- 1Department of Rheumatology, The Second Hospital of Shanxi Medical University, Taiyuan, Shanxi, China
- 2Shanxi Key Laboratory for Immunomicroecology, Taiyuan, Shanxi, China
- 3Shanxi Province Engineering Research Center of Precision Medicine for Rheumatology, Taiyuan, Shanxi, China
- 4Pathology, Joint Program in Transfusion Medicine, Brigham and Women’s Hospital/Children’s Hospital, Harvard Medical School, Boston, MA, United States
Rheumatoid arthritis (RA) affects millions of people worldwide, but there are limited drugs available to treat it, so acquiring a more comprehensive comprehension of the underlying reasons and mechanisms behind inflammation is crucial, as well as developing novel therapeutic approaches to manage it and mitigate or forestall associated harm. It is evident that current in vitro models cannot faithfully replicate all aspects of joint diseases, which makes them ineffective as tools for disease research and drug testing. Organ-on-a-chip (OoC) technology is an innovative platform that can mimic the microenvironment and physiological state of living tissues more realistically than traditional methods by simulating the spatial arrangement of cells and interorgan communication. This technology allows for the precise control of fluid flow, nutrient exchange, and the transmission of physicochemical signals, such as bioelectrical, mechanical stimulation and shear force. In addition, the integration of cutting-edge technologies like sensors, 3D printing, and artificial intelligence enhances the capabilities of these models. Here, we delve into OoC models with a particular focus on Synovial Joints-on-a-Chip, where we outline their structure and function, highlighting the potential of the model to advance our understanding of RA. We integrate the actual evidence regarding various OoC models and their possible integration for multisystem disease study in RA research for the first time and introduce the prospects and opportunities of the chip in RA etiology and pathological mechanism research, drug research, disease prevention and human precision medicine. Although many challenges remain, OoC holds great promise as an in vitro model that approaches physiology and dynamics.
1 Introduction
Rheumatoid arthritis (RA) manifests as an autoimmune disorder marked by persistent, aggressive arthritis. It affects about 0.5% to 1.0% of the global population and can occur at any age, with its highest incidence between 30-50 years, with the incidence of RA in women being 2-3 times higher than in men (1–4).The origins and development of RA remain intricate and a subject of ongoing research, with a prevailing belief that genetic, environmental, and autoimmune elements could be crucial in this condition (4, 5). Over time, these elements result in an overproduction of pro-inflammatory cytokines, represented by interleukin-6 (IL-6) and tumor necrosis factor (TNF), culminating in synovial cell proliferation, followed by cartilage destruction and bone erosion (4) (Figure 1). The primary clinical symptom of this illness is recurrent symmetrical polyarthritis, commonly seen in the hands, wrists, feet and other small joints (2, 8). In the initial stage of the disease, symptoms such as joint inflammation (redness, swelling, heat, pain) and joint disorders often occur (9). As the disease progresses, a range of different degrees of joint stiffness and deformity (10), or even disability (11), can be seen. However, since RA manifests as a systemic inflammatory condition, impacting not just joints but also various other extra-articular characteristics (4, 12, 13), such as skin, cardiovascular system, digestive system, respiratory system, etc. (14, 15) (Figure 2 Part A). The current treatment methods for RA have low remission rate, many adverse reactions, and unsatisfactory efficacy, which seriously affect the quality of everyday living (16) and physical and mental health (17), and bring burdens to individuals and society (18–20). Therefore, it is essential to deepen our comprehension of the etiology and pathogenesis of RA and develop new and more effective drugs to alleviate the suffering of patients.
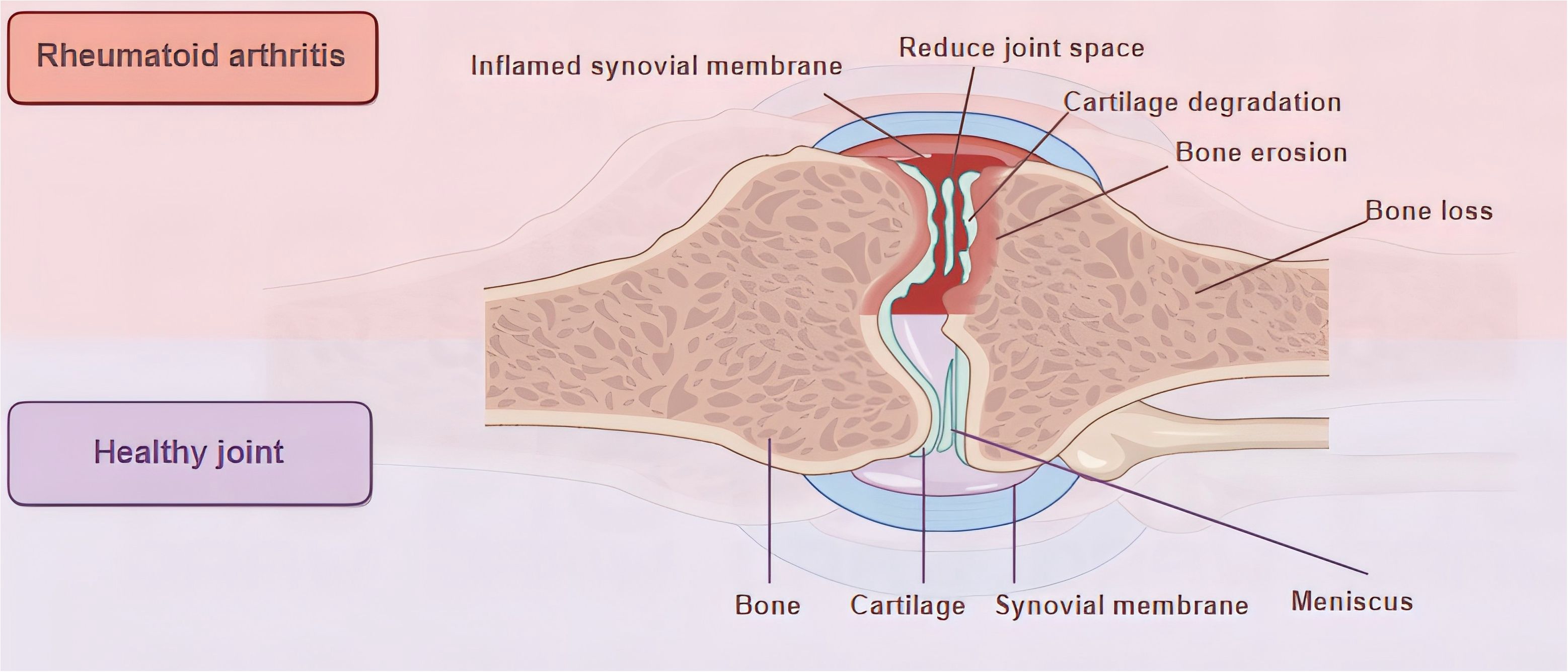
Figure 1. Joint characteristics of health and RA. During RA, the synovial membrane undergoes the following changes: infiltration of immune cells, new angiogenesis, uncontrolled proliferation of FLS, and the intima thickens and develops an aggressive pannus (6). Pannus tissue invades and destroys the underlying cartilage and bone (7), resulting in cartilage degeneration, bone destruction, and joint space narrowing.
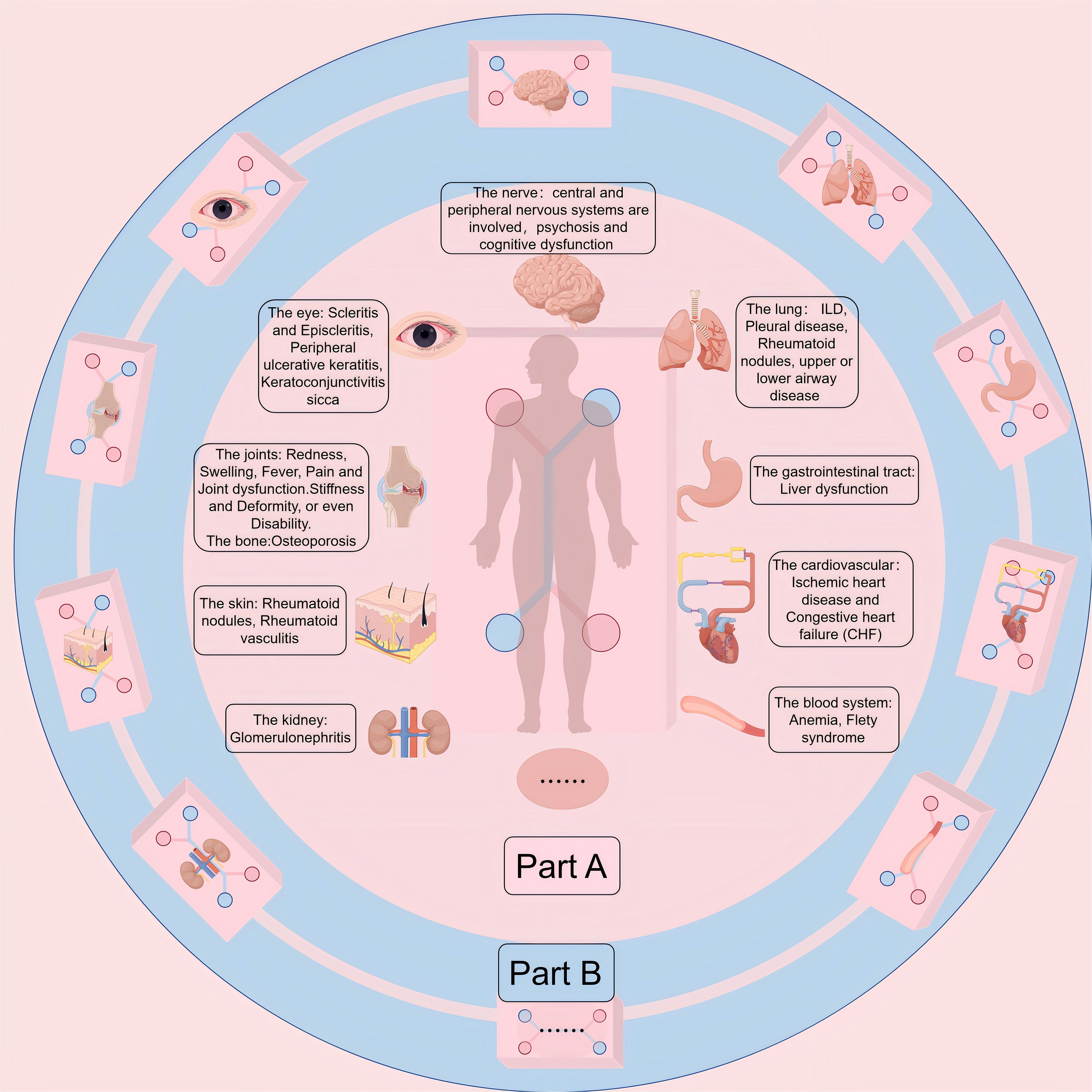
Figure 2. (Part A) Extra-Articular Manifestations (EMs) and comorbidities of RA. While synovitis serves as the pathological hallmark of RA, the intricate and persistent inflammatory and autoimmune nature of this disease gives rise to various Ems and comorbidities. These complications and comorbidities lead to increased morbidity and mortality. Among patients with RA, cardiovascular disease emerges as the primary cause of death, followed closely by respiratory ailments. (Part B) Multi-Organ-on-Chip (Multi-OoC) or Body-on-a-chip (BoC). OoC platforms can be interconnected to form more complex multi-OoC models or even BoC models, which can generalize interactions between various organs in the body, making it possible to study multi-tissue and even systemic diseases. Because intertissue crosstalk holds a pivotal position in the emergence and advancement of human, a common shared medium is often required for recycling that allows organ components to communicate with each other, while allowing them to retain their identity.
A wide range of in vitro models have been employed to reveal the tissue development and pathogenesis of joints. These include simple 2D monolayer cultures of fibroblast-like synoviocytes (FLS) (21, 22), as well as more complex models such as tissue explants of bone (23), cartilage (24, 25), synovium (26) and meniscus (27, 28), co-culture of cartilage and synovium (29) and multi-compartment bioreactor (30, 31), among others. Despite their contributions, traditional in vitro models have limitations when it comes to accurately reflecting physiological conditions (Table 1). Similarly, animal models (such as mice), which are a vital part of RA research, suffer from species-specific differences (32, 33) and high costs (34, 35). Consequently, it’s critically important to create innovative, dynamic, and physiological 3D cell culture models as substitutes for additional studies.
Compared with the traditional in vitro cell models, OoC enable precise control over cell cultivation (36), cell spatial configuration (37), and inter-organ interactions (38). Unlike static organoid culture, OoC technology enables the efficient movement of fluids, thereby improving nutrient transfer and the conveyance of physicochemical cues like bioelectrical stimulation (39), mechanical stimulation (40), and shear force. Consequently, this method provides a more precise depiction of the physiological states present in living tissue (41). Moreover, the use of OoC technology in physiological barrier models accurately mimics the delivery and penetration of compounds in vivo (42). In recent years, there has been a notable enhancement in the precision of OoC, enabling single-cell detection and high-throughput capabilities (43).
In this review, we outline the structural features and benefits of OoC, highlighting its advantages over traditional models in terms of replicating the complexity of biological systems. We then provide an overview of the current applications and accomplishments of the Synovial Joint-on-a-Chip (JoC), a specialized OoC model designed to mimic the human synovial joint. We describe the fundamental structure and functions of JoC, emphasizing its relevance to the study of RA. In addition, we delve into the potential applications of JoC in RA research, including its utility in investigating the underlying causes of the disease, understanding its pathological mechanisms and aiding drug development, and how JoC can contribute to disease prevention strategies and the field of precision medicine. We believe that although JoC still faces many challenges, with more in-depth research and the development of new technologies, the application of this chip in disease research, especially precision medicine, will be further promoted.
2 Organ-on-a-Chip: a novel and promising in vitro model
Organ-on-a-Chip (OoC, namely, microphysiological systems) is a micrometer-sized cell culture device that integrates microfluidic technology, biomaterials, and cell biology (6, 7), aiming to replicate the basic structural and functional characteristics of a specific tissue or organ (44–47). The creation of OoC systems is dependent on four key elements: First, microfluidics plays a crucial role in delivering nutrients and maintaining cellular homeostasis while also facilitating the removal of waste products. Second, living cells are integrated with biomaterials, such as hydrogels, to create a supportive environment that mimics the natural extracellular matrix. Third, physicochemical stimuli are applied to simulate the in vivo microenvironment, enhancing the system’s ability to replicate physiological conditions. Finally, sensors are incorporated to collect, process, and analyze data and images, providing valuable insights into cellular behavior and responses (7). Traditionally, microfluidic culture devices have been manufactured using soft lithography technology with materials like polydimethylsiloxane (PDMS). However, 3D printing has become a promising technology for microfluidic devices due to its low cost, standardization, and rapid production (48–50). In general, the corresponding OoC is constructed by simplifying and analyzing the different cell types and organ-specific microenvironments of the target organ and combining with the research objectives.
First and foremost, it is important to understand the target organ to determine the microstructure, including the biological context, size, and geometry-whether the microchamber or microchannel (single or multiple, parallel or sandwich structure). For example, a Synovial Membrane-on-a-Chip needs to be designed as a sandwich structure containing upper and lower layers separated by a membrane that supports intercellular communication. However, cartilage microarray usually only requires the design of a culture chamber to accommodate chondrocytes. The selection of appropriate cells is a critical step in the development of an OoC system. Cells are derived from primary human cells or mature, well-characterized cell lines (51), and stem cells, especially induced pluripotent stem cells (iPSC) (52), are an area of active and potential research. To create an environment that closely resembles the natural extracellular matrix (ECM), cells are often embedded within hydrogels. These materials can be synthetic, such as polyethylene glycol (PEG), or naturally derived, including agarose (53), alginates (54), or polysaccharides such as hyaluronic acid and glucan (55). The choice of hydrogel depends on the specific needs of the model and the desired mechanical and biochemical properties. The integration of cells within these hydrogels and their subsequent injection into the microstructures form the basis of the OoC system. This approach allows for the creation of a microenvironment that supports cell growth, function, and interaction, providing a powerful tool for studying organ-specific diseases such as RA.
Then, the integrity of OOC was increased depending on the environment to which the cells were exposed and the stimuli received. For instance, in an alveolar-capillary OoC model, alveolar epithelial cells and pulmonary microvascular endothelial cells are exposed to their respective tissue-specific environments, with air on the alveolar side and fluid on the vascular side, thus mimicking the natural conditions each cell type experiences (56). To simulate the mechanical forces that cells experience in the body, innovative design solutions have been employed. For example, vacuum pumps have been used to apply traction and compression forces to PDMS membranes, deforming them in a controlled manner (57). In the Intestinal-on-a-Chip, epithelial cells under drip and periodic mechanical deformation. In the study of musculoskeletal systems, increasing mechanical stimulation is essential to mimic the impact of physical activity on cells. Similarly, for tissues with significant electrical activity, such as those in the nervous system and the heart, integrating electrical stimulation into OoC systems is vital to enhance their physiological relevance. In addition, almost all cells are affected by biological chemical stimulus such as cytokines, growth factors, and signaling molecules, which play crucial roles in physiological responses, tissue development, and intercellular communication. Therefore, incorporating these factors into OoC models is crucial for accurately reflecting cellular behavior. Additionally, physical environmental conditions such as temperature, pH, and oxygen concentration must be carefully controlled to optimize the performance and quality of OoC systems, ensuring that they provide a reliable and representative model of the organ or tissue being studied.
In the past, the measurement of relevant parameters in the OoC platform could be achieved by multi-electrode matrix (MEA), transepithelial electrical resistance (TEER) and enzyme immunosorbent assay (ELISA) (40, 58, 59). However, the need to continuously monitor the environment and cellular behavior in real time has driven the inclusion of sensors. Such as measurements of plasma cytokines sensors (60), used to measure oxygen concentration colorimetric or fluorescence sensors (61) and electrochemical microsensors to monitor pH (62), oxygen concentration (63), and cell metabolism (64), etc.
OoC have been employed to simulate a wide range of functional tissues or organs, encompassing the lung (39, 57, 65–67), liver (68–71), heart (72–74), gut (75–77), kidney (78, 79), brain (80, 81), skin (82–84), and tumors (85–88),among others. Interlinking various OoC platforms can lead to the creation of intricate multi-OoC models (89, 90) or even Boc models(Figure 2 Part B) (91–94), facilitating the exploration of diseases affecting multiple systems or systemic diseases. RA manifests as a systemic condition that can accumulate almost every organ in the body, and people are very interested in the multi-OoC and even the BoC model that simulates RA. OoC devices have emerged as the forthcoming wave of in vitro models, bridging the divide between conventional preclinical in vitro models and clinical experiments (95).
3 Synovial Joint-on-a-Chip
The joint consists of a complex network of tissues, including various tissues such as articular cartilage, subchondral bone, synovial membrane, ligaments, and meniscus, as well as auxiliary tissues, including Hoffa fat pads, muscles, tendons, and knee patella, each with specific structural characteristics and functions. A JoC close to the physiological state should contain all parts of the joint structure, but in most cases, people add only the required parts appropriately according to their own research purposes (Table 2). Different cell types and compositions as well as complex tissue structures and interactions present a significant challenge in creating in vitro models that accurately represent the microenvironments of joint tissues. Therefore, creating innovative biological models for human joints to enhance the study of joint physiology and pathology, like osteoarthritis (OA) and RA, is essential yet challenging.
3.1 Osteochondral-on-a-Chip
Articular cartilage is a vascular and nerveless structure composed mainly of chondrocytes (110). Collagen fibers and glycosaminoglycans (GAG) are important extracellular matrix components (111). Different from cartilage, the subchondral bone is a highly vascularized and innervated tissue, characterized by its hard, calcified matrix made of type I collagen and calcium phosphate (hydroxyapatite). Physiological maintenance of bone homeostasis hinges on the equilibrium of bone development by osteoblasts and bone degradation by osteoclasts (112, 113). Calcified cartilage in the center divides the articular cartilage from the subchondral bone. Cartilage behavior is shaped by the architecture and composition of the subchondral bone, and there is molecular and physical crosstalk between the two types of tissue tissues (110, 114). In order to establish an accurate human Synovial JoC model to study the interactions between tissues, the combination of articular cartilage, osteochondral interface, and subchondral bone into an engineered Osteochondral-on-a-Chip has an important role in clarifying the development of joint ailments and assessing the effectiveness of possible treatments (31).
The Osteochondral-on-a-Chip is usually designed as a device of two compartments (Figure 3). Each compartment is composed of a suitable hydrogel matrix containing different cells (one compartment is chondrocytes, simulating articular cartilage; The other compartment is used to simulate subchondral bone, containing osteoblasts, osteoclasts and endothelial cells). It’s essential that the two hydrogel matrices possess varying hardness levels to effectively facilitate tissue formation and promote vascularization in specific areas. A thin, permeable porous membrane can divide these compartments, aiding in the communication between bone and cartilage. Furthermore, each of the two compartments will experience mechanical forces.
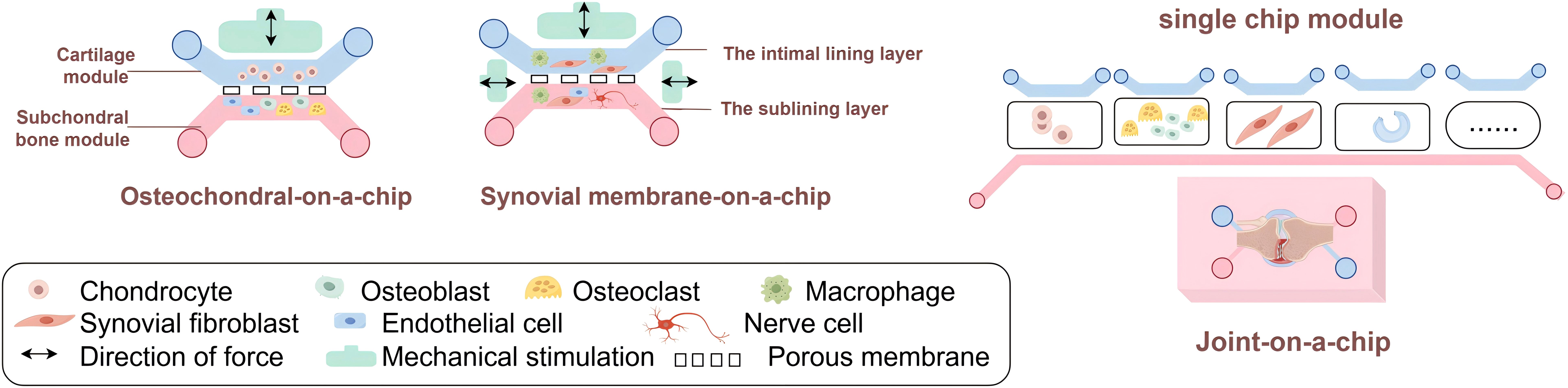
Figure 3. Synovial Joint-on-a-Chip. The multi-tissue characteristic of joint determines the complexity of synovial joint-on-a-chip. When making JoC, the usual method is to separate each joint tissue into a single chip module, and then connect the required part appropriately according to their own research purposes.
The Osteochondral-on-a-Chip of this structure have been reported several times. Research delves into how mechanical damage, contact with inflammatory cytokines, and weakened bone integrity influence the deterioration of cartilage in the osteochondral microsystem (31). Lin et al. (104) simulated the pathology of OA with microphysiological osteochondral tissue microchips and observed that celecoxib, a selective COX-2 inhibitor, down-regulated the levels of both pro-inflammatory cytokines and catabolic in the OA model, highlighting the potential usefulness of Osteochondral-on-a-Chip in drug testing. A similar chip mimics the tissue response to interleukin-1β (30).
3.2 Synovial Membrane-on-a-Chip
The synovial membrane consists of the intimal lining layer facing the joint cavity and the sublining layer. The intimal lining layer is home to two types of cells—macrophage-like synoviocytes (Type A cells) and fibroblast-like synoviocytes (FLS, Type B cells) (115–117). These loose structural features, on the one hand, allow the diffusion of nutrients to feed the avascular cartilage, and on the other, may lead to the accumulation of inflammatory substances such as immune complexes within the joint. The lamina propria contains synovial cells, macrophages, nerves and microvessels. The synovial fluid, rich in proteins, plasma, and lubricants like hyaluronic acid produced by FLS, plays a crucial role in joint nourishment and lubrication.
Synovial Membrane-on-a-Chip (also known as Synovium-on-a-Chip) is typically implemented through two microfluidic chamber devices separated by a thin membrane that support cell-to-cell communication. The upper chamber contains a layer of FLS or FLS and macrophages, while the lower chamber contains embedded FLS, macrophages, endothelial cells and nerve cells, all of which are embedded in the 3D hydrogel (Figure 3). To replicate the effects of shear stress caused by joint fluid during movement and blood circulation, either partial or ongoing perfusion in both chambers can be employed. Where membrane stretching is used, for example, in Lung-on-a-Chip (57) devices to simulate breathing and Intestine-on-a-Chip (118) devices to simulate peristalsis.
A variety of OoC systems have been created to investigate synovium’s function in rheumatic diseases. To illustrate, a vascularized Synovium-on-a-Chip that incorporates mechanical stimulation was reported to study healthy synovial membranes, synovial inflammation, and its effects on circulating monocyte behavior (98). A JoC model including vascularized synovial membrane and articular cartilage was developed and validated in a study to investigate the exfiltration of monocytes into the synovial membrane (119). A microfluidic chip-based co-culture system was evaluated for its ability to mimic bone erosion caused by FLS in RA and its potential for drug testing (100). Rothbauer and his team engineered a 3D Synovium-on-a-Chip setup to track the emergence and development of inflammatory responses in synovial tissues (109). For closer physiological proximity, the nearest Synovial Membrane-on-a-Chip incorporates synovial fluid (120).
3.3 Other joint tissues chips
Other joint tissues, including tendons, ligaments, meniscus, and Hoffa fat, we can envision designing these tissue chips in a plug-and-play form that can be flexibly adapted to applications driven by clinical needs. Lymphatic vessels have been incorporated into in vitro models of RA in several studies (121).When making the decision on which tissues or components to incorporate, it is important to find a middle ground between complexity and accuracy. The goal is to maintain a JoC model that remains biologically and/or physiologically relevant, while also promoting its widespread adoption through simplicity and user-friendliness.
3.4 Sensors for RA
Has now developed a variety of biological sensors used in RA. Some optical biosensors are used to detect RA-related biomarkers, including microRNAs (miRNAs), anti-citrullinated protein antibodies (ACPA) (122, 123), rheumatoid factor (RF) (124), and C-reactive protein (CRP) (125–127). Electrochemical nanosensor for the detection of ACPA (128). A sandwich dual electrochemical biosensor for the simultaneous detection of anti-cyclic citrullinated peptide (CCPA) and RF autoantibodies with high sensitivity and efficiency was recently reported (129). Lin et al. developed a peptide electrochemical sensor based on electrochemical impedance spectroscopy, for detecting RA autoantibodies (130). Due to the small size of nanomaterials and their unique electronic, physical and chemical properties (131), nanobiosensor devices have been developed for RA (132, 133) and will become a vital component of the next generation of point-of-care diagnostic tools, offering rapid and accurate testing options (134).
4 Prospects and opportunities of Synovial Joint-on-a-Chip in RA
4.1 To investigate the etiology and pathogenesis of RA
The onset and development of RA is linked to a range of different factors, including genetics, immunity, and the environment (such as smoking). A key benefit of using OoC in vitro models lies in their capacity to examine a single factor sequentially, thereby reducing potential disruptions in live studies (95). With the support of microfluidic technology, Several OoC devices, designed to mimic the etiology of diseases, have been created. A 3D Synovium-on-a-Chip system with four individual microcompartments has been developed to monitor the occurrence and progression of inflammatory synovial tissue responses (109).
4.1.1 Genetic susceptibility
Recent research has uncovered an expanding array of genetic factors associated with joint disorders, including epigenetic changes and genomic variations (135). Genetic factors stand out as the primary risk factor for RA (1). It is feasible to conceive of two main applications of synovial articular microchips in unraveling RA genetic susceptibility. Not only can we study specific gene functions by creating “genetically modified” OoC, but the utilization of comparative transcriptomics in the analysis of tissues derived from OoC models generated by cells from various patients and disease subgroups has the potential to elucidate previously unidentified genetic inclinations to joint diseases (135). We have reason to believe that in the near future, this new model will also be used in RA etiology and pathogenesis research.
4.1.2 Environmental factors
Smoking is known to significantly escalate the danger of RA. Benam et al. (136) constructed a smoking airway chip composed of four integrated components in vitro to study the smoke-induced pathophysiology. Another study connected small airway chips to biomimetic smoking robot microfluidics to investigate how e-cigarettes impact genetic, molecular, cellular, and tissue reactions in human lungs under controlled conditions (137). The development of cigarette chips, trachea chips, and lung chips is expected to further the understanding of how smoking affects RA.
4.1.3 Immune disorder
The immune system is pivotal in the emergence and advancement of numerous rheumatic conditions (138), and since immune malfunction is a key factor in the development of RA, it ought to be incorporated into the suggested JoC model. Recent extensive research has shown that changes in the gut microbiota of RA patients significantly contribute to the development of abnormal systemic immunity (139–141). Lately, the rapid evolution of Gut-on-a-Chip (or Intestinal-on-a-Chip) technology has opened new avenues for disease research, including RA. 1) Gut-Microbiome-on-a-Chip. The Gut-on-a-Chip developed by Jalili-Firoozinezhad’s team coculture human gut epithelial cells with either anaerobic or aerobic gut microbiota (by producing a controlled oxygen gradient) to examine the immediate connections between gut bacteria and intestinal tissues (142).A separate research by Gumuscu and colleagues involved co-culturing intestinal bacteria (E. coli) cells with Caco-2, perfusion continuously using a microfluidic apparatus, to initially assess the effects of drugs (143). 2) Gut-Immune Interactions-on-a-Chip. Kim and colleagues have created a bespoke human Gut-on-a-Chip system designed to explore the dynamics of gut and immune responses (144). Similarly, Shin and Kim designed an immune model using a similar approach to observe the early stages of inflammation in the gut (145).
Currently, the Gut-on-a-Chip is already used for conditions such as phenylketonuria (146), viral infections (147) and inflammatory bowel disease (IBD) (148). Although there is no precedent for RA, the gut is closely related to RA (intestinal infection can be the cause of RA, intestinal microbial disturbance is the pathogenesis of RA, RA can be manifested as digestive system involvement, and the gut itself and intestinal flora have a certain effect on drug efficacy). The growing research on the gut-joint axis suggests a promising future for Gut-on-a-Chip technology in the study of RA.
4.2 Screening of drugs
Developing new therapeutic agents and treatments is a recognized challenge due to its high cost, complexity, extended duration, and high failure rates. The OoC technology, with its high throughput, integration, and reproducibility, offers a promising solution to reduce the costs associated with drug research and development and is increasingly being utilized in drug screening and analysis.
4.2.1 Modeling of pharmacokinetics and pharmacodynamics
Following the identification of potential molecules and targets, studies focusing on pharmacokinetics and pharmacodynamics (PK and PD) are conducted. On the one hand, PK is used to describe the metabolic process of drug candidates in vivo, namely absorption, distribution, metabolism and elimination (ADME). On the other hand, PD refers to the drug’s impact on a specific tissue or organ, namely, how pharmacology or toxicology interacts with the drug’s dosage or concentration (149). Integrating PK/PD factors is vital for the advancement of new medications, as it forecasts potential drug reactions, thereby minimizing harmful metabolites and side effects (93, 150).
4.2.2 Evaluating drug safety and efficacy
The safety and efficacy of drugs are usually not predicted in animal models (151–153).The pharmacokinetics of drugs are affected by many factors, and toxicity testing and screening of drugs with inappropriate disease models may lead to potential multi-organ side effects (41). Multi-OoC (154) or human BoC models (94), which couple complex whole-body physiological reactions from two or more organ chips in vitro, facilitate the study of organ interactions and the identification of possible adverse effects (155). Additionally, these models offer a basis for examining interactions between drugs (156). A microfluidic chip co-culture of FLSs with osteoblasts and osteoclasts reestablishes the migration and invasion abilities of bone-associated cells, providing a valuable tool for anti-RA drug screening for FLS migration-mediated targeted bone erosion (100).
Furthermore, during the quest for novel medications, an additional critical factor impacting drug efficacy is the effective method of drug delivery within the patient’s system (157). Therefore, developments in Skin-on-a-Chip for transdermal administration assessment (158), Lung-on-a-Chip for inhalation administration evaluation (159, 160), Gut-on-a-Chip for oral/rectal administration testing (161, 162), and models for vascularization in intravenous administration testing (163) have been achieved. Additionally, this approach facilitates the examination of potential drugs in at-risk groups such as expectant mothers, kids, and the aged, who are frequently omitted from clinical studies (164).
4.2.3 Personalized drug therapy
In recent years, high-throughput OoC utilizing multi-chamber and compatible dosing concentration gradient generators is a new method for drug development or “personalized” drug therapy, which can automatically process multiple drug combinations of different concentrations within a brief span of time to improve the efficiency of drug development (165). The organoid chip developed by Schuster et al. (166) can perform individual, combined and ordered drug screening.
The continuous or sampling drug administration, coupled with managing the culture environment, enables the evaluation of intricate dosing doses and cycles, and even combined therapies. This will provide a more precise and personalized regimen for the periodic combination of medications in RA patients. Evidence has shown that the anti-inflammatory and anti-metabolic responses of certain drugs [Celecoxib, a drug that is non-steroidal and anti-inflammatory (167), cortisol hormone dexamethasone (168), interleukin-1 receptor antagonist (169)] can be predicted using in a Cartilage-on-a-Chip model (103). OoC, especially multi-OoC, a new in vitro model, is expected to screen potentially effective drugs for improving RA symptoms and controlling disease progression, and even take into account the functional status of patients’ organs to achieve personalized medication.
4.3 Diagnosis and early prevention
The progression of RA initiates in a state of health, succeeded by the onset of preclinical RA, which poses a risk for RA, then transitions to early synovitis, and ultimately culminates in established, destructive disease (1). According to the 2010 American College of Rheumatology/European League Against Rheumatism (ACR/EULAR), early diagnosis and treatment are important in improving the prognosis of RA. The detection method that simultaneously targets RF and anti-CCP is integrated in a 45 mm × 62 mm microfluidic chip, which is of great significance for the diagnosis of RA, especially early RA (170). Studies have shown that depending on the cells and stimuli used, OoC can be used to model the different stages of RA development (i.e., preclinical RA, early RA, and established RA) to understand the impact of RA pathology on various organs over both immediate and extended periods (171), which could lead to renewed optimism in the early detection and potential prevention of RA. However, at present, joint tissues are mainly obtained from patients with advanced joint diseases (172). Lack of joint tissue in healthy or early-stage joint disease is a major obstacle.
4.4 Individual precision medicine
Individuals exhibit unique genetic profiles, diverse living conditions, and varied disease mechanisms, leading to distinct clinical presentations and complications. Tailoring medical care to these individual differences is essential. It is meaningful to stratify patient subgroups and even create “Your Chip” or “Patient-on-a-Chip” to achieve true personalized medical care. Even within the same patient, changes in the chip’s responses at different disease stages can provide valuable insights for targeted interventions. OoC applications may be particularly useful in cases where alternative techniques fail to replicate genetic disorders (173), or when studies in humans are difficult (e.g., special populations such as pregnant women and young children, new drugs that have not yet been tested in clinical trials, potentially pathogenic radiation, etc.).
Despite the rapid development of OoC technology, integrating various mature tissues while maintaining their characteristics in multi-OoC systems is still in its infancy. Phenotypes during organ maturation are influenced by a variety of factors, such as the microenvironment composed of nutrients, metabolites, local substrates, circulating immune cells, and metabolites. As a result, the BoC has not yet been fully realized, although it is developing at a rapid pace.
5 Conclusion and future prospects
In this review, for the first time, we have compiled practical evidence that various systems are establishing OoC, and innovatively analyzed the possibility of integrating various systems into systemic disease RA. This move undoubtedly provides a new alternative model for studying RA. Although there are still challenges and shortcomings, OoC combines the advantages of medical biology and engineering, and can effectively solve the constraints of animal models and traditional in vitro models. In addition to realizing 3D cell culture, the system reproduces the relationship between cells and their matrix. Moreover, it can simulate the interstitial fluid and mechanical force stimulation of target organs in vivo, which has unparalleled advantages in the bionic microenvironment.
The future development trend of OoC will be a system composed of multiple organs with the help of connected pipes-human organ bionic chips. This requires more research on population proportions, differentiation conditions, spatial distribution among multicellular populations, and the determination of amplification factors to simulate the complex physiological environment in vivo. Due to its reproducibility and accuracy, OoC is likely to completely change the way medical research is conducted in the future, replacing animal and 2D cell experiments and becoming a new tool for more functional, integrated, automated and personalized preclinical research.
The utilization of emerging technologies, such as artificial intelligence and regulatory algorithms, to establish connections with the OoC systems has the potential to enhance their application in the fields of precision medicine and systems biology (174). With the breakthrough of engineering challenges, the integration of technologies in various fields, and the smooth advancement of the industrialization layout of corresponding companies, emerging technologies such as 3D printing, OoC and nanotechnology may be expected to trigger a revolution in the creation of novel medications and make the track full of vitality.
Author contributions
XZ: Writing – original draft, Conceptualization. RS: Writing – review & editing, Supervision, Methodology. HW: Writing – review & editing, Supervision. RW: Writing – review & editing, Methodology. YF: Writing – review & editing, Supervision. ZB: Writing – review & editing. CW: Writing – review & editing, Conceptualization, Supervision. CG: Writing – review & editing.
Funding
The author(s) declare financial support was received for the research, authorship, and/or publication of this article. This work was supported by National Natural Science Foundation of China (No.81971543), Four “Batches” Innovation Project of Invigorating Medical through Science and Technology of Shanxi Province (NO.2022XM05) and the Central Guidance Special Funds for Local Science and Technology Development (YDZJSX20231A061).
Conflict of interest
The authors declare that the research was conducted in the absence of any commercial or financial relationships that could be construed as a potential conflict of interest.
Publisher’s note
All claims expressed in this article are solely those of the authors and do not necessarily represent those of their affiliated organizations, or those of the publisher, the editors and the reviewers. Any product that may be evaluated in this article, or claim that may be made by its manufacturer, is not guaranteed or endorsed by the publisher.
References
1. Gravallese EM, Firestein GS. Rheumatoid arthritis — Common origins, divergent mechanisms. N Engl J Med. (2023) 388:529–42. doi: 10.1056/NEJMra2103726
3. Smolen JS, Aletaha D, Barton A, Burmester GR, Emery P, Firestein GS, et al. Rheumatoid arthritis. Nat Rev Dis Primers. (2018) 4:18001. doi: 10.1038/nrdp.2018.1
4. Smolen JS, Aletaha D, McInnes IB. Rheumatoid arthritis. Lancet. (2016) 388:2023–38. doi: 10.1016/S0140-6736(16)30173-8
5. McInnes IB. The pathogenesis of rheumatoid arthritis. New Engl J Med. (2011) 365:2205–19. doi: 10.1056/NEJMra1004965
6. Li J, Chen J, Bai H, Wang H, Hao S, Ding Y, et al. An overview of organs-on-chips based on deep learning. Res (Wash D C). (2022) 2022:9869518. doi: 10.34133/2022/9869518
7. Morais AS, Mendes M, Cordeiro MA, Sousa JJ, Pais AC, Mihăilă SM, et al. Organ-on-a-chip: ubi sumus? Fundamentals and design aspects. Pharmaceutics. (2024) 16:615. doi: 10.3390/pharmaceutics16050615
8. Makuch S, Więcek K, Woźniak M. The immunomodulatory and anti-inflammatory effect of curcumin on immune cell populations, cytokines, and in vivo models of rheumatoid arthritis. Pharm (Basel). (2021) 14:309. doi: 10.3390/ph14040309
10. Ding Q, Hu W, Wang R, Yang Q, Zhu M, Li M, et al. Signaling pathways in rheumatoid arthritis: implications for targeted therapy. Sig Transduct Target Ther. (2023) 8:68. doi: 10.1038/s41392-023-01331-9
11. Scott DL, Pugner K, Kaarela K, Doyle DV, Woolf A, Holmes J, et al. The links between joint damage and disability in rheumatoid arthritis. Rheumatol (Oxford). (2000) 39:122–32. doi: 10.1093/rheumatology/39.2.122
12. Scott DL, Wolfe F, Huizinga TWJ. Rheumatoid arthritis. Lancet. (2010) 376:1094–108. doi: 10.1016/S0140-6736(10)60826-4
13. Bullock J, Rizvi SAA, Saleh AM, Ahmed SS, Do DP, Ansari RA, et al. Rheumatoid arthritis: A brief overview of the treatment. Med Princ Pract. (2018) 27:501–7. doi: 10.1159/000493390
14. Turesson C, O’Fallon WM, Crowson CS, Gabriel SE, Matteson EL. Extra-articular disease manifestations in rheumatoid arthritis: incidence trends and risk factors over 46 years. Ann Rheum Dis. (2003) 62:722–7. doi: 10.1136/ard.62.8.722
15. Figus FA, Piga M, Azzolin I, McConnell R, Iagnocco A. Rheumatoid arthritis: Extra-articular manifestations and comorbidities. Autoimmun Rev. (2021) 20:102776. doi: 10.1016/j.autrev.2021.102776
16. Giacomelli R, Gorla R, Trotta F, Tirri R, Grassi W, Bazzichi L, et al. Quality of life and unmet needs in patients with inflammatory arthropathies: results from the multicentre, observational RAPSODIA study. Rheumatol (Oxford). (2015) 54:792–7. doi: 10.1093/rheumatology/keu398
17. Chaurasia N, Singh A, Singh I, Singh T, Tiwari T. Cognitive dysfunction in patients of rheumatoid arthritis. J Family Med Prim Care. (2020) 9:2219. doi: 10.4103/jfmpc.jfmpc_307_20
18. Roodenrijs NMT, van der Goes MC, Welsing PMJ, Tekstra J, Lafeber FPJG, Jacobs JWG, et al. Difficult-to-treat rheumatoid arthritis: contributing factors and burden of disease. Rheumatol (Oxford). (2021) 60:3778–88. doi: 10.1093/rheumatology/keaa860
19. Hsieh P-H, Wu O, Geue C, McIntosh E, McInnes IB, Siebert S. Economic burden of rheumatoid arthritis: a systematic review of literature in biologic era. Ann Rheum Dis. (2020) 79:771–7. doi: 10.1136/annrheumdis-2019-216243
20. Cross M, Smith E, Hoy D, Carmona L, Wolfe F, Vos T, et al. The global burden of rheumatoid arthritis: estimates from the global burden of disease 2010 study. Ann Rheum Dis. (2014) 73:1316–22. doi: 10.1136/annrheumdis-2013-204627
21. Braun HJ, Kim HJ, Chu CR, Dragoo JL. The effect of platelet-rich plasma formulations and blood products on human synoviocytes: implications for intra-articular injury and therapy. Am J Sports Med. (2014) 42:1204–10. doi: 10.1177/0363546514525593
22. Silverstein AM, Stefani RM, Sobczak E, Tong EL, Attur MG, Shah RP, et al. Toward understanding the role of cartilage particulates in synovial inflammation. Osteoarthritis Cartilage. (2017) 25:1353–61. doi: 10.1016/j.joca.2017.03.015
23. Coughlin TR, Schiavi J, Alyssa Varsanik M, Voisin M, Birmingham E, Haugh MG, et al. Primary cilia expression in bone marrow in response to mechanical stimulation in explant bioreactor culture. Eur Cell Mater. (2016) 32:111–22. doi: 10.22203/ecm.v032a07
24. Grenier S, Bhargava MM, Torzilli PA. An in vitro model for the pathological degradation of articular cartilage in osteoarthritis. J Biomech. (2014) 47:645–52. doi: 10.1016/j.jbiomech.2013.11.050
25. van Caam A, Madej W, Thijssen E, Garcia de Vinuesa A, van den Berg W, Goumans M-J, et al. Expression of TGFβ-family signalling components in ageing cartilage: age-related loss of TGFβ and BMP receptors. Osteoarthritis Cartilage. (2016) 24:1235–45. doi: 10.1016/j.joca.2016.02.008
26. Andersen M, Boesen M, Ellegaard K, Söderström K, Søe NH, Spee P, et al. Association between IL-6 production in synovial explants from rheumatoid arthritis patients and clinical and imaging response to biologic treatment: A pilot study. PloS One. (2018) 13:e0197001. doi: 10.1371/journal.pone.0197001
27. Hennerbichler A, Moutos FT, Hennerbichler D, Weinberg JB, Guilak F. Interleukin-1 and tumor necrosis factor alpha inhibit repair of the porcine meniscus in vitro. Osteoarthritis Cartilage. (2007) 15:1053–60. doi: 10.1016/j.joca.2007.03.003
28. Cucchiarini M, Schmidt K, Frisch J, Kohn D, Madry H. Overexpression of TGF-β via rAAV-Mediated Gene Transfer Promotes the Healing of Human Meniscal Lesions Ex Vivo on Explanted Menisci. Am J Sports Med. (2015) 43:1197–205. doi: 10.1177/0363546514567063
29. Beekhuizen M, Bastiaansen-Jenniskens YM, Koevoet W, Saris DBF, Dhert WJA, Creemers LB, et al. Osteoarthritic synovial tissue inhibition of proteoglycan production in human osteoarthritic knee cartilage: establishment and characterization of a long-term cartilage-synovium coculture. Arthritis Rheum. (2011) 63:1918–27. doi: 10.1002/art.30364
30. Lin H, Lozito TP, Alexander PG, Gottardi R, Tuan RS. Stem cell-based microphysiological osteochondral system to model tissue response to interleukin-1β. Mol Pharm. (2014) 11:2203–12. doi: 10.1021/mp500136b
31. Lozito TP, Alexander PG, Lin H, Gottardi R, Cheng AW-M, Tuan RS. Three-dimensional osteochondral microtissue to model pathogenesis of osteoarthritis. Stem Cell Res Ther. (2013) 4 Suppl 1:S6. doi: 10.1186/scrt367
32. McNamee K, Williams R, Seed M. Animal models of rheumatoid arthritis: How informative are they? Eur J Pharmacol. (2015) 759:278–86. doi: 10.1016/j.ejphar.2015.03.047
33. Denayer T, Stöhr T, Van Roy M. Animal models in translational medicine: Validation and prediction. New Horizons Trans Med. (2014) 2:5–11. doi: 10.1016/j.nhtm.2014.08.001
34. McCoy AM. Animal models of osteoarthritis: comparisons and key considerations. Vet Pathol. (2015) 52:803–18. doi: 10.1177/0300985815588611
35. Thysen S, Luyten FP, Lories RJU. Targets, models and challenges in osteoarthritis research. Dis Model Mech. (2015) 8:17–30. doi: 10.1242/dmm.016881
36. Booth R, Kim H. Characterization of a microfluidic in vitro model of the blood-brain barrier (μBBB). Lab Chip. (2012) 12:1784–92. doi: 10.1039/c2lc40094d
37. Ho C-T, Lin R-Z, Chen R-J, Chin C-K, Gong S-E, Chang H-Y, et al. Liver-cell patterning lab chip: mimicking the morphology of liver lobule tissue. Lab Chip. (2013) 13:3578–87. doi: 10.1039/c3lc50402f
38. Sung JH, Shuler ML. A micro cell culture analog (microCCA) with 3-D hydrogel culture of multiple cell lines to assess metabolism-dependent cytotoxicity of anti-cancer drugs. Lab Chip. (2009) 9:1385–94. doi: 10.1039/b901377f
39. Humayun M, Chow C-W, Young EWK. Microfluidic lung airway-on-a-chip with arrayable suspended gels for studying epithelial and smooth muscle cell interactions. Lab Chip. (2018) 18:1298–309. doi: 10.1039/c7lc01357d
40. Koutsouras DA, Perrier R, Villarroel Marquez A, Pirog A, Pedraza E, Cloutet E, et al. Simultaneous monitoring of single cell and of micro-organ activity by PEDOT: PSS covered multi-electrode arrays. Materials Sci Engineering: C. (2017) 81:84–9. doi: 10.1016/j.msec.2017.07.028
41. Saiding Q, Ma J, Ke C, Cui W. From “organs on a chip” to “patient on a chip. Innovation. (2022) 3:100282. doi: 10.1016/j.xinn.2022.100282
42. Griffith LG, Swartz MA. Capturing complex 3D tissue physiology in vitro. Nat Rev Mol Cell Biol. (2006) 7:211–24. doi: 10.1038/nrm1858
43. Shukla VC, Kuang T-R, Senthilvelan A, Higuita-Castro N, Duarte-Sanmiguel S, Ghadiali SN, et al. Lab-on-a-chip platforms for biophysical studies of cancer with single-cell resolution. Trends Biotechnol. (2018) 36:549–61. doi: 10.1016/j.tibtech.2018.02.007
44. Chen X, Zhang YS, Zhang X, Liu C. Organ-on-a-chip platforms for accelerating the evaluation of nanomedicine. Bioact Mater. (2021) 6:1012–27. doi: 10.1016/j.bioactmat.2020.09.022
45. Vunjak-Novakovic G, Ronaldson-Bouchard K, Radisic M. Organs-on-a-chip models for biological research. Cell. (2021) 184:4597–611. doi: 10.1016/j.cell.2021.08.005
46. Low LA, Mummery C, Berridge BR, Austin CP, Tagle DA. Organs-on-chips: into the next decade. Nat Rev Drug Discovery. (2021) 20:345–61. doi: 10.1038/s41573-020-0079-3
47. Bhatia SN, Ingber DE. Microfluidic organs-on-chips. Nat Biotechnol. (2014) 32:760–72. doi: 10.1038/nbt.2989
48. Prabhakar P, Sen RK, Dwivedi N, Khan R, Solanki PR, Srivastava AK, et al. 3D-printed microfluidics and potential biomedical applications. Front Nanotechnol. (2021) 3:609355. doi: 10.3389/fnano.2021.609355
49. Waheed S, Cabot JM, Macdonald NP, Lewis T, Guijt RM, Paull B, et al. 3D printed microfluidic devices: enablers and barriers. Lab Chip. (2016) 16:1993–2013. doi: 10.1039/c6lc00284f
50. Yi HG, Lee H, Cho DW. 3D printing of organs-on-chips. Bioengineering (Basel Switzerland). (2017) 4:10. doi: 10.3390/bioengineering4010010
51. Ikada Y. Challenges in tissue engineering. J R Soc Interface. (2006) 3:589–601. doi: 10.1098/rsif.2006.0124
52. Karagiannis P, Takahashi K, Saito M, Yoshida Y, Okita K, Watanabe A, et al. Induced pluripotent stem cells and their use in human models of disease and development. Physiol Rev. (2019) 99:79–114. doi: 10.1152/physrev.00039.2017
53. Salati MA, Khazai J, Tahmuri AM, Samadi A, Taghizadeh A, Taghizadeh M, et al. Agarose-based biomaterials: opportunities and challenges in cartilage tissue engineering. Polymers (Basel). (2020) 12:1150. doi: 10.3390/polym12051150
54. Almqvist KF, Dhollander AAM, Verdonk PCM, Forsyth R, Verdonk R, Verbruggen G. Treatment of cartilage defects in the knee using alginate beads containing human mature allogenic chondrocytes. Am J Sports Med. (2009) 37:1920–9. doi: 10.1177/0363546509335463
55. Jin R, Moreira Teixeira LS, Dijkstra PJ, Zhong Z, van Blitterswijk CA, Karperien M, et al. Enzymatically crosslinked dextran-tyramine hydrogels as injectable scaffolds for cartilage tissue engineering. Tissue Eng Part A. (2010) 16:2429–40. doi: 10.1089/ten.TEA.2009.0764
56. Park SE, Georgescu A, Huh D. Organoids-on-a-chip. Science. (2019) 364:960–5. doi: 10.1126/science.aaw7894
57. Huh D, Matthews BD, Mammoto A, Montoya-Zavala M, Hsin HY, Ingber DE. Reconstituting organ-level lung functions on a chip. Science. (2010) 328:1662–8. doi: 10.1126/science.1188302
58. Henry OYF, Villenave R, Cronce MJ, Leineweber WD, Benz MA, Ingber DE. Organs-on-chips with integrated electrodes for trans-epithelial electrical resistance (TEER) measurements of human epithelial barrier function. Lab Chip. (2017) 17:2264–71. doi: 10.1039/C7LC00155J
59. Maoz BM, Herland A, Henry OYF, Leineweber WD, Yadid M, Doyle J, et al. Organs-on-Chips with combined multi-electrode array and transepithelial electrical resistance measurement capabilities. Lab Chip. (2017) 17:2294–302. doi: 10.1039/C7LC00412E
60. Zhu J, He J, Verano M, Brimmo AT, Glia A, Qasaimeh MA, et al. An integrated adipose-tissue-on-chip nanoplasmonic biosensing platform for investigating obesity-associated inflammation. Lab Chip. (2018) 18:3550–60. doi: 10.1039/c8lc00605a
61. Grist SM, Chrostowski L, Cheung KC. Optical oxygen sensors for applications in microfluidic cell culture. Sensors (Basel). (2010) 10:9286–316. doi: 10.3390/s101009286
62. Bonk SM, Stubbe M, Buehler SM, Tautorat C, Baumann W, Klinkenberg E-D, et al. Design and characterization of a sensorized microfluidic cell-culture system with electro-thermal micro-pumps and sensors for cell adhesion, oxygen, and pH on a glass chip. Biosensors (Basel). (2015) 5:513–36. doi: 10.3390/bios5030513
63. Rivera KR, Yokus MA, Erb PD, Pozdin VA, Daniele M. Measuring and regulating oxygen levels in microphysiological systems: design, material, and sensor considerations. Analyst. (2019) 144:3190–215. doi: 10.1039/c8an02201a
64. Marzioch J, Kieninger J, Weltin A, Flamm H, Aravindalochanan K, Sandvik JA, et al. On-chip photodynamic therapy - monitoring cell metabolism using electrochemical microsensors. Lab Chip. (2018) 18:3353–60. doi: 10.1039/c8lc00799c
65. Doryab A, Amoabediny G, Salehi-Najafabadi A. Advances in pulmonary therapy and drug development: Lung tissue engineering to lung-on-a-chip. Biotechnol Adv. (2016) 34:588–96. doi: 10.1016/j.bioteChadv.2016.02.006
66. Shrestha J, Razavi Bazaz S, Aboulkheyr Es H, Yaghobian Azari D, Thierry B, Ebrahimi Warkiani M, et al. Lung-on-a-chip: the future of respiratory disease models and pharmacological studies. Crit Rev Biotechnol. (2020) 40:213–30. doi: 10.1080/07388551.2019.1710458
67. Sisodia Y, Shah K, Ali Sayyed A, Jain M, Ali SA, Gondaliya P, et al. Lung-on-chip microdevices to foster pulmonary drug discovery. Biomater Sci. (2023) 11:777–90. doi: 10.1039/d2bm00951j
68. Moradi E, Jalili-Firoozinezhad S, Solati-Hashjin M. Microfluidic organ-on-a-chip models of human liver tissue. Acta Biomater. (2020) 116:67–83. doi: 10.1016/j.actbio.2020.08.041
69. Kim J, Lee C, Kim I, Ro J, Kim J, Min Y, et al. Three-dimensional human liver-chip emulating premetastatic niche formation by breast cancer-derived extracellular vesicles. ACS Nano. (2020) 14:14971–88. doi: 10.1021/acsnano.0c04778
70. Jellali R, Bricks T, Jacques S, Fleury M-J, Paullier P, Merlier F, et al. Long-term human primary hepatocyte cultures in a microfluidic liver biochip show maintenance of mRNA levels and higher drug metabolism compared with Petri cultures. Biopharm Drug Dispos. (2016) 37:264–75. doi: 10.1002/bdd.2010
71. Bhise NS, Manoharan V, Massa S, Tamayol A, Ghaderi M, Miscuglio M, et al. A liver-on-a-chip platform with bioprinted hepatic spheroids. Biofabrication. (2016) 8:14101. doi: 10.1088/1758-5090/8/1/014101
72. Zhang YS, Aleman J, Arneri A, Bersini S, Piraino F, Shin SR, et al. From cardiac tissue engineering to heart-on-a-chip: beating challenges. BioMed Mater. (2015) 10:34006. doi: 10.1088/1748-6041/10/3/034006
73. Ribas J, Sadeghi H, Manbachi A, Leijten J, Brinegar K, Zhang YS, et al. Cardiovascular organ-on-a-chip platforms for drug discovery and development. Appl In Vitro Toxicol. (2016) 2:82–96. doi: 10.1089/aivt.2016.0002
74. Wang G, McCain ML, Yang L, He A, Pasqualini FS, Agarwal A, et al. Modeling the mitochondrial cardiomyopathy of Barth syndrome with induced pluripotent stem cell and heart-on-chip technologies. Nat Med. (2014) 20:616–23. doi: 10.1038/nm.3545
75. Guo Y, Luo R, Wang Y, Deng P, Song T, Zhang M, et al. Corrigendum to “SARS-CoV-2 induced intestinal responses with a biomimetic human gut-on-chip” [Sci. Bull. (2021) 66 (8) 783–793. Sci Bull. (2021) 66:2144. doi: 10.1016/j.scib.2021.07.014
76. Ashammakhi N, Nasiri R, Barros NRD, Tebon P, Thakor J, Goudie M, et al. Gut-on-a-chip: Current progress and future opportunities. Biomaterials. (2020) 255:120196. doi: 10.1016/j.biomaterials.2020.120196
77. Puschhof J, Pleguezuelos-Manzano C, Clevers H. Organoids and organs-on-chips: Insights into human gut-microbe interactions. Cell Host Microbe. (2021) 29:867–78. doi: 10.1016/j.chom.2021.04.002
78. Lee J, Kim S. Kidney-on-a-chip: A new technology for predicting drug efficacy, interactions, and drug-induced nephrotoxicity. Curr Drug Metab. (2018) 19:577–83. doi: 10.2174/1389200219666180309101844
79. Ashammakhi N, Wesseling-Perry K, Hasan A, Elkhammas E, Zhang YS. Kidney-on-a-chip: untapped opportunities. Kidney Int. (2018) 94:1073–86. doi: 10.1016/j.kint.2018.06.034
80. Wang YI, Abaci HE, Shuler ML. Microfluidic blood-brain barrier model provides in vivo-like barrier properties for drug permeability screening. Biotechnol Bioeng. (2017) 114:184–94. doi: 10.1002/bit.26045
81. Park T-E, Mustafaoglu N, Herland A, Hasselkus R, Mannix R, FitzGerald EA, et al. Hypoxia-enhanced Blood-Brain Barrier Chip recapitulates human barrier function and shuttling of drugs and antibodies. Nat Commun. (2019) 10:2621. doi: 10.1038/s41467-019-10588-0
82. Bal-Öztürk A, Miccoli B, Avci-Adali M, Mogtader F, Sharifi F, Çeçen B, et al. Current strategies and future perspectives of skin-on-a-chip platforms: innovations, technical challenges and commercial outlook. Curr Pharm Des. (2018) 24:5437–57. doi: 10.2174/1381612825666190206195304
83. Mori N, Morimoto Y, Takeuchi S. Skin integrated with perfusable vascular channels on a chip. Biomaterials. (2017) 116:48–56. doi: 10.1016/j.biomaterials.2016.11.031
84. Chen Z, Kheiri S, Gevorkian A, Young EWK, Andre V, Deisenroth T, et al. Microfluidic arrays of dermal spheroids: a screening platform for active ingredients of skincare products. Lab Chip. (2021) 21:3952–62. doi: 10.1039/d1lc00619c
85. Liu X, Fang J, Huang S, Wu X, Xie X, Wang J, et al. Tumor-on-a-chip: from bioinspired design to biomedical application. Microsyst Nanoeng. (2021) 7:50. doi: 10.1038/s41378-021-00277-8
86. Sontheimer-Phelps A, Hassell BA, Ingber DE. Modelling cancer in microfluidic human organs-on-chips. Nat Rev Cancer. (2019) 19:65–81. doi: 10.1038/s41568-018-0104-6
87. Shang M, Soon RH, Lim CT, Khoo BL, Han J. Microfluidic modelling of the tumor microenvironment for anti-cancer drug development. Lab Chip. (2019) 19:369–86. doi: 10.1039/c8lc00970h
88. Wang H-F, Ran R, Liu Y, Hui Y, Zeng B, Chen D, et al. Tumor-vasculature-on-a-chip for investigating nanoparticle extravasation and tumor accumulation. ACS Nano. (2018) 12:11600–9. doi: 10.1021/acsnano.8b06846
89. Cecen B, Karavasili C, Nazir M, Bhusal A, Dogan E, Shahriyari F, et al. Multi-organs-on-chips for testing small-molecule drugs: challenges and perspectives. Pharmaceutics. (2021) 13:1657. doi: 10.3390/pharmaceutics13101657
90. Picollet-D’hahan N, Zuchowska A, Lemeunier I, Le Gac S. Multiorgan-on-a-chip: A systemic approach to model and decipher inter-organ communication. Trends Biotechnol. (2021) 39:788–810. doi: 10.1016/j.tibtech.2020.11.014
91. Sung JH, Wang YI, Narasimhan N Sriram, Jackson M, Long C, Hickman JJ, et al. Recent advances in body-on-a-chip systems. Anal Chem. (2019) 91:330–51. doi: 10.1021/acs.analchem.8b05293
92. Skardal A, Aleman J, Forsythe S, Rajan S, Murphy S, Devarasetty M, et al. Drug compound screening in single and integrated multi-organoid body-on-a-chip systems. Biofabrication. (2020) 12:025017. doi: 10.1088/1758-5090/ab6d36
93. Jalili-Firoozinezhad S, Miranda CC, Cabral JMS. Modeling the human body on microfluidic chips. Trends Biotechnol. (2021) 39:838–52. doi: 10.1016/j.tibtech.2021.01.004
94. Li ZA, Tuan RS. Towards establishing human body-on-a-chip systems. Stem Cell Res Ther. (2022) 13:431. doi: 10.1186/s13287-022-03130-5
95. Zou Z, Luo X, Chen Z, Zhang YS, Wen C. Emerging microfluidics-enabled platforms for osteoarthritis management: from benchtop to bedside. Theranostics. (2022) 12:891–909. doi: 10.7150/thno.62685
96. Rothbauer M, Byrne RA, Schobesberger S, Olmos Calvo I, Fischer A, Reihs EI, et al. Establishment of a human three-dimensional chip-based chondro-synovial coculture joint model for reciprocal cross talk studies in arthritis research. Lab Chip. (2021) 21:4128–43. doi: 10.1039/D1LC00130B
97. Oliveira IM, Carvalho MR, Fernandes DC, Abreu CM, Maia FR, Pereira H, et al. Modulation of inflammation by anti-TNF α mAb-dendrimer nanoparticles loaded in tyramine-modified gellan gum hydrogels in a cartilage-on-a-chip model. J Mater. Chem B. (2021) 9:4211–8. doi: 10.1039/d1tb00802a
98. Thompson CL, Hopkins T, Bevan C, Screen HRC, Wright KT, Knight MM. Human vascularised synovium-on-a-chip: a mechanically stimulated, microfluidic model to investigate synovial inflammation and monocyte recruitment. BioMed Mater. (2023) 18:065013. doi: 10.1088/1748-605X/acf976
99. Rosser J, Bachmann B, Jordan C, Ribitsch I, Haltmayer E, Gueltekin S, et al. Microfluidic nutrient gradient-based three-dimensional chondrocyte culture-on-a-chip as an in vitro equine arthritis model. Mater Today Bio. (2019) 4:100023. doi: 10.1016/j.mtbio.2019.100023
100. Ma H-P, Deng X, Chen D-Y, Zhu D, Tong J-L, Zhao T, et al. A microfluidic chip-based co-culture of fibroblast-like synoviocytes with osteoblasts and osteoclasts to test bone erosion and drug evaluation. R Soc Open Sci. (2018) 5:180528. doi: 10.1098/rsos.180528
101. Park I-S, Choi WH, Park DY, Park SR, Park S-H, Min B-H. Effect of joint mimicking loading system on zonal organization into tissue-engineered cartilage. PloS One. (2018) 13:e0202834. doi: 10.1371/journal.pone.0202834
102. Paggi CA, Venzac B, Karperien M, Leijten JCH, Le Gac S. Monolithic microfluidic platform for exerting gradients of compression on cell-laden hydrogels, and application to a model of the articular cartilage. Sensors Actuators B: Chem. (2020) 315:127917. doi: 10.1016/j.snb.2020.127917
103. Occhetta P, Mainardi A, Votta E, Vallmajo-Martin Q, Ehrbar M, Martin I, et al. Hyperphysiological compression of articular cartilage induces an osteoarthritic phenotype in a cartilage-on-a-chip model. Nat BioMed Eng. (2019) 3:545–57. doi: 10.1038/s41551-019-0406-3
104. Lin Z, Li Z, Li EN, Li X, Del Duke CJ, Shen H, et al. Osteochondral tissue chip derived from iPSCs: modeling OA pathologies and testing drugs. Front Bioeng Biotechnol. (2019) 7:411. doi: 10.3389/fbioe.2019.00411
105. Mondadori C, Visone R, Rasponi M, Redaelli A, Moretti M, Lopa S. Development of an organotypic microfluidic model to reproduce monocyte extravasation process in the osteoarthritic joint. Osteoarthritis Cartilage. (2018) 26:S122. doi: 10.1016/j.joca.2018.02.267
106. Li Z, Lin Z, Liu S, Yagi H, Zhang X, Yocum L, et al. Human mesenchymal stem cell-derived miniature joint system for disease modeling and drug testing. Advanced Sci. (2022) 9:2105909. doi: 10.1002/advs.202105909
107. Makarczyk MJ, Hines S, Yagi H, Li ZA, Aguglia AM, Zbikowski J, et al. Using microphysiological system for the development of treatments for joint inflammation and associated cartilage loss—A pilot study. Biomolecules. (2023) 13:384. doi: 10.3390/biom13020384
108. Paggi CA, Hendriks J, Karperien M, Le Gac S. Emulating the chondrocyte microenvironment using multi-directional mechanical stimulation in a cartilage-on-chip. Lab Chip. (2022) 22:1815–28. doi: 10.1039/D1LC01069G
109. Rothbauer M, Höll G, Eilenberger C, Kratz SRA, Farooq B, Schuller P, et al. Monitoring tissue-level remodelling during inflammatory arthritis using a three-dimensional synovium-on-a-chip with non-invasive light scattering biosensing. Lab Chip. (2020) 20:1461–71. doi: 10.1039/c9lc01097a
110. Goldring SR, Goldring MB. Changes in the osteochondral unit during osteoarthritis: structure, function and cartilage-bone crosstalk. Nat Rev Rheumatol. (2016) 12:632–44. doi: 10.1038/nrrheum.2016.148
111. Paggi CA, Teixeira LM, Le Gac S, Karperien M. Joint-on-chip platforms: entering a new era of in vitro models for arthritis. Nat Rev Rheumatol. (2022) 18:217–31. doi: 10.1038/s41584-021-00736-6
112. Takayanagi H. Osteoimmunology: shared mechanisms and crosstalk between the immune and bone systems. Nat Rev Immunol. (2007) 7:292–304. doi: 10.1038/nri2062
113. Shim J-H, Stavre Z, Gravallese EM. Bone loss in rheumatoid arthritis: basic mechanisms and clinical implications. Calcif Tissue Int. (2018) 102:533–46. doi: 10.1007/s00223-017-0373-1
114. Hu Y, Zhang H, Wang S, Cao L, Zhou F, Jing Y, et al. Bone/cartilage organoid on-chip: Construction strategy and application. Bioactive Materials. (2023) 25:29–41. doi: 10.1016/j.bioactmat.2023.01.016
115. Bartok B, Firestein GS. Fibroblast-like synoviocytes: key effector cells in rheumatoid arthritis. Immunol Rev. (2010) 233:233–55. doi: 10.1111/j.0105-2896.2009.00859.x
116. Komatsu N, Takayanagi H. Mechanisms of joint destruction in rheumatoid arthritis - immune cell-fibroblast-bone interactions. Nat Rev Rheumatol. (2022) 18:415–29. doi: 10.1038/s41584-022-00793-5
117. Nygaard G, Firestein GS. Restoring synovial homeostasis in rheumatoid arthritis by targeting fibroblast-like synoviocytes. Nat Rev Rheumatol. (2020) 16:316–33. doi: 10.1038/s41584-020-0413-5
118. Kim HJ, Huh D, Hamilton G, Ingber DE. Human gut-on-a-chip inhabited by microbial flora that experiences intestinal peristalsis-like motions and flow. Lab Chip. (2012) 12:2165–74. doi: 10.1039/c2lc40074j
119. Mondadori C, Palombella S, Salehi S, Talò G, Visone R, Rasponi M, et al. Recapitulating monocyte extravasation to the synovium in an organotypic microfluidic model of the articular joint. Biofabrication. (2021) 13. doi: 10.1088/1758-5090/ac0c5e
120. Krebs JC, Alapan Y, Dennstedt BA, Wera GD, Gurkan UA. Microfluidic processing of synovial fluid for cytological analysis. BioMed Microdevices. (2017) 19:20. doi: 10.1007/s10544-017-0163-6
121. Kraus SE, Lee E. Engineering approaches to investigate the roles of lymphatics vessels in rheumatoid arthritis. Microcirculation. (2023) 30:e12769. doi: 10.1111/micc.12769
122. Lokate AMC, Beusink JB, Besselink GAJ, Pruijn GJM, Schasfoort RBM. Biomolecular interaction monitoring of autoantibodies by scanning surface plasmon resonance microarray imaging. J Am Chem Soc. (2007) 129:14013–8. doi: 10.1021/ja075103x
123. van Beers JJ, Willemze A, Jansen JJ, Engbers GH, Salden M, Raats J, et al. ACPA fine-specificity profiles in early rheumatoid arthritis patients do not correlate with clinical features at baseline or with disease progression. Arthritis Res Ther. (2013) 15:R140. doi: 10.1186/ar4322
124. Corgier BP, Marquette CA, Blum LJ. Direct electrochemical addressing of immunoglobulins: Immuno-chip on screen-printed microarray. Biosensors Bioelectronics. (2007) 22:1522–6. doi: 10.1016/j.bios.2006.05.016
125. Yeom S-H, Han M-E, Kang B-H, Kim K-J, Yuan H, Eum N-S, et al. Enhancement of the sensitivity of LSPR-based CRP immunosensors by Au nanoparticle antibody conjugation. Sensors Actuators B: Chem. (2013) 177:376–83. doi: 10.1016/j.snb.2012.10.099
126. Kitayama Y, Takeuchi T. Localized surface plasmon resonance nanosensing of C-reactive protein with poly(2-methacryloyloxyethyl phosphorylcholine)-grafted gold nanoparticles prepared by surface-initiated atom transfer radical polymerization. Anal Chem. (2014) 86:5587–94. doi: 10.1021/ac501322x
127. Meyer MHF, Hartmann M, Keusgen M. SPR-based immunosensor for the CRP detection—A new method to detect a well known protein. Biosensors Bioelectronics. (2006) 21:1987–90. doi: 10.1016/j.bios.2005.09.010
128. Selvam SP, Chinnadayyala SR, Cho S. Electrochemical nanobiosensor for early detection of rheumatoid arthritis biomarker: Anti- cyclic citrullinated peptide antibodies based on polyaniline (PANI)/MoS2-modified screen-printed electrode with PANI-Au nanomatrix-based signal amplification. Sensors Actuators B: Chem. (2021) 333:129570. doi: 10.1016/j.snb.2021.129570
129. Guerrero S, Sánchez-Tirado E, Martínez-García G, González-Cortés A, Yáñez-Sedeño P, Pingarrón JM. Electrochemical biosensor for the simultaneous determination of rheumatoid factor and anti-cyclic citrullinated peptide antibodies in human serum. Analyst. (2020) 145:4680–7. doi: 10.1039/D0AN00481B
130. Lin C-Y, Nhat Nguyen UT, Hsieh H-Y, Tahara H, Chang Y-S, Wang B-Y, et al. Peptide-based electrochemical sensor with nanogold enhancement for detecting rheumatoid arthritis. Talanta. (2022) 236:122886. doi: 10.1016/j.talanta.2021.122886
131. Ram RK, Dutta N, Shukla J, Dutta G. Lab-On-Chip Electrochemical Biosensor for Rheumatoid Arthritis. In: Guha K, Dutta G, Biswas A, Srinivasa Rao K, editors. MEMS and Microfluidics in Healthcare, vol. 989 . Springer Nature Singapore, Singapore (2023). p. 157–81. doi: 10.1007/978-981-19-8714-4_8
132. de Gracia Villa M, Jiménez-Jorquera C, Haro I, Gomara MJ, Sanmartí R, Fernández-Sánchez C, et al. Carbon nanotube composite peptide-based biosensors as putative diagnostic tools for rheumatoid arthritis. Biosensors Bioelectronics. (2011) 27:113–8. doi: 10.1016/j.bios.2011.06.026
133. Zhao Y, Liu Y, Li X, Wang H, Zhang Y, Ma H, et al. Label-free ECL immunosensor for the early diagnosis of rheumatoid arthritis based on asymmetric heterogeneous polyaniline-gold nanomaterial. Sensors Actuators B: Chem. (2018) 257:354–61. doi: 10.1016/j.snb.2017.10.184
134. Dutta G. Next-Generation Nanobiosensor Devices for Point-Of-Care Diagnostics. Singapore: Springer Nature (2023). doi: 10.1007/978-981-19-7130-3
135. Young DA, Barter MJ, Soul J. Osteoarthritis year in review: genetics, genomics, epigenetics. Osteoarthritis Cartilage. (2022) 30:216–25. doi: 10.1016/j.joca.2021.11.004
136. Benam KH, Novak R, Nawroth J, Hirano-Kobayashi M, Ferrante TC, Choe Y, et al. Matched-comparative modeling of normal and diseased human airway responses using a microengineered breathing lung chip. Cell Syst. (2016) 3:456–466.e4. doi: 10.1016/j.cels.2016.10.003
137. Benam KH, Novak R, Ferrante TC, Choe Y, Ingber DE. Biomimetic smoking robot for in vitro inhalation exposure compatible with microfluidic organ chips. Nat Protoc. (2020) 15:183–206. doi: 10.1038/s41596-019-0230-y
138. Scherer HU, Burmester G-R. Adaptive immunity in rheumatic diseases: bystander or pathogenic player? Best Pract Res Clin Rheumatol. (2011) 25:785–800. doi: 10.1016/j.berh.2011.11.005
139. Kinashi Y, Hase K. Partners in leaky gut syndrome: intestinal dysbiosis and autoimmunity. Front Immunol. (2021) 12:673708. doi: 10.3389/fimmu.2021.673708
140. Chen B, Sun L, Zhang X. Integration of microbiome and epigenome to decipher the pathogenesis of autoimmune diseases. J Autoimmun. (2017) 83:31–42. doi: 10.1016/j.jaut.2017.03.009
141. Zhang X, Zhang D, Jia H, Feng Q, Wang D, Liang D, et al. The oral and gut microbiomes are perturbed in rheumatoid arthritis and partly normalized after treatment. Nat Med. (2015) 21:895–905. doi: 10.1038/nm.3914
142. Jalili-Firoozinezhad S, Gazzaniga FS, Calamari EL, Camacho DM, Fadel CW, Bein A, et al. A complex human gut microbiome cultured in an anaerobic intestine-on-a-chip. Nat BioMed Eng. (2019) 3:520–31. doi: 10.1038/s41551-019-0397-0
143. Gumuscu B, Albers HJ, van den Berg A, Eijkel JCT, van der Meer AD. Compartmentalized 3D tissue culture arrays under controlled microfluidic delivery. Sci Rep. (2017) 7:3381. doi: 10.1038/s41598-017-01944-5
144. Kim HJ, Li H, Collins JJ, Ingber DE. Contributions of microbiome and mechanical deformation to intestinal bacterial overgrowth and inflammation in a human gut-on-a-chip. Proc Natl Acad Sci U.S.A. (2016) 113:E7–15. doi: 10.1073/pnas.1522193112
145. Shin W, Kim HJ. Intestinal barrier dysfunction orchestrates the onset of inflammatory host-microbiome cross-talk in a human gut inflammation-on-a-chip. Proc Natl Acad Sci U.S.A. (2018) 115:E10539–47. doi: 10.1073/pnas.1810819115
146. Nelson MT, Charbonneau MR, Coia HG, Castillo MJ, Holt C, Greenwood ES, et al. Characterization of an engineered live bacterial therapeutic for the treatment of phenylketonuria in a human gut-on-a-chip. Nat Commun. (2021) 12:2805. doi: 10.1038/s41467-021-23072-5
147. Zhang M, Wang P, Luo R, Wang Y, Li Z, Guo Y, et al. Biomimetic human disease model of SARS-coV-2-induced lung injury and immune responses on organ chip system. Adv Sci (Weinh). (2021) 8:2002928. doi: 10.1002/advs.202002928
148. Beaurivage C, Naumovska E, Chang YX, Elstak ED, Nicolas A, Wouters H, et al. Development of a gut-on-A-chip model for high throughput disease modeling and drug discovery. Int J Mol Sci. (2019) 20:5661. doi: 10.3390/ijms20225661
149. Ma C, Peng Y, Li H, Chen W. Organ-on-a-chip: A new paradigm for drug development. Trends Pharmacol Sci. (2021) 42:119–33. doi: 10.1016/j.tips.2020.11.009
150. Lavé T, Parrott N, Grimm HP, Fleury A, Reddy M. Challenges and opportunities with modelling and simulation in drug discovery and drug development. Xenobiotica. (2007) 37:1295–310. doi: 10.1080/00498250701534885
151. Di L, Feng B, Goosen TC, Lai Y, Steyn SJ, Varma MV, et al. A perspective on the prediction of drug pharmacokinetics and disposition in drug research and development. Drug Metab Dispos. (2013) 41:1975–93. doi: 10.1124/dmd.113.054031
152. Pound P, Ritskes-Hoitinga M. Is it possible to overcome issues of external validity in preclinical animal research? Why most animal models are bound to fail. J Transl Med. (2018) 16:304. doi: 10.1186/s12967-018-1678-1
153. Tuntland T, Ethell B, Kosaka T, Blasco F, Zang RX, Jain M, et al. Implementation of pharmacokinetic and pharmacodynamic strategies in early research phases of drug discovery and development at Novartis Institute of Biomedical Research. Front Pharmacol. (2014) 5:174. doi: 10.3389/fphar.2014.00174
154. Ronaldson-Bouchard K, Teles D, Yeager K, Tavakol DN, Zhao Y, Chramiec A, et al. A multi-organ chip with matured tissue niches linked by vascular flow. Nat BioMed Eng. (2022) 6:351–71. doi: 10.1038/s41551-022-00882-6
155. Herland A, Maoz BM, Das D, Somayaji MR, Prantil-Baun R, Novak R, et al. Quantitative prediction of human pharmacokinetic responses to drugs via fluidically coupled vascularized organ chips. Nat BioMed Eng. (2020) 4:421–36. doi: 10.1038/s41551-019-0498-9
156. Ma C, Zhao L, Zhou E-M, Xu J, Shen S, Wang J. On-chip construction of liver lobule-like microtissue and its application for adverse drug reaction assay. Anal Chem. (2016) 88:1719–27. doi: 10.1021/acs.analchem.5b03869
157. Monteduro AG, Rizzato S, Caragnano G, Trapani A, Giannelli G, Maruccio G. Organs-on-chips technologies – A guide from disease models to opportunities for drug development. Biosensors Bioelectronics. (2023) 231:115271. doi: 10.1016/j.bios.2023.115271
158. Alberti M, Dancik Y, Sriram G, Wu B, Teo YL, Feng Z, et al. Multi-chamber microfluidic platform for high-precision skin permeation testing. Lab Chip. (2017) 17:1625–34. doi: 10.1039/c6lc01574c
159. Cidem A, Bradbury P, Traini D, Ong HX. Modifying and Integrating in vitro and ex vivo Respiratory Models for Inhalation Drug Screening. Front Bioeng Biotechnol. (2020) 8:581995. doi: 10.3389/fbioe.2020.581995
160. Borghardt JM, Kloft C, Sharma A. Inhaled therapy in respiratory disease: the complex interplay of pulmonary kinetic processes. Can Respir J. (2018) 2018:2732017. doi: 10.1155/2018/2732017
161. Kulthong K, Duivenvoorde L, Sun H, Confederat S, Wu J, Spenkelink B, et al. Microfluidic chip for culturing intestinal epithelial cell layers: Characterization and comparison of drug transport between dynamic and static models. Toxicol In Vitro. (2020) 65:104815. doi: 10.1016/j.tiv.2020.104815
162. Pocock K, Delon L, Bala V, Rao S, Priest C, Prestidge C, et al. Intestine-on-a-chip microfluidic model for efficient in vitro screening of oral chemotherapeutic uptake. ACS Biomater Sci Eng. (2017) 3:951–9. doi: 10.1021/acsbiomaterials.7b00023
163. Sobrino A, Phan DTT, Datta R, Wang X, Hachey SJ, Romero-López M, et al. 3D microtumors in vitro supported by perfused vascular networks. Sci Rep. (2016) 6:31589. doi: 10.1038/srep31589
164. Keuper-Navis M, Walles M, Poller B, Myszczyszyn A, van der Made TK, Donkers J, et al. The application of organ-on-chip models for the prediction of human pharmacokinetic profiles during drug development. Pharmacol Res. (2023) 195:106853. doi: 10.1016/j.phrs.2023.106853
165. Zhang Z, Nagrath S. Microfluidics and cancer: are we there yet? BioMed Microdevices. (2013) 15:595–609. doi: 10.1007/s10544-012-9734-8
166. Schuster B, Junkin M, Kashaf SS, Romero-Calvo I, Kirby K, Matthews J, et al. Automated microfluidic platform for dynamic and combinatorial drug screening of tumor organoids. Nat Commun. (2020) 11:5271. doi: 10.1038/s41467-020-19058-4
167. Zweers MC, de Boer TN, van Roon J, Bijlsma JWJ, Lafeber FPJG, Mastbergen SC. Celecoxib: considerations regarding its potential disease-modifying properties in osteoarthritis. Arthritis Res Ther. (2011) 13:239. doi: 10.1186/ar3437
168. Grodzinsky AJ, Wang Y, Kakar S, Vrahas MS, Evans CH. Intra-articular dexamethasone to inhibit the development of post-traumatic osteoarthritis. J Orthop Res. (2017) 35:406–11. doi: 10.1002/jor.23295
169. Braddock M, Quinn A. Targeting IL-1 in inflammatory disease: new opportunities for therapeutic intervention. Nat Rev Drug Discovery. (2004) 3:330–9. doi: 10.1038/nrd1342
170. Wu T-H, Tsai Y-C, Kuo F-C, Lee MS, Hu C-C, Lee G-B. A microfluidic platform for detection and quantification of two biomarkers for rheumatoid arthritis. Sensors Actuators B: Chem. (2023) 383:133587. doi: 10.1016/j.snb.2023.133587
171. Badillo-Mata JA, Camacho-Villegas TA, Lugo-Fabres PH. 3D cell culture as tools to characterize rheumatoid arthritis signaling and development of new treatments. Cells. (2022) 11:3410. doi: 10.3390/cells11213410
172. Wang N, He Y, Liu S, Makarcyzk MJ, Lei G, Chang A, et al. Engineering osteoarthritic cartilage model through differentiating senescent human mesenchymal stem cells for testing disease-modifying drugs. Sci China Life Sci. (2022) 65:309–27. doi: 10.1007/s11427-021-1933-7
173. Ingber DE. Human organs-on-chips for disease modelling, drug development and personalized medicine. Nat Rev Genet. (2022) 23:467–91. doi: 10.1038/s41576-022-00466-9
Keywords: rheumatoid arthritis, Organ-on-a-Chip, Synovial Joint-on-a-Chip, disease models, precision medicine, microphysiological systems
Citation: Zhang X, Su R, Wang H, Wu R, Fan Y, Bin Z, Gao C and Wang C (2024) The promise of Synovial Joint-on-a-Chip in rheumatoid arthritis. Front. Immunol. 15:1408501. doi: 10.3389/fimmu.2024.1408501
Received: 28 March 2024; Accepted: 26 August 2024;
Published: 11 September 2024.
Edited by:
Monica Neagu, Victor Babes National Institute of Pathology (INCDVB), RomaniaReviewed by:
Simona Neri, Rizzoli Orthopedic Institute (IRCCS), ItalyGorachand Dutta, Indian Institute of Technology Kharagpur, India
Copyright © 2024 Zhang, Su, Wang, Wu, Fan, Bin, Gao and Wang. This is an open-access article distributed under the terms of the Creative Commons Attribution License (CC BY). The use, distribution or reproduction in other forums is permitted, provided the original author(s) and the copyright owner(s) are credited and that the original publication in this journal is cited, in accordance with accepted academic practice. No use, distribution or reproduction is permitted which does not comply with these terms.
*Correspondence: Caihong Wang, snwch@sina.com