- 1Department of Biotherapy, Cancer Center, West China Hospital of Sichuan University, Chengdu, China
- 2West China School of Medicine, Sichuan University, Chengdu, China
- 3Department of Radiology, West China Hospital, Sichuan University, Chengdu, China
Background: Patients with resectable esophageal squamous cell carcinoma (ESCC) receiving neoadjuvant immunotherapy (NIT) display variable treatment responses. The purpose of this study is to establish and validate a radiomics based on enhanced computed tomography (CT) and combined with clinical data to predict the major pathological response to NIT in ESCC patients.
Methods: This retrospective study included 82 ESCC patients who were randomly divided into the training group (n = 57) and the validation group (n = 25). Radiomic features were derived from the tumor region in enhanced CT images obtained before treatment. After feature reduction and screening, radiomics was established. Logistic regression analysis was conducted to select clinical variables. The predictive model integrating radiomics and clinical data was constructed and presented as a nomogram. Area under curve (AUC) was applied to evaluate the predictive ability of the models, and decision curve analysis (DCA) and calibration curves were performed to test the application of the models.
Results: One clinical data (radiotherapy) and 10 radiomic features were identified and applied for the predictive model. The radiomics integrated with clinical data could achieve excellent predictive performance, with AUC values of 0.93 (95% CI 0.87–0.99) and 0.85 (95% CI 0.69–1.00) in the training group and the validation group, respectively. DCA and calibration curves demonstrated a good clinical feasibility and utility of this model.
Conclusion: Enhanced CT image-based radiomics could predict the response of ESCC patients to NIT with high accuracy and robustness. The developed predictive model offers a valuable tool for assessing treatment efficacy prior to initiating therapy, thus providing individualized treatment regimens for patients.
1 Introduction
Esophageal carcinoma (EC) is the sixth most common cause of cancer-related mortality and a crucial threat to global public health (1). In China, esophageal squamous cell carcinoma (ESCC) presents the dominant histological subtype accounting for 85.29% of all the ECs (2). Surgery remains the cornerstone of the treatment strategy for early-stage patients. Nevertheless, some of the patients present with locally advanced tumors at initial diagnosis due to insidious symptoms, and it is challenging to achieve R0 resection for such a population. Furthermore, the efficacy of surgery alone for locally advanced patients is quite limited, with a 5-year survival rate of 25% (3). Conventionally, neoadjuvant chemoradiotherapy or neoadjuvant chemotherapy has been recognized as the standard treatment for locally advanced patients. Although neoadjuvant chemoradiotherapy or neoadjuvant chemotherapy achieved a longer survival than surgery alone, the effects are not ideal enough owing to a low pathological response rate and local recurrence after surgery (4, 5). Hence, it is necessary to explore a novel and highly effective neoadjuvant therapy mode to maximize patient survival.
In recent years, immunotherapy has revolutionized the treatment landscape of most malignant tumors. By reactivating and enhancing the function of immune cells, immunotherapy could realize a precision attack on tumor cells and a durable immune response. Consequently, emerging trials have attempted to apply immunotherapy in the neoadjuvant setting. Neoadjuvant immunochemotherapy demonstrated satisfactory efficacy and manageable safety, with pathologic complete response rates of 16.7% to 50.0% (6). Furthermore, patients with ESCC who achieved major pathologic response (MPR) after neoadjuvant immunochemotherapy had significantly longer overall survival (91.4% vs. 47.7%) in the latest report (7). Despite this, part of the patients do not respond to neoadjuvant immunotherapy (NIT) and possibly bear high drug expenditure and the risk of immunotherapy-related adverse events (irAEs). Therefore, it is essential to predict the treatment response and identify the priority population for NIT to avoid unnecessary adverse events and costs. Many biomarkers have been used to judge the applicability of immunotherapy in ECs such as programmed death ligand 1 (PD-L1), CD8+ T infiltration, and tumor mutation burden (TMB) (8–10). Nonetheless, the predicting effect of these biomarkers has not been curtained in the NIT setting for ESCC. Furthermore, these biomarkers are usually obtained from a small proportion of tumor samples in an invasive, expensive, and time-consuming way, which could not reflect a comprehensive tumor information due to tumor heterogeneity (11, 12). Consequently, novel and noninvasive forecasting tools still need to be developed. Enhanced computed tomography (CT) plays an essential role in disease diagnosis and efficacy evaluation with convenience and rapid nature. However, due to the unique mechanism of immunotherapy, radiologic patterns of response are diverse and atypical, such as delayed response, pseudoprogression, hyperprogression, and mixed response, which confound the classical response evaluation based on the response evaluation criteria in solid tumors criteria (13–15). Hence, relying solely on enhanced CT to determine the response to immunotherapy is not precise or adequate. Currently, radiomics has become a critical technology in medical data mining by extracting abundant and multidimensional image features to facilitate the process of screening, diagnosis, and forecasting the treatment response and survival of cancer (16). Moreover, radiomics provides an underlying solution to the evaluation of intricate immune response and represents a pivotal role in immunotherapy imaging. Several studies have demonstrated reliable predicting capacity and feasibility of the treatment response of NIT in several tumors (17–20). However, there have been no studies using radiomics to evaluate the response of NIT. Therefore, this study aims to construct and validate a radiomics based on enhanced CT to preoperatively predict the therapeutic response after NIT in ESCC patients. Furthermore, this study integrated the clinicopathological data with radiomics into a multidimensional prediction system to assist the advancement of individual precision treatment.
2 Materials and methods
2.1 Patient selection
This study retrospectively selected ESCC patients who received immunotherapy in the neoadjuvant setting from January 2020 to October 2023 in West China Hospital, Sichuan University. The inclusion criteria were as follows: (i) pathologically confirmed, (ii) stage I–stage Iva, (iii) treated with immunotherapy before surgery, and (iv) available enhanced CT scan within 1 month prior to neoadjuvant therapy. Patients were excluded for the following reasons: (i) patients rejected surgery resulting in the absence of efficacy evaluation; (ii) neoadjuvant treatment regimens contained other drugs (such as targeted drugs) in addition to immunotherapy, chemotherapy, and radiotherapy; and (iii) critical clinicopathological data were missed. The flow chart of patient screening is shown in Figure 1. The study was approved by the Institutional Review Board of West China Hospital, Sichuan University (Approval number: 2024–0390). Informed consent from participants was waived, and patients’ details were hidden.
2.2 Treatments and response evaluation
All patients received a series of pretreatment workups, including lesion biopsy, disease evaluation, and related examinations. Patients were staged according to the 8th Edition of the American Joint Committee on Cancer TNM classification. Subsequently, patients received immunochemotherapy or with radiotherapy in the neoadjuvant setting. The specific strategy was enacted by the multidisciplinary team decision and patients’ willingness. Following the neoadjuvant therapy, a radical resection of tumors was performed. Based on the postoperative pathological result, treatment response was determined as pathologic complete response (defined as no residual tumor cells in both tumor tissue and lymph node), MPR (defined as residual tumor cells ≤10%), partial pathological response (defined as residual tumor cells >10%), or no treatment response (defined as abundant residual tumor cells). Then, patients with pathologic complete response or MPR were classified in the MPR group, while the rest were classified in the non-MPR group.
2.3 Imaging acquisition and feature extraction
CT scan was performed in West China Hospital, Sichuan University, within 1 month prior to the first treatment. The target CT images were exported from picture archiving and communication systems and reserved in Picture Archiving and Communication System in Digital Imaging and Communications in Medicine format. The 3D slicer software (version 5.40) was used to process the image data. The regions of interest in the image were segmented by two radiologists, with 5 years of working experience, who were blinded to the treatment response. The final result of image segmentation would be checked and corrected by a third radiologist with 10 years of working experience. Through 3D slicer software, the image features of regions of interest were derived. A total of 851 features (including four dimensions: shape feature, first-order statistics features, texture-based features, and high-order features) were packaged into R software (Version 4.1.1).
2.4 Model construction
We randomly divided the included patients by 7:3 ratio into the training group and the validation group. The clinical data were first evaluated with univariate logistic regression analysis to select the potential predictive factors. Then, multivariate logistic regression was performed to integrate and further determine the clinical prediction parameters. For the image part, zero mean normalization was used to normalize the image characteristic to reduce the variability of patients. Inter-class correlation coefficient (ICC) was used to test the reproducibility of data, and the feature with an ICC value of more than 0.8 indicated good consistency and could be further selected. Max-relevance and min-redundancy and least absolute shrinkage and selection operator (LASSO) regression were performed to reduce redundant features and select the most meaningful features for prediction. Then, a radiomics score (Rad-score) was calculated for each patient as a linear combination of selected features that were weighted by their respective coefficients. The receiver operating characteristic curve (ROC) to estimate the ability of the Rad-score was plotted, and the area under the curve (AUC) was calculated concurrently. Then, the radiomics model, clinical model, and the combined model integrating image and clinical data were established and verified using the data from the validation group. Additionally, an optimal predictive model would be determined and presented as a nomogram. Decision curve analysis was performed to test clinical utility, and calibration curves were applied to evaluate the agreement between prediction and clinical practice with the Hosmer–Lemeshow test.
2.5 Statistics analysis
The data analysis was handled in SPSS software and R language. Categorical variables, expressed as counts and percentages, were compared using the chi-square test or Fisher’s exact test, where applicable. LASSO regression analysis was completed using the “glmnet” package of R software, while ROC curve analysis was performed in the R software with the “survive ROC” package. Odds ratio (OR) and 95% confidence interval (CI) were used to describe the risk of clinical data. A two-tailed p-value less than 0.05 was recognized as significant for univariable and multivariable analysis.
3 Results
3.1 Clinical characteristics
A total of 82 patients met the inclusion and exclusion criteria and were finally included in the analysis. We randomly assigned all patients into the training group (n = 57) and the validation group (n = 25) by a 7:3 ratio. There was no significant characteristic difference between the training group and the validation group (Supplementary Table 1). The characteristics of all patients are shown in Table 1. For the most part, participants with potentially resectable ESCC were in the II–III stages, with more than half of the patients receiving radiotherapy. The vast majority of patients were treated with two cycles of NIT, while others prolonged the treatment cycle. All patients accepted standard surgical procedures and were evaluated for treatment response of NIT, except for two patients who discarded surgery due to disease progression judged by image. Overall, the rate of MRP was 56.1% (46/82).
3.2 Feature selection
A total of 851 features of tumor volume of interest were extracted. After selecting the features of ICC more than 0.8, a sum of 626 features was further analyzed in LASSO regression to discard the redundant features (Figures 2A, B). Eventually, 10 optimal features were selected to establish radiomics and nomogram. Then, a fitting formula was applied to calculate the linear association of selected features. In the radiomics model, the rad score of the MPR group was higher than that of the non-MPR group in the training cohort (Figure 2C). A similar result was also found in the validation cohort.
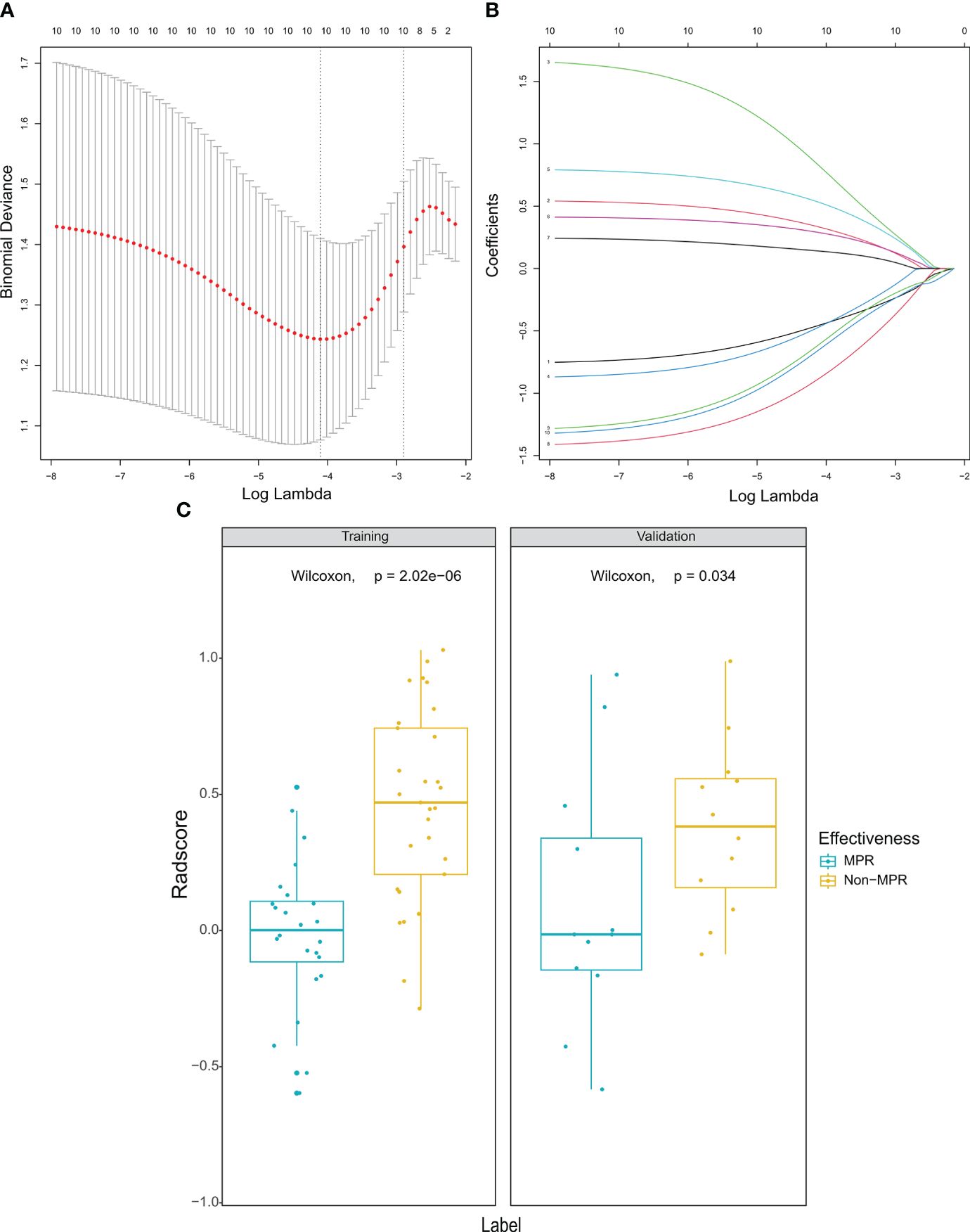
Figure 2 Selection of radiomic features and comparison of radiomics score. (A) Selection of the regulation weight parameter (λ) for the least absolute shrinkage and selection operator. (B) coefficient curves for 10 radiomic features. (C) There were significant differences in the radiomics score between the MPR group and non-MPR group in both training cohort and validation cohort. MPR, major pathological response.
3.3 Model construction
Univariable analysis was performed to initially identify the independent factors (Table 2). Multivariable analysis showed that radiotherapy was associated with a higher MPR, and eventually, it was included in the model construction. Then, three predictive models were established, namely radiomics model, clinical model, and radiomics-clinical model. The predictive ability of the three models is presented in the ROC curve (Figure 3). The AUC values of the clinical model and the radiomics model in the training cohort were 0.77 (95% CI 0.66–0.88) and 0.87 (95% CI 0.78–0.96), respectively. In the validation setting, the clinical model and the radiomics model had an AUC value of 0.68 (95% CI 0.49–0.86) and 0.75 (95% CI 0.54–0.96). The radiomics–clinical model had the most excellent performance both in the training cohort and validation cohort, with the AUC values of 0.94 (95% CI 0.89–1.00) and 0.77 (95% CI 0.58–0.96), respectively.
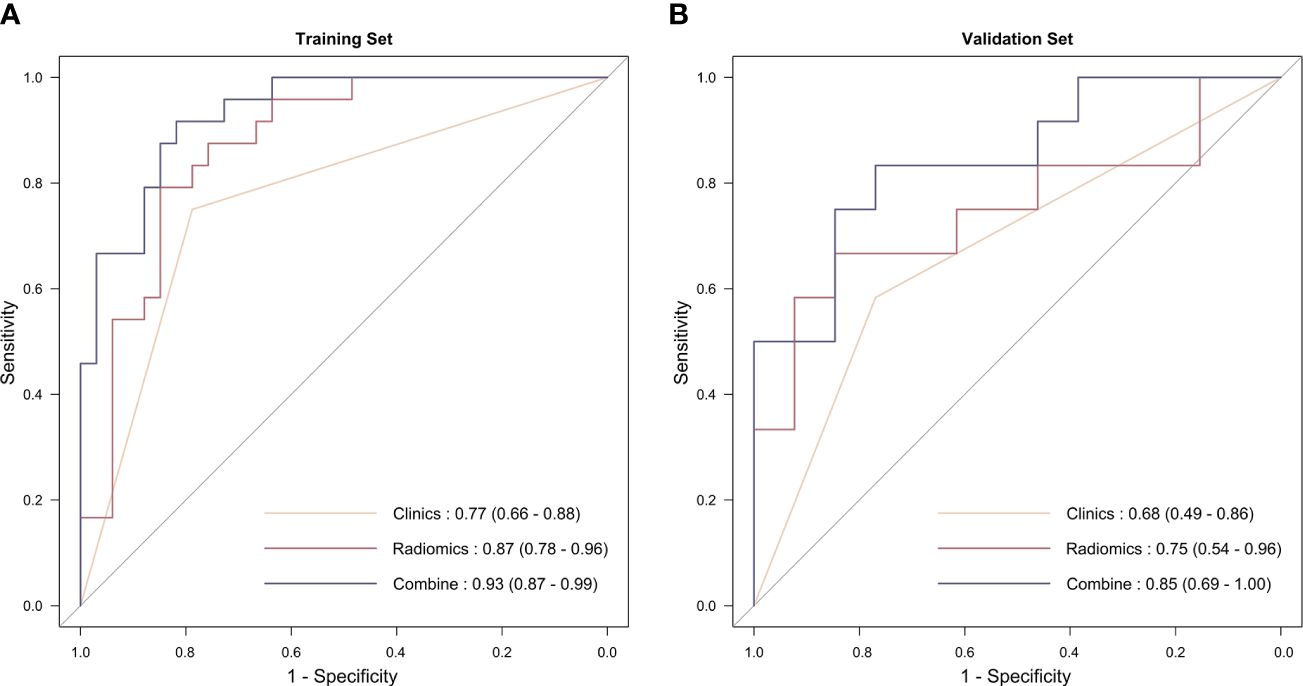
Figure 3 The receiver operating characteristic curve of the three models. (A) In the training group; (B) in the validation group.
3.4 Nomogram construction
The combined model incorporating Rad-score and radiotherapy was established and presented with a nomogram (Figure 4A). The calibration curve demonstrated that probability of treatment response had a good agreement between nomogram-evaluated and actual response (Figure 4B, C). The Hosmer–Lemeshow test in calibration curves yielded a statistically insignificant p-value of 0.932 for the training group and 0.581 for the validation group suggesting that the nomogram worked with a good fit. The decision curve analysis for the nomogram is shown in Figure 4D. The decision curve demonstrated that the performance of the three models was at least equivalent to a strategy of treating all patients or treating none. Furthermore, regardless of the risk threshold, utilizing the combined model for predicting MPR resulted in a greater net benefit compared to the other two models.
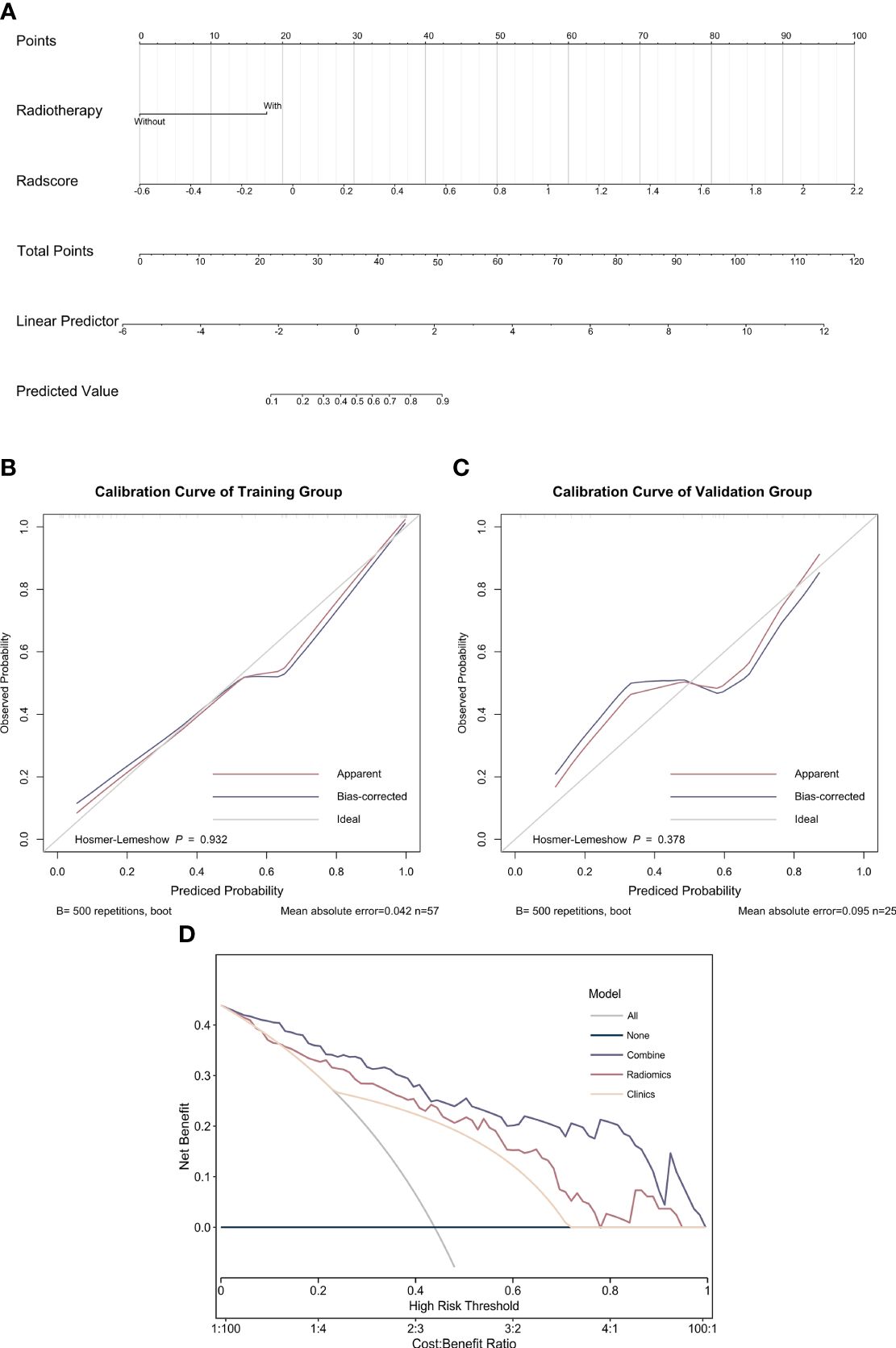
Figure 4 Nomogram, calibration curve, and decision curve analysis of the combined model. (A) Nomogram of the combined model; (B) calibration curve for the major pathological response in the training group; (C) calibration curve for the major pathological response in the validation group; (D) decision curve analysis of the three models.
4 Discussion
This present study constructed and validated a nomogram to predict the MPR of NIT in ESCC patients. One clinical factor and 10 image features were incorporated into this predictive model, and the model demonstrated excellent predictive accuracy, with the AUC value of 0.93 in the training cohort and 0.95 in the validation cohort. This convenient tool could serve in a pretreatment setting and provide a reference for clinical decision making.
Presently, NIT demonstrates promising efficacy in ESCC, and emerging clinical trials are ongoing to explore the wider application of NIT. Despite this, the treatment effect is varied, and some of the patients bear the risk of irAEs. Therefore, seeking novel biomarkers to forecast treatment response is reasonable and urgent especially in the era of immunotherapy-predominant treatment. Theoretically, PD-L1 expression is a powerful marker to predict the effect of immunotherapy; however, there is still a controversy about whether PD-L1 could serve as a predictor to identify those ESCC patients who would benefit from immunotherapy. Many trials have revealed that patients could benefit from immunotherapy combined with chemotherapy regardless of the expression level of PD-L1 (21, 22). Additionally, several studies verified that there were no significant differences in PD-L1 expression between the pathological response and the non-response group (23, 24). Similarly, TMB is a debatable predictor in the NIT setting, as some studies verified its predictive role (24, 25), while some trials displayed no correlation between response and TMB (26). In addition, studies focus on the change of components from tumor microenvironment. M2-like macrophages (27), tumor-infiltrating CD8+ T cells (28, 29), and chemokines (30) were investigated, but their predictive roles lack evidence. To date, reliable predictive biomarkers have not been determined.
Radiomics, a novel strategy that extracts quantitative features from images and converts these features into mineable data, has an extensive application in the medical field. Importantly, radiomics could recognize subtle differences reflecting the microenvironment and genomic heterogeneity, which are critical for treatment response, especially for newly treated cancer patients. In a retrospective analysis, including lung cancer and melanoma, specific texture and shape features were closely related to treatment response and survival. Concretely, response rate was higher in those tumor images showing heterogeneous morphological profiles, uneven density, and compact borders (31). In addition, radiomics could indirectly build up a link with treatment response by capturing gene phenotypes and established biomarkers (32, 33). For ESCC, radiomics has demonstrated good predictive ability in treatment response and prognosis, with AUCs of 0.68–0.86 (34–38). The application of radiomics in the neoadjuvant setting might be reasonable and accurate, since other treatment phases may give rise to a controversy about the optimal imaging time considering altered tumor heterogeneity due to treatment (39). In a meta-analysis integrating 16 studies, the median AUC was 0.84 (0.81–0.87) to predict neoadjuvant chemoradiotherapy for EC patients suggesting the feasibility of radiomics (40).To our knowledge, this is the first radiomics for ESCC patients treated with NIT, with similar predictive effectiveness with other cancers (17, 18). Such a predictive tool could have an impact on the early identification of non-responders so that patients seek alternative treatment and save cost. Certainly, to promote clinical translation of radiomics, standardized image acquisition, normalized data processing and analysis, and large sample size from multi-centers are indispensable. In addition, the combination of genomics, proteomics, metabolomics, or other omics with radiomics further enhances robust and comprehensive predictive ability providing detailed information for decision making and precision medicine (41, 42).
In this present study, the overall MPR rate was 56.1% consistent with the results of most trials (43) suggesting that NIT was a promising way for ESCC. In addition, we found that radiotherapy was associated with a higher MPR. Presently, quite a few studies explore the utility of radiotherapy and find that radiotherapy might be associated with a higher response rate, which was consistent with our study (43, 44). In the meta-analysis, Wang et al. summarized the efficacy of NIT for EC patients and revealed that patients treated with neoadjuvant immunochemotherapy plus radiotherapy developed a higher MPR rate than those with neoadjuvant immunochemotherapy (39.8% vs. 88.8%) (44). In addition to its own killing ability, radiotherapy might have a synergistic effect on immune response through the following mechanisms (1): escalating the expression of PD-L1 or other neoantigens (2), inducing immunogenic cell deaths and increasing the release of abundant cytokines and chemokines recruiting immune cells to the tumor microenvironment, and (3) increasing the neoantigen presentation and accelerating the identification of cytotoxic T lymphocytes (45–47). Yet, the implementation of radiotherapy did not demonstrate an extra benefit in all clinical trials, and the coordination of the two regimens is required to be optimized (47).
Although this study constructed a predictive model with promising performance, there were several limitations. First, this was a retrospective study using the data from a single center of the Chinese population, which inevitably introduced the bias and confounding factors and limited the generality of the predictive model. Second, the predictive model was constructed with a relatively small sample size and lacked external validation, which could limit the robustness and wider applicability of the predictive model. Therefore, research with a multi-center, prospective setting on a large scale is required to further verify the feasibility of the predictive model and address these limitations. In addition, adhering to a uniform protocol for image acquisition is also necessary to ensure the reproducibility of radiomics. Finally, this predictive model utilized image data, and potential factors correlated with treatment response were not integrated. Multi-omics involving genomic characteristics, hematological data, and proteomics should be attempted in future studies to obtain an optimal immunotherapy predictive model.
5 Conclusion
In summary, this study integrated image features of tumor volume and clinical data of resectable ESCC patients to construct a nomogram to predict the treatment response of NIT. This nomogram model could forecast MPR before treatment with high accuracy and robustness, which help guide individualized therapy for patients and reduce the unnecessary risk of irAEs.
Data availability statement
The raw data supporting the conclusions of this article will be made available by the authors, without undue reservation.
Ethics statement
The studies involving humans were approved by Institutional Review Board of West China Hospital, Sichuan University. The studies were conducted in accordance with the local legislation and institutional requirements. The ethics committee/institutional review board waived the requirement of written informed consent for participation from the participants or the participants’ legal guardians/next of kin because this was a retrospective analysis and all patients’ details were hidden.
Author contributions
J-LW: Conceptualization, Data curation, Formal analysis, Writing – original draft. L-ST: Conceptualization, Formal analysis, Investigation, Writing – original draft. XZ: Methodology, Resources, Software, Writing – original draft. YW: Formal analysis, Methodology, Software, Writing – original draft. Y-JF: Formal analysis, Project administration, Resources, Software, Writing – original draft. YZ: Conceptualization, Methodology, Project administration, Supervision, Validation, Writing – review & editing. J-YL: Conceptualization, Project administration, Supervision, Writing – review & editing.
Funding
The author(s) declare that no financial support was received for the research, authorship, and/or publication of this article.
Acknowledgments
This research acknowledges the help of radiologists in identifying the area of interest and obtaining the CT images. The content of this manuscript was not presented as a preprint or thesis.
Conflict of interest
The authors declare that the research was conducted in the absence of any commercial or financial relationships that could be construed as a potential conflict of interest.
Publisher’s note
All claims expressed in this article are solely those of the authors and do not necessarily represent those of their affiliated organizations, or those of the publisher, the editors and the reviewers. Any product that may be evaluated in this article, or claim that may be made by its manufacturer, is not guaranteed or endorsed by the publisher.
Supplementary material
The Supplementary Material for this article can be found online at: https://www.frontiersin.org/articles/10.3389/fimmu.2024.1405146/full#supplementary-material
References
1. Sung H, Ferlay J, Siegel RL, Laversanne M, Soerjomataram I, Jemal A, et al. Global cancer statistics 2020: GLOBOCAN estimates of incidence and mortality worldwide for 36 cancers in 185 countries. CA Cancer J Clin. (2021) 71:209–49. doi: 10.3322/caac.21660
2. He Y, Liang D, Du L, Guo T, Liu Y, Sun X, et al. Clinical characteristics and survival of 5283 esophageal cancer patients: A multicenter study from eighteen hospitals across six regions in China. Cancer Commun (Lond). (2020) 40:531–44. doi: 10.1002/cac2.12087
3. Herskovic A, Russell W, Liptay M, Fidler MJ, Al-Sarraf M. Esophageal carcinoma advances in treatment results for locally advanced disease: review. Ann Oncol. (2012) 23:1095–103. doi: 10.1093/annonc/mdr433
4. van Hagen P, Hulshof MC, van Lanschot JJ, Steyerberg EW, van Berge Henegouwen MI, Wijnhoven BP, et al. Preoperative chemoradiotherapy for esophageal or junctional cancer. N Engl J Med. (2012) 366:2074–84. doi: 10.1056/NEJMoa1112088
5. Yang H, Liu H, Chen Y, Zhu C, Fang W, Yu Z, et al. Neoadjuvant chemoradiotherapy followed by surgery versus surgery alone for locally advanced squamous cell carcinoma of the esophagus (NEOCRTEC5010): A phase III multicenter, randomized, open-label clinical trial. J Clin Oncol. (2018) 36:2796–803. doi: 10.1200/JCO.2018.79.1483
6. Li Q, Liu T, Ding Z. Neoadjuvant immunotherapy for resectable esophageal cancer: A review. Front Immunol. (2022) 13:1051841. doi: 10.3389/fimmu.2022.1051841
7. Yang Y, Liu J, Liu Z, Zhu L, Chen H, Yu B, et al. Two-year outcomes of clinical N2–3 esophageal squamous cell carcinoma after neoadjuvant chemotherapy and immunotherapy from the phase 2 NICE study. J Thorac Cardiovasc surgery. (2024) 167:838–47.e1. doi: 10.1016/j.jtcvs.2023.08.056
8. Kojima T, Shah MA, Muro K, Francois E, Adenis A, Hsu CH, et al. Randomized phase III KEYNOTE-181 study of pembrolizumab versus chemotherapy in advanced esophageal cancer. J Clin Oncol. (2020) 38:4138–48. doi: 10.1200/JCO.20.01888
9. Wu H, Leng X, Liu Q, Mao T, Jiang T, Liu Y, et al. Intratumoral microbiota composition regulates chemoimmunotherapy response in esophageal squamous cell carcinoma. Cancer Res. (2023) 83:3131–44. doi: 10.1158/0008-5472.CAN-22-2593
10. Cui Y, Chen H, Xi R, Cui H, Zhao Y, Xu E, et al. Whole-genome sequencing of 508 patients identifies key molecular features associated with poor prognosis in esophageal squamous cell carcinoma. Cell Res. (2020) 30:902–13. doi: 10.1038/s41422-020-0333-6
11. Ilie M, Long-Mira E, Bence C, Butori C, Lassalle S, Bouhlel L, et al. Comparative study of the PD-L1 status between surgically resected specimens and matched biopsies of NSCLC patients reveal major discordances: a potential issue for anti-PD-L1 therapeutic strategies. Ann Oncol. (2016) 27:147–53. doi: 10.1093/annonc/mdv489
12. McLaughlin J, Han G, Schalper KA, Carvajal-Hausdorf D, Pelekanou V, Rehman J, et al. Quantitative assessment of the heterogeneity of PD-L1 expression in non-small-cell lung cancer. JAMA Oncol. (2016) 2:46–54. doi: 10.1001/jamaoncol.2015.3638
13. Chiou VL, Burotto M. Pseudoprogression and immune-related response in solid tumors. J Clin Oncol. (2015) 33:3541–3. doi: 10.1200/JCO.2015.61.6870
14. Dercle L, Sun S, Seban R-D, Mekki A, Sun R, Tselikas L, et al. Emerging and evolving concepts in cancer immunotherapy imaging. Radiology (2023) 306(1):32–46. doi: 10.1148/radiol.210518
15. Huang Q, Liu Z, Yu Y, Rong Z, Wang P, Wang S, et al. Prediction of response to neoadjuvant chemo-immunotherapy in patients with esophageal squamous cell carcinoma by a rapid breath test. Br J cancer. (2024) 130:694–700. doi: 10.1038/s41416-023-02547-w
16. Lambin P, Rios-Velazquez E, Leijenaar R, Carvalho S, van Stiphout RG, Granton P, et al. Radiomics: extracting more information from medical images using advanced feature analysis. Eur J Cancer. (2012) 48:441–6. doi: 10.1016/j.ejca.2011.11.036
17. Liu C, Zhao W, Xie J, Lin H, Hu X, Li C, et al. Development and validation of a radiomics-based nomogram for predicting a major pathological response to neoadjuvant immunochemotherapy for patients with potentially resectable non-small cell lung cancer. Front Immunol. (2023) 14:1115291. doi: 10.3389/fimmu.2023.1115291
18. van der Hulst HJ, Vos JL, Tissier R, Smit LA, Martens RM, Beets-Tan RGH, et al. Quantitative diffusion-weighted imaging analyses to predict response to neoadjuvant immunotherapy in patients with locally advanced head and neck carcinoma. Cancers. (2022) 14:6235. doi: 10.3390/cancers14246235
19. Seban RD, Arnaud E, Loirat D, Cabel L, Cottu P, Djerroudi L, et al. [18F]FDG PET/CT for predicting triple-negative breast cancer outcomes after neoadjuvant chemotherapy with or without pembrolizumab. Eur J Nucl Med Mol Imaging. (2023) 50:4024–35. doi: 10.1007/s00259-023-06394-y
20. Vos JL, Zuur CL, Smit LA, de Boer JP, Al-Mamgani A, van den Brekel MWM, et al. [(18)F]FDG-PET accurately identifies pathological response early upon neoadjuvant immune checkpoint blockade in head and neck squamous cell carcinoma. Eur J Nucl Med Mol Imaging. (2022) 49:2010–22. doi: 10.1007/s00259-021-05610-x
21. Huang J, Xu B, Mo H, Zhang W, Chen X, Wu D, et al. Safety, activity, and biomarkers of SHR-1210, an anti-PD-1 antibody, for patients with advanced esophageal carcinoma. Clin Cancer research: an Off J Am Assoc Cancer Res. (2018) 24:1296–304. doi: 10.1158/1078-0432.CCR-17-2439
22. Sun JM, Shen L, Shah MA, Enzinger P, Adenis A, Doi T, et al. Pembrolizumab plus chemotherapy versus chemotherapy alone for first-line treatment of advanced oesophageal cancer (KEYNOTE-590): a randomised, placebo-controlled, phase 3 study. Lancet (London England). (2021) 398:759–71. doi: 10.1016/S0140-6736(21)01234-4
23. Yan X, Duan H, Ni Y, Zhou Y, Wang X, Qi H, et al. Tislelizumab combined with chemotherapy as neoadjuvant therapy for surgically resectable esophageal cancer: A prospective, single-arm, phase II study (TD-NICE). Int J Surg. (2022) 103:106680. doi: 10.1016/j.ijsu.2022.106680
24. Chen X, Xu X, Wang D, Liu J, Sun J, Lu M, et al. Neoadjuvant sintilimab and chemotherapy in patients with potentially resectable esophageal squamous cell carcinoma (KEEP-G 03): an open-label, single-arm, phase 2 trial. J Immunother Cancer. (2023) 11:e005830. doi: 10.1136/jitc-2022-005830
25. Forde PM, Chaft JE, Smith KN, Anagnostou V, Cottrell TR, Hellmann MD, et al. Neoadjuvant PD-1 blockade in resectable lung cancer. New Engl J Med. (2018) 378:1976–86. doi: 10.1056/NEJMoa1716078
26. Chaft JE, Oezkan F, Kris MG, Bunn PA, Wistuba II, DJ K, et al. Neoadjuvant atezolizumab for resectable non-small cell lung cancer: an open-label, single-arm phase II trial. Nat Med. (2022) 28:2155–61. doi: 10.1038/s41591-022-01962-5
27. Yang W, Xing X, Yeung SJ, Wang S, Chen W, Bao Y, et al. Neoadjuvant programmed cell death 1 blockade combined with chemotherapy for resectable esophageal squamous cell carcinoma. J Immunother Cancer. (2022) 10:e003497. doi: 10.1136/jitc-2021-003497
28. Liu Z, Zhang Y, Ma N, Yang Y, Ma Y, Wang F, et al. Progenitor-like exhausted SPRY1(+)CD8(+) T cells potentiate responsiveness to neoadjuvant PD-1 blockade in esophageal squamous cell carcinoma. Cancer Cell. (2023) 41:1852–70.e9. doi: 10.1016/j.ccell.2023.09.011
29. Ma N, Hua R, Yang Y, Liu ZC, Pan J, Yu BY, et al. PES1 reduces CD8(+) T cell infiltration and immunotherapy sensitivity via interrupting ILF3-IL15 complex in esophageal squamous cell carcinoma. J Biomed science. (2023) 30:20. doi: 10.1186/s12929-023-00912-8
30. Han D, Han Y, Guo W, Wei W, Yang S, Xiang J, et al. High-dimensional single-cell proteomics analysis of esophageal squamous cell carcinoma reveals dynamic alterations of the tumor immune microenvironment after neoadjuvant therapy. J Immunother Cancer. (2023) 11:e007847. doi: 10.1136/jitc-2023-007847
31. Trebeschi S, Drago SG, Birkbak NJ, Kurilova I, C,ril AM, Delli Pizzi A, et al. Predicting response to cancer immunotherapy using noninvasive radiomic biomarkers. Ann oncology: Off J Eur Soc Med Oncol. (2019) 30:998–1004. doi: 10.1093/annonc/mdz108
32. Gevaert O, Xu J, Hoang CD, Leung AN, Xu Y, Quon A, et al. Non-small cell lung cancer: identifying prognostic imaging biomarkers by leveraging public gene expression microarray data–methods and preliminary results. Radiology. (2012) 264:387–96. doi: 10.1148/radiol.12111607
33. Rizzo S, Petrella F, Buscarino V, De Maria F, Raimondi S, Barberis M, et al. CT radiogenomic characterization of EGFR, K-RAS, and ALK mutations in non-small cell lung cancer. Eur Radiol. (2016) 26:32–42. doi: 10.1007/s00330-015-3814-0
34. Jin X, Zheng X, Chen D, Jin J, Zhu G, Deng X, et al. Prediction of response after chemoradiation for esophageal cancer using a combination of dosimetry and CT radiomics. Eur Radiol. (2019) 29:6080–8. doi: 10.1007/s00330-019-06193-w
35. Yang Z, He B, Zhuang X, Gao X, Wang D, Li M, et al. CT-based radiomic signatures for prediction of pathologic complete response in esophageal squamous cell carcinoma after neoadjuvant chemoradiotherapy. J Radiat Res. (2019) 60:538–45. doi: 10.1093/jrr/rrz027
36. Xie C, Yang P, Zhang X, Xu L, Wang X, Li X, et al. Sub-region based radiomics analysis for survival prediction in oesophageal tumours treated by definitive concurrent chemoradiotherapy. EBioMedicine. (2019) 44:289–97. doi: 10.1016/j.ebiom.2019.05.023
37. Qiu Q, Duan J, Deng H, Han Z, Gu J, Yue NJ, et al. Development and validation of a radiomics nomogram model for predicting postoperative recurrence in patients with esophageal squamous cell cancer who achieved pCR after neoadjuvant chemoradiotherapy followed by surgery. Front Oncol. (2020) 10:1398. doi: 10.3389/fonc.2020.01398
38. Cui J, Li L, Liu N, Hou W, Dong Y, Yang F, et al. Model integrating CT-based radiomics and genomics for survival prediction in esophageal cancer patients receiving definitive chemoradiotherapy. biomark Res. (2023) 11:44. doi: 10.1186/s40364-023-00480-x
39. Rao SX, Lambregts DM, Schnerr RS, Beckers RC, Maas M, Albarello F, et al. CT texture analysis in colorectal liver metastases: A better way than size and volume measurements to assess response to chemotherapy? United Eur Gastroenterol J. (2016) 4:257–63. doi: 10.1177/2050640615601603
40. Yang Z, Gong J, Li J, Sun H, Pan Y, Zhao L. The gap before real clinical application of imaging-based machine-learning and radiomic models for chemoradiation outcome prediction in esophageal cancer: a systematic review and meta-analysis. Int J Surg. (2023) 109:2451–66. doi: 10.1097/JS9.0000000000000441
41. Wang HH, Steffens EN, Kats-Ugurlu G, van Etten B, Burgerhof JGM, Hospers GAP, et al. Potential predictive immune and metabolic biomarkers of tumor microenvironment regarding pathological and clinical response in esophageal cancer after neoadjuvant chemoradiotherapy: A systematic review. Ann Surg Oncol. (2024) 31:433–51. doi: 10.1245/s10434-023-14352-z
42. Wu B, Fu L, Guo X, Hu H, Li Y, Shi Y, et al. Multi-omics profiling and digital image analysis reveal the potential prognostic and immunotherapeutic properties of CD93 in stomach adenocarcinoma. Front Immunol. (2023) 14:984816. doi: 10.3389/fimmu.2023.984816
43. Liu Y, Bao Y, Yang X, Sun S, Yuan M, Ma Z, et al. Efficacy and safety of neoadjuvant immunotherapy combined with chemoradiotherapy or chemotherapy in esophageal cancer: A systematic review and meta-analysis. Front Immunol. (2023) 14:1117448. doi: 10.3389/fimmu.2023.1117448
44. Wang Z, Shao C, Wang Y, Duan H, Pan M, Zhao J, et al. Efficacy and safety of neoadjuvant immunotherapy in surgically resectable esophageal cancer: A systematic review and meta-analysis. Int J Surg. (2022) 104:106767. doi: 10.1016/j.ijsu.2022.106767
45. Vanpouille-Box C, Alard A, Aryankalayil MJ, Sarfraz Y, Diamond JM, Schneider RJ, et al. DNA exonuclease Trex1 regulates radiotherapy-induced tumour immunogenicity. Nat Commun. (2017) 8:15618. doi: 10.1038/ncomms15618
46. Deng L, Liang H, Burnette B, Beckett M, Darga T, Weichselbaum RR, et al. Irradiation and anti-PD-L1 treatment synergistically promote antitumor immunity in mice. J Clin Invest. (2014) 124:687–95. doi: 10.1172/JCI67313
Keywords: neoadjuvant immunotherapy, esophageal squamous cell cancer, major pathological response, radiomics, computed tomography
Citation: Wang J-L, Tang L-S, Zhong X, Wang Y, Feng Y-J, Zhang Y and Liu J-Y (2024) A machine learning radiomics based on enhanced computed tomography to predict neoadjuvant immunotherapy for resectable esophageal squamous cell carcinoma. Front. Immunol. 15:1405146. doi: 10.3389/fimmu.2024.1405146
Received: 22 March 2024; Accepted: 29 May 2024;
Published: 14 June 2024.
Edited by:
Hailin Tang, Sun Yat-sen University Cancer Center (SYSUCC), ChinaReviewed by:
Leilei Wu, Tongji University, ChinaAshuai Du, Guizhou Provincial People’s Hospital, China
Copyright © 2024 Wang, Tang, Zhong, Wang, Feng, Zhang and Liu. This is an open-access article distributed under the terms of the Creative Commons Attribution License (CC BY). The use, distribution or reproduction in other forums is permitted, provided the original author(s) and the copyright owner(s) are credited and that the original publication in this journal is cited, in accordance with accepted academic practice. No use, distribution or reproduction is permitted which does not comply with these terms.
*Correspondence: Yun Zhang, drzhangy91@163.com; Ji-Yan Liu, liujiyan1972@163.com
†These authors have contributed equally to this work and share first authorship
‡ORCID: Yun Zhang, orcid.org/0000-0001-9621-1408
Ji-Yan Liu, orcid.org/0000-0003-4885-5481