- 1Department of Oncology, The Central Hospital of Enshi Tujia and Miao Autonomous Prefecture, Enshi Clinical College of Wuhan University, Enshi, China
- 2Department of Radiation Oncology and Medical Oncology, Zhongnan Hospital of Wuhan University, Wuhan, China
- 3Department of Radiology, The First People’s Hospital of Tianmen City, Tianmen, China
- 4Department of Immunology, Wuhan University TaiKang Medical School (School of Basic Medical Sciences), Wuhan, China
Background: Hyperprogressive disease (HPD) is a novel pattern of paradoxically rapid tumor progression, which often leads to early death, mostly in the first 2 months of treatment with immune checkpoint inhibitors (ICIs). Currently, there is no validated biomarker to assess patients at risk of HPD.
Aim: The aim of this study was to systematically evaluate the predictive value of the neutrophil-to-lymphocyte ratio (NLR) in HPD and establish a reliable variable to support clinicians in defining personalized treatment strategies.
Methods: PubMed, Embase, Web of Science, Scopus, and Cochrane Library databases were searched for studies published before 31 December 2023. The Newcastle–Ottawa Scale (NOS) was used to evaluate the quality of eligible studies. The pooled odds ratios (ORs) and 95% confidence intervals (CIs) were calculated using a random-effects or a fixed-effects model to evaluate the association between the NLR and the risk of HPD.
Results: A total of 17 studies with 2,964 patients were included for meta-analysis. The incidence of HPD across different types of tumors ranged from 6.3% to 35.6%. In the pooled analysis of the NLR and HPD, we identified that the NLR significantly associated with the risk of HPD (OR = 0.65; 95% CI: 0.46 to 0.91; p = 0.01) (I2 = 52%, p = 0.007).
Conclusion: In the future, the NLR may serve as a remarkable biomarker for predicting the risk of HPD in clinical practice.
1 Introduction
Cancer ranks as one of the most fatal diseases, with its incidence rapidly growing, and remains a major public health problem in the world (1, 2). Although a variety of treatment methods have been applied to cancer, including surgery, chemotherapy, radiotherapy, and targeted therapies over the past few decades, the prognosis remains unsatisfactory (2). Fortunately, the advent of immunotherapy has revolutionized cancer treatment and has been the cornerstone of success in treating several malignancies (3, 4). Immune checkpoint inhibitors (ICIs) have become a novel and effective therapeutic strategy and the mainstay of treatment for many cancers (5, 6). Despite proven clinical efficacy, a significant proportion of patients do not respond to immunotherapy. A subset of patients who experienced an extremely rapid boost in tumor volume during the treatment with ICIs that far exceeded the pretreatment growth rate, which was defined as hyperprogressive disease (7). Patients with HPD often showed both shorter progression-free survival and overall survival than patients with natural progressive disease (PD) and loss of eligibility for subsequent systemic treatments owing to clinical deterioration (8). Thus, early identification of HPD is very crucial. Multiple studies have reported possible predictive factors of HPD, including serum lactate dehydrogenase above the upper normal limit, the presence of more than two metastatic sites, liver metastases, programmed cell death ligand-1 (PD-L1) positivity, a Royal Marsden Hospital prognostic score of 2 or above, and an Eastern Cooperative Oncology Group Performance Score ≥ 2 (9, 10). Apart from these factors, numerous studies have been conducted to evaluate the neutrophil-to-lymphocyte ratio (NLR) for HPD (11–28). However, these studies have produced different results, and many do not support each other. The predictive value of the NLR for HPD remains controversial. Thus, we performed a systematic review and meta-analysis on this topic to identify the predictive value of HPD.
2 Materials and methods
2.1 Literature search strategy and eligibility criteria
This systematic review and meta-analysis was conducted based on PRISMA guidelines (29). Two investigators (BP and HC) independently searched PubMed, Embase, Web of Science, Scopus, and Cochrane Library databases for studies published before 31 December 2023. The following keywords were used for the search: immunotherapy, programmed death receptor-1, PD-1, programmed cell death-ligand 1, PD-L1, cytotoxic T-lymphocyte-associated protein 4, CTLA-4, ipilimumab, tremelimumab, nivolumab, pembrolizumab, ICI, hyperprogression, and HPD. Additional studies were selected for full-text review by exploring the references and relevant reviews cited in the selected articles. Articles that were published in English with full texts. Finally, we reviewed the list of retrieved articles to select potentially relevant literature and discussed differences in specific studies, which were then resolved with the consensus of both investigators.
The inclusion criteria were as follows: (1) prospective or retrospective studies that reported the characteristics of patients who developed HPD during immunotherapy, regardless of tumor type; (2) all patients were diagnosed as having malignant tumors by biopsy; and (3) the value of the NLR was calculated according to the level of neutrophils and lymphocytes.
The exclusion criteria included the following: (1) duplicate studies, reviews, case reports, letters, reference abstracts, or full text unavailable in English; (2) non-human studies, such as in vitro or animal studies; or (3) studies that did not provide the value of the NLR.
2.2 Data extraction and quality assessment
From each study, BP and HC extracted the name of the study, first author and year of publication, study design, country and institution, underlying malignancy, treatment regimen (PD-1/PD-L1 inhibitor monotherapy or combined with other therapies), drugs, the definition of HPD, total number of HPD cases and groups, and NLR cutoff values. When duplicate publications were identified, we included only the most recent and complete reports of controlled trials.
Quality assessments were assessed using the Newcastle–Ottawa Scale (NOS) (30), which evaluated the study design based on eight aspects: population selection, comparability, and exposure. Studies with final scores of 6 to 9 were regarded as high quality.
2.3 Statistical analysis
The pooled odds ratios (ORs) and 95% confidence intervals (CIs) were calculated using a random-effects or a fixed-effects model to evaluate the association between the NLR and the risk of HPD. We performed the χ2-based Q test to assess interstudy heterogeneity and calculated the I² statistic, representing the percentage of total variability observed due to study heterogeneity. The heterogeneity between studies was considered to indicate a statistically significant difference with heterogeneity p < 0.1 or I2 > 50%. Publication biases were evaluated with funnel plots. Furthermore, Egger’s and Begg’s tests would proceed if required. In addition, each study was individually excluded from the meta-analysis for sensitivity analyses (31). All statistical analyses were performed with Revman ver.5.3.
3 Results
3.1 The characteristics of the included studies
A total of 459 articles were reviewed, and 45 potentially relevant articles were screened after full-text screening. Finally, 18 studies (3,370 patients) fulfilled the inclusion criteria for abstracts and full article reviews (11–28). From the 18 studies, we found that 2 potentially eligible studies (15, 20) were performed in the same group, and only the most recent (20) was included to avoid duplication. Therefore, a total of 17 studies were included for this meta-analysis, with publication dates ranging from 2018 to 2023 (Figure 1).
The sample size enrolled in each trial ranged between 51 and 406. We found 10 studies with PD-1/PD-L1 inhibitor monotherapy, including nivolumab, atezolizumab, pembrolizumab, durvalumab, avelumab, and camrelizumab, while the other 7 studies have PD-1/PD-L1 inhibitor monotherapy or in combination with CTLA-4 or other ICIs. Among all patients included, 1,210 were diagnosed with non-small cell lung cancer (NSCLC), 245 were diagnosed with recurrent and/or metastatic head and neck squamous carcinoma (R/M HNSCC), 283 were diagnosed with liver cancer (LC), and the rest were diagnosed with other cancers (Table 1). The incidence of HPD across different types of tumors ranged from 6.3% to 35.6% in cohorts of patients with NSCLC, 16.7%–27.5% for AGC, 14.4%–18.3% for R/M HNSCC, 10% for breast cancer, and 9.4%–14.5% for liver cancer. Additionally, we found that all identified eligible studies were retrospective.
The definition of HPD varied across the included studies due to the lack of standard criteria. The criteria reported by the studies included in this analysis were adopted (Table 2). Among these studies, Yildirim et al. and Petrova et al. adopted criteria that combined clinical and radiologic parameters (11, 14). Other studies evaluated the acceleration of tumor growth with volume or the sum of the largest diameters based on three imaging time points (pretreatment, baseline, and posttreatment). It should be noted that the definition of tumor growth kinetics (TGK) was also different in the studies of Kim CG (16) and Kim Y (17). Kim CG and colleagues defined TGK as the difference in the sum of the longest diameter of the target lesions according to RECIST 1.1 each month, whereas it was defined by Kim Y and colleagues as the difference in the total tumor volume of the target lesions per unit time. Moreover, the cutoff values of the NLR were different for the enrolled studies, and the value of 3 was most commonly used.
3.2 Relationship between the NLR and HPD in cancers treated with immunotherapy
A total of 17 studies with 2,964 patients treated with immunotherapy provided the NLR values and the number of HPD. The data of ORs and 95% CIs from these studies were combined. In the pooled analysis of the NLR and HPD, we identified that the NLR significantly associated with the risk of HPD (OR = 0.65; 95% CI: 0.46 to 0.91; p = 0.01) (I2 = 52%, p = 0.007) (Figure 2). To detect the source of heterogeneity, we performed subset analyses on certain clinical factors that might influence the final result, such as the treatment regimen (Figure 3).
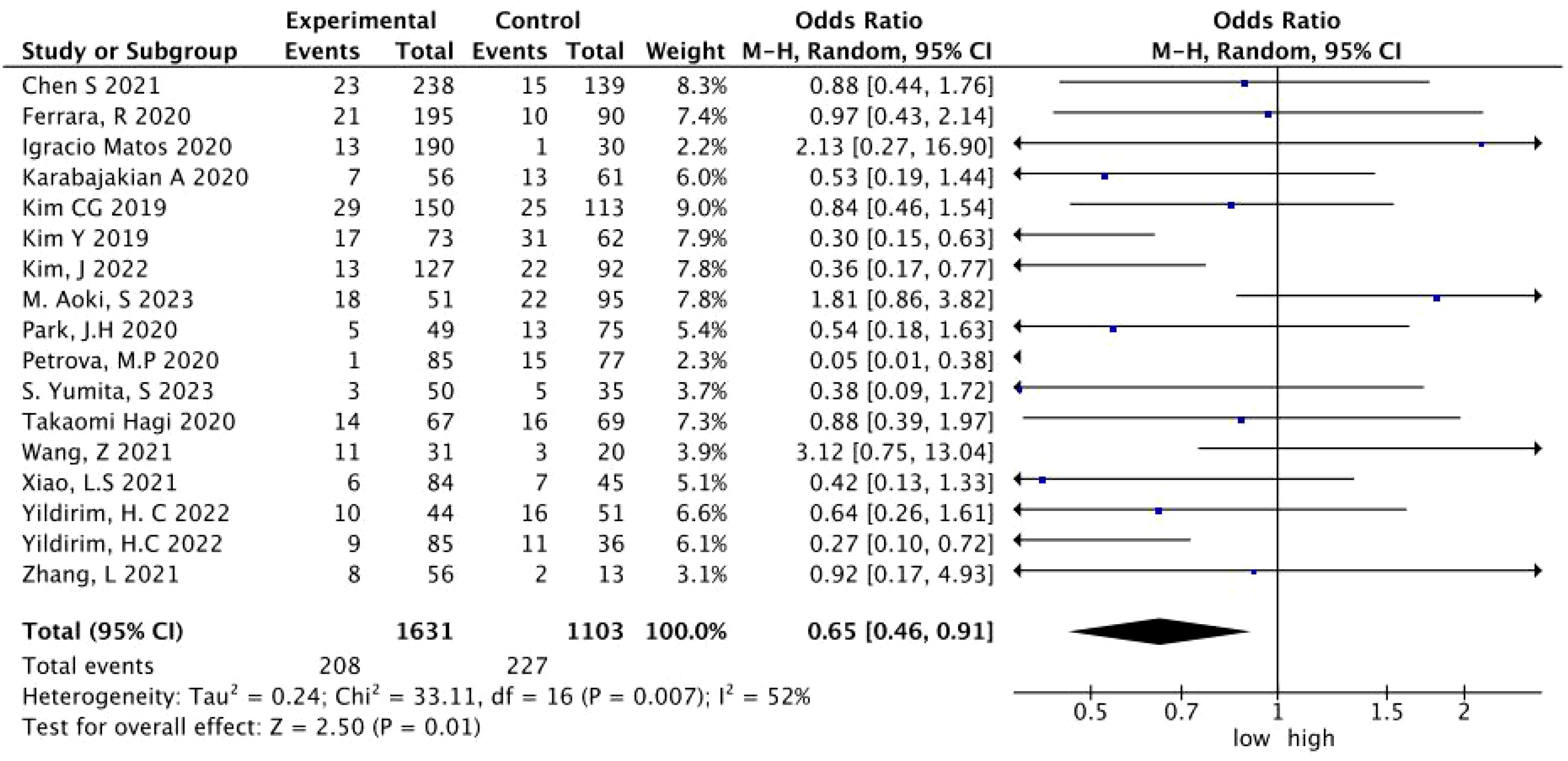
Figure 2. A forest plot of the association between the NLR and HPD in patients treated with immunotherapy. NLR, neutrophil-to-lymphocyte ratio; HPD, hyperprogressive disease; CI, confidence interval.
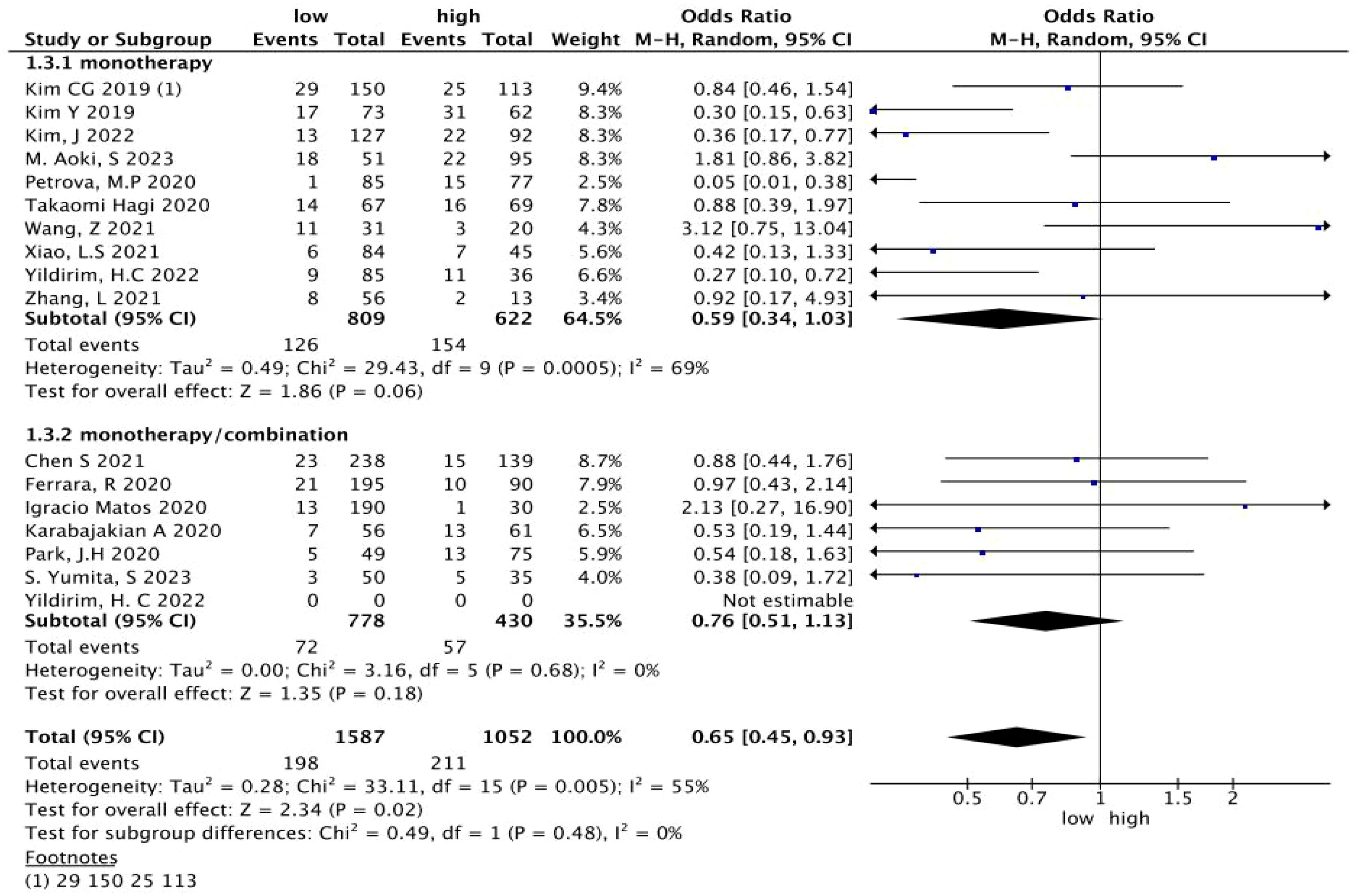
Figure 3. A subgroup analysis of the relationship between the NLR and HPD in patients treated with immunotherapy. NLR, neutrophil-to-lymphocyte ratio; HPD, hyperprogressive disease; CI, confidence interval.
3.3 Sensitivity analysis and publication bias
We found moderate heterogeneity among studies (p = 0.007; I2 = 52%). Therefore, we performed a sensitivity analysis on all included studies. Each study was individually excluded from the meta-analysis to evaluate the effect of each study on the pooled OR value. The sensitivity analysis results showed that the combined ORs in our meta-analysis were robust (Supplementary Figures 1-17). As can be seen from Figure 4, all included studies were symmetrically distributed on the left and right sides of the inverted funnel plot, suggesting that no potential publication bias was identified.
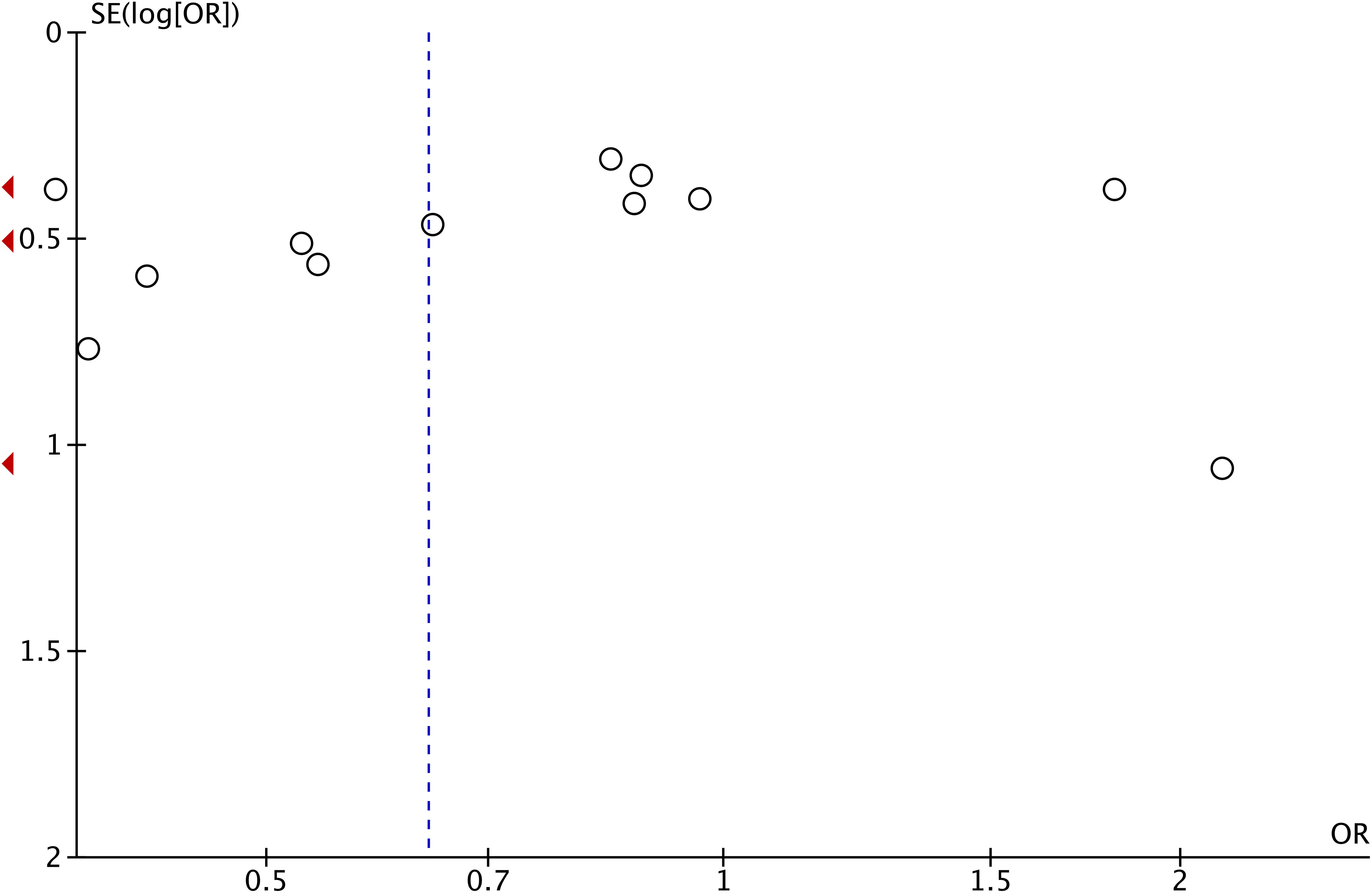
Figure 4. A funnel plot of meta-analysis of the association of the NLR with HPD. NLR, neutrophil-to-lymphocyte ratio; HPD, hyperprogressive disease.
4 Discussion
HPD is a paradoxical acceleration of tumor growth phenomenon, which often leads to early death commonly in the first 2 months of treatment with ICIs (32). The incidence of HPD after treatment with ICIs ranged from 1.2% to 43.1% (10), while in our meta-analysis, the pooled incidence of HPD varied from 6.3% to 35.6%, which may be related to the inclusion of different tumor types and different assessment methods for HPD in previous studies (33). Multiple factors are thought to contribute to the occurrence of HPD, including clinical features, genetic features, and tumor immune microenvironment characteristics (9, 10, 34, 35). The mechanism of HPD occurrence and the exact causes are poorly understood. In some preclinical models, ICI causes HPD. Recently, Li et al. found a triple-high gene signature score (IFNγ–FGF2–β-catenin) associated with HPD in patients and revealed crosstalk between metabolic, immunogenic, and oncogenic pathways underlying HPD associated with immunotherapy (36).
HPD is not unique to immunotherapy (37), the incidence of which will increase. It also occurs in conventional chemotherapy and targeted therapies. The overall survival of patients with HPD was significantly shortened, suggesting that once HPD occurs, it can seriously affect the prognosis of patients (38, 39). Although some researchers caution that HPD represents the natural disease course of a subset of patients with aggressive cancers (40–42), most studies now support that patients with HPD had a worse outcome than patients with so-called “conventional” progression (43). Once these patients develop HPD after receiving immunotherapy, they are often deprived of their chance to receive subsequent treatment (44). It is a particular phenomenon that patients cannot benefit from immunotherapy because of the lack of prediction methods (45). A recent study identifies SAA1 and specific metabolomic signatures as potential predictive biomarkers for HPD in patients undergoing immunotherapy across various cancers (46). However, metabolic biomarkers are preferred for the dynamic monitoring of HPD due to their susceptibility to fluctuations caused by metabolic disorders; they will take a long time and may delay HPD diagnosis. Furthermore, many immunohistochemistry specimens cannot be obtained due to various reasons. In this scenario, it is important to identify HPD biomarkers for selection before ICI therapy.
The NLR is defined as the absolute neutrophil count divided by the absolute lymphocyte count. It is a practical, easy acquisition, and low-cost marker of the immune system’s inflammatory response (47). In the oncological context, an elevated NLR is often observed in individuals presenting with advanced or aggressive forms of cancer (48). This may be due to tumor cells secreting granulocyte colony-stimulating factor (G-CSF) and/or granulocyte monocyte colony-stimulating factor (GM-CSF), which not only are direct growth factors for tumor cells, but also may lead to increased NLR in patients. Thus, bone marrow hematopoiesis shifts from the lymphocyte lineage to the granulocyte lineage (49, 50). Accumulating evidence has revealed that the NLR is associated with tumor malignant degree invasive biological features and the efficacy of immunotherapy (51). In the era of immunotherapy, the NLR often represents a significant, feasible prognostic factor (52, 53). An evidence synthesis from 30 meta-analyses indicated that the NLR is associated with poor outcomes in patients with cancer receiving immunotherapy (54). There may be a certain correlation, and a similar situation exists in HPD. An increasing number of researchers have speculated that the NLR may be a biomarker for predicting the occurrence of HPD. However, inconsistent results have emerged from multiple studies. For example, original cohort studies conducted by Petrova et al. (14), Karabajakian et al. (19), Kim et al. (21), Takahashi et al. (55), Milla et al. (56), and Maesaka et al. (57) have concluded that the risk of HPD occurrence will increase with elevated NLR, which can be used as a marker for HPD. Our study was in line with this conclusion. We found that patients with high NLR had a higher probability of developing HPD after ICI treatment, and the difference was statistically significant (OR = 0.65; 95% CI: 0.46 to 0.891; p = 0.01). However, the other subset of original cohort studies had been conducted by Champiat et al. (7), Kim et al. (8), Zhang et al. (12), Xiao et al. (13), Chen et al. (18), Ferrara et al. (20), Wang et al. (22), Park et al. (23), and Choi et al. (58), and their colleagues showed that elevated NLR was not significantly associated with the occurrence of HPD. Meanwhile, two meta-analyses had drawn similar conclusions (10, 59). Numerous factors may account for these results. First, some studies included a small sample size. Second, different researchers included different cancer types; for instance, Liu et al. only included solid tumors, and we added hematologic tumors to our study. Last but not least, there is currently no consensus cutoff value of the NLR for predicting the occurrence of HPD. Future studies with larger sample sizes and an optimal cutoff value of the NLR to validate the results of this study are needed.
The exact mechanism between an elevated NLR and HPD has yet to be elucidated. The possible mechanisms are as follows: first, tumor cells can induce bone marrow to produce more neutrophils into the blood and promote tumor progression through multiple immunosuppressive pathways. Neutrophils can interact with other immune cells, such as macrophages and myeloid-derived suppressor cells (MDSCs), to create an immunosuppressive environment. These interactions can lead to the polarization of macrophages towards an M2 phenotype, which is immunosuppressive and supports tumor progression (60). Second, a high NLR often indicates a greater presence of neutrophils, which induce angiogenesis, tumor growth, and metastasis by secreting tumor growth factors, cytokines, and chemokines, such as transforming growth factor P (TGP-P), vascular endothelial growth factor (VEGF), interleukin-6 (IL-6), interleukin-8 (IL-8), interleukin-12 (IL-12), and stromal metalloproteinases (61–63). Third, neutrophils are part of the innate immune system and can contribute to an inflammatory environment that supports tumor progression. An elevated NLR may signal a pro-inflammatory state within the tumor (64). Additionally, neutrophils have been shown to participate in immune evasion strategies employed by tumors. They can release factors that inhibit T-cell function, such as arginase and reactive oxygen species (ROS), which can suppress the activity of cytotoxic T cells that are essential for antitumor immunity (65, 66). Last but not the least, in the latest study, Ng et al. revealed that tumor-reprogrammed neutrophils converge immature and mature neutrophils to a terminally differentiated T3 state, which promotes angiogenesis by localizing in hypoxic–glycolytic niches and enhancing blood vessel formation in the tumor microenvironment, thus promoting the occurrence and development of tumors (67). At the same time, lymphocytes play a vital role in antitumor immunity and are the leading performers of immune functions in the antitumor process. T lymphocytes can recognize and kill tumor cells, thus inhibiting tumor proliferation. Lymphocytes also participate in antitumor immunity by releasing cytokines (68). Since ICIs depend on the suppressive signaling function of T lymphocytes, a decrease in lymphocyte counts decreases the antitumor immune response and affects the efficacy of ICIs. Increased lymphocyte infiltration in the tumor immune microenvironment is associated with better prognosis and immunotherapy efficacy (69, 70).While the number of lymphocytes is insufficient, it leads to an inadequate immune response to the tumor, which promotes tumor progression and metastasis. Increased neutrophils suggest a poor prognosis, while tumor-associated lymphocytes are associated with a better prognosis. The NLR can measure the immune system’s inflammatory state, reflecting the balance between tumor protection and destruction. Therefore, it is inferred that the NLR can be used as a predictor for the occurrence of HPD.
Admittedly, there are several limitations in our study. The meta-analysis included 17 studies, all of which were retrospective studies, and the type of studies in this category was somewhat confounded with bias. It is insufficient to reveal a causal relationship between specific indicators or clinical features and HPD, yet such high-quality prospective studies examining the interaction of these indicators with HPD events are still lacking. At the same time, the assessment criteria for HPD, tumor growth kinetic indicators, and the definition of HPD vary, which may affect the consolidation of results across studies and lead to selection and reporting bias. Thus, we should develop and validate a standardized HPD definition as early as possible in further studies and conduct more high-quality, large-scale multi-center studies to confirm the predictive value of the NLR for the occurrence of HPD and provide strong evidence for clinical decision-making.
Given the limitations of ICI due to HPD incidence, there is an urgent need for reliable biomarkers to predict the occurrence of HPD and the efficacy of ICI (71). Although radiomic features can help identify the features of HPD after immunotherapy has been reported (72), the definition of HPD based on TGR ratio or TKR ratio may preclude its clinical use in many patients due to the lack of pre-baseline imaging data. Such complexity may be the barrier in incorporating the HPD concept into clinical practice. It is imperative for us to search a simpler and more routinely available tool to assess HPD. To a certain extent, the NLR can compensate for the deficiency of imaging examinations, achieve dynamic monitoring, and identify HPD early and accurately, which may reduce the incidence of HPD. For patients who have developed HPD, the combination with other ICIs, radiotherapy, chemotherapy, and targeted therapies may provide a synergistic antitumor effect and become a reliable approach to treating HPD. The study of Park and colleagues suggested that patients who experienced HPD with ICIs should not be excluded from the subsequent salvage chemotherapy treatments, owing to potentially enriched therapeutic benefits of post-ICI chemotherapy in R/M-HNSCC (23). Clinical studies of multiple combination treatment options are currently being explored, and the results of these studies are expected to bring new hope for the clinical treatment of HPD.
In summary, this systematic review and meta-analysis revealed that the NLR might be an easy, low-cost, and readily available method and biomarker to predict the occurrence of HPD. It can be used to identify HPD early and take measures to reduce the incidence of HPD. The result is of great importance for evidence-based clinical decision-making in oncology practice, where immunotherapy has become a mainstay of cancer medical therapy. Therefore, before patients receive ICI therapy, combining routine clinical examinations with an evaluation of NLR is needed to provide them with a safe and optimal treatment option.
Data availability statement
The original contributions presented in the study are included in the article/Supplementary Material. Further inquiries can be directed to the corresponding author.
Author contributions
BP: Conceptualization, Funding acquisition, Project administration, Writing – original draft. JZ: Writing – review & editing. LL: Writing – review & editing. HC: Conceptualization, Data curation, Project administration, Software, Writing – original draft.
Funding
The author(s) declare financial support was received for the research, authorship, and/or publication of this article. This work was supported by the Natural Science Foundation of Enshi Tujia and Miao Autonomous Prefecture Government (E20170002) and the Beijing Bethune Public Welfare Foundation (2023-YJ-041-J-005).
Conflict of interest
The authors declare that the research was conducted in the absence of any commercial or financial relationships that could be construed as a potential conflict of interest.
Publisher’s note
All claims expressed in this article are solely those of the authors and do not necessarily represent those of their affiliated organizations, or those of the publisher, the editors and the reviewers. Any product that may be evaluated in this article, or claim that may be made by its manufacturer, is not guaranteed or endorsed by the publisher.
Supplementary material
The Supplementary Material for this article can be found online at: https://www.frontiersin.org/articles/10.3389/fimmu.2024.1393925/full#supplementary-material
References
1. Siegel RL, Giaquinto AN, Jemal A. Cancer statistics, 2024. CA Cancer J Clin. (2024) 74:12–49. doi: 10.3322/caac.21820
2. Han B, Zheng R, Zeng H, Wang S, Sun K, Chen R, et al. Cancer incidence and mortality in China, 2022. J Natl Cancer Cent. (2024) 4:47–53. doi: 10.1016/j.jncc.2024.01.006
3. Noori M, Jafari-Raddani F, Davoodi-Moghaddam Z, Delshad M, Safiri S, Bashash D, et al. Immune checkpoint inhibitors in gastrointestinal Malignancies: an Umbrella review. Cancer Cell Int. (2024) 24:10. doi: 10.1186/s12935-023-03183-3
4. Olsen TA, Zhuang TZ, Caulfield S, Martini DJ, Brown JT, Carthon BC, et al. Advances in knowledge and management of immune-related adverse events in cancer immunotherapy. Front Endocrinol (Lausanne). (2022) 13:779915. doi: 10.3389/fendo.2022.779915
5. Suijkerbuijk KPM, van Eijs MJM, van Wijk F, Eggermont AMM. Clinical and translational attributes of immune-related adverse events. Nat Cancer. (2024) 5(4):557–71. doi: 10.1038/s43018-024-00730-3
6. Xin Yu J, Hubbard-Lucey VM, Tang J. Immuno-oncology drug development goes global. Nat Rev Drug Discovery. (2019) 18:899–900. doi: 10.1038/d41573-019-00167-9
7. Champiat S, Dercle L, Ammari S, Massard C, Hollebecque A, Postel-Vinay S, et al. Hyperprogressive disease is a new pattern of progression in cancer patients treated by anti-PD-1/PD-L1. Clin Cancer Res. (2017) 23:1920–8. doi: 10.1158/1078-0432.CCR-16-1741
8. Kim CG, Hong M, Jeung HC, Lee G, Chung HC, Rha SY, et al. Hyperprogressive disease during PD-1 blockade in patients with advanced gastric cancer. Eur J Cancer. (2022) 172:387–99. doi: 10.1016/j.ejca.2022.05.042
9. Kim JY, Lee KH, Kang J, Borcoman E, Saada-Bouzid E, Kronbichler A, et al. Hyperprogressive disease during anti-PD-1 (PDCD1)/PD-L1 (CD274) therapy: A systematic review and meta-analysis. Cancers (Basel). (2019) 11:1699. doi: 10.3390/cancers11111699
10. Zhao Z, Bian J, Zhang J, Zhang T, Lu X. Hyperprogressive disease in patients suffering from solid Malignancies treated by immune checkpoint inhibitors: A systematic review and meta-analysis. Front Oncol. (2022) 12:843707. doi: 10.3389/fonc.2022.843707
11. Yildirim HC, Guven DC, Aktepe OH, Taban H, Yilmaz F, Yasar S, et al. Blood based biomarkers as predictive factors for hyperprogressive disease. J Clin Med. (2022) 11(17):5171. doi: 10.3390/jcm11175171
12. Zhang L, Wu L, Chen Q, Zhang B, Liu J, Liu S, et al. Predicting hyperprogressive disease in patients with advanced hepatocellular carcinoma treated with anti-programmed cell death 1 therapy. EClinicalMedicine. (2021) 31:100673. doi: 10.1016/j.eclinm.2020.100673
13. Xiao LS, Li QM, Hu CY, Cui H, Hong C, Huang CY, et al. Lung metastasis and lymph node metastasis are risk factors for hyperprogressive disease in primary liver cancer patients treated with immune checkpoint inhibitors. Ann Palliat Med. (2021) 10:11244–54. doi: 10.21037/apm-21-2023
14. Petrova MP, Donev IS, Radanova MA, Eneva MI, Dimitrova EG, Valchev GN, et al. Sarcopenia and high NLR are associated with the development of hyperprogressive disease after second-line pembrolizumab in patients with non-small-cell lung cancer. Clin Exp Immunol. (2020) 202:353–62. doi: 10.1111/cei.13505
15. Ferrara R, Mezquita L, Texier M, Lahmar J, Audigier-Valette C, Tessonnier L, et al. Hyperprogressive disease in patients with advanced non-small cell lung cancer treated with PD-1/PD-L1 inhibitors or with single-agent chemotherapy. JAMA Oncol. (2018) 4:1543–52. doi: 10.1001/jamaoncol.2018.3676
16. Kim CG, Kim KH, Pyo KH, Xin CF, Hong MH, Ahn BC, et al. Hyperprogressive disease during PD-1/PD-L1 blockade in patients with non-small-cell lung cancer. Ann Oncol. (2019) 30:1104–13. doi: 10.1093/annonc/mdz123
17. Kim Y, Kim CH, Lee HY, Lee SH, Kim HS, Lee S, et al. Comprehensive clinical and genetic characterization of hyperprogression based on volumetry in advanced non-small cell lung cancer treated with immune checkpoint inhibitor. J Thorac Oncol. (2019) 14:1608–18. doi: 10.1016/j.jtho.2019.05.033
18. Chen S, Gou M, Yan H, Fan M, Pan Y, Fan R, et al. Hyperprogressive disease caused by PD-1 inhibitors for the treatment of pan-cancer. Dis Markers. (2021) 2021:6639366. doi: 10.1155/2021/6639366
19. Karabajakian A, Garrivier T, Crozes C, Gadot N, Blay JY, Bérard F, et al. Hyperprogression and impact of tumor growth kinetics after PD1/PDL1 inhibition in head and neck squamous cell carcinoma. Oncotarget. (2020) 11:1618–28. doi: 10.18632/oncotarget.v11i18
20. Ferrara R, Mezquita L, Texier M, Lahmar J, Audigier-Valette C, Tessonnier L, et al. Comparison of fast-progression, hyperprogressive disease, and early deaths in advanced non-small-cell lung cancer treated with PD-1/PD-L1 inhibitors or chemotherapy. JCO Precis Oncol. (2020) 4:829–40. doi: 10.1200/PO.20.00021
21. Kim J, Kim T, Jang TW, Kang H, Kim MH, Yoon SH, et al. Clinical outcomes of hyperprogression based on volumetry in non-small cell lung cancer after immune checkpoint inhibitor treatment. Thorac Cancer. (2022) 13:2170–9. doi: 10.1111/1759-7714.14539
22. Wang Z, Liu C, Bai Y, Zhao X, Cui L, Peng Z, et al. Redefine hyperprogressive disease during treatment with immune-checkpoint inhibitors in patients with gastrointestinal cancer. Front Oncol. (2021) 11:761110. doi: 10.3389/fonc.2021.761110
23. Park JH, Chun SH, Lee YG, Chang H, Lee KW, Kim HR, et al. Hyperprogressive disease and its clinical impact in patients with recurrent and/or metastatic head and neck squamous cell carcinoma treated with immune-checkpoint inhibitors: Korean cancer study group HN 18-12. J Cancer Res Clin Oncol. (2020) 146:3359–69. doi: 10.1007/s00432-020-03316-5
24. Aoki M, Kadowaki S, Takahashi N, Suzuki T, Oshima K, Ando T, et al. Pattern of disease progression during third-line or later chemotherapy with nivolumab associated with poor prognosis in advanced gastric cancer: a multicenter retrospective study in Japan. Gastric Cancer. (2023) 26:132–44. doi: 10.1007/s10120-022-01349-y
25. Yumita S, Ogasawara S, Nakagawa M, Maruta S, Okubo T, Itokawa N, et al. Hyperprogressive disease during atezolizumab plus bevacizumab treatment in patients with advanced hepatocellular carcinoma from Japanese real-world practice. BMC Gastroenterol. (2023) 23:101. doi: 10.1186/s12876-023-02731-5
26. Yildirim HC, Guven DC, Aktepe OH, Taban H, Yilmaz F, Yasar S, et al. Differences between hyperprogressive disease and progressive disease in patients receiving immunotherapy. EJMO. (2022) 6:59–63. doi: 10.14744/ejmo.2022.78280
27. Matos I, Martin-Liberal J, García-Ruiz A, Hierro C, Ochoa de Olza M, Viaplana C, et al. Capturing hyperprogressive disease with immune-checkpoint inhibitors using RECIST 1.1 criteria. Clin Cancer Res. (2020) 26:1846–55. doi: 10.1158/1078-0432.CCR-19-2226
28. Hagi T, Kurokawa Y, Kawabata R, Omori T, Matsuyama J, Fujitani K, et al. Multicentre biomarker cohort study on the efficacy of nivolumab treatment for gastric cancer. Br J Cancer. (2020) 123:965–72. doi: 10.1038/s41416-020-0975-7
29. Moher D, Liberati A, Tetzlaff J, Altman DG. Preferred reporting items for systematic reviews and meta-analyses: the PRISMA statement. PloS Med. (2009) 6:e1000097. doi: 10.1371/journal.pmed.1000097
30. Wells GA, Shea B, Connell DO, et al. The Newcastle-Ottawa Scale (NOS) for assessing the quality of nonrandomised studies in meta-analyses. Available online at: http://www.ohri.ca/programs/clinical_epidemiology/oxford.asp (Accessed 15 Jan 2020).
32. Choi YJ, Kim T, Kim EY, Lee SH, Kwon DS, Chang YS. Prediction model for hyperprogressive disease in non-small cell lung cancer treated with immune checkpoint inhibitors. Thorac Cancer. (2020) 11:2793–803. doi: 10.1111/1759-7714.13594
33. Kim MJ, Hong SPD, Park Y, Chae YK. Incidence of immunotherapy-related hyperprogressive disease (HPD) across HPD definitions and cancer types in observational studies: A systematic review and meta-analysis. Cancer Med. (2024) 13:e6970. doi: 10.1002/cam4.v13.3
34. Zhang L, Feng J, Kuang T, Chai D, Qiu Z, Deng W, et al. Blood biomarkers predict outcomes in patients with hepatocellular carcinoma treated with immune checkpoint Inhibitors: A pooled analysis of 44 retrospective sudies. Int Immunopharmacol. (2023) 118:110019. doi: 10.1016/j.intimp.2023.110019
35. Gong C, Zhang W, Sun Y, Shou J, Jiang Z, Liu T, et al. Exploration of the immunogenetic landscape of hyperprogressive disease after combined immunotherapy in cancer patients. iScience. (2023) 26:106720. doi: 10.1016/j.isci.2023.106720
36. Li G, Choi JE, Kryczek I, Sun Y, Liao P, Li S, et al. Intersection of immune and oncometabolic pathways drives cancer hyperprogression during immunotherapy. Cancer Cell. (2023) 41:304–322.e7. doi: 10.1016/j.ccell.2022.12.008
37. Fournier M, Mortier L, Dereure O, Dalac S, Oriano B, Dalle S, et al. Hyperprogression in advanced melanoma is not restricted to immunotherapy. Eur J Cancer. (2023) 193:113289. doi: 10.1016/j.ejca.2023.113289
38. Hwang I, Park I, Yoon SK, Lee JL. Hyperprogressive disease in patients with urothelial carcinoma or renal cell carcinoma treated with PD-1/PD-L1 inhibitors. Clin Genitourin Cancer. (2020) 18:e122–e33. doi: 10.1016/j.clgc.2019.09.009
39. Petrioli R, Mazzei MA, Giorgi S, Cesqui E, Gentili F, Francini G, et al. Hyperprogressive disease in advanced cancer patients treated with nivolumab: a case series study. Anticancer Drugs. (2020) 31:190–5. doi: 10.1097/CAD.0000000000000864
40. Trotier D, Grover P, Park C, McGrath JE, Wise-Draper T. 974P Genetic and molecular analysis of solid tumors with hyperprogressive disease after treatment with immunotherapy. Ann Oncol. (2021) 32:S836–S7. doi: 10.1016/j.annonc.2021.08.1358
41. Ferrara R, Facchinetti F, Calareso G, Kasraoui I, Signorelli D, Proto C, et al. 1278P Hyperprogressive disease (HPD) upon first-line PD-1/PD-L1 inhibitors (ICI) as single agent or in combination with platinum-based chemotherapy in non-small cell lung cancer (NSCLC) patients (pts). Ann Oncol. (2020) 31. doi: 10.1016/j.annonc.2020.08.1592
42. Kang YK, Reck M, Nghiem P, Feng Y, Plautz G, Kim HR, et al. Assessment of hyperprogression versus the natural course of disease development with nivolumab with or without ipilimumab versus placebo in phase III, randomized, controlled trials. J Immunother Cancer. (2022) 10:e004273. doi: 10.1136/jitc-2021-004273
43. Park HJ, Kim KW, Won SE, Yoon S, Chae YK, Tirumani SH, et al. Definition, incidence, and challenges for assessment of hyperprogressive disease during cancer treatment with immune checkpoint inhibitors: A systematic review and meta-analysis. JAMA Netw Open. (2021) 4:e211136. doi: 10.1001/jamanetworkopen.2021.1136
44. Kim CG, Kim C, Yoon SE, Kim KH, Choi SJ, Kang B, et al. Hyperprogressive disease during PD-1 blockade in patients with advanced hepatocellular carcinoma. J Hepatol. (2021) 74:350–9. doi: 10.1016/j.jhep.2020.08.010
45. Long Y, Yang W, Bai Y, Tao H, Zhang F, Wang L, et al. Prediction model for hyperprogressive disease in patients with advanced solid tumors received immune-checkpoint inhibitors: a pan-cancer study. Cancer Cell Int. (2023) 23:224. doi: 10.1186/s12935-023-03070-x
46. Wang X, Zhang L, Liao L, Li N, Tang T, Sun J, et al. SAA1 and metabolomic signatures predict hyperprogression with immunotherapy in pan cancers. Clin Transl Med. (2024) 14:e1624. doi: 10.1002/ctm2.v14.3
47. Schmid P, Adams S, Rugo HS, Schneeweiss A, Barrios CH, Iwata H, et al. Atezolizumab and nab-paclitaxel in advanced triple-negative breast cancer. N Engl J Med. (2018) 379:2108–21. doi: 10.1056/NEJMoa1809615
48. Guthrie GJ, Charles KA, Roxburgh CS, Horgan PG, McMillan DC, Clarke SJ, et al. The systemic inflammation-based neutrophil-lymphocyte ratio: experience in patients with cancer. Crit Rev Oncol Hematol. (2013) 88:218–30. doi: 10.1016/j.critrevonc.2013.03.010
49. He K, Liu X, Hoffman RD, Shi RZ, Lv GY, Gao JL, et al. G-CSF/GM-CSF-induced hematopoietic dysregulation in the progression of solid tumors. FEBS Open Bio. (2022) 12:1268–85. doi: 10.1002/2211-5463.13445
50. Kumar A, Taghi Khani A, Sanchez Ortiz A, Swaminathan S. GM-CSF: A double-edged sword in cancer immunotherapy. Front Immunol. (2022) 13:901277. doi: 10.3389/fimmu.2022.901277
51. Shinko D, Diakos CI, Clarke SJ, Charles KA. Cancer-related systemic inflammation: the challenges and therapeutic opportunities for personalized medicine. Clin Pharmacol Ther. (2017) 102:599–610. doi: 10.1002/cpt.v102.4
52. Bigot F, Castanon E, Baldini C, Hollebecque A, Carmona A, Postel-Vinay S, et al. Prospective validation of a prognostic score for patients in immunotherapy phase I trials: The Gustave Roussy Immune Score (GRIm-Score). Eur J Cancer. (2017) 84:212–8. doi: 10.1016/j.ejca.2017.07.027
53. Arkenau HT, Barriuso J, Olmos D, Ang JE, de Bono J, Judson I, et al. Prospective validation of a prognostic score to improve patient selection for oncology phase I trials. J Clin Oncol. (2009) 27:2692–6. doi: 10.1200/JCO.2008.19.5081
54. Wang H, Yang R, Liu D, Li W. Association of pretreatment neutrophil-to-lymphocyte ratio with clinical outcomes in cancer immunotherapy: An evidence synthesis from 30 meta-analyses. Int Immunopharmacol. (2024) 132:111936. doi: 10.1016/j.intimp.2024.111936
55. Takahashi R, Shibata E, Higashiyama T, Kamei T, Tada A, Ishigaki H, et al. Neutrophil-to-Lymphocyte ratio as a predictive factor for hyperprogressive disease in NSCLC patients treated with immune checkpoint inhibitor. Ann Oncol. (2019) 30. doi: 10.1093/annonc/mdz438.019
56. Milla P, Mariyana E, Jeliazko LA, Jeliazko A, Nikolay VC, Eleonora, et al. High neutrophil to lymphocyte ratio as a predictor for pembrolizumab as a second line. J Clin Oncol. (2020) 38. doi: 10.1200/jco.2020.38.1_suppl.e21546
57. Maesaka K, Sakamori R, Yamada R, Tahata Y, Imai Y, Ohkawa K, et al. Hyperprogressive disease in patients with unresectable hepatocellular carcinoma receiving atezolizumab plus bevacizumab therapy. Hepatol Res. (2022) 52:298–307. doi: 10.1111/hepr.13741
58. Choi JY, Park JY, Lee SW, Kim JH, Kim YM, Kim YT, et al. Factors predicting hyperprogression in patients with advanced ovarian cancer receiving anti-programmed cell death 1-therapy. Gynecologic Oncol. (2020) 159. doi: 10.1016/j.ygyno.2020.05.218
59. Liu J, Wu Q, Wu SJ, Xie X. Investigation on potential biomarkers of hyperprogressive disease (HPD) triggered by immune checkpoint inhibitors (ICIs). Clin Transl Oncol. (2021) 23:1782–93. doi: 10.1007/s12094-021-02579-9
60. Li W, Zhang X, Chen Y, Xie Y, Liu J, Feng Q, et al. G-CSF is a key modulator of MDSC and could be a potential therapeutic target in colitis-associated colorectal cancers. Protein Cell. (2016) 7:130–40. doi: 10.1007/s13238-015-0237-2
61. Bausch D, Pausch T, Krauss T, Hopt UT, Fernandez-del-Castillo C, Warshaw AL, et al. Neutrophil granulocyte derived MMP-9 is a VEGF independent functional component of the angiogenic switch in pancreatic ductal adenocarcinoma. Angiogenesis. (2011) 14:235–43. doi: 10.1007/s10456-011-9207-3
62. Templeton AJ, McNamara MG, Seruga B, Vera-Badillo FE, Aneja P. Prognostic role of neutrophil-to-lymphocyte ratio in solid tumors: a systematic review and meta-analysis. J Natl Cancer Inst. (2014) 106:dju124. doi: 10.1093/jnci/dju124
63. Grivennikov SI, Greten FR, Karin M. Immunity, inflammation, and cancer. Cell. (2010) 140:883–99. doi: 10.1016/j.cell.2010.01.025
64. Fridlender ZG, Sun J, Kim S, Worthen GS, Albelda SM. Polarization of tumor-associated neutrophil phenotype by TGF-beta: “N1” versus “N2” TAN. Cancer Cell. (2009) 16:183–94. doi: 10.1016/j.ccr.2009.06.017
65. Si Y, Merz SF, Jansen P, Wang B, Bruderek K, Altenhoff P, et al. Multidimensional imaging provides evidence for down-regulation of T cell effector function by MDSC in human cancer tissue. Sci Immunol. (2019) 4:eaaw9159. doi: 10.1126/sciimmunol.aaw9159
66. Geh D, Leslie J, Rumney R, Reeves HL, Bird TG, Mann DA, et al. Neutrophils as potential therapeutic targets in hepatocellular carcinoma. Nat Rev Gastroenterol Hepatol. (2022) 19:257–73. doi: 10.1038/s41575-021-00568-5
67. Ng MSF, Kwok I, Tan L, Shi C, Cerezo-Wallis D, Tan Y, et al. Deterministic reprogramming of neutrophils within tumors. Science. (2024) 383:eadf6493. doi: 10.1126/science.adf6493
68. Stanton SE, Disis ML. Clinical significance of tumor-infiltrating lymphocytes in breast cancer. J Immunother Cancer. (2016) 4:59. doi: 10.1186/s40425-016-0165-6
69. Jansen CS, Prokhnevska N, Master VA, Sanda MG, Carlisle JW, Bilen MA, et al. An intra-tumoral niche maintains and differentiates stem-like CD8 T cells. Nature. (2019) 576:465–70. doi: 10.1038/s41586-019-1836-5
70. Gooden MJ, de Bock GH, Leffers N, Daemen T, Nijman HW. The prognostic influence of tumour-infiltrating lymphocytes in cancer: a systematic review with meta-analysis. Br J Cancer. (2011) 105:93–103. doi: 10.1038/bjc.2011.189
71. Wang X, Wang F, Zhong M, Yarden Y, Fu L. The biomarkers of hyperprogressive disease in PD-1/PD-L1 blockage therapy. Mol Cancer. (2020) 19:81. doi: 10.1186/s12943-020-01200-x
Keywords: hyperprogressive disease, immunotherapy, immune checkpoint inhibitors, meta-analysis, neutrophil-to-lymphocyte ratio
Citation: Pei B, Zhang J, Lai L and Chen H (2024) Neutrophil-to-lymphocyte ratio as a predictive biomarker for hyperprogressive disease mediated by immune checkpoint inhibitors: a systematic review and meta-analysis. Front. Immunol. 15:1393925. doi: 10.3389/fimmu.2024.1393925
Received: 29 February 2024; Accepted: 27 August 2024;
Published: 23 September 2024.
Edited by:
Petra Huehnchen, Charité University Medicine Berlin, GermanyReviewed by:
Yejun Tan, University of Minnesota Health Twin Cities, United StatesHester Doyle, Yale University, United States
Copyright © 2024 Pei, Zhang, Lai and Chen. This is an open-access article distributed under the terms of the Creative Commons Attribution License (CC BY). The use, distribution or reproduction in other forums is permitted, provided the original author(s) and the copyright owner(s) are credited and that the original publication in this journal is cited, in accordance with accepted academic practice. No use, distribution or reproduction is permitted which does not comply with these terms.
*Correspondence: Hui Chen, Y2gxMzk4Njk0Mjc4OEAxNjMuY29t