- 1Department of Neurology, Zhaoyuan People’s Hospital, Zhaoyuan, China
- 2Department of Hematology, Shandong Provincial Hospital Affiliated to Shandong First Medical University, Jinan, Shandong, China
- 3Department of Laboratory Medicine, Shandong Provincial Hospital Affiliated to Shandong First Medical University, Jinan, Shandong, China
A Commentary on
Flow cytometry quantification of tumor-infiltrating lymphocytes to predict the survival of patients with diffuse large B-cell lymphoma
By Yu T, Xu-Monette ZY, Lagoo A, Shuai W, Wang B, Neff J, Carrillo LF, Carlsen ED, Pina-Oviedo S and Young KH (2024). Front. Immunol. 15:1335689. doi: 10.3389/fimmu.2024.1335689
Introduction
Immune cells responsible for anti-tumor surveillance are often inhibited or dysfunctional in cancer, yet they remain to be critical for the clinical outcome of chemotherapies and immunotherapies (1–3). In clinical practice, immune cells are quantified by flow cytometry for the diagnosis of hematologic malignancies, including leukemia, myeloma, and lymphoma (4, 5). In the article collection of “Tumor Microenvironment and Hematological Malignancies: New Evidences and New Questions” in Frontier in Immunology, Yu and colleagues at Duke University Medical Center collected flow cytometry data for fresh diagnostic biopsies from 102 patients with de novo diffuse large B-cell lymphoma (DLBCL), the most common lymphoma, and demonstrated the favorable prognostic effects of tumor-infiltrating lymphocytes (TILs) in DLBCL (Figure 1). To predict clinical outcome, independent prognostic factors were used to build a LASSO-Cox model and a graphic tool (6), demonstrating the proof-of-concept that clinical flow cytometry data can be used for risk prediction in DLBCL, as well as the prognostic significance of immune cell functions in the context of DLBCL disease with various immune escape mechanisms and treatments (7, 8).
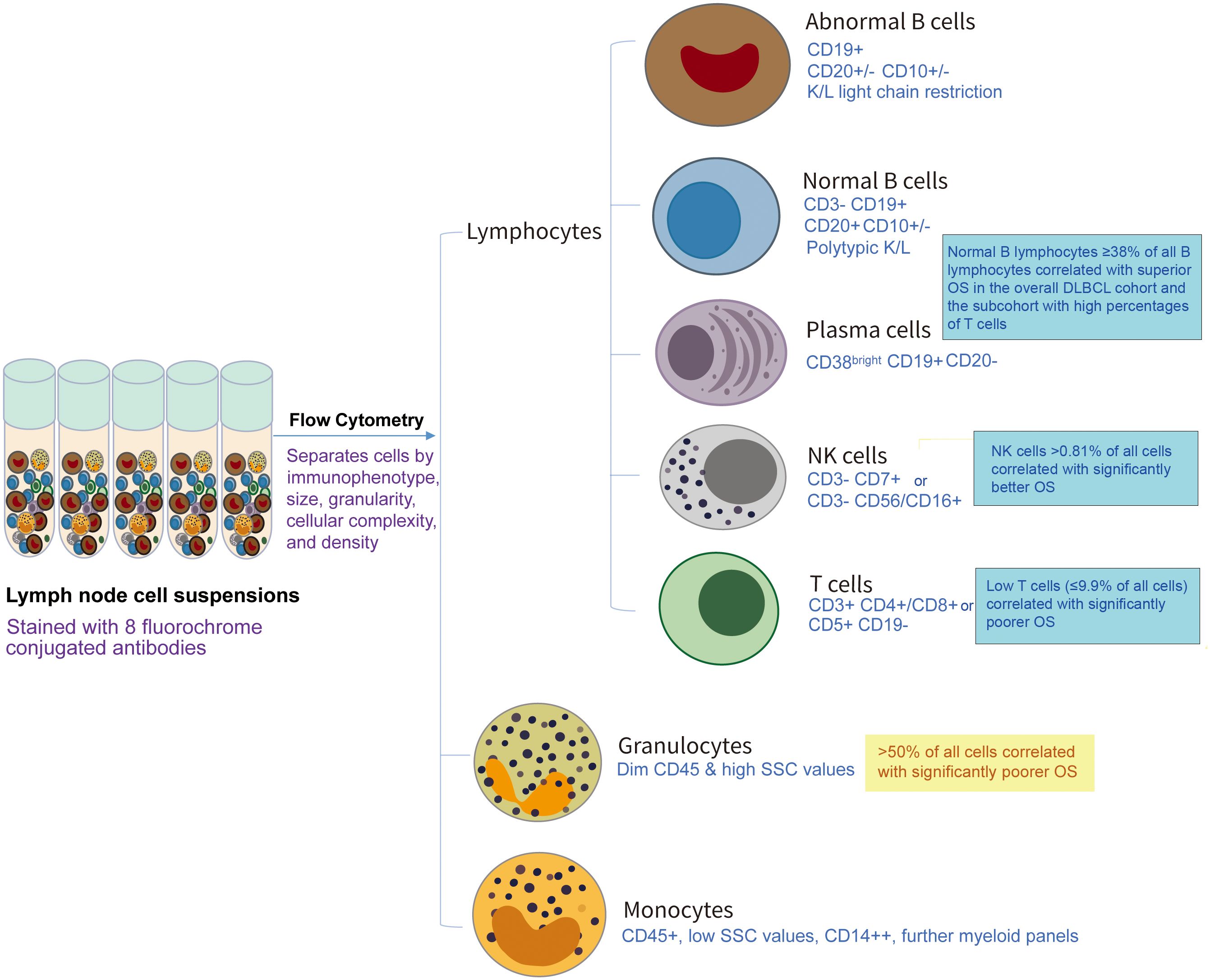
Figure 1 Schematic illustration of the prognostic values of tumor-infiltrating immune cells by flow cytometry analysis in a recent study (Ref. 6) by Yu et al. in Front Immunol 2024, Volume 15, doi: 10.3389/fimmu.2024.1335689. In that study, cell populations were identified and quantified by various antibody panels and different gating strategies by clinical workups according to the Duke Clinical Flow Cytometry Laboratory Standard of Operating Procedures in 2011-2022, and this figure only lists key markers used to identify different immune cell types as summarized in Reference 6.
Clinical flow cytometry data to predict prognosis and facilitate treatment decision
The prognostic role of the tumor microenvironment (TME) in DLBCL has been demonstrated by independent studies using different approaches at the DNA (9), RNA (10, 11), and protein (12) levels. Notably, methods used in these previous studies are generally for researches only; it is important to develop a clinical decision support tool for tailoring personalized regimens and selection of immunotherapy. The flow cytometry study by Yu et al. (6) showed that integrating TME data into risk prediction is feasible in clinical practice.
However, larger scale of studies are needed to build a robust model, and a lot of future work is needed to optimize the flow cytometry risk scores for clinical application. Single-cell RNA sequencing (scRNA-seq), which comes with high cost hindering its application for large-scale prognostic analysis, nonetheless has revealed subclonal heterogeneity, including the drug-sensitive and -resistant DLBCL cell populations separated by CD48 and CD62 markers, as well as heterogeneity of T-cell populations (e.g., cytotoxic, Th1, Th2, regulatory, PD-1+ T cells) (13) which may explain why in the flow cytometry study by Yu et al, after excluding patients with low T cell percentages, high T-cell percentages did not show further prognostic significance. Normal B cells are also heterogeneous, and a scRNA-seq study in non-small cell lung cancer found that tumor-infiltrating naïve-like but not plasma-like B cells were predictive (14). Hence, it is necessary to delve into the heterogeneity of each cell type and the crosstalk between lymphoma and immune cells, and to further establish practical flow cytometry marker panels and gating strategies for isolating tumor/immune cell subclones. Moreover, as new techniques such as phosphoflow cytometry have been applied in clinical studies (15–17), flow cytometry-based prediction models can be substantially improved in the future. Markers of tumor and TME interaction can also be integrated into the standard prognostic scoring systems as proposed in chronic lymphocytic leukemia (18).
The limitation of flow cytometry quantification should also be recognized. In the study by Yu et al, only cases with abnormal B cells successfully identified by flow cytometry were selected, thus excluding DLBCL cases missed by flow cytometry, which occurred in 3-30% of DLBCL cases (19–25). The median percentage of overall B cells in the study cohort was still lower (also lower macrophage percentage while higher T-cell percentage) than that by fluorescent multiplex immunohistochemistry. The authors also suggested that gating strategies and light chain kappa/lambda restriction analysis may not always accurately isolate normal B cell populations (6). Similarly, scRNA-seq results may not truly represent macrophage subpopulations (26). Another disadvantage of flow cytometry data is the incapability to identify the ‘mesenchymal’ category of lymphoma TME, which features abundant lymphatic and vascular endothelial cells, fibroblasts, fibroblastic reticular cells, and extracellular matrix (10).
Increased normal B cells as a novel favorable prognostic factor in DLBCL
Compared with tumor-infiltrating T cells, tumor-infiltrating B lymphocytes (TIL-Bs) are less studied. In recent years, multiple studies have shown favorable prognostic effects of TIL-Bs in solid tumors (27, 28). Flow cytometry can distinguish normal B cells from lymphoma B cells, and Yu et al. (6) have validated the favorable prognostic association of TIL-B abundance determined by flow cytometry in DLBCL patients, which was first shown in an International Lymphoma Consortium cohort through ultra-deep sequencing of immunoglobulin genes (9). In that study by Xu-Monette et al, higher frequencies of normal B cells in total B cells determined by genetic assays were associated with significant prognostic effects in overall DLBCL, DLBCL cell-of-origin subtypes, and the EZB genetic subtype. These novel findings may provide a basis for developing more effective immunotherapies. For example, cytokines promoting interaction between normal B cells and T cells may enhance the efficacy of chimeric antigen receptor T cell therapies. If antibodies secreted by TIL-Bs can be identified, they could be used to target tumors while spare normal B cells, thereby having advantage over rituximab, anti-CD19 antibodies, antibody-drug conjugates, and engineered chimeric antigen receptors currently used in DLBCL treatment.
Further mechanistic and therapeutic studies using other approaches are warranted. First, we need determine whether B-cell functions in cell-mediated or humoral immunity, or both, are responsible for the observed superior survival. To be more specific, whether antigen-presentation function of B cells, contribution to optimal activation of T cells, cytokine production, or antibody-dependent mechanisms are more critical (27). Second, we should examine B cell functions and subsets (e.g., naïve, germinal-center, and memory B cells, antibody-secreting plasma cells), as well as the differences between DLBCL subtypes. Third, we need determine whether and how therapies influence the quantity and antitumor function of TIL-Bs. In the study by Yu et al, TIL-Bs showed more remarkable prognostic effects in treated patients, especially those receiving upfront rituximab.
Discussion
In summary, Yu et al. showed that in a single-center DLBCL cohort, real-world flow cytometry data of tumor-infiltrating T, NK, and B cells had predictive values, which has important clinical and therapeutic implications. We look forward to future clinical and mechanistic investigations of TIL-B functions, both of which hold paramount importance for developing a robust clinical decision support tool and novel therapies for DLBCL.
Author contributions
ZS: Conceptualization, Writing – original draft. RT: Visualization, Writing – review & editing. HW: Writing – review & editing, Methodology. XF: Conceptualization, Funding acquisition, Writing – original draft.
Funding
The author(s) declare that financial support was received for the research, authorship, and/or publication of this article. The article processing cost is supported by the Natural Science Foundation of Shandong Province (ZR2022MH310, ZR2023MH376).
Conflict of interest
The authors declare that the research was conducted in the absence of any commercial or financial relationships that could be construed as a potential conflict of interest.
Publisher’s note
All claims expressed in this article are solely those of the authors and do not necessarily represent those of their affiliated organizations, or those of the publisher, the editors and the reviewers. Any product that may be evaluated in this article, or claim that may be made by its manufacturer, is not guaranteed or endorsed by the publisher.
References
1. Thommen DS, Schumacher TN. T cell dysfunction in cancer. Cancer Cell. (2018) 33:547–62. doi: 10.1016/j.ccell.2018.03.012
2. Tilsed CM, Fisher SA, Nowak AK, Lake RA, Lesterhuis WJ. Cancer chemotherapy: insights into cellular and tumor microenvironmental mechanisms of action. Front Oncol. (2022) 12:960317. doi: 10.3389/fonc.2022.960317
3. Zhang Y, Zhang Z. The history and advances in cancer immunotherapy: understanding the characteristics of tumor-infiltrating immune cells and their therapeutic implications. Cell Mol Immunol. (2020) 17:807–21. doi: 10.1038/s41423-020-0488-6
4. Singh AP, Courville EL. Advances in monitoring and prognostication for lymphoma by flow cytometry. Clin Lab Med. (2023) 43:351–61. doi: 10.1016/j.cll.2023.04.010
5. Li W. Flow cytometry in the diagnosis of leukemias. In: Leukemia [Internet]. Brisbane (AU): Exon Publications. (2022) Chapter 4. doi: 10.36255/exon-publications-leukemia
6. Yu T, Xu-Monette ZY, Lagoo A, Shuai W, Wang B, Neff J, et al. (2024). Flow cytometry quantification of tumor-infiltrating lymphocytes to predict the survival of patients with diffuse large B-cell lymphoma. Front Immunol. (2024) 15:1335689. doi: 10.3389/fimmu.2024.1335689
7. Ansell SM. Fundamentals of immunology for understanding immunotherapy for lymphoma. Am Soc Hematology. Educ Program. (2020) 2020:585–9. doi: 10.1182/hematology.2020002537
8. Mancuso S, Mattana M, Carlisi M, Santoro M, Siragusa S. Effects of B-cell lymphoma on the immune system and immune recovery after treatment: the paradigm of targeted therapy. Int J Mol Sci. (2022) 23:3368. doi: 10.3390/ijms23063368
9. Xu-Monette ZY, Li Y, Snyder T, Yu T, Lu T, Tzankov A, et al. Tumor-infiltrating normal B cells revealed by immunoglobulin repertoire clonotype analysis are highly prognostic and crucial for antitumor immune responses in DLBCL. Clin Cancer Res. (2023) 29:4808–21. doi: 10.1158/1078-0432.CCR-23-1554
10. Kotlov N, Bagaev A, Revuelta MV, Phillip JM, Cacciapuoti MT, Antysheva Z, et al. Clinical and biological subtypes of B-cell lymphoma revealed by microenvironmental signatures. Cancer Discovery. (2021) 11:1468–89. doi: 10.1158/2159-8290.CD-20-0839
11. Steen CB, Luca BA, Esfahani MS, Azizi A, Sworder BJ, Nabet BY, et al. The landscape of tumor cell states and ecosystems in diffuse large B cell lymphoma. Cancer Cell. (2021) 39:1422–1437 e10. doi: 10.1016/j.ccell.2021.08.011
12. Xu-Monette ZY, Xiao M, Au Q, Padmanabhan R, Xu B, Hoe N, et al. Immune profiling and quantitative analysis decipher the clinical role of immune-checkpoint expression in the tumor immune microenvironment of DLBCL. Cancer Immunol Res. (2019) 7:644–57. doi: 10.1158/2326-6066.CIR-18-0439
13. Roider T, Seufert J, Uvarovskii A, Frauhammer F, Bordas M, Abedpour N, et al. Dissecting intratumour heterogeneity of nodal B-cell lymphomas at the transcriptional, genetic and drug-response levels. Nat Cell Biol. (2020) 22:896–906. doi: 10.1038/s41556-020-0532-x
14. Chen J, Tan Y, Sun F, Hou L, Zhang C, Ge T, et al. Single-cell transcriptome and antigen-immunoglobin analysis reveals the diversity of B cells in non-small cell lung cancer. Genome Biol. (2020) 21:152. doi: 10.1186/s13059-020-02064-6
15. Lyons YA, Wu SY, Overwijk WW, Baggerly KA, Sood AK. Immune cell profiling in cancer: molecular approaches to cell-specific identification. NPJ Precis Oncol. (2017) 1:26. doi: 10.1038/s41698-017-0031-0
16. Toney NJ, Schlom J, Donahue RN. Phosphoflow cytometry to assess cytokine signaling pathways in peripheral immune cells: potential for inferring immune cell function and treatment response in patients with solid tumors. J Exp Clin Cancer Res. (2023) 42:247. doi: 10.1186/s13046-023-02802-1
17. Wu S, Jin L, Vence L, Radvanyi LG. Development and application of ‘phosphoflow’ as a tool for immunomonitoring. Expert Rev Vaccines. (2010) 9:631–43. doi: 10.1586/erv.10.59
18. Giudice V, Serio B, Bertolini A, Mettivier L, D’Alto F, Pezzullo L, et al. Implementation of International Prognostic Index with flow cytometry immunophenotyping for better risk stratification of chronic lymphocytic leukemia. Eur J haematology. (2022) 109:483–93. doi: 10.1111/ejh.13833
19. Peng D, Kodituwakku A, Le S, Smith S, Qiu MR, Earls P, et al. Factors determining whether diffuse large B-cell lymphoma samples are detected by flow cytometry. Int J Lab Hematol. (2023) 45:927–34. doi: 10.1111/ijlh.14158
20. Vallangeon BD, Tyer C, Williams B, Lagoo AS. Improved detection of diffuse large B-cell lymphoma by flow cytometric immunophenotyping-Effect of tissue disaggregation method. Cytometry B Clin Cytom. (2016) 90:455–61. doi: 10.1002/cyto.b.21322
21. Henrique RM, Sousa ME, Godinho MI, Costa I, Barbosa IL, Lopes CA. Immunophenotyping by flow cytometry of fine needle aspirates in the diagnosis of lymphoproliferative disorders: A retrospective study. J Clin Lab Anal. (1999) 13:224–8. doi: 10.1002/(ISSN)1098-2825
22. Bertram HC, Check IJ, Milano MA. Immunophenotyping large B-cell lymphomas. Flow cytometric pitfalls and pathologic correlation. Am J Clin Pathol. (2001) 116:191–203. doi: 10.1309/BA3U-RMTU-D7UJ-M8DR
23. Demurtas A, Stacchini A, Aliberti S, Chiusa L, Chiarle R, Novero D. Tissue flow cytometry immunophenotyping in the diagnosis and classification of non-Hodgkin’s lymphomas: a retrospective evaluation of 1,792 cases. Cytometry B Clin Cytom. (2013) 84:82–95. doi: 10.1002/cyto.b.21065
24. Martínez A, Aymerich M, Castillo M, Colomer D, Bellosillo B, Campo E, et al. Routine use of immunophenotype by flow cytometry in tissues with suspected hematological Malignancies. Cytometry B Clin Cytom. (2003) 56:8–15. doi: 10.1002/cyto.b.10044
25. Verstovsek G, Chakraborty S, Ramzy I, Jorgensen JL. Large B-cell lymphomas: fine-needle aspiration plays an important role in initial diagnosis of cases which are falsely negative by flow cytometry. Diagn Cytopathol. (2002) 27:282–5. doi: 10.1002/dc.10192
26. Hume DA, Millard SM, Pettit AR. Macrophage heterogeneity in the single-cell era: facts and artifacts. Blood. (2023) 142:1339–47. doi: 10.1182/blood.2023020597
27. Laumont CM, Banville AC, Gilardi M, Hollern DP, Nelson BH. Tumour-infiltrating B cells: immunological mechanisms, clinical impact and therapeutic opportunities. Nat Rev Cancer. (2022) 22:414–30. doi: 10.1038/s41568-022-00466-1
Keywords: flow cytometry, TIL-B, tumor-infiltrating lymphocytes, TME, TIL, DLBCL, prognosis, clinical decision support tool
Citation: Sun Z, Tan R, Wu H and Fang X (2024) Commentary: Flow cytometry quantification of tumor-infiltrating lymphocytes to predict the survival of patients with diffuse large B-cell lymphoma. Front. Immunol. 15:1377221. doi: 10.3389/fimmu.2024.1377221
Received: 27 January 2024; Accepted: 08 April 2024;
Published: 18 April 2024.
Edited by:
Masaki Hikida, Akita Univerity, JapanReviewed by:
Ester Marina-Zárate, La Jolla Institute for Immunology (LJI), United StatesCopyright © 2024 Sun, Tan, Wu and Fang. This is an open-access article distributed under the terms of the Creative Commons Attribution License (CC BY). The use, distribution or reproduction in other forums is permitted, provided the original author(s) and the copyright owner(s) are credited and that the original publication in this journal is cited, in accordance with accepted academic practice. No use, distribution or reproduction is permitted which does not comply with these terms.
*Correspondence: Xiaosheng Fang, ZnhzaF8xMDEwQDEyNi5jb20=
†These authors share first authorship