis in Koreans. Dig Liver Dis. (2011) 43:856–61. doi: 10.1016/j.dld.2011.07.006
47. Kataoka H, Kawaguchi M. Hepatocyte growth factor activator (HGFA): pathophysiological functions in vivo. FEBS J. (2010) 277:2230–7. doi: 10.1111/j.1742-4658.2010.07640.x
48. Kawaguchi M, Orikawa H, Baba T, Fukushima T, Kataoka H. Hepatocyte growth factor activator is a serum activator of single-chain precursor macrophage-stimulating protein. FEBS J. (2009) 276:3481–90. doi: 10.1111/j.1742-4658.2009.07070.x
49. Sargsyan A, Doridot L, Hannou SA, Tong W, Srinivasan H, Ivison R, et al. HGFAC is a ChREBP-regulated hepatokine that enhances glucose and lipid homeostasis. JCI Insight. (2023) 8. doi: 10.1172/jci.insight.153740
50. Shouval DS, Ouahed J, Biswas A, Goettel JA, Horwitz BH, Klein C, et al. Interleukin 10 receptor signaling: master regulator of intestinal mucosal homeostasis in mice and humans. Adv Immunol. (2014) 122:177–210. doi: 10.1016/B978-0-12-800267-4.00005-5
51. Kuhn R, Lohler J, Rennick D, Rajewsky K, Muller W. Interleukin-10-deficient mice develop chronic enterocolitis. Cell. (1993) 75:263–74. doi: 10.1016/0092-8674(93)80068-P
52. Liu M, Yuan W, Park S. Association between IL-10 rs3024505 and susceptibility to inflammatory bowel disease: A systematic review and meta-analysis. Cytokine. (2022) 149:155721. doi: 10.1016/j.cyto.2021.155721
53. Franke A, Balschun T, Karlsen TH, Sventoraityte J, Nikolaus S, Mayr G, et al. Sequence variants in IL10, ARPC2 and multiple other loci contribute to ulcerative colitis susceptibility. Nat Genet. (2008) 40:1319–23. doi: 10.1038/ng.221
54. Cooper AM, Khader SA. IL-12p40: an inherently agonistic cytokine. Trends Immunol. (2007) 28:33–8. doi: 10.1016/j.it.2006.11.002
55. Wang J, Liu H, Wang Y, Wu J, Wang C, Liu K, et al. The polymorphisms of interleukin-12B gene and susceptibility to inflammatory bowel diseases: A meta-analysis and trial sequential analysis. Immunol Invest. (2021) 50:987–1006. doi: 10.1080/08820139.2020.1863981
56. Zhao H, Qian YZ, Qian HH. Interleukin-12B gene rs6887695 and rs2288831 polymorphisms are associated with an increased risk of ulcerative colitis development in Chinese Han population: A case-control study. J Clin Lab Anal. (2020) 34:e23472. doi: 10.1002/jcla.23472
57. Abraham C, Cho J. Interleukin-23/Th17 pathways and inflammatory bowel disease. Inflammation Bowel Dis. (2009) 15:1090–100. doi: 10.1002/ibd.20894
58. Abraham C, Cho JH. IL-23 and autoimmunity: new insights into the pathogenesis of inflammatory bowel disease. Annu Rev Med. (2009) 60:97–110. doi: 10.1146/annurev.med.60.051407.123757
59. Zwiers A, Kraal L, van de Pouw Kraan TC, Wurdinger T, Bouma G, Kraal G. Cutting edge: a variant of the IL-23R gene associated with inflammatory bowel disease induces loss of microRNA regulation and enhanced protein production. J Immunol. (2012) 188:1573–7. doi: 10.4049/jimmunol.1101494
60. Zhu Y, Jiang H, Chen Z, Lu B, Li J, Shen X. Genetic association between IL23R rs11209026 and rs10889677 polymorphisms and risk of Crohn's disease and ulcerative colitis: evidence from 41 studies. Inflammation Res. (2020) 69:87–103. doi: 10.1007/s00011-019-01296-y
61. Danese S, Peyrin-Biroulet L. Are all the IL-23 blockers the same in inflammatory bowel disease? Nat Rev Gastroenterol Hepatol. (2024) 21:138–9. doi: 10.1038/s41575-023-00889-7
62. Verstockt B, Salas A, Sands BE, Abraham C, Leibovitzh H, Neurath MF, et al. IL-12 and IL-23 pathway inhibition in inflammatory bowel disease. Nat Rev Gastroenterol Hepatol. (2023) 20:433–46. doi: 10.1038/s41575-023-00768-1
63. Landy E, Carol H, Ring A, Canna S. Biological and clinical roles of IL-18 in inflammatory diseases. Nat Rev Rheumatol. (2024) 20:33–47. doi: 10.1038/s41584-023-01053-w
64. Momozawa Y, Dmitrieva J, Theatre E, Deffontaine V, Rahmouni S, Charloteaux B, et al. IBD risk loci are enriched in multigenic regulatory modules encompassing putative causative genes. Nat Commun. (2018) 9:2427. doi: 10.1038/s41467-018-04365-8
65. Mokry LE, Zhou S, Guo C, Scott RA, Devey L, Langenberg C, et al. Interleukin-18 as a drug repositioning opportunity for inflammatory bowel disease: A Mendelian randomization study. Sci Rep. (2019) 9:9386. doi: 10.1038/s41598-019-45747-2
66. Schmitz J, Owyang A, Oldham E, Song Y, Murphy E, McClanahan TK, et al. IL-33, an interleukin-1-like cytokine that signals via the IL-1 receptor-related protein ST2 and induces T helper type 2-associated cytokines. Immunity. (2005) 23:479–90. doi: 10.1016/j.immuni.2005.09.015
67. Oboki K, Ohno T, Kajiwara N, Saito H, Nakae S. IL-33 and IL-33 receptors in host defense and diseases. Allergol Int. (2010) 59:143–60. doi: 10.2332/allergolint.10-RAI-0186
68. Akhabir L, Sandford A. Genetics of interleukin 1 receptor-like 1 in immune and inflammatory diseases. Curr Genomics. (2010) 11:591–606. doi: 10.2174/138920210793360907
69. Friedrich M, Pohin M, Jackson MA, Korsunsky I, Bullers SJ, Rue-Albrecht K, et al. IL-1-driven stromal-neutrophil interactions define a subset of patients with inflammatory bowel disease that does not respond to therapies. Nat Med. (2021) 27:1970–81. doi: 10.1038/s41591-021-01520-5
70. Wu WKK, Sun R, Zuo T, Tian Y, Zeng Z, Ho J, et al. A novel susceptibility locus in MST1 and gene-gene interaction network for Crohn's disease in the Chinese population. J Cell Mol Med. (2018) 22:2368–77. doi: 10.1111/jcmm.13530
71. Pap D, Veres-Szekely A, Szebeni B, Vannay A. PARK7/DJ-1 as a therapeutic target in gut-brain axis diseases. Int J Mol Sci. (2022) 23. doi: 10.3390/ijms23126626
72. Lippai R, Veres-Szekely A, Sziksz E, Iwakura Y, Pap D, Rokonay R, et al. Immunomodulatory role of Parkinson's disease 7 in inflammatory bowel disease. Sci Rep. (2021) 11:14582. doi: 10.1038/s41598-021-93671-1
73. Nguyen PM, Putoczki TL, Ernst M. STAT3-activating cytokines: A therapeutic opportunity for inflammatory bowel disease? J Interferon Cytokine Res. (2015) 35:340–50. doi: 10.1089/jir.2014.0225
74. Salas A, Hernandez-Rocha C, Duijvestein M, Faubion W, McGovern D, Vermeire S, et al. JAK-STAT pathway targeting for the treatment of inflammatory bowel disease. Nat Rev Gastroenterol Hepatol. (2020) 17:323–37. doi: 10.1038/s41575-020-0273-0
75. Anderson CA, Boucher G, Lees CW, Franke A, D'Amato M, Taylor KD, et al. Meta-analysis identifies 29 additional ulcerative colitis risk loci, increasing the number of confirmed associations to 47. Nat Genet. (2011) 43:246–52. doi: 10.1038/ng.764
76. Krause P, Zahner SP, Kim G, Shaikh RB, Steinberg MW, Kronenberg M. The tumor necrosis factor family member TNFSF14 (LIGHT) is required for resolution of intestinal inflammation in mice. Gastroenterology. (2014) 146:1752–62 e4. doi: 10.1053/j.gastro.2014.02.010
77. Wu NL, Huang DY, Hsieh SL, Dai YS, Lin WW. Decoy receptor 3 is involved in epidermal keratinocyte commitment to terminal differentiation via EGFR and PKC activation. Exp Mol Med. (2022) 54:542–51. doi: 10.1038/s12276-022-00762-8
Keywords: inflammatory bowel disease, mendelian randomization, protein, therapeutic target, singer cell
Citation: Li B, Hu P, Liang H, Zhao X, Zhang A, Xu Y, Zhang B and Zhang J (2024) Evaluating the causal effect of circulating proteome on the risk of inflammatory bowel disease-related traits using Mendelian randomization. Front. Immunol. 15:1434369. doi: 10.3389/fimmu.2024.1434369
Received: 17 May 2024; Accepted: 17 July 2024;
Published: 31 July 2024.
Edited by:
Peng Wang, Coriell Institute For Medical Research, United StatesReviewed by:
Yuan Zhang, Children’s Hospital of Philadelphia, United StatesZhen Tian, Coriell Institute For Medical Research, United States
Chunbao Rao, University of Pennsylvania, United States
Malihatosadat Gholam Zadeh, University of Pennsylvania, United States
Poonam Dhillon, GlaxoSmithKline (United States), United States
Lite Ren, Regeneron Pharmaceuticals, Inc., United States
Yuan Liu, University of Pennsylvania, United States
Copyright © 2024 Li, Hu, Liang, Zhao, Zhang, Xu, Zhang and Zhang. This is an open-access article distributed under the terms of the Creative Commons Attribution License (CC BY). The use, distribution or reproduction in other forums is permitted, provided the original author(s) and the copyright owner(s) are credited and that the original publication in this journal is cited, in accordance with accepted academic practice. No use, distribution or reproduction is permitted which does not comply with these terms.
*Correspondence: Bin Zhang, cGhpbGV4Y2hhbmdAMTI2LmNvbQ==; Jie Zhang, emhhbmdqaWVfeGhrQHRtdS5lZHUuY24=
†These authors have contributed equally to this work and share first authorship