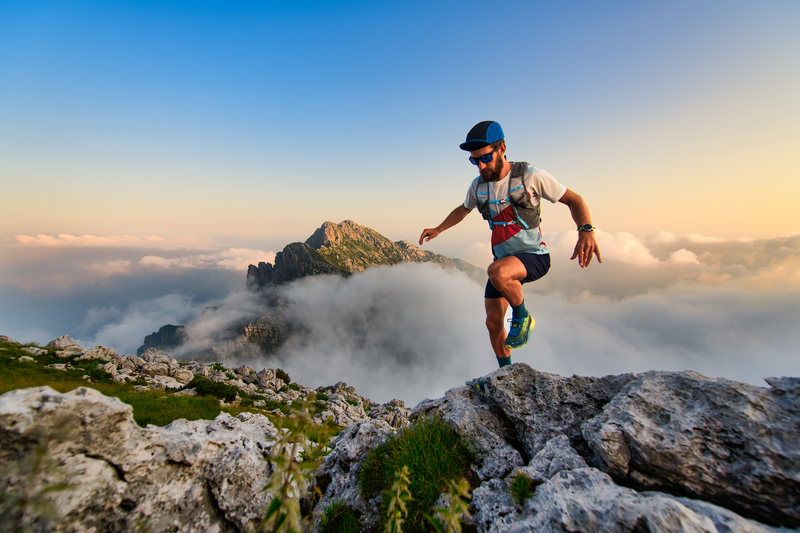
95% of researchers rate our articles as excellent or good
Learn more about the work of our research integrity team to safeguard the quality of each article we publish.
Find out more
ORIGINAL RESEARCH article
Front. Immunol. , 11 December 2024
Sec. Autoimmune and Autoinflammatory Disorders: Autoinflammatory Disorders
Volume 15 - 2024 | https://doi.org/10.3389/fimmu.2024.1426127
Psoriatic arthritis (PsA) affects approximately one in five individuals with psoriasis. Early identification of patients with psoriasis at risk of developing PsA is crucial to prevent poor prognosis. We established a derivation cohort comprising 1,661 patients with psoriasis from 49 hospitals. Clinical and demographic variables ascertained at hospital admission were screened using the Least Absolute Shrinkage and Selection Operator and logistic regression to construct a prediction model and a new web-based calculator. Ultimately, six significant independent predictors were identified: history of unexplained swollen joints (odds ratio [OR]: 5.814, 95% confidence interval [95% CI]: 3.304–10.117; p< 0.001), history of arthritis (OR: 3.543, 95% CI: 1.982–6.246; p< 0.001), history of unexplained swollen and painful fingers or toes (OR: 2.707, 95% CI: 1.463–4.915; p = 0.001), nail involvement (OR: 1.907, 95% CI: 1.235–2.912; p = 0.003), hyperlipidemia (OR: 4.265, 95% CI: 0.921–15.493; p = 0.042), and prolonged topical use of glucocorticosteroids (OR: 1.581, 95% CI: 1.052–2.384, p = 0.028). The web-based calculator derived from this model can assist clinicians in promptly determining the probability of developing PsA in patients with psoriasis, thereby facilitating improved clinical decision-making.
Psoriatic arthritis (PsA) affects approximately one in five patients with psoriasis (1), and a diagnostic delay of 6 months can lead to peripheral joint erosion and deterioration of physical function (2). Although significant advancements have been made in psoriasis treatment, early screening and preventive measures remain crucial for minimizing irreversible joint damage and improving long-term patient outcomes (3).
While factors such as obesity, nail pitting, and uveitis have been identified as potential predictors of the progression from psoriasis to PsA, existing screening tools often lack the precision needed to offer rapid and practical clinical guidance (4, 5). The limitations of these tools present a significant challenge in preventing the transition from psoriasis to PsA, emphasizing the need for more accurate and efficient predictive strategies.
Early diagnosis is essential for initiating interventions to prevent structural damage associated with PsA. Developing convenient, personalized prediction tools is critical not only for raising awareness about the importance of early intervention, but also for enabling healthcare providers to deliver targeted care. These tools can optimize the allocation of healthcare resources, ultimately leading to better management of PsA risk and improved patient outcomes. The primary objective of this study was to develop and validate a risk prediction model for PsA in patients with psoriasis with the goal of preventing disease progression and mitigating the long-term effects of structural damage.
To describe the development and validation of our multivariable prediction model, we followed the Transparent Reporting of a Multivariable Prediction Model for Individual Prognosis or Diagnosis (TRIPOD) statement (Supplementary Table S1). This study was approved by the Ethics Committee of the Yueyang Hospital of Integrated Traditional Chinese and Western Medicine (No: 2021-127; November 26, 2021). Informed consent was obtained using a web-based form, and all data were de-identified.
The China Psoriasis Diagnostic and Treatment Center platform (http://www.psocenter.cn/) was established in 2019 and includes observational, prospective, and retrospective studies. These studies investigated various aspects of psoriasis, including its burden, specific types, drug efficacy and safety, disease progression, comorbidity characteristics, and psychological impact. All the participating units underwent qualification audits. Collaborating with the platform, we collected a derivation cohort comprising 1,661 patients with psoriasis from 49 hospitals nationwide between June 24, 2020, and June 30, 2021, to develop a risk prediction model. Data from 707 patients with psoriasis collected between July 1, 2021, and December 30, 2021, were used for external validation. Patients diagnosed with PsA at baseline by a rheumatologist were excluded. The follow-up period was 1 year, and the primary outcome was the development of PsA within a one-year follow-up period. The patients were classified into two distinct groups based on whether they developed PsA. Missing data were analyzed using multiple imputations (Supplementary Figure S1).
During each follow-up visit, rheumatologists evaluated the patient’s muscles and bones. If clinical indications suggested abnormalities, further laboratory and imaging tests were performed to confirm whether the patient had developed PsA.
For patients suspected of having arthritis based on their clinical symptoms, rheumatologists performed further imaging examinations. These include:
1. X-rays: To identify any changes in bone, such as erosions or new bone formation, which can be indicative of PsA.
2. Ultrasound: To assess joint inflammation, synovitis, enthesitis, and dactylitis, which are common in PsA, in real time.
3. MRI: To provide detailed images of the joints and soft tissues, revealing inflammation and structural damage not visible on X-rays.
4. Laboratory tests: Including C-reactive protein (CRP), erythrocyte sedimentation rate (ESR), rheumatoid factor (RF), and anti-cyclic citrullinated peptide antibodies (ACPA).
The following potential predictive variables were collected at the time of initial admission: sex; age; duration of psoriasis; body mass index; marital status; educational level; smoking history; drug allergy; tumor history; photoallergy; history of unexplained joint swelling (early, potentially transient joint inflammation, which might not yet meet the diagnostic criteria for arthritis), arthritis (diagnosed with arthritis by a rheumatologist, but not including rheumatoid arthritis.), unexplained heel pain, and unexplained painful swelling of fingers or toes; family history of psoriasis; nail, scalp, or palmoplantar involvement; body surface area (BSA) involved; psoriasis area and severity index (PASI); pre-existing cardiovascular disease, type-1 or type-2 diabetes, hyperlipidemia, and hyperuricemia; abnormal transaminases; use of topical vitamin D3 derivatives, glucocorticosteroids, and tretinoin, oral methotrexate, and use of biologic therapies; satisfaction with treatment; and history of atopic dermatitis, tuberculosis, fatty liver disease, gastric ulcer, rheumatoid arthritis, and allergic rhinitis.
The following conditions were included in our study to explore their potential role as risk factors for PsA and provide a comprehensive understanding of the disease burden in patients with psoriasis.
Atopic Dermatitis (AD): Atopic dermatitis and psoriasis share overlapping immune pathways. In some cases, patients with AD may exhibit a mixed inflammatory pattern of Th2/Th17 cells (6). In addition, both psoriasis and AD involve skin barrier dysfunction and disorders of the skin microbiota that may promote inflammation. By recording the history of AD, our study aimed to investigate whether these shared pathways contribute to the development of PsA in patients with psoriasis.
Tuberculosis (TB): Patients with psoriasis, especially those treated with immunosuppressive therapies (e.g., biologics and corticosteroids), are at an increased risk of tuberculosis reactivation (7). Chronic inflammation also correlates with a higher risk of TB, which may be relevant for patients with PsA owing to the inflammatory nature of the disease.
Gastric Ulcer: Although the direct association between gastric ulcers and PsA is unclear, long-term use of NSAIDs or corticosteroids in managing psoriasis and PsA may increase the risk of gastric ulcers. By monitoring this comorbidity, we can evaluate how management strategies contribute to gastrointestinal complications in patients with psoriasis (8).
Rheumatoid Arthritis (RA): Rheumatoid arthritis (RA) and psoriatic arthritis (PsA) are distinct joint diseases. Although RA is not directly linked to PsA, its presence suggests that an individual has a propensity to develop other non-psoriatic arthritic conditions.
Allergic Rhinitis: Similar to atopic dermatitis, allergic rhinitis is driven by Th2 responses and may share immune pathways with psoriasis and PsA. Studies have suggested a possible link between allergic rhinitis and an increased risk of autoimmune diseases. Including this comorbidity allowed us to explore whether Th2-driven immune activity plays a role in PsA development (9).
In summary, these comorbidities were recorded to assess their potential role as risk factors for PsA in psoriasis patients. Understanding these associations may facilitate early detection and provide insights into the immune mechanisms underlying PsA.
A total of 39 candidate variables were included in the predictive model. Least Absolute Shrinkage and Selection Operator (LASSO) regression were used to reduce the dimensionality. LASSO regression compresses the variables by imposing a penalty function called “lambda,” simplifying the model and reducing multicollinearity and overfitting between the variables. As lambda increases, the coefficients of each variable are gradually reduced, and the unimportant variables are gradually compressed to zero, thus enabling variable selection. A 10-fold cross-validation was performed to select the best parameter (lambda). Finally, the optimal lambda corresponds to the 19 predictor variables indicated by the dotted line on the left side (Figure 1). Subsequently, these variables were entered into a multifactorial logistic regression model. Significant variables identified in the logistic regression results were used to construct a risk-prediction model and develop a web-based calculator.
Figure 1. Least Absolute Shrinkage and Selection Operator (LASSO) Regression and 10-fold Cross-Validation.
We used the area under the receiver operating characteristic curve (AUC) to quantify sample discrimination, where an AUC > 0.75 indicates a high degree of discrimination (10). Calibration was evaluated using a calibration curve based on 1,000 bootstrap samples and the Hosmer–Lemeshow test. The Hosmer–Lemeshow test p-value greater than 0.05 indicates that the predicted probability is not statistically different from the actual result, suggesting that the model has a good calibration (11). The Youden index, defined as the Youden index = true positive rate + true negative rate – 1. The Youden index can be used to calculate the optimal threshold probability for maximizing the benefits of the model. Decision curve analysis (DCA) and clinical impact curve (CIC) were implemented to validate the model’s net benefits and effectiveness.
All statistical analyses were performed using the R software (version 4.1.3; R Core Team [2022]. Vienna, Austria. URL: https://www.R-project.org/). Significance was set at p< 0.05.
The derivation cohort comprised 1,661 patients with psoriasis, 156 (9%) of whom were diagnosed with PsA at the 1-year follow-up visit. Table 1 presents the demographic and clinical characteristics of the patients with or without PsA in the derivation cohort. The validation cohort consisted of 707 patients with psoriasis, 67 (9%) of whom were diagnosed with PsA during the 1-year follow-up visit. Supplementary Table S2 presents the demographic and clinical characteristics of the external validation cohort.
After LASSO regression, the following 19 predictors were identified: age, duration of psoriasis, marital status, smoking history, history of unexplained joint swelling, arthritis, unexplained heel pain, unexplained swelling and pain in the fingers or toes, nail involvement, palmoplantar and genital involvement, BSA, presence of cardiovascular and fatty liver diseases, hyperlipidemia, rheumatoid arthritis, allergic rhinitis, prolonged topical use of glucocorticoids, and prior biological therapy.
The optimal model was selected after including these 19 variables in the logistic regression model. Ultimately, six statistically significant independent predictors were identified: history of unexplained swollen joints (odds ratio [OR]: 5.814, 95% confidence interval [95% CI]: 3.304–10.117; p< 0.001), history of arthritis (OR: 3.543, 95% CI: (1.982–6.246; p< 0.001), history of unexplained swollen and painful fingers or toes (OR: 2.707, 95% CI: 1.463–4.915; p = 0.001), nail involvement (OR: 1.907, 95% CI: 1.235–2.912; p = 0.003), hyperlipidemia (OR: 4.265, 95% CI: 0.921–15.493; p = 0.042), and prolonged topical use of glucocorticosteroids (OR: 1.581, 95% CI: 1.052–2.384, p = 0.028) (Table 2).
Table 2. Multivariate logistic regression model for predicting the development of psoriatic arthritis in patients with psoriasis.
A prediction model and a web-based calculator were developed using logistic regression analysis. The web-based calculator (https://detection.shinyapps.io/PsA-Risk/) was designed to enable clinicians to input information regarding the six predictive variables and automatically calculate the likelihood (with a 95% CI) that a patient with psoriasis will develop PsA within 1 year. Additionally, a common nomogram was created as a reference (Figure 2).
Figure 2. Online Web-Based Calculator and Common Nomogram for Predicting PsA in Patients with Psoriasis. (A) Webpage of an Online Web-Based Calculator (https://detection.shinyapps.io/PsA-Risk/). (B) QR Code for the Online Web-Based Calculator. (C) Common Nomogram of Prediction Model.
The prediction model demonstrated excellent discrimination, with AUCs of 0.80 (95% CI: 0.75–0.84) and 0.77 (95% CI: 0.70–0.84) when using the derivation and validation cohorts, respectively. Based on the Youden index, we identified potential optimal threshold probability points on a receiver operating characteristic (ROC) curve. In the derivation and validation cohorts, the optimal threshold probabilities were determined to be 0.18 (specificity = 0.95, sensitivity = 0.59) and 0.23 (specificity = 0.96, sensitivity = 0.54), respectively (Figure 3A; Supplementary Figure S2). When the probability calculated by the prediction model exceeds the threshold probability, individuals with psoriasis may be considered to be at risk of developing PsA within the subsequent year.
Figure 3. Evaluation of the Prediction Model. (A) Receiver Operator Characteristic (ROC) curve in Derivation Cohort. (B) Calibration Curve in Derivation Cohort. (C) Decision Curve Analysis (DCA) curve in Derivation Cohort. (D) Clinical Impact Curve (CIC) in Derivation Cohort. Net benefit = true positive rate - (false positive rate × weighting factor). .
The model exhibited favorable performance in terms of calibration. The p-values from the Hosmer–Lemeshow test in the derivation and validation cohorts were 0.16 and 0.11, respectively. Additionally, the calibration curve based on 1,000 bootstrap resamples indicated an excellent goodness of fit for the model (Figure 3B).
DCA and CIC were plotted to assess the model’s clinical effectiveness and net benefits. In the DCA, the X-axis represents the threshold probability, whereas the y-axis represents the net benefit.
The orange line in the DCA represents the net benefit of the model for various threshold probabilities. Additionally, two unique lines are included in the figure: a horizontal dotted line representing a scenario in which no one is treated (resulting in a net benefit of zero, regardless of the threshold probability) and a black line illustrating the change in net benefit as the probability threshold is adjusted if everyone is treated, the two lines represent the two extremes. A higher net benefit indicates better model performance for a given probability threshold and the farther the orange curve is from the two unique lines, the better the clinical performance of the model (Figure 3C). In the CIC, the X-axis corresponds to the threshold probability, whereas the y-axis represents the number of high-risk individuals. The orange line in the CIC indicates the number of high-risk patients identified using the model at various threshold probabilities. The dashed black line represents the number of high-risk individuals who developed PsA at the different probability thresholds. The closer the solid orange and dashed gray lines are to each other, the better the predictive power of the model aligns with actual clinical outcomes (Figure 3D). The DCA and CIC curves provided insights into the actual clinical performance of the model under different threshold probabilities. The DCA curve demonstrated a favorable performance, indicating a net benefit even at higher risk judgment threshold probabilities. Meanwhile, the CIC curve illustrates that the model’s predictions increasingly align with actual clinical outcomes as the risk determination threshold probability exceeds 0.2. When the threshold probability exceeds 0.6, the predicted results are almost the same as the actual results, indicating strong clinical performance of the model.
The inclusion variables were a history of unexplained swollen joints, arthritis, unexplained swollen and painful fingers or toes, nail involvement, hyperlipidemia, and prolonged topical use of glucocorticosteroids. Previous studies have shown a close association between joint tenderness and swelling in patients with PsA (12, 13). Patients with nail involvement have a higher risk of systemic enthesitis, a key feature of PsA (14). Hyperlipidemia is associated with an increased risk of psoriasis and psoriasis with PsA. HDL cholesterol is significantly lower, and LDL is markedly higher in patients with psoriatic arthritis (15, 16). Dactylitis, characterized by swollen fingers or toes, is a common sign of PsA and an important diagnostic marker for early PsA detection (17). Although a limited number of studies have suggested a potential association between topical glucocorticosteroid use and PsA, the evidence remains inconclusive (18). The direct correlation between glucocorticosteroid use and PsA development in patients with psoriasis cannot be understood in isolation. Long-term use of topical glucocorticoids may reflect the severity of the patient’s condition rather than the direct cause of PsA development.
In this study, we developed and validated a clinical prediction model using six optimal predictors to predict the probability of developing PsA in patients with psoriasis. Our model performed well, exhibiting high discrimination and calibration in both the derivation and validation cohorts. The DCA and CIC curves further underscore the practical clinical utility of the model. In addition to utilizing the optimal threshold probability point (0.18) identified on the ROC curve, clinicians can leverage DCA and CIC curves to develop individualized medical plans tailored to local medical resources and patient-specific circumstances. By referring to the DCA and CIC curves, clinicians can adjust the risk determination threshold probability upwards, aligning model predictions more closely with actual patient outcomes. This approach conserves healthcare resources and maintains the net benefit of the model’s predictions. Moreover, the model passed the external validation, and both the internal and external validation AUCs were more significant than 0.75, indicating that the model had a high level of generalizability.
Compared with existing predictive models, particularly the PRESTO model developed by Gladman et al., which utilizes predictors such as nail pitting, morning stiffness, uveitis, and biologic therapy usage, our model exhibits superior performance (19). The PRESTO model reported AUCs of 72.3% for 1-year predictions and 74.9% for 5-year predictions, whereas our model achieved an AUC of 80% for 1-year predictions, with a specificity of 85% and a sensitivity of 78%. This enhanced predictive accuracy underscores the capability of our model to effectively identify patients at risk of developing PsA. Moreover, the limitations of the existing predictive models highlight the need for more comprehensive performance metrics. Our model’s enhanced predictive capability, operational simplicity, and adaptability to resource-limited settings make it a valuable tool for the early detection and intervention of PsA. By improving risk stratification, our model aimed to facilitate timely clinical interventions that could significantly alter the disease trajectory in patients with psoriasis.
Finally, we developed a web-based risk calculator to help clinicians determine the risk of developing PsA within a year in patients with psoriasis. This would enable clinicians to make better decisions. Users scan the QR code to enter the website, select variables from the six predictors according to their conditions, and obtain the corresponding PsA risk probability with a 95% confidence interval.
This study has some limitations. First, additional laboratory-related variables should be included. Second, the data used for the derivation and validation cohorts were obtained exclusively from Chinese patients. Therefore, the generalizability of our model may be limited when applied to populations outside of China. Finally, in our cohort, 9.48% (67/707) of patients with psoriasis developed PsA within 1 year. This rate is higher than that reported in other studies. For example, Eder et al. conducted a cohort study at the Toronto Psoriasis Cohort (2006–2014) and reported an annual PsA incidence of 2.7 per 100 psoriasis patients (95% CI 2.1–3.6), increasing to 3.2 per 100 when including suspected cases (5). Similarly, Lindberg et al. (2007–2017) in Sweden reported an incidence of 1.69 per 100 patient-years, rising to 5.49 per 100 patient-years in biologically treated patients (20). The higher incidence in our cohort may be due to the inclusion of more severe psoriasis cases, as nail involvement and higher PASI scores are strong predictors of PsA.
In this study, we developed a clinical prediction model and web-based calculator based on six predictors to estimate the risk of developing PsA in patients with psoriasis. Our model provides clinicians with a valuable tool to assess the likelihood of developing PsA. This capability facilitates informed clinical decisions, enables tailored treatment strategies, and optimizes the use of medical resources.
The original contributions presented in the study are included in the article/Supplementary Material. Further inquiries can be directed to the corresponding authors.
This study was approved by the Ethics Committee of Yueyang Hospital of Integrated Traditional Chinese and Western Medicine (No: 2021-127; November 26, 2021). Written informed consent was obtained from the patients for data related to the study. All patients understood and signed an informed consent form.
CW: Data curation, Investigation, Methodology, Project administration, Software, Visualization, Writing – original draft, Writing – review & editing. SW: Data curation, Methodology, Project administration, Software, Validation, Visualization, Writing – original draft, Writing – review & editing, Conceptualization. LL: Data curation, Validation, Writing – review & editing. JW: Data curation, Validation, Writing – review & editing. XC: Data curation, Validation, Writing – review & editing. MZ: Data curation, Validation, Writing – review & editing. XS: Conceptualization, Project administration, Validation, Writing – review & editing. XL: Conceptualization, Funding acquisition, Methodology, Supervision, Writing – review & editing.
The author(s) declare financial support was received for the research, authorship, and/or publication of this article. This study was supported by the National Natural Science Foundation of China (Nos. 82374445, 82305233, and 82074427); the Key Discipline Construction Project of Shanghai’s Three-Year Action Plan for Strengthening the Construction of Public Health System (2023–2025) (No. GWVI-11.1-24); the Shanghai 2022 ‘Science and Technology Innovation Action Plan’ Medical Innovation Research Special Project (No. 22Y11922200); the Scientific Research Project of Shanghai Municipal Health Commission (No. 20224Z0019); the 2022 “Dawn” Program (No. 22SG41); the High-level Chinese Medicine Key Discipline Construction Project (Integrative Chinese and Western Medicine Clinic) of National Administration of TCM (No. zyyzdxk-2023065); the Clinical Research Plan of Shanghai Shenkang Hospital Development Center (Nos. SHDC2202230 and SHDC22022302); and the evidence-based dermatology base sponsored by the State Administration of Traditional Chinese Medicine.
We are grateful for the support provided by the China Psoriasis Diagnostic and Treatment Centre Platform.
The authors declare that the research was conducted in the absence of any commercial or financial relationships that could be construed as a potential conflict of interest.
All claims expressed in this article are solely those of the authors and do not necessarily represent those of their affiliated organizations, or those of the publisher, the editors and the reviewers. Any product that may be evaluated in this article, or claim that may be made by its manufacturer, is not guaranteed or endorsed by the publisher.
The Supplementary Material for this article can be found online at: https://www.frontiersin.org/articles/10.3389/fimmu.2024.1426127/full#supplementary-material
Supplementary Figure 1 | Proportion of Missing Data in Derivation Cohort and Validation Cohort.
Supplementary Figure 2 | Receiver Operator Characteristic (ROC) curve and Calibration Curve in Validation Cohort.
1. Alinaghi F, Calov M, Kristensen LE, Gladman DD, Coates LC, Jullien D, et al. Prevalence of psoriatic arthritis in patients with psoriasis: A systematic review and meta-analysis of observational and clinical studies. J Am Acad Dermatol. (2019) 80:251–265.e19. doi: 10.1016/j.jaad.2018.06.027
2. Haroon M, Gallagher P, FitzGerald O. Diagnostic delay of more than 6 months contributes to poor radiographic and functional outcome in psoriatic arthritis. Ann Rheum Dis. (2015) 74:1045–50. doi: 10.1136/annrheumdis-2013-204858
3. Coates LC, Soriano ER, Corp N, Bertheussen H, Callis Duffin K, Campanholo CB, et al. Group for Research and Assessment of Psoriasis and Psoriatic Arthritis (GRAPPA): updated treatment recommendations for psoriatic arthritis 2021. Nat Rev Rheumatol. (2022) 18:465–79. doi: 10.1038/s41584-022-00798-0
4. Love TJ, Zhu Y, Zhang Y, Wall-Burns L, Ogdie A, Gelfand JM, et al. Obesity and the risk of psoriatic arthritis: a population-based study. Ann Rheum Dis. (2012) 71:1273–7. doi: 10.1136/annrheumdis-2012-201299
5. Eder L, Haddad A, Rosen CF, Lee KA, Chandran V, Cook R, et al. The incidence and risk factors for psoriatic arthritis in patients with psoriasis: A prospective cohort study. Arthritis Rheumatol. (2016) 68:915–23. doi: 10.1002/art.39494
6. Guttman-Yassky E, Krueger JG, Lebwohl MG. Systemic immune mechanisms in atopic dermatitis and psoriasis with implications for treatment. Exp Dermatol. (2018) 27:409–17. doi: 10.1111/exd.13336
7. Nogueira M, Warren RB, Torres T. Risk of tuberculosis reactivation with interleukin (IL)-17 and IL-23 inhibitors in psoriasis - time for a paradigm change. J Eur Acad Dermatol Venereol. (2021) 35:824–34. doi: 10.1111/jdv.16866
8. Sostres C, Gargallo CJ, Lanas A. Nonsteroidal anti-inflammatory drugs and upper and lower gastrointestinal mucosal damage. Arthritis Res Ther. (2013) 15 Suppl 3:S3. doi: 10.1186/ar4175
9. Han JH, Bang CH, Han K, Ryu JY, Lee JY, Park YM, et al. The risk of psoriasis in patients with allergic diseases: A nationwide population-based cohort study. Allergy Asthma Immunol Res. (2021) 13:638–45. doi: 10.4168/aair.2021.13.4.638
10. Tzoulaki I, Liberopoulos G, Ioannidis JP. Assessment of claims of improved prediction beyond the Framingham risk score. Jama. (2009) 302:2345–52. doi: 10.1001/jama.2009.1757
11. Bascom KE, Dziodzio J, Vasaiwala S, Mooney M, Patel N, McPherson J, et al. Derivation and validation of the CREST model for very early prediction of circulatory etiology death in patients without ST-segment-elevation myocardial infarction after cardiac arrest. Circulation. (2018) 137:273–82. doi: 10.1161/circulationaha.116.024332
12. Gessl I, Popescu M, Schimpl V, Supp G, Deimel T, Durechova M, et al. Role of joint damage, malalignment and inflammation in articular tenderness in rheumatoid arthritis, psoriatic arthritis and osteoarthritis. Ann Rheum Dis. (2021) 80:884–90. doi: 10.1136/annrheumdis-2020-218744
13. Bosch P, Lackner A, Dreo B, Husic R, Ficjan A, Gretler J, et al. The role of tender and swollen joints for the assessment of inflammation in PsA using ultrasound. Rheumatol (Oxford). (2022) 61:Si92–si96. doi: 10.1093/rheumatology/keab764
14. Ash ZR, Tinazzi I, Gallego CC, Kwok C, Wilson C, Goodfield M, et al. Psoriasis patients with nail disease have a greater magnitude of underlying systemic subclinical enthesopathy than those with normal nails. Ann Rheum Dis. (2012) 71:553–6. doi: 10.1136/annrheumdis-2011-200478
15. Wu S, Li WQ, Han J, Sun Q, Qureshi AA. Hypercholesterolemia and risk of incident psoriasis and psoriatic arthritis in US women. Arthritis Rheumatol. (2014) 66:304–10. doi: 10.1002/art.38227
16. Jones SM, Harris CP, Lloyd J, Stirling CA, Reckless JP, McHugh NJ. Lipoproteins and their subfractions in psoriatic arthritis: identification of an atherogenic profile with active joint disease. Ann Rheum Dis. (2000) 59:904–9. doi: 10.1136/ard.59.11.904
17. Dubash S, Alabas OA, Michelena X, Garcia-Montoya L, Wakefield RJ, Helliwell PS, et al. Dactylitis is an indicator of a more severe phenotype independently associated with greater SJC, CRP, ultrasound synovitis and erosive damage in DMARD-naive early psoriatic arthritis. Ann Rheum Dis. (2022) 81:490–5. doi: 10.1136/annrheumdis-2021-220964
18. Thumboo J, Uramoto K, Shbeeb MI, O'Fallon WM, Crowson CS, Gibson LE, et al. Risk factors for the development of psoriatic arthritis: a population based nested case control study. J Rheumatol. (2002) 29:757–62.
19. Eder L, Lee KA, Chandran V, Widdifield J, Drucker AM, Ritchlin C, et al. Derivation of a multivariable psoriatic arthritis risk estimation tool (PRESTO): A step towards prevention. Arthritis Rheumatol. (2023) 1–10. doi: 10.1002/art.42661
20. Lindberg I, Lilja M, Geale K, Tian H, Richardson C, Scott A, et al. Incidence of psoriatic arthritis in patients with skin psoriasis and associated risk factors: A retrospective population-based cohort study in swedish routine clinical care. Acta Derm Venereol. (2020) 100:adv00324. doi: 10.2340/00015555-3682
Keywords: psoriatic arthritis, prediction model, web-based calculator, psoriasis, decision curve analysis
Citation: Wang C, Wang S, Liu L, Wang J, Cai X, Zhang M, Sun X and Li X (2024) Early detection of psoriatic arthritis in patients with psoriasis: construction of a multifactorial prediction model. Front. Immunol. 15:1426127. doi: 10.3389/fimmu.2024.1426127
Received: 30 April 2024; Accepted: 18 November 2024;
Published: 11 December 2024.
Edited by:
Kei Ikeda, Dokkyo Medical University, JapanReviewed by:
Zisman Devy, Carmel Medical Center, IsraelCopyright © 2024 Wang, Wang, Liu, Wang, Cai, Zhang, Sun and Li. This is an open-access article distributed under the terms of the Creative Commons Attribution License (CC BY). The use, distribution or reproduction in other forums is permitted, provided the original author(s) and the copyright owner(s) are credited and that the original publication in this journal is cited, in accordance with accepted academic practice. No use, distribution or reproduction is permitted which does not comply with these terms.
*Correspondence: Xin Li, MTM2NjE5NTYzMjZAMTYzLmNvbQ==; Xiaoying Sun, ZHJfeGlhb3lpbmdAMTI2LmNvbQ==
†These authors have contributed equally to this work
Disclaimer: All claims expressed in this article are solely those of the authors and do not necessarily represent those of their affiliated organizations, or those of the publisher, the editors and the reviewers. Any product that may be evaluated in this article or claim that may be made by its manufacturer is not guaranteed or endorsed by the publisher.
Research integrity at Frontiers
Learn more about the work of our research integrity team to safeguard the quality of each article we publish.