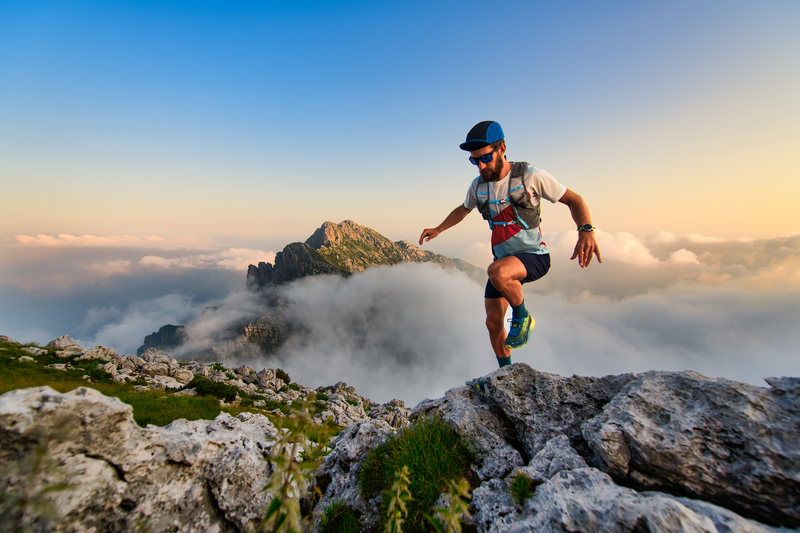
94% of researchers rate our articles as excellent or good
Learn more about the work of our research integrity team to safeguard the quality of each article we publish.
Find out more
EDITORIAL article
Front. Immunol. , 20 February 2024
Sec. T Cell Biology
Volume 15 - 2024 | https://doi.org/10.3389/fimmu.2024.1377259
This article is part of the Research Topic Quantification and Prediction of T-cell Cross-reactivity Through Experimental and Computational Methods View all 8 articles
Editorial on the Research Topic
Quantification and prediction of T-cell cross-reactivity through experimental and computational methods
T-cell receptor (TCR) molecules play a central role in adaptive cellular immunity, enabling T-cell lymphocytes to recognize peptide-loaded Major Histocompatibility Complexes (pMHCs) at the surface of other cells (Figure 1). In turn, this molecular interaction can trigger T-cell activation and function (e.g., cytotoxicity or immunomodulation) (1). The specificity of the TCRpMHC interaction is essential for the efficiency of cellular immunity against infectious pathogens and cancer cells, as well as for the safety of new game-changing T-cell-based immunotherapies (2). However, it is well established that the same TCR can recognize multiple pMHC complexes with varying affinities/avidities (3–6), and such “promiscuity” has several biomedical implications, related to mechanisms of self-tolerance (1), heterologous immunity between pathogens (7, 8), autoimmunity, transplant rejection (1, 9), allergies (10), and off-target toxicity in T-cell based immunotherapies (11–13). Unfortunately, the complexity and diversity of the molecules involved in these interactions has slowed the development of both experimental and computational methods to detect, quantify, and predict these T-cell cross-reactivity events. But this picture has been changing in recent years, with exciting developments in both high-throughput experimental methods (5, 14, 15) and scalable computational approaches (16–21) for the study of TCRpMHC interactions. This Research Topic highlights recent studies in this field, including further analysis of T-cell cross-reactivity in antiviral immunity, and new computational approaches to predict TCR specificity and off-target toxicity.
Figure 1 Artistic representation of the key molecules involved in T-cell recognition of an antigenic target. TCR chains (α and β) depicted in shades of purple, CD3 chains in shades of green, MHC chains (α and β2m) in shades of blue, and the peptide-target in red. Cell membranes are depicted in grey. Image obtained with UCSF ChimeraX, using PDB codes 6JXR and 5BRZ.
TCR cross-reactivity is an intrinsic feature of T-cell biology, required to maximize immunity against an overwhelming diversity of antigenic peptide-targets (4, 13, 22, 23). The benefits of cross-reactivity for antiviral immunity are further evidenced by Petrova et al. They analyzed the recall CD8+ T-cell response to variants of the well-characterized Influenza A M158−66 peptide, to show how cross-reactive T-cells can be selected by naturally occurring non-infective variants or quasispecies of RNA viruses. Since immunological history also affects the selection of these cross-reactive cells, Tarabini et al. leveraged existing immunoinformatics methods to investigate the potential link between BCG vaccination and reduced severity of COVID-19 cases. Building upon previous work on image-based analysis of modeled pMHC complexes (6, 24–26), they screened over 13.5 million possible cross-reactive peptide pairs from BCG and SARS-CoV-2, identifying multiple high-density “neighborhoods” of cross-reactive peptides which could be driving heterologous immunity induced by BCG vaccination. Similarly, Antonio et al. used pMHC structural modeling to investigate how previous infections may produce heterologous immunity in a global scale, therefore mitigating the full lethal potential of the COVID-19 pandemic. They identified similar structural fingerprints across peptides derived from other coronaviruses and from unrelated viruses involved in endemic human infections, which could trigger cross-reactive T-cell responses against SARS-CoV-2 variants. In fact, cross-reactivities with SARS-CoV-2-derived peptides have been since reported in multiple studies (27, 28).
These efforts to leverage pMHC structural data represent a more recent trend among computational methods to interpret or predict T-cell cross-reactivity, as previously reviewed by others (6, 29, 30). Attesting to the fast progress in the field, our Research Topic includes three additional computational tools for cross-reactivity prediction guided by structural data. First, Mendes et al. leveraged the analysis of electrostatic potentials over the TCR-interacting surfaces of pMHC complexes to develop MatchTope. The tool relies on a modified version of PIPSA (31) to enable the structure-based clustering of similar pMHC structures, which are in turn more likely to be recognized by the same TCR. Second, Hall-Swan et al. implemented PepSim, a webserver for T-cell cross-reactivity prediction based on a novel similarity score for pMHC structures. PepSim represents the pMHC solvent-accessible surface as a triangular mesh, which is then annotated with biochemical features, including electrostatic potential, hydrophobicity, and hydrogen bond potential. Finally, Fonseca and Antunes introduced CrossDome, an R-based tool to predict the risk for off-target toxicity in T-cell-based immunotherapy. By default, the tool performs a sequence-based peptide-centered search for biochemically similar off-targets, leveraging publicly available multiomics data from healthy tissues (e.g., immunopeptidomics and gene expression data). However, the authors also demonstrate how structural data of TCRpMHC complexes can be used to perform a TCR-centered prediction, enabling to refine the list of putative off-targets for a specific T-cell clone. This is an important direction for future development, considering the greater availability of TCR sequences (e.g., single-cell TCRseq), and the growing interest in analyzing TCR specificity across T-cell repertoires (32). For now, analyses at that scale are mostly limited to sequence-based methods, as reviewed by Ghoreyshi and George. But the authors also describe the emergence of hybrid quantitative computational approaches for studying TCR specificity, with emphasis on the growing role of deep learning architectures behind these methods.
This Research Topic highlights a transition to a new age in the study of T-cell cross-reactivity, in which AI-powered structure-guided computational prediction of TCR specificity for polyclonal T-cell repertoires, and high-throughput experimental validation of T-cell activation, will be used to guide the development of better and safer vaccines and T-cell-based immunotherapies (13, 33). However, there are still challenges ahead, as we try to incorporate in these scalable computational methods a more refined understanding of the forces and dynamics driving the affinity, avidity and specificity of TCRpMHC interactions (34, 35).
DA: Conceptualization, Supervision, Writing – original draft, Writing – review & editing. BB: Writing – review & editing. MC: Writing – review & editing. LS: Writing – review & editing.
The authors declare that the research was conducted in the absence of any commercial or financial relationships that could be construed as a potential conflict of interest.
The author(s) declared that they were an editorial board member of Frontiers, at the time of submission. This had no impact on the peer review process and the final decision
All claims expressed in this article are solely those of the authors and do not necessarily represent those of their affiliated organizations, or those of the publisher, the editors and the reviewers. Any product that may be evaluated in this article, or claim that may be made by its manufacturer, is not guaranteed or endorsed by the publisher.
1. Degauque N, Brouard S, Soulillou JP. Cross-reactivity of TCR repertoire: Current concepts, challenges, and implication for allotransplantation. Front Immunol. (2016) 7:89. doi: 10.3389/fimmu.2016.00089
2. Spear TT, Evavold BD, Baker BM, Nishimura MI. Understanding TCR affinity, antigen specificity, and cross-reactivity to improve TCR gene-modified T cells for cancer immunotherapy. Cancer Immunol Immunother. (2019) 68:1881–9. doi: 10.1007/s00262-019-02401-0.
3. Selin LK, Nahill SR, Welsh RM. Cross-reactivities in memory cytotoxic T lymphocyte recognition of heterologous viruses. J Exp Med. (1994) 179:1933–43. doi: 10.1084/jem.179.6.1933.
4. Welsh RM, Selin LK. No one is naive: the significance of heterologous T-cell immunity. Nat Rev Immunol. (2002) 2:417–26. doi: 10.1038/nri820.
5. Birnbaum ME, Mendoza JL, Sethi DK, Dong S, Glanville J, Dobbins J, et al. Deconstructing the peptide-MHC specificity of T cell recognition. Cell. (2014) 157:1073–87. doi: 10.1016/j.cell.2014.03.047.
6. Antunes DA, Rigo MM, Freitas MV, Mendes MFA, Sinigaglia M, Lizee´ G, et al. Interpreting T-cell cross-reactivity through structure: Implications for TCR-based cancer immunotherapy. Front Immunol. (2017) 8:1210. doi: 10.3389/fimmu.2017.01210.
7. Selin LK, Cornberg M, Brehm MA, Kim SK, Calcagno C, Ghersi D, et al. CD8 memory T cells: cross-reactivity and heterologous immunity. Semin Immunol. (2004) 16:335–47. doi: 10.1016/j.smim.2004.08.014.
8. Cornberg M, Wedemeyer H. Hepatitis C virus infection from the perspective of heterologous immunity. Curr Opin Virol. (2016) 16:41–8. doi: 10.1016/j.coviro.2016.01.005
9. Khorki ME, Shi T, Cianciolo EE, Burg AR, Chukwuma PC, Picarsic JL, et al. Prior viral infection primes cross-reactive CD8+ T cells that respond to mouse heart allografts. Front Immunol. (2023) 14:1287546. doi: 10.3389/fimmu.2023.1287546.
10. Lund G, Christensen LH, Ihlemann J, Andersen PS, Wambre E, Rtzen PA, et al. T cells specific to multiple Bet v 1 peptides are highly cross-reactive toward the corresponding peptides from the homologous group of tree pollens. Front Immunol. (2023) 14:1291666. doi: 10.3389/fimmu.2023.1291666.
11. Antunes DA, Devaurs D, Moll M, Lizee´ G, Kavraki LE. General prediction of peptide-MHC binding modes using incremental docking: A proof of concept. Sci Rep. (2018) 8:4327. doi: 10.1038/s41598-018-22173-4.
12. Klebanoff CA, Chandran SS, Baker BM, Quezada SA, Ribas A. T cell receptor therapeutics: immunological targeting of the intracellular cancer proteome. Nat Rev Drug Discov. (2023) 22:996–1017. doi: 10.1038/s41573-023-00809-z.
13. Bieberich F, Reddy ST. The unexpected benefit of TCR cross-reactivity in cancer immunotherapy. Cancer Res. (2023) 83:3168–9. doi: 10.1158/0008-5472.CAN-23-2594.
14. Kula T, Dezfulian MH, Wang CI, Abdelfattah NS, Hartman ZC, Wucherpfennig KW, et al. T-scan: A genome-wide method for the systematic discovery of T cell epitopes. Cell. (2019) 178:1016–28. doi: 10.1016/j.cell.2019.07.009.
15. Li Y, Qi J, Liu Y, Zheng Y, Zhu H, Zang Y, et al. High-throughput screening of functional neoAntigens and their specific T-cell receptors via the jurkat reporter system combined with droplet microfluidics. Anal Chem. (2023) 95:9697–705. doi: 10.1021/acs.analchem.3c01754
16. Dash P, Fiore-Gartland AJ, Hertz T, Wang GC, Sharma S, Souquette A, et al. Quantifiable predictive features define epitope-specific T cell receptor repertoires. Nature. (2017) 547(7661):89–93. doi: 10.1038/nature22383
17. Glanville J, Huang H, Nau A, Hatton O, Wagar LE, Rubelt F, et al. Identifying specificity groups in the T cell receptor repertoire. Nature. (2017) 547:94–8. doi: 10.1038/nature22976
18. Jaravine V, Raffegerst S, Schendel DJ, Frishman D. Assessment of cancer and virus antigens for cross-reactivity in human tissues. Bioinformatics. (2017) 33:104–11. doi: 10.1093/bioinformatics/btw567
19. Lanzarotti E, Marcatili P, Nielsen M. Chain sequence and structural CDR loop similarities. Front Immunol. (2019) 10:2080. doi: 10.3389/fimmu.2019.02080.
20. Ehrlich R, Kamga L, Gil A, Luzuriaga K, LK S, Ghersi D. SwarmTCR: a computational approach to predict the specificity of T cell receptors. BMC Bioinf. (2021) 22:422. doi: 10.1186/s12859-021-04335-w.
21. Yarmarkovich M, Marshall QF, Warrington JM, Premaratne R, Farrel A, Groff D, et al. Targeting of intracellular oncoproteins with peptide-centric CARs. Nature. (2023) 623:820–7. doi: 10.1038/s41586-023-06706-0.
22. Clark F, Gil A, Thapa I, Aslan N, Ghersi D, Selin LK. Cross-reactivity influences changes in human influenza A virus and Epstein Barr virus specific CD8 memory T cell receptor alpha and beta repertoires between young and old. Front Immunol. (2022) 13:1011935. doi: 10.3389/fimmu.2022.1011935.
23. Soon CF, Zhang S, Suneetha PV, Antunes DA, Manns MP, Raha S, et al. Hepatitis E virus (HEV)Specific T cell receptor cross-recognition: implications for immunotherapy. Front Immunol. (2019) 10:2076. doi: 10.3389/fimmu.2019.02076.
24. Antunes DA, Rigo MM, Silva JP, Cibulski SP, Sinigaglia M, Chies JA, et al. Structural in silico analysis of cross-genotype-reactivity among naturally occurring HCV NS3-1073-variants in the context of HLA-A*02:01 allele. Mol Immunol. (2011) 48:1461–7. doi: 10.1016/j.molimm.2011.03.019.
25. Mendes MF, Antunes DA, Rigo MM, Sinigaglia M, Vieira GF. Improved structural method for T-cell cross-reactivity prediction. Mol Immunol. (2015) 67:303–10. doi: 10.1016/j.molimm.2015.06.017.
26. Antunes DA, Abella JR, Devaurs D, Rigo MM, Kavraki LE. Structure-based methods for binding mode and binding affinity prediction for peptide-MHC complexes. Curr Top Med Chem. (2018) 18:2239–55. doi: 10.2174/1568026619666181224101744.
27. Benede N, Tincho MB, Walters A, Subbiah V, Ngomti A, Baguma R, et al. cell polyfunctional profile in SARS-CoV-2 seronegative children associated with endemic human coronavirus cross-reactivity. iScience. (2024) 27:108728. doi: 10.1016/j.isci.2023.108728.
28. Nesamari R, Omondi MA, Baguma R, ft MA, Ngomti A, Nkayi AA, et al. Cell response to SARSCoV-2 is durable, broadly targeted, and cross-reactive to the hypermutated BA.2.86 variant. Cell Host Microbe. (2024). doi: 10.1016/j.chom.2023.12.003.
29. Zvyagin IV, Tsvetkov VO, Chudakov DM, Shugay M. An overview of immunoinformatics approaches and databases linking T cell receptor repertoires to their antigen specificity. Immunogenetics. (2020) 72:77–84. doi: 10.1007/s00251-019-01139-4.
30. Lee CH, Salio M, Napolitani G, Ogg G, Simmons A, Koohy H. Predicting cross-reactivity and antigen specificity of T cell receptors. Front Immunol. (2020) 11:565096. doi: 10.3389/fimmu.2020.565096.
31. Richter S, Wenzel A, Stein M, Gabdoulline RR, Wade RC. webPIPSA: a web server for the comparison of protein interaction properties. Nucleic Acids Res. (2008). 36:W276–280. doi: 10.1093/nar/gkn181
32. Luo J, Wang X, Zou Y, Chen L, Liu W, Zhang W, et al. Quantitative annotations of T-Cell repertoire specificity. Brief Bioinform 24. (2023). doi: 10.1093/bib/bbad175.
33. Gouttefangeas C, Klein R, Maia A. The good and the bad of T cell cross-reactivity: challenges and opportunities for novel therapeutics in autoimmunity and cancer. Front Immunol. (2023) 14:1212546. doi: 10.3389/fimmu.2023.1212546.
34. Evavold BD. Force in immunology: there is often a catch. J Immunol. (2023) 211:307. doi: 10.4049/jimmunol.2300376.
Keywords: TCR (T cell receptor), MHC, cross-reactivity, T-cell specificity, TCRpMHC, computational biology & bioinformatics
Citation: Antunes DA, Baker BM, Cornberg M and Selin LK (2024) Editorial: Quantification and prediction of T-cell cross-reactivity through experimental and computational methods. Front. Immunol. 15:1377259. doi: 10.3389/fimmu.2024.1377259
Received: 27 January 2024; Accepted: 05 February 2024;
Published: 20 February 2024.
Edited and Reviewed by:
Mariolina Salio, Immunocore, United KingdomCopyright © 2024 Antunes, Baker, Cornberg and Selin. This is an open-access article distributed under the terms of the Creative Commons Attribution License (CC BY). The use, distribution or reproduction in other forums is permitted, provided the original author(s) and the copyright owner(s) are credited and that the original publication in this journal is cited, in accordance with accepted academic practice. No use, distribution or reproduction is permitted which does not comply with these terms.
*Correspondence: Dinler A. Antunes, ZGlubGVyQHVoLmVkdQ==
Disclaimer: All claims expressed in this article are solely those of the authors and do not necessarily represent those of their affiliated organizations, or those of the publisher, the editors and the reviewers. Any product that may be evaluated in this article or claim that may be made by its manufacturer is not guaranteed or endorsed by the publisher.
Research integrity at Frontiers
Learn more about the work of our research integrity team to safeguard the quality of each article we publish.