- 1Department of Internal Medicine, Division of Pulmonary, Critical Care, and Sleep Medicine, The Ohio State University, Columbus, OH, United States
- 2Clinical Trials Management Office, College of Medicine, The Ohio State University, Columbus, OH, United States
- 3The Center for RNA Biology, College of Medicine, The Ohio State University, Columbus, OH, United States
Introduction: The acute respiratory distress syndrome (ARDS) is a common complication of severe COVID-19 and contributes to patient morbidity and mortality. ARDS is a heterogeneous syndrome caused by various insults, and results in acute hypoxemic respiratory failure. Patients with ARDS from COVID-19 may represent a subgroup of ARDS patients with distinct molecular profiles that drive disease outcomes. Here, we hypothesized that longitudinal transcriptomic analysis may identify distinct dynamic pathobiological pathways during COVID-19 ARDS.
Methods: We identified a patient cohort from an existing ICU biorepository and established three groups for comparison: 1) patients with COVID-19 ARDS that survived hospitalization (COVID survivors, n = 4), 2) patients with COVID-19 ARDS that did not survive hospitalization (COVID non-survivors, n = 5), and 3) patients with ARDS from other causes as a control group (ARDS controls, n = 4). RNA was isolated from peripheral blood mononuclear cells (PBMCs) at 4 time points (Days 1, 3, 7, and 10 following ICU admission) and analyzed by bulk RNA sequencing.
Results: We first compared transcriptomes between groups at individual timepoints and observed significant heterogeneity in differentially expressed genes (DEGs). Next, we utilized the likelihood ratio test to identify genes that exhibit different patterns of change over time between the 3 groups and identified 341 DEGs across time, including hemoglobin subunit alpha 2 (HBA1, HBA2), hemoglobin subunit beta (HBB), von Willebrand factor C and EGF domains (VWCE), and carbonic anhydrase 1 (CA1), which all demonstrated persistent upregulation in the COVID non-survivors compared to COVID survivors. Of the 341 DEGs, 314 demonstrated a similar pattern of persistent increased gene expression in COVID non-survivors compared to survivors, associated with canonical pathways of iron homeostasis signaling, erythrocyte interaction with oxygen and carbon dioxide, erythropoietin signaling, heme biosynthesis, metabolism of porphyrins, and iron uptake and transport.
Discussion: These findings describe significant differences in gene regulation during patient ICU course between survivors and non-survivors of COVID-19 ARDS. We identified multiple pathways that suggest heme and red blood cell metabolism contribute to disease outcomes. This approach is generalizable to larger cohorts and supports an approach of longitudinal sampling in ARDS molecular profiling studies, which may identify novel targetable pathways of injury and resolution.
Introduction
From emergence to January 2024, coronavirus disease 2019 (COVID-19) has led to over 7 million deaths worldwide (1). Acute respiratory distress syndrome (ARDS) is one complication of severe COVID-19 and contribute to COVID-19 related death (2, 3). Among hospitalized patients with COVID-19, nearly one-third develop ARDS (4). Autopsy studies of COVID-19 reveal evidence of ARDS in most decedents (5, 6). Epidemiologic data from 2020 suggests at least a five-fold increase in ARDS-related deaths during the height of the COVID-19 pandemic (7). ARDS is a form of acute, non-cardiogenic, hypoxemic respiratory failure, characterized by bilateral lung infiltrates (8) that accounts for 10% of ICU admissions (9) with mortality rates ranging from around 30-50% (9). ARDS is notoriously heterogeneous, affecting varied patient populations with lung injury from various causes. One current focus of ARDS research is the identification of distinct patient subgroups that display varied outcomes and responses to targeted therapies (10–13). While clinical trials have demonstrated the efficacy of corticosteroids as treatment in COVID-19 ARDS (14, 15), studies evaluating immune modulating therapies in diverse ARDS populations have yielded mixed results (16, 17). Despite decades of clinical trials in ARDS, supportive care remains the primary treatment approach in diverse ARDS populations (18), and effective, targeted therapeutics for ARDS are lacking.
A better understanding of ARDS pathobiology may inform the limitations of current treatments, and the COVID-19 global pandemic has renewed the importance and urgency of new approaches to ARDS research. Recent studies have employed molecular profiling tools, such as RNA-sequencing, single-cell RNA-seq, and proteomics, with varied study design to better understand gene signatures that correlate with disease severity among COVID-19 ARDS patients. In this respect, multiple studies have highlighted the importance of type I interferon signaling and acute pro-inflammatory mediators in peripheral blood mononuclear cells (PBMCs) collected from patients early in disease course that correlate with COVID-19 disease severity (19–21), suggesting that PBMC analysis provides valuable insight into the dysregulated biology of COVID-19. As COVID-19 is dynamic with an evolving disease course over hours and days, other groups have examined transcriptomic data from patients at multiple time-points with varied sampling schema, including defined clinical stages (treatment, convalescence, and rehabilitation) (20), early and late ICU time points (22), and sampling from admission through two months of follow-up (23). Optimal strategies for patient sampling during the course of COVID-19 ARDS illness and recovery remain unclear. Our approach of longitudinal patient sampling provides opportunities to identify mechanisms that may contribute to disease progression or resolution.
To better understand how dynamic gene expression correlates with clinical outcomes among a group of patients with COVID-19 ARDS, we performed RNA-sequencing of patient PBMCs collected at 4 fixed time intervals across ICU admission. We hypothesized that longitudinal analysis may identify distinct transcriptomic changes compared to analysis at single time points to better characterize dynamic processes of injury and repair in COVID-19 ARDS. Here, we established three groups for comparison, including patients with COVID-19 ARDS that survived hospitalization, patients with fatal COVID-19 ARDS, and ARDS patients without COVID-19 as a control comparison group. We analyzed differential gene expression at each individual time point and performed longitudinal analysis to identify unique patterns of dynamic gene expression throughout acute illness.
Methods
Study design and identification of patient cohort
Patients with ARDS with available longitudinal peripheral blood samples were identified from the Ohio State University Intensive Care Unit Registry (BuckICU), a pre-existing, IRB-approved (IRB #2020H0175) biorepository that enrolls patients within 48 hours of admission to the intensive care units at the Ohio State University Wexner Medical Center and the Arthur G. James Cancer Hospital and Richard J. Solove Research Institute with acute respiratory failure and/or suspicion of sepsis. For inclusion in the BuckICU biorepository, acute respiratory failure is defined by an increase in supplemental oxygen requirement to maintain oxygen saturation (SpO2) greater than 92% or the need for adjunctive respiratory support, including high flow nasal cannula, non-invasive positive pressure ventilation or mechanical ventilation. To screen patients for enrollment, BuckICU defines suspicion of sepsis as meeting SIRS criteria ((any two or more of White Blood Cell count > 12 or < 4 x 109/L, heart rate > 90 beats per minute, respiratory rate > 20 breaths per minute, or temperature > 38°C or < 36°C) and clinical suspicion of infection (collection of any clinical culture specimen OR initiation of antibiotics)) (24). Following completion of the study protocol, patient cases are adjudicated by two pulmonary and critical care physicians. While SIRS criteria were used for screening purposes, Sepsis-3 guidelines were used to define sepsis during case adjudication. Sepsis-3 defines sepsis as organ dysfunction caused by dysregulated host response to infection represented by an increase in Sequential Organ Failure Assessment (SOFA) score of at least 2 points due to infection (25, 26). For this study, we identified patients between May 2020 and June 2021 who required mechanical ventilation at ICU admission with ARDS as defined by the Berlin definition (8), with at least 3 available longitudinal blood samples (days 1, 3, and 7). As this study aimed to identify differences in gene expression with correlation to COVID-19 ARDS mortality, we selected 9 patients with ARDS and positive SARS-CoV-2 upper respiratory tract testing by PCR and an additional 4 patients with ARDS and negative SARS-CoV-2 testing to characterize differences in gene expression that may be specific to COVID-19 status. As shown in Figure 1, we defined 3 groups: 1) patients with COVID-19 ARDS who survived hospitalization (COVID survivors, n = 4); 2) patients with COVID-19 ARDS who did not survive hospitalization (COVID non-survivors, n = 5); and 3) patients with ARDS from other causes (ARDS controls, n = 4).
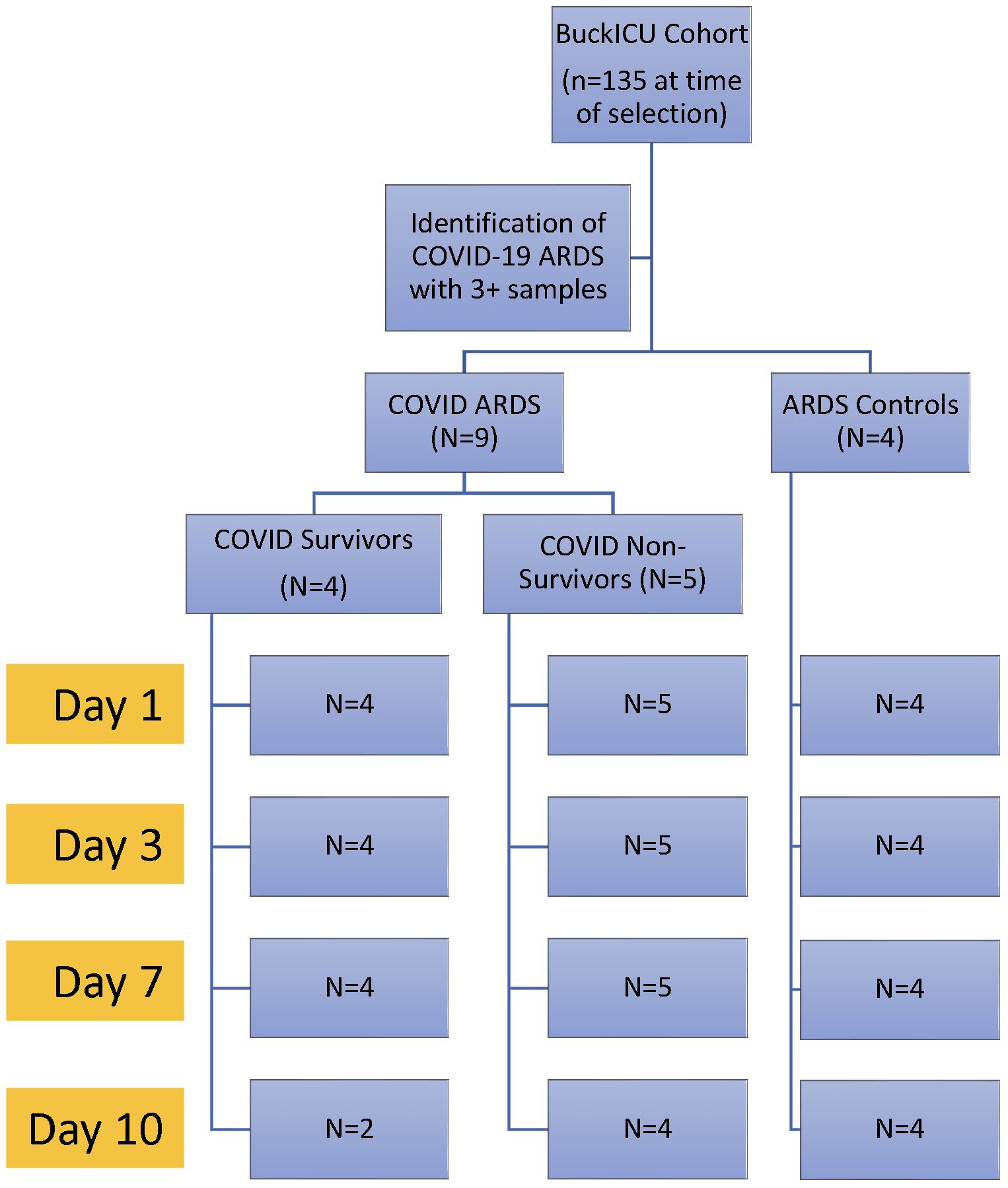
Figure 1 Experimental design. Patients were identified from the Ohio State University Intensive Care Unit Registry (BuckICU). Subjects with available longitudinal biosamples with COVID-19 ARDS or non-COVID-19 ARDS controls were selected for inclusion in the study.
RNA extraction, RNA-seq library construction and sequencing
For gene expression profiling, we used longitudinal, banked, peripheral blood mononuclear cells (PBMCs), isolated by Ficoll (Sigma, Cytiva 17-1440-03) density gradient centrifugation of whole blood, lysed in Trizol (Invitrogen, 15596026), and stored at -80°C. We extracted RNA using the Direct-zol RNA Miniprep Plus kit (Zymo Research, R2071) followed by RNA cleanup with the Monarch RNA Cleanup Kit (New England BioLabs, #T2040, 50 μg) with additional in tube DNaseI treatment, per the manufacturer’s protocols. Total RNA was quantified using the Invitrogen Qubit RNA HS Assay kit (Invitrogen, Carlsbad, CA) and RNA quality was assessed by RNA integrity scoring using Agilent 2100 Bioanalyzer and/or 2200 TapeStation (Agilent, Santa Clara CA). RNA seq libraries were prepared using kits from New England Biolabs (Ipswich, MA) with 100 ng total RNA by targeted depletion of rRNA (NEB E#E6310x). Fragmentation and amplification were done using NEBNext Ultra II Directional (stranded) RNA Library Prep Kit (NEB#E7760L) and NEBNext (E64490S/L). Samples were sequenced to a depth of 40 million paired-end 150 bp clusters on the Illumina NovaSeq platform (Illumina, Inc., San Diego, CA) through the Ohio State University Genomics Shared Resource.
Sequence alignment, gene count generation, and differential expression analysis
RNA sequencing sample reads underwent pre-alignment quality control with fastqc followed by alignment to the human genome GRCh38.96 (GCF_000001405.26) using HiSAT2 (v2.1.0) to obtain feature counts for each sample. Read alignment was performed through the Ohio Supercomputer Center (27). These counts were used for Differential Expression Analysis using the DESeq2 package in R (version 4.3.2). Lowly expressed genes were removed, and counts underwent normalization prior to differential expression analysis. Data visualizations were performed in R. We used the likelihood ratio test (LRT) to compare groups with or without time as an interaction term. Genes were determined to be significantly expressed based on adjusted p < 0.05 (false discovery rate). A variance stabilizing transformation (VST) was performed for normalization to create heat maps or cluster diagrams.
Ingenuity pathway analysis
We utilized Ingenuity Pathway Analysis (IPA v23.0, Qiagen) software to analyze the resultant gene expression data to identify canonical pathways common to the DEGs in each comparison. DESeq2 results were uploaded to IPA along with their corresponding adjusted p values, as the LRT does not provide fold change results. Pathways were filtered for significance at a -log10 p value of 1.3, which corresponds to an FDR of 0.05.
Results
Subject cohort characteristics
Subject characteristics are shown in Table 1. Per group definitions, all subjects in the COVID non-survivors group did not survive hospitalization, while all subjects in the COVID survivors group survived critical illness. All patients met ARDS criteria during ICU admission, required mechanical ventilation with low tidal volume ventilation, and received standard ICU supportive care. At Day 1, P/F ratios were similar across groups. Subjects with COVID-19 showed decreased P/F at Day 3, compared to Day 1, and COVID-19 survivors showed improvements in P/F later in ICU admission, compared to COVID-19 non-survivors. ARDS controls and COVID-19 non-survivors had significantly higher SOFA scores at Day 1 with higher SOFA scores throughout ICU admission compared to COVID-19 survivors. Further, vasopressor requirements were higher in the ARDS controls and COVID-19 non-survivors compared to COVID-19 survivors. All ARDS controls had sepsis from a bacterial infection as a risk factor leading to ARDS. These patients received treatment per current sepsis care guidelines (28, 29), including source control, early antibiotics, and volume resuscitation. All COVID-19 subjects tested positive for SARS-CoV-2 by upper respiratory tract nucleic acid amplification test, and all subjects were unvaccinated against SARS-CoV-2. Some COVID-19 patients had positive bacterial cultures during the study period, but COVID-19 was determined to be the primary risk factor for ARDS. There were differences in steroid exposure among groups, as all patients in the COVID-19 survivors group received dexamethasone, while 4 of 5 COVID-19 non-survivors and no ARDS Controls received dexamethasone.
Analysis of differential gene expression at single time points highlights heterogeneity of host responses during ARDS
Prior to analyzing dynamic gene expression patterns across ICU admission, we first performed differential expression analysis at each time point (days 1, 3, 7, and 10), comparing the three groups (COVID survivors, COVID non-survivors, and ARDS controls). Prior studies had analyzed transcriptomes of biospecimens from early time points of severe COVID-19 and identified genes associated with interferon signaling, T cell activation, and acute inflammation that correlated with worse outcomes. Comparing our 3 groups at each collection day, we identified 150 differentially expressed genes (DEGs) at Day 1, 803 DEGs at Day 3, 514 DEGs at Day 7, and 172 DEGs at Day 10. We next identified common differences in gene expression across time points by comparing the lists of DEGs and observed that the majority of DEGs identified at each timepoint were unique (Figure 2A). For example, differences in gene expression profiles peaked at Day 3. However, of the 803 DEGs identified at Day 3, only 82 of these transcripts were determined to be significantly different at the other time points. We observed greatest overlap between Days 1 and 3 (43 common DEGs) and Days 7 and 10 (62 common DEGs).
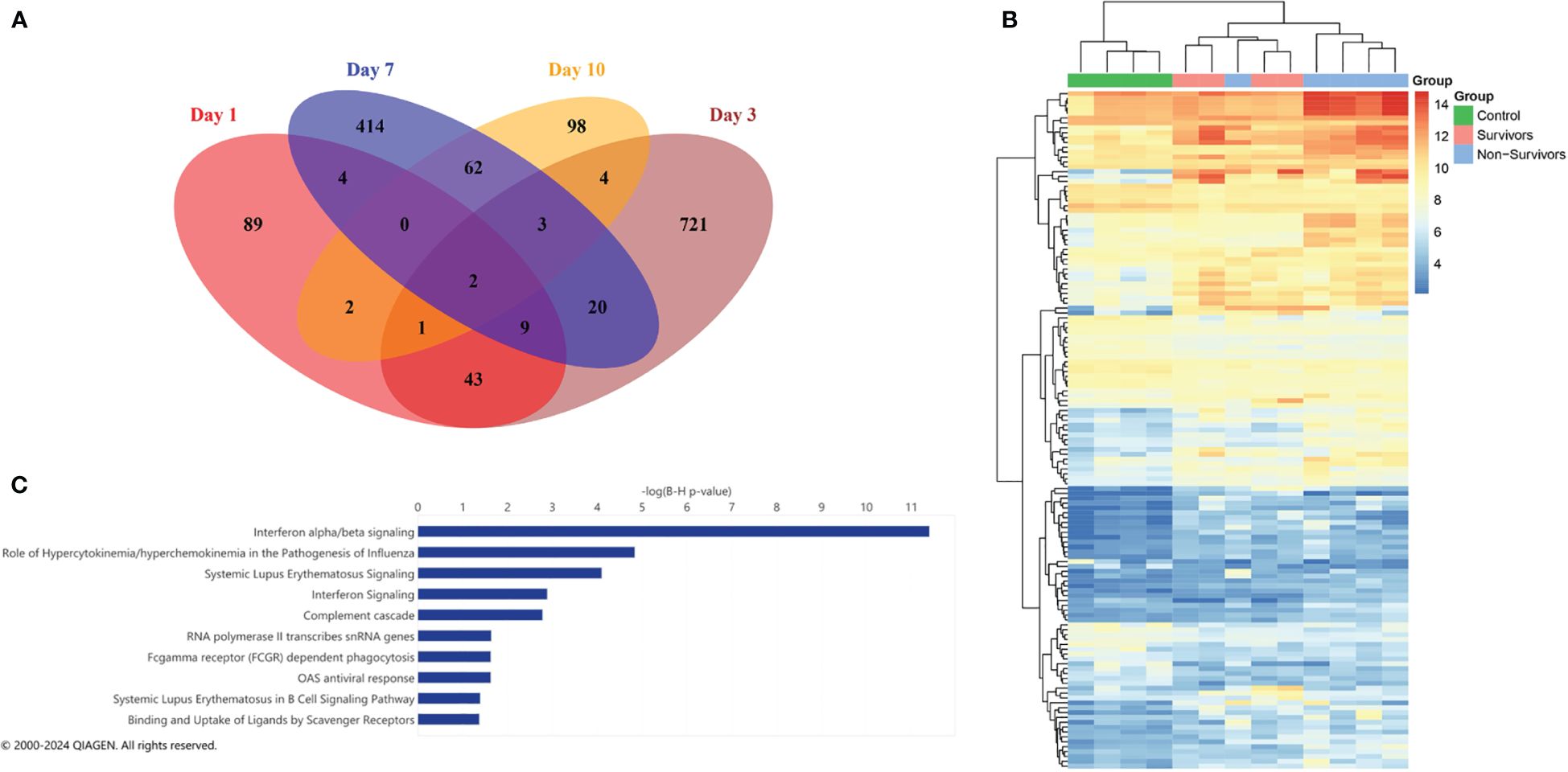
Figure 2 Differential expression analysis on individual days. (A) Venn diagram showing overlap of significant differentially expressed genes for each day. (B) Heatmap of the 150 significant DEGs (FDR < 0.05) identified at Day 1 with column clustering by gene expression pattern. (C) Ingenuity pathway analysis (IPA) of canonical pathways identified by DEGs at Day 1. The length of the bar indicates statistical significance of each pathway using -log10 BH multiple correction p-value.
As prior studies have characterized differential expression at early time points in COVID-19, we further examined the 150 DEGs identified at Day 1. The top 20 DEGs identified at Day 1 between the three groups are shown in Table 2. Clustering of DEGs revealed correlation with our pre-defined comparison groups apart from one non-survivor, who displayed a pattern of expression similar to COVID survivors (Figure 2B). Considering all COVID-19 patients, we observed increases in interferon-stimulated genes, including interferon alpha-inducible protein 6 (IFI6), interferon alpha-inducible protein 27, mitochondrial (IFI27) (Table 2). Ingenuity pathway analysis (IPA) of DEGs revealed canonical pathways primarily related to interferon signaling (Figure 2C).
Longitudinal analysis of gene expression reveals additional host response mechanisms
As we observed significant heterogeneity of differential gene expression at each time point, we next compared dynamic gene expression across time. As opposed to identifying differences at one timepoint, this approach allows for identification of transcripts that show differential patterns of expression across time (i.e. Which genes change over time? Are those patterns of change different between groups)? Here we identified 341 genes with significant differential expression across timepoints (p < 0.05). The top 20 significant DEGs identified by our longitudinal analysis are shown in Table 3. Notably, this approach only determines significance and does not specify fold change. After filtering for significant DEGs, we next performed additional visualizations to determine directionality and patterns of change. First, clustering analysis identified transcripts that demonstrate similar patterns of temporal change. Of the 341 DEGs, 314 showed similar temporal patterns (Figure 3). The top 20 genes demonstrating the most significant differences include hemoglobin subunit alpha 2 (HBA1, HBA2), hemoglobin subunit beta (HBB), von Willebrand factor C and EGF domains (VWCE), and carbonic anhydrase 1 (CA1) (Table 3). Among COVID survivors, the 314 genes showed downregulation of expression over time with a nadir by Day 7, compared to the COVID non-survivors and ARDS controls. The ARDS control patients demonstrated a pattern of increase in temporal gene expression, and the COVID non-survivors demonstrated delayed increases in these significantly changed genes over time (Figure 3).
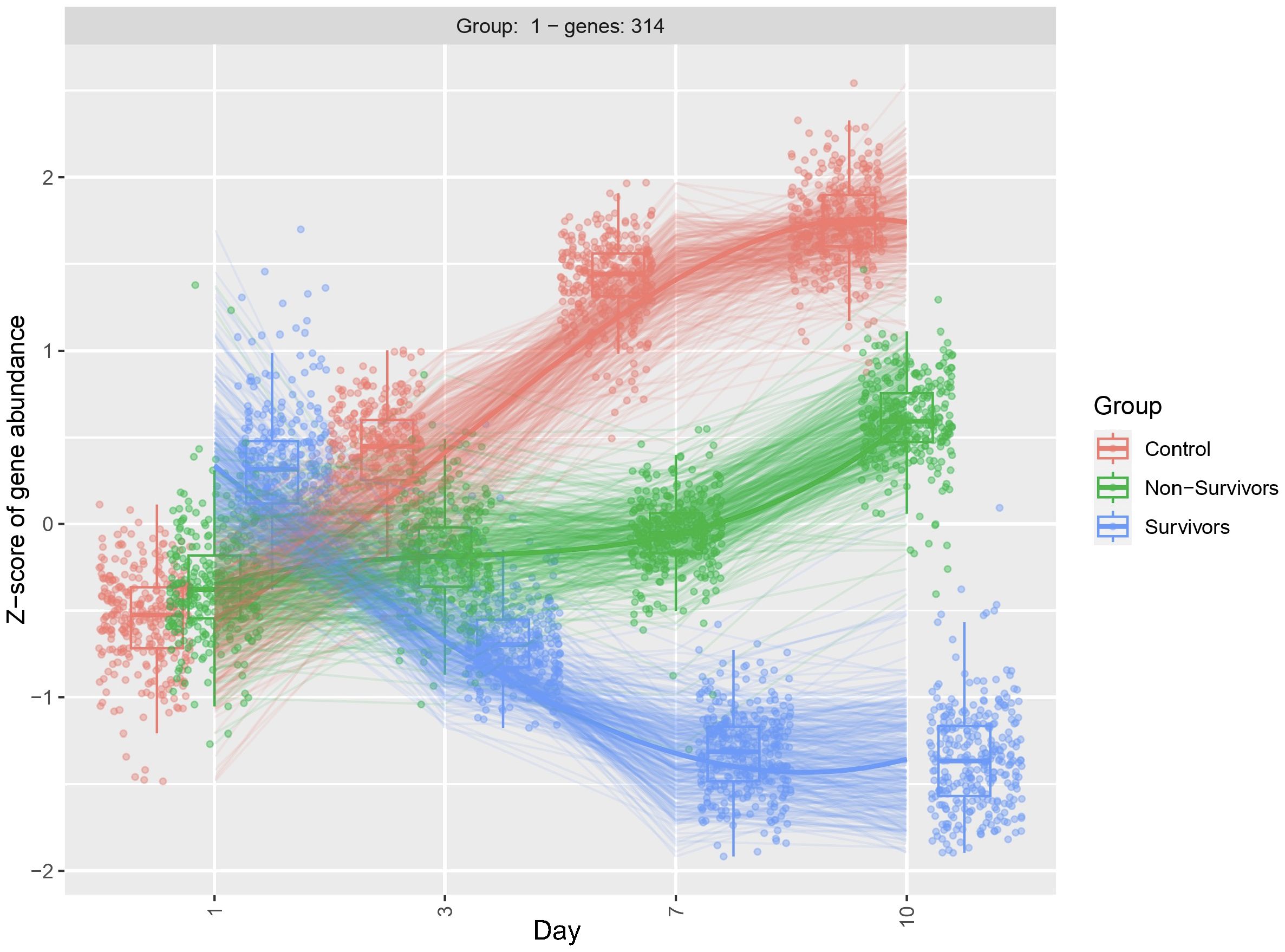
Figure 3 Clustering diagram demonstrates differential dynamic gene expression across time. Using the likelihood ratio test, we compared differences in dynamic gene expression across 3 patient groups, COVID-19 ARDS survivors, COVID-19 ARDS non-survivors, and ARDS controls and 4 time points with Day 1 as reference. DEGs were clustered by similar patterns of gene expression. 314 of the 341 DEGs identified by the longitudinal analysis showed a similar pattern of dynamic change. Significance determined by the adjusted p-value from DESeq2 analysis.
To better understand dynamic gene expression at the level of individual genes, we used box plots to visualize gene expression of the top 20 genes identified by the LRT. As expected, these genes demonstrate a similar pattern of change that was observed in the clustering analysis (Figure 3). Specifically, we observe the most variability in gene expression at day 1 for the COVID survivors, compared to the other two comparison groups. Further, differences in gene expression between groups increases across time (Figure 4). Together, these findings suggest that differences in host response across disease course may better inform underlying biological processes contributing to outcomes than differential gene expression at ICU admission.
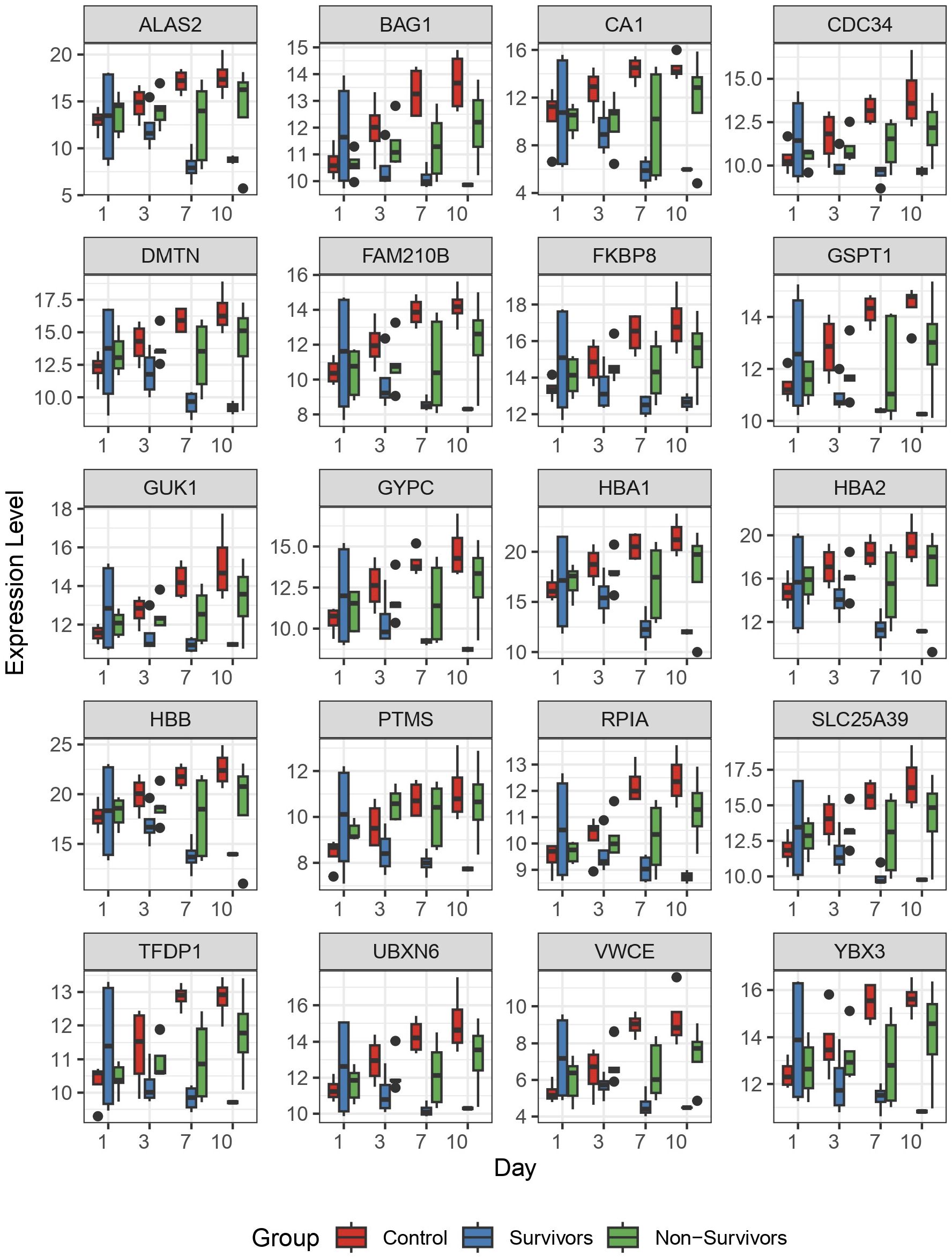
Figure 4 The top 20 differentially expressed genes identified by longitudinal analysis. Following identification of significantly differentially expressed genes by longitudinal analysis, we plotted the 20 genes with most significant differences to observe individual patterns of change. Here, we plotted normalized gene expression by variance stabilizing transformation (y-axis) against time (days 1, 3, 7, and 10 on the x-axis). Colored boxes represent the 3 comparison groups.
Perturbations in hematopoiesis and erythrocyte function pathways correlated with COVID-19 fatality
To identify common biological pathways among the significant DEGs observed in our LRT comparisons, we utilized Ingenuity Pathway Analysis (IPA) to elucidate the pathways engaged among COVID survivors, non-survivors, and ARDS controls. As we compared 3 groups, this analysis does not specify fold-change differences or directionality and describes only significance and pathway enrichment, determined by the number of DEGs involved in each pathway. Among the most represented pathways, we identified iron homeostasis signaling, erythrocyte interaction with oxygen and carbon dioxide, transcriptional activity of SMAD2/SMAD3:SMAD4 heterotrimer, deubiquitination, erythropoietin signaling, heme biosynthesis, metabolism of porphyrins, and iron uptake and transport among the top 12 IPA canonical pathways (Figure 5). These results suggest that oxygen carrying capacity and metabolism of heme may be important modulators of disease course in COVID-19 ARDS.
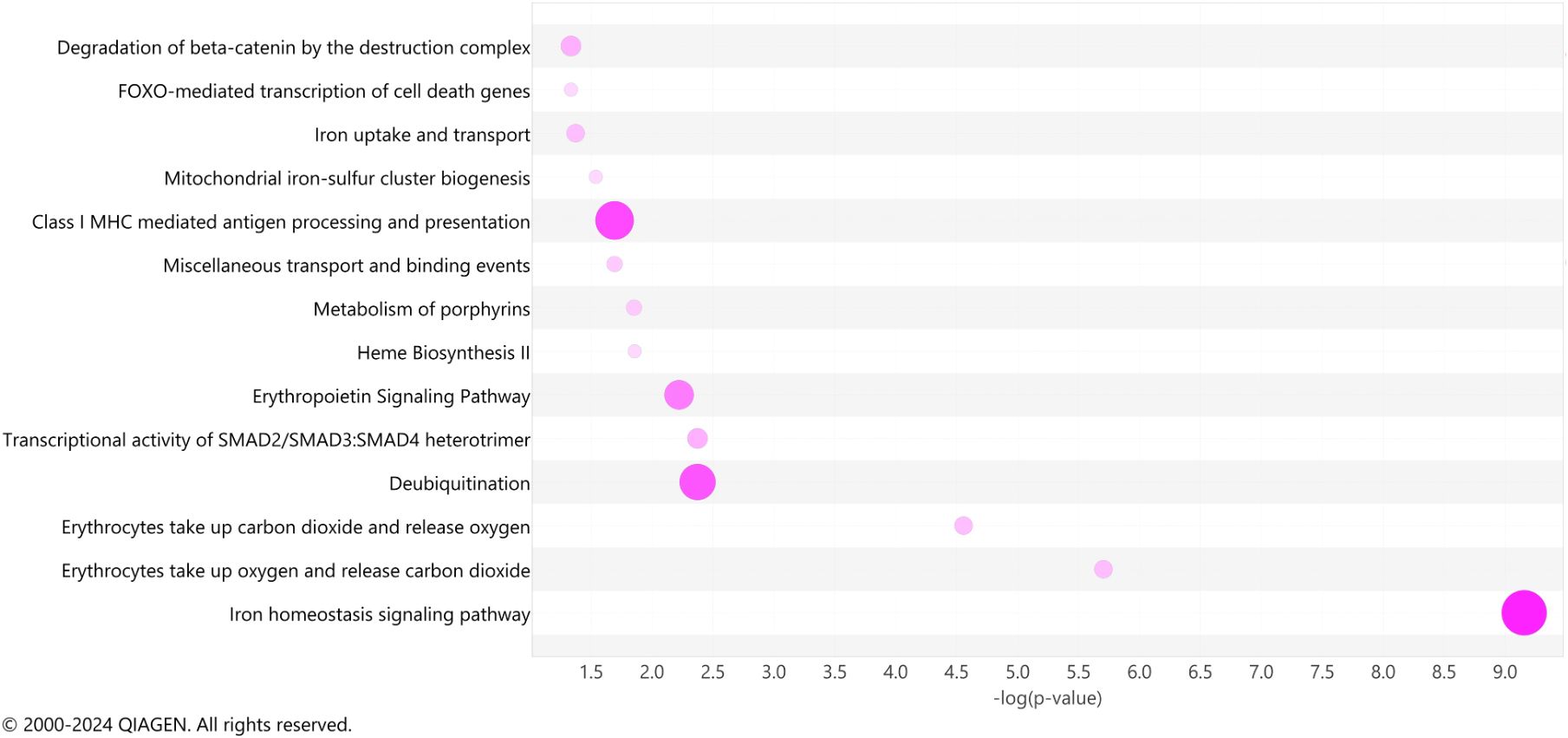
Figure 5 Ingenuity pathway analysis. A bubble chart shows the top pathway categories of the IPA canonical pathways of significant genes (adjusted p < 0.05) detected across all days simultaneously. The size and color intensity of bubbles indicates the number of genes overlapping each pathway.
Discussion
Here we performed longitudinal RNA sequencing analysis of PBMCs from patients with ARDS at a single center during the COVID-19 pandemic (May 2020 – June 2021) and compared temporal gene expression changes between COVID-19 ARDS survivors and non-survivors, as well as non-COVID ARDS patients, across 10 days of ICU admission. Longitudinal analysis revealed 341 transcripts with significantly different patterns of dynamic gene expression over time with most significant differences in pathways of iron homeostasis, heme biosynthesis, and erythrocyte function, that remained upregulated throughout ICU course in fatal COVID-19 ARDS compared to survivors of COVID-19 ARDS. Enriched gene signatures of hemoglobin metabolism have previously been described in blood leukocytes from septic patients (30). In vitro studies have shown induction of hemoglobin genes during cellular stress in murine macrophages (31) and human PBMCs (32). The role of heme in macrophages is complex (32–34) and additional data are needed to support strategies targeting heme biosynthesis in ARDS patients. Notably, our study employing longitudinal sampling and analysis identified distinct pathway regulation throughout disease course that was not identified at single time points. This study highlights the dynamic nature of COVID-19 ARDS and represents a novel approach towards better understanding of COVID-19 ARDS pathobiology.
The COVID-19 pandemic increased the global incidence of severe viral pneumonia, acute respiratory failure, and ARDS (7). Clinicians and researchers alike observed unique features of COVID-19 ARDS and raised the question: Is COVID-19 ARDS somehow different than “regular” ARDS (35, 36)? As a syndrome, ARDS is known for its heterogeneity and efforts to identify subtypes and subgroups of ARDS patients predate the pandemic (10, 13). Our study identified a cohort of ARDS patients with COVID-19 and varied clinical outcomes and compared to a group of patients with ARDS and bacterial sepsis as the primary ARDS risk factor. Our differential expression analysis on Day 1 of study enrollment was characterized by significant activation of immune system pathways and interferon signaling consistent with prior reports in studies of severe COVID-19 (37–40). Dysregulated interferon signaling has been implicated in COVID-19 as an important driver of pathology (21). Compared to healthy controls, interferon responses are elevated in COVID-19 (41). However, when compared to other viral infections or among COVID-19 patients with varied clinical course, interferon responses are more variable (42). Subjects in our comparison group of non-COVID-19 ARDS had sepsis from bacterial sources as their primary risk factor for ARDS. Host responses to bacterial versus viral infection yield different transcriptomic signatures (43–45), which may account for the significant differences in interferon responses in our analysis. While multiple interferon-stimulated genes showed significant upregulation in our analysis, one specific gene, interferon-inducible protein 27 (IFI27), showed significant upregulation at study enrollment in COVID-19 ARDS compared to non-COVID-19 ARDS. This recapitulates findings of other study that suggest robust and specific upregulation of IFI27 in COVID-19. A re-analysis of nine independent cohort studies that analyzed peripheral blood gene expression across multiple infections, including COVID-19, influenza, and bacterial pneumonia, described a COVID-19-specific gene signature comprised of 149 genes, among which IFI27 was the sole gene directly associated with the interferon response (46). A single-center cohort study comparing peripheral blood transcriptomes at one time point showed IFI27 was highly upregulated in COVID-19, even when compared to influenza and seasonal coronaviridae (42). Despite reports of varied interferon responses, robust induction of IFI27 may represent a peripheral blood gene signature unique to COVID-19 infection. Notably, our study, like the above referenced COVID-19 transcriptomics studies, focused on mixed populations of peripheral blood cells, which may reflect transcriptomic signatures driven by a single cell type or changes in relative cell populations. Further, PBMCs represent transcriptional signatures in peripheral blood, which may differ from the lung transcriptome (47).
Despite multiple prior reports of transcriptomics in COVID-19, our study is unique in our sampling and analysis strategy. In opposition to single time-point studies or limited longitudinal sampling, we analyzed peripheral blood gene expression throughout acute illness at multiple short intervals. While our study is limited by the small sample size and single-center design, it provides proof of concept that longitudinal molecular profiling can be valuable for identifying dynamic molecular mechanisms that function during ARDS disease course. In ARDS studies, analysis at individual timepoints allows for identification of relative differences in gene expression between groups. However, this design is vulnerable to baseline patient heterogeneity and lead-time bias. While longitudinal sampling is subject to the same obstacles, our approach allows each patient to function as their own baseline control and focuses on dynamic gene expression during acute illness. We identified gene signatures related to iron homeostasis, erythropoietin signaling, heme biosynthesis, and iron uptake that we grouped into processes contributing to erythropoiesis, as well as upregulation of erythrocyte function in fatal COVID-19 compared to COVID-19 survivors. Among ARDS controls, we observed early upregulation of these genes compared to the other groups with decreased rate of change between days 7 and 10. Among the COVID-19 non-survivors, gene expression related to erythropoiesis and erythrocyte function showed late increased compared to COVID-19 survivors, which showed relatively low expression throughout ICU course. Other groups have employed sampling at multiple time points with various strategies and have also identified gene expression correlating with pathways of hemopoiesis, reactive oxygen species, and erythrocyte functioning (20, 22, 23). Zheng et al. examined longitudinal transcriptomes but defined three ad hoc clinical stages (treatment, convalescence, and rehabilitation) as opposed to consistent time intervals; they identified early downregulation of genes related to humoral immunity and type I interferon response and upregulated gene expression related to hemopoiesis, regulation of inflammatory response, mRNA splicing via spliceosome, and epithelial cell proliferation during COVID-19 recovery (20). Another study applied longitudinal multi-omics with 2 – 7 times points from 0 – 55 days after admission and demonstrated increased protein catabolism, erythrocyte differentiation, ferroptosis, and organelle disassembly in clusters primarily corresponding to COVID non-survivors compared to COVID survivors (23). As our study and others have identified common pathways of iron homeostasis and erythrocyte function, it is intriguing to hypothesize that interventions targeting effective erythropoiesis, iron handling, and erythrocyte function represent a novel therapeutic strategy in COVID-19 ARDS. Indeed, studies have demonstrated perturbations in iron handling and ferroptosis (iron-dependent cell death) due to COVID-19 in humans (48), animal models (49), and human cells (50), suggesting that COVID-19 may uniquely impact iron homeostasis and downstream pathways during disease course. Further, observational human studies, including ours, may be capturing a compensatory mechanism of increased erythropoiesis and erythrocyte function in severe COVID-19. Steroid treatment with dexamethasone is a common and accepted treatment in severe COVID-19 (14, 15) and may impact iron metabolism pathways (51). In our cohort, steroid exposure was high in the COVID-19 groups, as 8 of 9 subjects received dexamethasone. However, ARDS controls did not receive steroid treatment. All patients in our cohort met sepsis criteria, recognizing the frequent overlap between critical illness syndromes, such as ARDS and sepsis. Among septic patients, heme metabolism and iron homeostasis genes are enriched in white blood cells (neutrophils and PBMCs) compared to controls (30), and transcriptomic differences in heme biosynthesis genes correlated with sepsis subgroups or endotypes (52). In non-erythroid cells, hemoglobin scavenges free radicals and functions in nitric oxide metabolism (53). Cellular damage through reactive oxygen species is a known pathologic mechanism in both sepsis and ARDS. Our study and others (23, 52) demonstrate increased heme-related transcript expression correlated with worse outcomes in certain critically ill populations. We postulate that upregulation of these transcripts in non-erythroid cells represents a compensatory mechanism due to ongoing oxidative stress. As iron and heme metabolism are foundational biologic processes, it is unclear if these pathways represent viable interventional targets during COVID-19 ARDS.
Our study supports a novel patient sampling strategy and demonstrates a unique analysis of dynamic gene expression. We focused on patients with severe COVID-19 ARDS as a subgroup of ARDS patient to gain insights into disease pathology. Our findings suggest an approach for future studies, generalizable to larger COVID-19 and ARDS cohorts. Our study is limited by several factors. First, all patients were enrolled from a single center for analysis, which may not be representative of the general population. As we analyzed multiple samples from each subject, the sample size of the cohort was small. While we attempted to select age- and sex-matched subjects, the sample size does not allow for proper control of these potentially confounding variables. Despite the small cohort size, we were able to identify several biologically relative pathways, reflected in correlation with prior studies, and provide new insights via our longitudinal approach. We defined outcomes by mortality in this study. While our COVID-19 ARDS non-survivors demonstrate impaired oxygenation throughout ICU stay, consistent with worsened ARDS, this group also had baseline higher SOFA scores that increased over time and increased vasopressor requirements, which may confound our findings. Further, this study used ARDS as a primary inclusion criterion, but severe COVID-19 is a systemic disease. All patients in our cohort met criteria for sepsis, and we did not attempt to differentiate COVID-19 ARDS, sepsis, or both as independent groups. Our transcriptomic findings in peripheral blood cells may be related to systemic responses instead of lung-specific pathology related to COVID-19 or ARDS. Disease classification in critical illness is currently limited by often overlapping syndrome-based paradigms (54, 55). However, transcriptomic studies have the potential to identify dysregulated host response pathways during critical illness that inform clinical disease course beyond established definitions of ARDS or sepsis (56).
Conclusion
Patients with ARDS from COVID-19 may represent a subgroup of ARDS patients with distinct molecular pathophysiology that drive disease outcomes. Our study identified differences in dynamic expression of genes related to iron homeostasis and erythrocyte function that correlate to survival in COVID-19 ARDS. Our findings are supported by prior studies of molecular profiling in COVID-19 and suggest that iron handling and ferroptosis may be putative mechanisms of ongoing lung injury during SARS-CoV-2 infection. Additional research is needed to examine the therapeutic potential of these pathways. Finally, our study suggests that short interval longitudinal sampling during acute illness may uncover novel mechanisms of injury, repair, and resolution during COVID-19 ARDS. This approach should be considered further for future ARDS molecular profiling study design.
Data availability statement
The data presented in the study are deposited in the Gene Expression Omnibus (https://www.ncbi.nlm.nih.gov/geo/), accession number GSE273149.
Ethics statement
The studies involving humans were approved by The Ohio State University Biomedical Sciences Institutional Review Board. The studies were conducted in accordance with the local legislation and institutional requirements. The human samples used in this study were acquired from an existing biorepository that collects and stores human biosamples and data from critically-ill patients at Ohio State University. The biorepository project utilizes broad consent to collect and store samples for use in secondary analyses. Written informed consent for participation was not required from the participants or the participants’ legal guardians/next of kin in accordance with the national legislation and institutional requirements.
Author contributions
ME: Formal Analysis, Validation, Visualization, Writing – original draft, Writing – review & editing, Data curation, Investigation. FJ: Data curation, Investigation, Writing – review & editing, Methodology. DF: Investigation, Methodology, Writing – review & editing, Supervision. LL: Investigation, Writing – review & editing, Data curation. SC: Data curation, Investigation, Writing – review & editing. KH: Data curation, Investigation, Writing – review & editing. SK: Data curation, Investigation, Writing – review & editing. GS: Data curation, Investigation, Writing – review & editing. SP: Data curation, Investigation, Writing – review & editing, Conceptualization, Methodology. JH: Methodology, Writing – review & editing, Funding acquisition, Project administration, Resources, Supervision. RM: Writing – review & editing, Funding acquisition, Methodology, Project administration, Resources, Supervision. JE: Methodology, Writing – review & editing, Data curation, Investigation, Writing – original draft. JB: Methodology, Writing – original draft, Writing – review & editing, Conceptualization, Formal Analysis, Funding acquisition, Project administration, Visualization.
Funding
The authors declare financial support was received for the research, authorship, and/or publication of this article. This project was supported, in part, by the National Center for Advancing Translational Sciences (NCATS) of the National Institutes of Health (NIH) under Grant Numbers UM1TR004548 and KL2TR002734. This project was also supported by NIH R01HL142767 awarded to J.A.E., R01HL141195 awarded to J.C.H., P01HL114453, R01HL097376, R01HL081784, and R01HL096376 awarded to R.K.M., K08HL169725 awarded to J.S.B., and the Ohio State University Office of Research 2020 COVID-19 Seed Funding Program and the Department of Internal Medicine 2021 Junior Investigator Award, both awarded to J.S.B.
Acknowledgments
We thank the Ohio State University Clinical Trials Management Office and Critical Care Clinical Trials group, specifically Brent Oleksak, Preston So, and Madison So, for collection and processing of biospecimens and clinical data. We thank all clinical staff that helped in sample collection. We thank Pearlly Yan and Estela Puchulu-Campanella from the Ohio State Shared Genomics Resource for sample processing and sequencing services. We are forever grateful to the patients and their family members who participated in this research that would not have been possible without their support.
Conflict of interest
The authors declare that the research was conducted in the absence of any commercial or financial relationships that could be construed as a potential conflict of interest.
Publisher’s note
All claims expressed in this article are solely those of the authors and do not necessarily represent those of their affiliated organizations, or those of the publisher, the editors and the reviewers. Any product that may be evaluated in this article, or claim that may be made by its manufacturer, is not guaranteed or endorsed by the publisher.
Author disclaimer
The content is solely the responsibility of the authors and does not necessarily represent the official views of the National Institutes of Health.
References
1. World Health Organization (WHO). WHO COVID-19 dashboard. (2024). Available at: https://data.who.int/dashboards/covid19/cases.
2. Baud D, Qi X, Nielsen-Saines K, Musso D, Pomar L, Favre G. Real estimates of mortality following COVID-19 infection. Lancet Infect Dis. (2020) 20:773. doi: 10.1016/S1473-3099(20)30195-X
3. Zhou F, Yu T, Du R, Fan G, Liu Y, Liu Z, et al. Clinical course and risk factors for mortality of adult inpatients with COVID-19 in Wuhan, China: a retrospective cohort study. Lancet. (2020) 395:1054–62. doi: 10.1016/S0140-6736(20)30566-3
4. Tzotzos SJ, Fischer B, Fischer H, Zeitlinger M. Incidence of ARDS and outcomes in hospitalized patients with COVID-19: a global literature survey. Crit Care. (2020) 24:516. doi: 10.1186/s13054-020-03240-7
5. Schaller T, Hirschbuhl K, Burkhardt K, Braun G, Trepel M, Markl B, et al. Postmortem examination of patients with COVID-19. JAMA. (2020) 323:2518–20. doi: 10.1001/jama.2020.8907
6. Elsoukkary SS, Mostyka M, Dillard A, Berman DR, Ma LX, Chadburn A, et al. Autopsy findings in 32 patients with COVID-19: A single-institution experience. Pathobiology. (2021) 88:56–68. doi: 10.1159/000511325
7. Oud L, Garza J. The contribution of COVID-19 to acute respiratory distress syndrome-related mortality in the United States. J Clin Med Res. (2023) 15:279–81. doi: 10.14740/jocmr4915
8. ARDS Definition Task Force, Ranieri VM, Rubenfeld GD, Thompson BT, Ferguson ND, Caldwell E, et al. Acute respiratory distress syndrome: The berlin definition. JAMA. (2012) 307:2526–33. doi: 10.1001/jama.2012.5669
9. Bellani G, Laffey JG, Pham T, Fan E, Brochard L, Esteban A, et al. Epidemiology, patterns of care, and mortality for patients with acute respiratory distress syndrome in intensive care units in 50 countries. JAMA. (2016) 315:788–800. doi: 10.1001/jama.2016.0291
10. Calfee CS, Delucchi K, Parsons PE, Thompson BT, Ware LB, Matthay MA. Subphenotypes in acute respiratory distress syndrome: latent class analysis of data from two randomised controlled trials. Lancet Respir Med. (2014) 2:611–20. doi: 10.1016/S2213-2600(14)70097-9
11. Calfee CS, Janz DR, Bernard GR, May AK, Kangelaris KN, Matthay MA, et al. Distinct molecular phenotypes of direct vs indirect ARDS in single-center and multicenter studies. Chest. (2015) 147:1539–48. doi: 10.1378/chest.14-2454
12. Reilly JP, Bellamy S, Shashaty MGS, Gallop R, Meyer NJ, Lanken PN, et al. Heterogeneous phenotypes of acute respiratory distress syndrome after major trauma. Ann Am Thorac Soc. (2014) 11:728–36. doi: 10.1513/AnnalsATS.201308-280OC
13. Englert JA, Cho MH, Lamb AE, Shumyatcher M, Barragan-Bradford D, Basil MC, et al. Whole blood RNA sequencing reveals a unique transcriptomic profile in patients with ARDS following hematopoietic stem cell transplantation. Respir Res. (2019) 20:15. doi: 10.1186/s12931-019-0981-6
14. Group RC, Horby P, Lim WS, Emberson JR, Mafham M, Bell JL, et al. Dexamethasone in hospitalized patients with Covid-19. N Engl J Med. (2021) 384:693–704. doi: 10.1056/NEJMoa2021436
15. Tomazini BM, Maia IS, Cavalcanti AB, Berwanger O, Rosa RG, Veiga VC, et al. Effect of dexamethasone on days alive and ventilator-free in patients with moderate or severe acute respiratory distress syndrome and COVID-19: the coDEX randomized clinical trial. JAMA. (2020) 324:1307–16. doi: 10.1001/jama.2020.17021
16. Villar J, Ferrando C, Martinez D, Ambros A, Munoz T, Soler JA, et al. Dexamethasone treatment for the acute respiratory distress syndrome: a multicentre, randomised controlled trial. Lancet Respir Med. (2020) 8:267–76. doi: 10.1016/S2213-2600(19)30417-5
17. Steinberg KP, Hudson LD, Goodman RB, Hough CL, Lanken PN, Hyzy R, et al. Efficacy and safety of corticosteroids for persistent acute respiratory distress syndrome. N Engl J Med. (2006) 354:1671–84. doi: 10.1056/NEJMoa051693
18. Fan E, Brodie D, Slutsky AS. Acute respiratory distress syndrome: Advances in diagnosis and treatment. Jama. (2018) 319:698–710. doi: 10.1001/jama.2017.21907
19. Hadjadj J, Yatim N, Barnabei L, Corneau A, Boussier J, Smith N, et al. Impaired type I interferon activity and inflammatory responses in severe COVID-19 patients. Science. (2020) 369:718–24. doi: 10.1126/science.abc6027
20. Zheng HY, Xu M, Yang CX, Tian RR, Zhang M, Li JJ, et al. Longitudinal transcriptome analyses show robust T cell immunity during recovery from COVID-19. Signal Transduct Target Ther. (2020) 5:294. doi: 10.1038/s41392-020-00457-4
21. Lee JS, Park S, Jeong HW, Ahn JY, Choi SJ, Lee H, et al. Immunophenotyping of COVID-19 and influenza highlights the role of type I interferons in development of severe COVID-19. Sci Immunol. (2020) 5:eabd1554. doi: 10.1126/sciimmunol.abd1554
22. Rombauts A, Bodalo Torruella M, Abelenda-Alonso G, Perera-Bel J, Ferrer-Salvador A, Acedo-Terrades A, et al. Dynamics of gene expression profiling and identification of high-risk patients for severe COVID-19. Biomedicines. (2023) 11:1348. doi: 10.3390/biomedicines11051348
23. Sun C, Sun Y, Wu P, Ding W, Wang S, Li J, et al. Longitudinal multi-omics transition associated with fatality in critically ill COVID-19 patients. Intensive Care Med Exp. (2021) 9:13. doi: 10.1186/s40635-021-00373-z
24. Levy MM, Fink MP, Marshall JC, Abraham E, Angus D, Cook D, et al. 2001 SCCM/ESICM/ACCP/ATS/SIS international sepsis definitions conference. Intensive Care Med. (2003) 29:530–8. doi: 10.1007/s00134-003-1662-x
25. Singer M, Deutschman CS, Seymour CW, Shankar-Hari M, Annane D, Bauer M, et al. The third international consensus definitions for sepsis and septic shock (Sepsis-3). JAMA. (2016) 315:801–10. doi: 10.1001/jama.2016.0287
26. Guarino M, Perna B, Cesaro AE, Maritati M, Spampinato MD, Contini C, et al. 2023 Update on sepsis and septic shock in adult patients: Management in the emergency department. J Clin Med. (2023) 12:3188. doi: 10.3390/jcm12093188
28. Dellinger RP, Levy MM, Rhodes A, Annane D, Gerlach H, Opal SM, et al. Surviving Sepsis Campaign Guidelines Committee including the Pediatric, Surviving sepsis campaign: international guidelines for management of severe sepsis and septic shock: 2012. Crit Care Med. (2013) 41:580–637. doi: 10.1097/CCM.0b013e31827e83af
29. Dellinger RP, Levy MM, Carlet JM, Bion J, Parker MM, Jaeschke R, et al. Surviving Sepsis Campaign: international guidelines for management of severe sepsis and septic shock: 2008. Crit Care Med. (2008) 36:296–327. doi: 10.1097/01.CCM.0000298158.12101.41
30. Leite GGF, Scicluna BP, van der Poll T, Salomao R. Genetic signature related to heme-hemoglobin metabolism pathway in sepsis secondary to pneumonia. NPJ Syst Biol Appl. (2019) 5:26. doi: 10.1038/s41540-019-0105-4
31. Liu L, Zeng M, Stamler JS. Hemoglobin induction in mouse macrophages. Proc Natl Acad Sci U.S.A. (1999) 96:6643–7. doi: 10.1073/pnas.96.12.6643
32. Brunyanszki A, Erdelyi K, Szczesny B, Olah G, Salomao R, Herndon DN, et al. Upregulation and mitochondrial sequestration of hemoglobin occur in circulating leukocytes during critical illness, conferring a cytoprotective phenotype. Mol Med. (2015) 21:666–75. doi: 10.2119/molmed.2015.00187
33. Pradhan P, Vijayan V, Gueler F, Immenschuh S. Interplay of heme with macrophages in homeostasis and inflammation. Int J Mol Sci. (2020) 21:740. doi: 10.3390/ijms21030740
34. Olonisakin TF, Suber T, Gonzalez-Ferrer S, Xiong Z, Penaloza HF, van der Geest R, et al. Stressed erythrophagocytosis induces immunosuppression during sepsis through heme-mediated STAT1 dysregulation. J Clin Invest 131. (2021) 131:e137468. doi: 10.1172/JCI137468
35. Fan E, Beitler JR, Brochard L, Calfee CS, Ferguson ND, Slutsky AS, et al. COVID-19-associated acute respiratory distress syndrome: is a different approach to management warranted? Lancet Respir Med. (2020) 8:816–21. doi: 10.1016/S2213-2600(20)30304-0
36. Hariri L, Hardin CC. Covid-19, angiogenesis, and ARDS endotypes. N Engl J Med. (2020) 383:182–3. doi: 10.1056/NEJMe2018629
37. Ragab D, Salah Eldin H, Taeimah M, Khattab R, Salem R. The COVID-19 cytokine storm; what we know so far. Front Immunol. (2020) 11. doi: 10.3389/fimmu.2020.01446
38. García-González P, Tempio F, Fuentes C, Merino C, Vargas L, Simon V, et al. Dysregulated immune responses in COVID-19 patients correlating with disease severity and invasive oxygen requirements. Front Immunol. (2021) 12. doi: 10.3389/fimmu.2021.769059
39. Montazersaheb S, Hosseiniyan Khatibi SM, Hejazi MS, Tarhriz V, Farjami A, Ghasemian Sorbeni F, et al. COVID-19 infection: an overview on cytokine storm and related interventions. Virol J. (2022) 19:92. doi: 10.1186/s12985-022-01814-1
40. Tan LY, Komarasamy TV, RMT Balasubramaniam V. Hyperinflammatory immune response and COVID-19: A double edged sword. Front Immunol. (2021) 12. doi: 10.3389/fimmu.2021.742941
41. Wilk AJ, Rustagi A, Zhao NQ, Roque J, Martínez-Colón GJ, McKechnie JL, et al. A single-cell atlas of the peripheral immune response in patients with severe COVID-19. Nat Med. (2020) 26:1070–6. doi: 10.1038/s41591-020-0944-y
42. McClain MT, Constantine FJ, Henao R, Liu Y, Tsalik EL, Burke TW, et al. Dysregulated transcriptional responses to SARS-CoV-2 in the periphery. Nat Commun. (2021) 12:1079. doi: 10.1038/s41467-021-21289-y
43. Ramilo O, Allman W, Chung W, Mejias A, Ardura M, Glaser C, et al. Gene expression patterns in blood leukocytes discriminate patients with acute infections. Blood. (2007) 109:2066–77. doi: 10.1182/blood-2006-02-002477
44. Bhattacharya S, Rosenberg AF, Peterson DR, Grzesik K, Baran AM, Ashton JM, et al. Transcriptomic biomarkers to discriminate bacterial from nonbacterial infection in adults hospitalized with respiratory illness. Sci Rep. (2017) 7:6548. doi: 10.1038/s41598-017-06738-3
45. Suarez NM, Bunsow E, Falsey AR, Walsh EE, Mejias A, Ramilo O. Superiority of transcriptional profiling over procalcitonin for distinguishing bacterial from viral lower respiratory tract infections in hospitalized adults. J Infect Dis. (2015) 212:213–22. doi: 10.1093/infdis/jiv047
46. Välikangas T, Junttila S, Rytkönen KT, Kukkonen-Macchi A, Suomi T, Elo LL. COVID-19-specific transcriptomic signature detectable in blood across multiple cohorts. Front Genet. (2022) 13. doi: 10.3389/fgene.2022.929887
47. Daamen AR, Bachali P, Owen KA, Kingsmore KM, Hubbard EL, Labonte AC, et al. Comprehensive transcriptomic analysis of COVID-19 blood, lung, and airway. Sci Rep. (2021) 11:7052. doi: 10.1038/s41598-021-86002-x
48. Peleman C, Van Coillie S, Ligthart S, Choi SM, De Waele J, Depuydt P, et al. Ferroptosis and pyroptosis signatures in critical COVID-19 patients. Cell Death Differ. (2023) 30:2066–77. doi: 10.1038/s41418-023-01204-2
49. Bednash JS, Kagan VE, Englert JA, Farkas D, Tyurina YY, Tyurin VA, et al. Syrian hamsters as a model of lung injury with SARS-CoV-2 infection: Pathologic, physiologic, and detailed molecular profiling. Transl Res. (2022) 240:1–16. doi: 10.1016/j.trsl.2021.10.007
50. Jankauskas SS, Kansakar U, Sardu C, Varzideh F, Avvisato R, Wang X, et al. COVID-19 causes ferroptosis and oxidative stress in human endothelial cells. Antioxidants (Basel). (2023) 12:326. doi: 10.3390/antiox12020326
51. Vallelian F, Schaer CA, Kaempfer T, Gehrig P, Duerst E, Schoedon G, et al. Glucocorticoid treatment skews human monocyte differentiation into a hemoglobin-clearance phenotype with enhanced heme-iron recycling and antioxidant capacity. Blood. (2010) 116:5347–56. doi: 10.1182/blood-2010-04-277319
52. Scicluna BP, van Vught LA, Zwinderman AH, Wiewel MA, Davenport EE, Burnham KL, et al. Classification of patients with sepsis according to blood genomic endotype: a prospective cohort study. Lancet Respir Med. (2017) 5:816–26. doi: 10.1016/S2213-2600(17)30294-1
53. Tezel G, Yang X, Luo C, Cai J, Kain AD, Powell DW, et al. Hemoglobin expression and regulation in glaucoma: Insights into retinal ganglion cell oxygenation. Invest Ophthalmol Visual Sci. (2010) 51:907–19. doi: 10.1167/iovs.09-4014
54. Wang DH, Jia HM, Zheng X, Xi XM, Zheng Y, Li WX. Attributable mortality of ARDS among critically ill patients with sepsis: a multicenter, retrospective cohort study. BMC Pulm Med. (2024) 24:110. doi: 10.1186/s12890-024-02913-1
55. Maslove DM, Tang B, Shankar-Hari M, Lawler PR, Angus DC, Baillie JK, et al. Redefining critical illness. Nat Med. (2022) 28:1141–8. doi: 10.1038/s41591-022-01843-x
Keywords: COVID - 19, ARDS (acute respiratory disease syndrome), RNA seq analysis, longitudinal analysis, SARS-CoV-2
Citation: Eltobgy M, Johns F, Farkas D, Leuenberger L, Cohen SP, Ho K, Karow S, Swoope G, Pannu S, Horowitz JC, Mallampalli RK, Englert JA and Bednash JS (2024) Longitudinal transcriptomic analysis reveals persistent enrichment of iron homeostasis and erythrocyte function pathways in severe COVID-19 ARDS. Front. Immunol. 15:1397629. doi: 10.3389/fimmu.2024.1397629
Received: 07 March 2024; Accepted: 17 July 2024;
Published: 05 August 2024.
Edited by:
Prasun K. Datta, Tulane University, United StatesReviewed by:
Matthew D. Taylor, Feinstein Institute for Medical Research, United StatesHilary Faust, University of Wisconsin-Madison, United States
Copyright © 2024 Eltobgy, Johns, Farkas, Leuenberger, Cohen, Ho, Karow, Swoope, Pannu, Horowitz, Mallampalli, Englert and Bednash. This is an open-access article distributed under the terms of the Creative Commons Attribution License (CC BY). The use, distribution or reproduction in other forums is permitted, provided the original author(s) and the copyright owner(s) are credited and that the original publication in this journal is cited, in accordance with accepted academic practice. No use, distribution or reproduction is permitted which does not comply with these terms.
*Correspondence: Joseph S. Bednash, am9zZXBoLmJlZG5hc2hAb3N1bWMuZWR1