- 1The First Clinical Medical College, Shandong University of Traditional Chinese Medicine, Jinan, China
- 2Department of Neurosurgery Ward 5, Binzhou Medical University Hospital, Binzhou, Shandong, China
- 3Cardiovascular Department, Affiliated Hospital of Shandong University of Traditional Chinese Medicine, Jinan, Shandong, China
Objective: Ferroptosis and necroptosis are two recently identified forms of non-apoptotic cell death. Their dysregulation plays a critical role in the development and progression of Psoriasis (PsD) and Atherosclerosis (AS). This study explores shared Ferroptosis and necroptosis-related genes and elucidates their molecular mechanisms in PsD and AS through the analysis of public databases.
Methods: Data sets for PsD (GSE30999) and AS (GSE28829) were retrieved from the GEO database. Differential gene expression (DEG) and weighted gene co-expression network analysis (WGCNA) were performed. Machine learning algorithms identified candidate biomarkers, whose diagnostic values were assessed using Receiver Operating Characteristic (ROC) curve analysis. Additionally, the expression levels of these biomarkers in cell models of AS and PsD were quantitatively measured using Western Blot (WB) and real-time quantitative PCR (RT-qPCR). Furthermore, CIBERSORT evaluated immune cell infiltration in PsD and AS tissues, highlighting the correlation between characteristic genes and immune cells. Predictive analysis for candidate drugs targeting characteristic genes was conducted using the DGIdb database, and an lncRNA-miRNA-mRNA network related to these genes was constructed.
Results: We identified 44 differentially expressed ferroptosis-related genes (DE-FRGs) and 30 differentially expressed necroptosis-related genes (DE-NRGs). GO and KEGG enrichment analyses revealed significant enrichment of these genes in immune-related and inflammatory pathways, especially in NOD-like receptor and TNF signaling pathways. Two ferroptosis-related genes (NAMPT, ZFP36) and eight necroptosis-related genes (C7, CARD6, CASP1, CTSD, HMOX1, NOD2, PYCARD, TNFRSF21) showed high sensitivity and specificity in ROC curve analysis. These findings were corroborated in external validation datasets and cell models. Immune infiltration analysis revealed increased levels of T cells gamma delta, Macrophages M0, and Macrophages M2 in PsD and AS samples. Additionally, we identified 43 drugs targeting 5 characteristic genes. Notably, the XIST-miR-93–5p-ZFP36/HMOX1 and NEAT1-miR-93–5p-ZFP36/HMOX1 pathways have been identified as promising RNA regulatory pathways in AS and PsD.
Conclusion: The two ferroptosis-related genes (NAMPT, ZFP36) and eight necroptosis-related genes (C7, CARD6, CASP1, CTSD, HMOX1, NOD2, PYCARD, TNFRSF21) are potential key biomarkers for PsD and AS. These genes significantly influence the pathogenesis of PsD and AS by modulating macrophage activity, participating in immune regulation, and mediating inflammatory responses.
1 Introduction
Psoriasis (PsD) is a chronic, immune-mediated inflammatory skin condition with a genetic predisposition, affecting an estimated 2%-5% of the global population (1). It primarily affects the skin and joints, but also significantly influences the cardiovascular system through systemic inflammation (2). This inflammation not only exacerbates PsD symptoms but also affects the vascular system and contributes to the formation and progression of Atherosclerosis (AS) plaques (3). Emerging evidence identifies PsD as an independent risk factor for AS, with patients experiencing PsD for over eight years facing a heightened risk of developing AS (4, 5). Although PsD is recognized as an independent risk factor for AS, the pathogenic mechanisms of their coexistence are not fully understood.
The relationship between PsD and AS is complex, with existing research highlighting shared immune mechanisms involving the IL-12/Th1 and IL-23/Th17 pathways. Differentiated Th1 cells produce TNF-α and IFN-γ, which promote plaque growth, while Th17 effector cytokines contribute to neovascularization and bleeding within plaques, thus enhancing their fragility (6). Furthermore, a decrease in Treg cells and their functions leads to increased Th1 and Th17 proliferation. This is accompanied by reductions in TGF-β and IL-10, both known for their anti-inflammatory properties and roles in AS protection (7). In PsD, activated Th17 cells produce pro-inflammatory cytokines IL-17A-F, IL-22, IL-21, and TNF-α. TNF-α stimulates IL-23 formation, thereby enhancing memory T cell synthesis of IL-17 and IFN-γ, which perpetuates skin inflammation (8, 9). These insights indicate that immune-mediated inflammation plays a crucial role in the dynamics between PsD and AS, creating a detrimental cycle that significantly impacts patients. Therefore, understanding the pathogenesis of PsD and AS and identifying shared therapeutic targets is of paramount importance.
Moreover, ferroptosis and necroptosis are distinct from autophagy, pyroptosis, and apoptosis, and they play a key role in AS and PsD (10, 11). For instance, studies have shown a significant reduction in GPX4 in PsD lesions compared to healthy uninvolved skin, along with a marked increase in Nrf2 downstream targets (12). Li et al. observed elevated levels of lipid ROS and divalent iron in the epidermis of PsD patients and confirmed the activation of ferroptosis in PsD patients and mouse models using transmission electron microscopy (13). Similarly, Fer-1 has been shown to mitigate lipid peroxidation and endothelial dysfunction in mouse aortic endothelial cells by upregulating SLC7A11 and GPX4 expression, thereby delaying the pathological progression of AS (14). Additionally, studies indicate that knocking out ApoA1 leads to increased formation of necrotic cores in atherosclerotic plaques and elevated phosphorylation levels of necroptotic mediators RIPK3 and MLKL (15). In PsD, increased expression of necroptotic molecules RIPK1, RIPK3, and MLKL has been noted, particularly in keratin-forming cells of the epidermis (16). These findings underscore the significance of ferroptosis and necroptosis in both PsD and AS. However, the specific molecular mechanisms driving this relationship remain unclear. Increasing evidence suggests that ferroptosis and necroptosis are not independent processes, but rather interact extensively. For instance, mitochondrial ROS are essential for the pathogenesis of both ferroptosis and necroptosis, facilitating the autophosphorylation of RIPK1, which subsequently recruits RIPK3 to form functional necrosomes (17). Additionally, studies have shown that overexpression of GPX4 can reduce mitochondrial ROS levels, thereby preventing both ferroptosis and necroptosis (18). Furthermore, knocking out ACSL4 inhibits ferroptosis in iron-sensitive mouse and human cells, but increases their sensitivity to necroptosis (19). Recent research indicates that the release of damage-associated molecular patterns (DAMPs) from plasma membrane pores may be a common feature of both ferroptosis and necroptosis, suggesting that DAMPs released during ferroptosis may promote necroptosis. These findings highlight the interconnected nature of ferroptosis and necroptosis (20). However, most studies to date have focused on either ferroptosis or necroptosis as singular cell death mechanisms in relation to disease, with less attention given to their combined pathogenic mechanisms in diseases. Therefore, further exploration of the shared genetic traits and potential molecular mechanisms of ferroptosis and necroptosis in PsD and AS holds broad prospects for the diagnosis and treatment of these comorbid conditions.
With the advancement of microarray high-throughput squencing technology in recent years, microarrays have become a pioneering method for identifying genes associated with ferroptosis and necroptosis in PsD and AS, facilitating early diagnosis and treatment (21). Integrating bioinformatics approaches enables the precise identification of potential molecular biomarkers of ferroptosis and necroptosis in PsD and AS, thereby enhancing our understanding of their biological mechanisms and pinpointing exact molecular targets for therapy. Accordingly, this study utilizes the limma package and Weighted Gene Co-expression Network Analysis (WGCNA) to pinpoint differentially expressed genes in PsD and AS. We then employ machine learning techniques to identify characteristic genes of ferroptosis and necroptosis, and to explore their biological processes and immune infiltration mechanisms. These characteristic genes could prove to be crucial regulators and potential therapeutic targets in PsD and AS (Figure 1).
2 Materials and methods
2.1 Dataset acquisition
Expression data for atherosclerosis (AS), psoriatic dermatitis (PsD), and normal samples were obtained from the GEO database. The selection criteria included: 1) Usage of the GPL570 [HG-U133_Plus_2] Affymetrix Human Genome U133 Plus 2.0 Array for all datasets; 2) Inclusion of only human subjects; 3) A minimum of 20 samples per study; 4) Collection of samples from either skin or arterial tissues. Datasets GSE28829 and GSE30999 were selected as the training set for this study, owing to their comprehensive and reliable data extensively cited in numerous publications (22, 23). Gene expression datasets for GSE28829 and GSE30999 were retrieved from the GEO database utilizing the Affymetrix GPL570 platform. The GSE28829 dataset includes 16 advanced atherosclerotic plaques and 13 early atherosclerotic plaques, while the GSE30999 dataset contains 85 paired samples from psoriasis patients, featuring both lesional skin (LS) and adjacent normal tissues (NL).Additionally, datasets GSE100927 and GSE14905, noted for their extensive citation and verification through in vitro studies, were selected as the validation sets for this research (24, 25).
2.2 Differential expression analysis
We employed the R package “limma” (version 3.40.6), which uses a generalized linear model approach to identify differentially expressed genes (DEGs) between the diseased and normal groups within the GSE28829 and GSE30999 datasets (26). The selection criteria were a P.Value < 0.05 and an absolute log2 fold change (log2FC) of ≥ 0.5. Volcano plots for the DEGs were generated using the “ggplot2” package (27). DEGs common to both datasets were selected as candidate genes for further analysis.
2.3 WGCNA analysis
WGCNA, a systems bioinformatics technique, efficiently groups highly correlated genes into modules and analyzes correlations between these modules and phenotypes (28). We conducted “WGCNA” on the GSE28829 and GSE30999 datasets using the “WGCNA” package in R. The analysis began with sample clustering, excluding genes with an average expression below 0.5. The soft-thresholding power β was determined using the pickSoftThreshold function in R. Hierarchical clustering analysis was performed with a minimum module size of 60 and a merge height cutoff of 0.25. Finally, we calculated the gene significance and module membership for disease-associated modules. Notably, the grey module was categorized as a collection of genes not assigned to any module. We selected modules for further analysis based on a Pearson correlation coefficient |r| > 0.5 and P < 0.05.
2.4 Identification of differentially expressed genes related to ferroptosis and necroptosis
Genes associated with ferroptosis were sourced from the FerrDb and GeneCards databases, while those pertaining to necroptosis were obtained from the GeneCards and Harmonizome databases. The differentially expressed genes (DEGs) identified in Section 1.2 and module genes from Section 1.3 were merged. This combined set was then cross-referenced with the ferroptosis and necroptosis gene sets to pinpoint candidate genes for further analysis.
2.5 Functional enrichment analysis
To elucidate the biological functions and pathways related to DE-FRGs and DE-NRGs in PsD and AS, gene ontology (GO) and Kyoto Encyclopedia of Genes and Genomes (KEGG) enrichment analyses were conducted using the DAVID database (29). GO analysis examined the genes’ biological processes (BP), cellular components (CC), and molecular functions (MF), while KEGG analysis assessed the pathways in which these genes are predominantly involved. Significance was indicated by a P-value < 0.05, highlighting significant enrichment of DE-FRGs and DE-NRGs in various GO and KEGG categories.
2.6 Immune cell infiltration analysis
CIBERSORT, a tool for deconvoluting human immune cell subtype expression matrices using linear support vector regression, determines the proportions of different immune cell types based on known gene expression profiles. This analysis helps delineate the immune cell composition within the immune microenvironment of PsD and AS, identifying key immune cells involved in the development and progression of these diseases (30). The “CIBERSORT” package in R was utilized for analyzing immune infiltration in control samples versus PsD and AS samples. Bar graphs depicted the proportions of various immune cell types, while violin plots contrasted the levels of immune cell infiltration between PsD and control groups, and between AS and control groups. Additionally, this study demonstrated the correlation between the expression levels of hub genes and the degree of infiltration of various immune cell types in the samples.
2.7 Identification of key DE-FRGs and DE-NRGs
To pinpoint key DE-FRGs and DE-NRGs linked to PsD and AS, three machine learning algorithms were employed: LASSO, SVM-RFE, and RF. The LASSO algorithm was used to identify genetic biomarkers of PsD and AS by contrasting DE-FRGs and DE-NRGs against normal samples (31). SVM, a supervised machine learning method for data classification, assessed model performance through the average false-positive rate obtained from 10-fold cross-validation (32). The “randomForest” package in the RF algorithm assessed the feature importance of each gene, deeming those with an importance value above 2 as potential biomarkers for further investigation (33). Ultimately, the genes identified by LASSO, SVM-RFE, and RF were intersected to aid in the diagnosis of PsD and AS.
2.8 Identification and analysis of hub genes
The diagnostic value of hub genes in PsD and AS was determined using the pROC R package, which constructed Receiver Operating Characteristic (ROC) curves and calculated the area under the curve (AUC) for each gene. An AUC > 0.5 was considered to have diagnostic value (34). The expression levels of these hub genes in PsD and AS were confirmed using the training sets (GSE28829 and GSE30999) and validated in the validation sets (GSE100927 and GSE14905). Additionally, the corrplot R package generated correlation heatmaps, illustrating the relationships between immune cells and candidate diagnostic genes.
2.9 Prediction of candidate drugs for hub genes
Drugs associated with the hub genes were identified using the Drug-Gene Interaction database (DGIdb) (35). The DGIdb database facilitated the exploration of potential drugs or compounds that interact with DE-FRGs and DE-NRGs. Subsequently, a drug-gene interaction network was visualized using Cytoscape software, offering an in-depth view of the interactions between these hub genes and potential therapeutic agents.
2.10 Construction of the CeRNA network
MiRNAs targeting the hub genes were identified using the miRanda, miRDB, and TargetScan databases, while lncRNAs potentially targeting these miRNAs were sourced from the starBase database. A ceRNA network involving lncRNA-miRNA-mRNA interactions was then constructed using Cytoscape software, providing a comprehensive framework for understanding these interactions.
2.11 Cell culture and treatment
Human epidermal keratinocytes (HaCaT) and human umbilical vein endothelial cells (HUVEC) cell lines were obtained from Wuhan Pricella Biotechnology Co., Ltd. (Wuhan, China). HaCaT cells were cultured in DMEM containing 10% serum, 1% penicillin, and 1% streptomycin, while HUVEC cells were maintained in complete endothelial cell culture medium (ZQ1304, Zhongqiaoxinzhou Biotech). Both cell types were incubated at 37°C in a 5% CO2 incubator and passaged upon reaching approximately 80% confluence. As previously described, HaCaT cells were treated with a concentration of 10ng/ml of M5 (TNF-α, IL-17A, IL-22, IL-1α, and oncostatin M) for approximately 24 hours to induce a PsD inflammation cell model (36). Additionally, HUVEC were exposed to 100 μg/mL ox-LDL for 24 hours to simulate an in vitro AS injury model (37).
2.12 Western blotting
Total protein was extracted from HaCaT and HUVEC cells using RIPA lysis buffer containing protease and phosphatase inhibitors. Protein concentration was estimated using the BCA assay. Samples were separated on SDS-polyacrylamide gel electrophoresis (SDS-PAGE) and then transferred to PVDF membranes. To block the membranes, 10% non-fat milk was used for two hours, followed by overnight incubation at 4°C with primary antibodies diluted appropriately (anti-NAMPT, ZFP36, CTSD, HMOX1, CASP1, and PYCARD antibodies). Membranes were then incubated with HRP-conjugated secondary antibodies at room temperature for one hour. Detection was performed using a highly sensitive multi-function imager (GE, USA), and images were analyzed using Image J software.
2.13 Real-time quantitative PCR analysis
Total RNA was isolated from HaCaT and HUVEC cells using TRIzol reagent. The extracted RNA was reverse-transcribed into complementary DNA using a reverse transcription kit. qRT-PCR analysis was conducted using a SYBR Green reagent kit and a real-time fluorescence PCR instrument. The levels of target mRNA were normalized to the levels of GAPDH mRNA (internal control). Primers used in the qRT-PCR analysis are listed in Table 1.
2.14 Statistical analysis
All data processing and analyses in this study were conducted using GraphPad Prism and R software (version 4.1.0). For comparisons between two groups involving continuous variables, the Student’s t-test was used for normally distributed data, with results presented as mean ± SD. ROC curve analyses were performed using the “pROC” package in R, assessing diagnostic performance by calculating the area under the curve (AUC). Correlations between different genes were calculated using Spearman’s rank correlation analysis. All statistical p-values were two-sided, with p < 0.05 considered statistically significant.
3 Results
3.1 WGCNA identified key modules in psoriasis and atherosclerosis
Analysis of datasets GSE28829 and GSE30999 identified 750 and 4358 DEGs, respectively (Figures 2A, D), with 360 DEGs common between the two (Figure 2G). WGCNA revealed 18 relevant modules in GSE30999 and 7 in GSE28829, each represented by a unique color. Module-trait relationship heatmaps were generated to assess each module’s disease association (Figures 2B, C, E, F). The “blue” (|r|=0.57, p=0.001), “black” (|r|=0.6, p=5e-04), “green” (|r|=0.51, p=0.004), and “turquoise” (|r|=0.82, p=6e-08) modules were highly correlated with AS, comprising 928 genes. The “blue” (|r|=0.91, p=4e-66), “brown” (|r|=0.71, p=4e-27), “cyan” (|r|=0.69, p=2e-25), “magenta” (|r|=0.6, p=4e-18), “pink” (|r|=0.53, p=9e-14), and “yellow” (|r|=0.61, p=6e-19) modules were strongly linked with PsD, encompassing 4758 genes. In total, 519 genes were shared across modules associated with both conditions (Figure 2H). By integrating the 360 shared DEGs detected by limma with the 519 from WGCNA and eliminating duplicates, a total of 879 shared genes were isolated for further analysis.
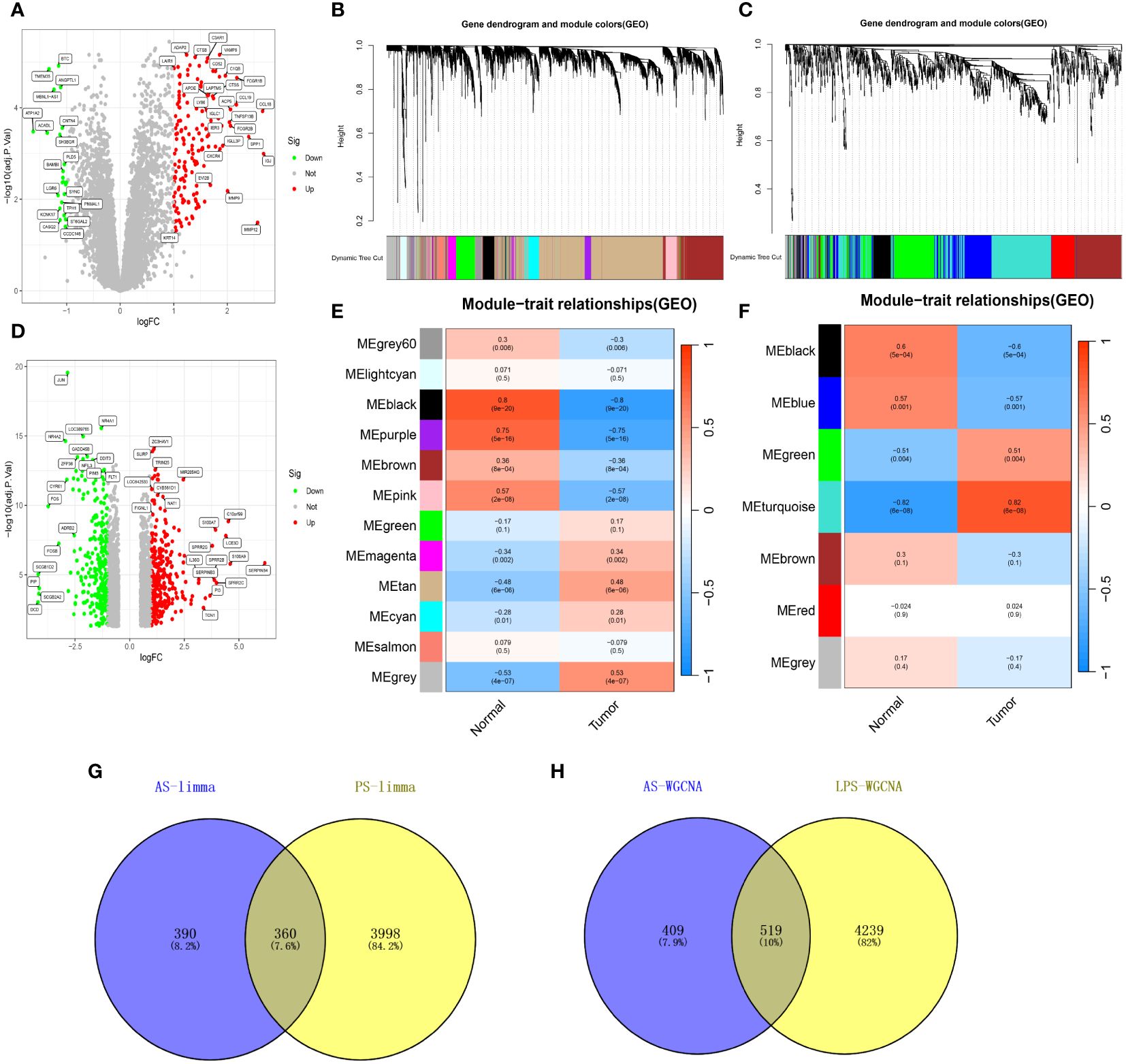
Figure 2 Shared genes in AS and PsD. (A) Volcano plot of DEGs in AS. (B, C) Hierarchical clustering of genes into different modules (each color represents a module). (D) Volcano plot of DEGs in PsD. (E) Heatmap of module-trait gene relationships in PsD. (F) Heatmap of module-trait gene relationships in AS. (G) Venn diagram of common DEGs in AS and PsD. (H) Venn diagram of genes in related modules in AS and PsD.
3.2 Selection of DE-FRGs and DE-NRGs
The 879 shared genes were cross-referenced with iron death and necroptotic apoptosis gene sets, yielding 44 DE-FRGs and 30 DE-NRGs (Figures 3A, B). The differential expression of these genes is depicted in Figures 3C, F, while their expression levels are shown in bar scatter plots (Figures 3D, G). The chromosomal locations of the 44 DE-FRGs and 30 DE-NRGs are detailed in Figures 3E, H.
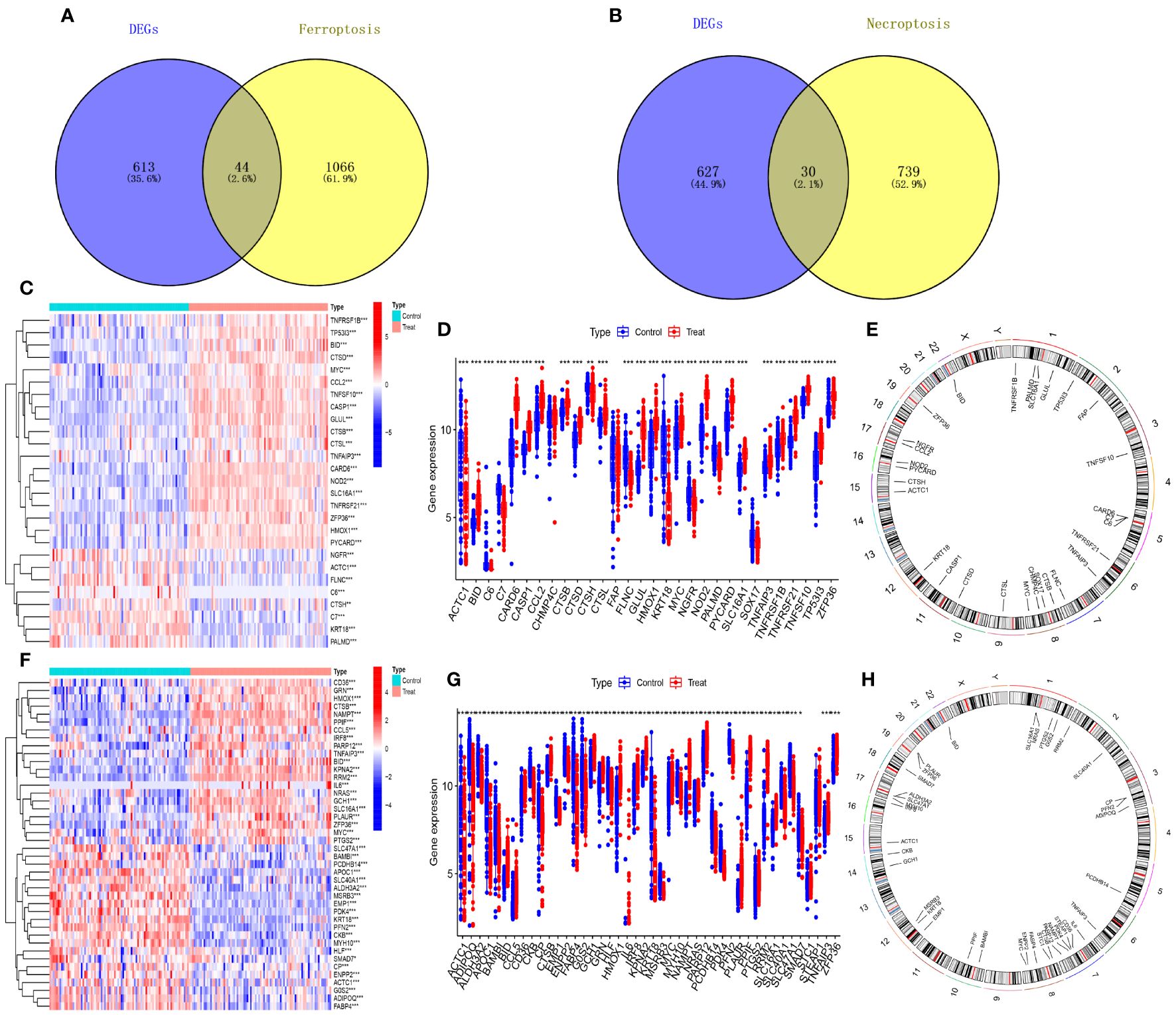
Figure 3 Identification of DE-FRGs and DE-NRGs. (A) Venn diagram of shared genes intersecting with ferroptosis-related genes. (B) Venn diagram of shared genes intersecting with necroptosis-related genes. (C, F) Heatmaps of expression patterns for DE-NRGs and DE-FRGs, respectively. (D, G) Bar scatter plots showing expression levels of DE-NRGs and DE-FRGs. (E, H) Chromosomal positions of DE-NRGs and DE-FRGs. *P<0.05, **P<0.01, ***P<0.001.
3.3 GO and KEGG enrichment analyses were conducted to identify biological processes and signaling pathways associated with differentially expressed genes
To elucidate the biological processes and pathways of shared differentially expressed genes, we conducted GO function and KEGG pathway enrichment analyses on the 44 DE-FRGs and 30 DE-NRGs using the DAVID online tool. The GO function enrichment analysis showed that DE-FRGs are predominantly enriched in regulation of smooth muscle cell proliferation, epithelial cell apoptotic process, response to oxidative stress, presence of lipid droplets, endoplasmic reticulum lumen, cytokine receptor binding, transforming growth factor beta receptor binding, and cytokine activity. Conversely, DE-NRGs are mainly enriched in positive regulation of endopeptidase activity, cellular response to tumor necrosis factor, inflammasome complex, pore complex, peptidase activator activity in the apoptotic process, and death receptor activity. The KEGG enrichment analysis indicated significant enrichment of DE-FRGs in pathways such as Ferroptosis, NOD-like receptor signaling pathway, TNF signaling pathway, PPAR signaling pathway, and IL-17 signaling pathway. Conversely, DE-NRGs showed significant enrichment in Necroptosis, NOD-like receptor signaling pathway, TNF signaling pathway, and p53 signaling pathway. These findings highlight the crucial role of inflammatory responses in both PsD and AS, with extracellular immunomediators such as cytokines and pathways like NOD-like receptor signaling and TNF signaling pathways playing central roles (Figure 4).
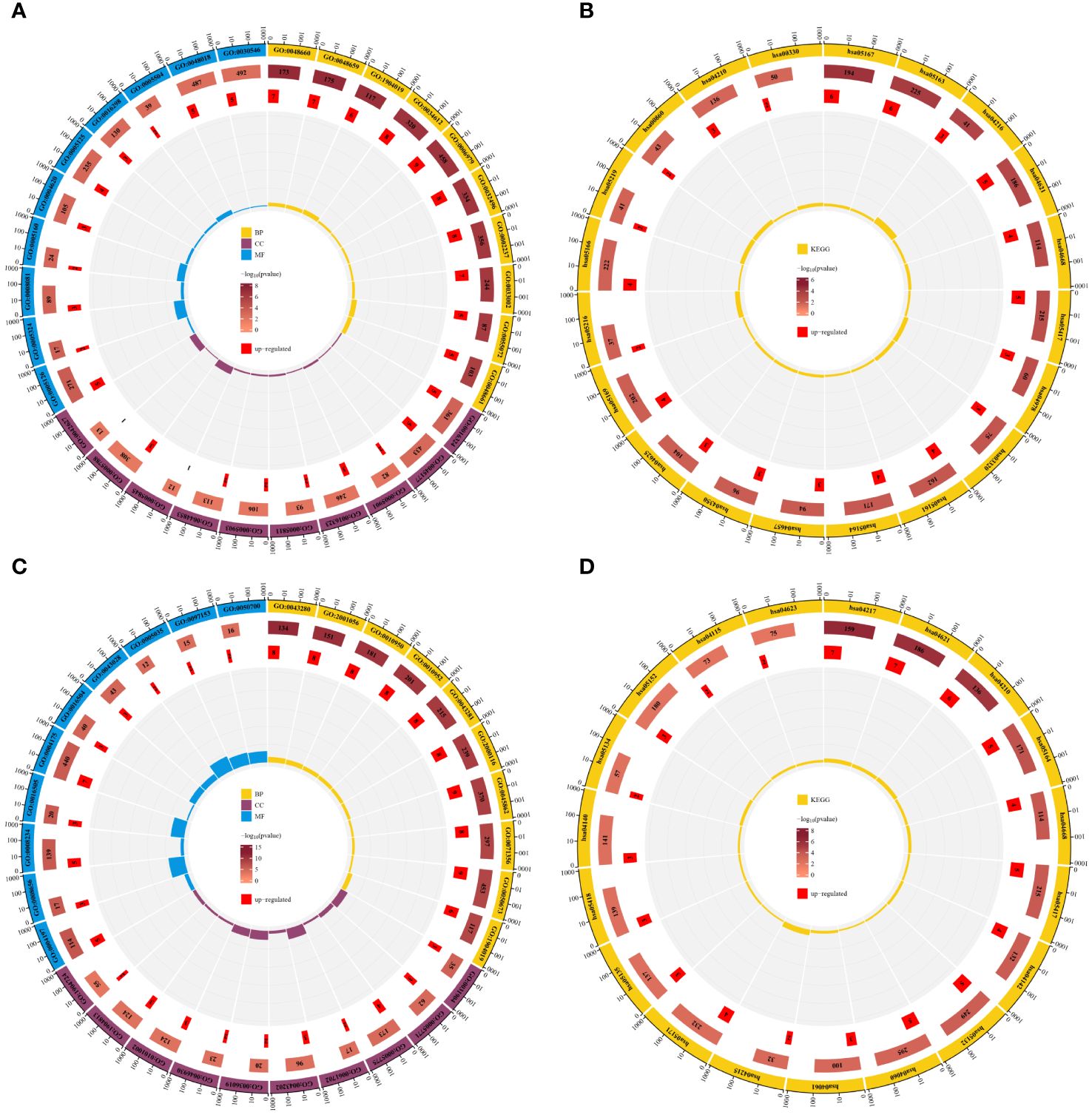
Figure 4 Enrichment analysis of DE-FRGs and DE-NRGs. (A) GO function enrichment analysis for DE-FRGs. (B) KEGG enrichment analysis for DE-FRGs. (C) GO function enrichment analysis for DE-NRGs. (D) KEGG enrichment analysis for DE-NRGs.
3.4 Potential biomarkers for atherosclerosis and psoriasis were screened using three machine learning methods
To determine diagnostic biomarkers for ferroptosis and necroptosis in PsD and AS, we utilized LASSO, SVM-RFE, and RF algorithms for feature gene selection. The LASSO algorithm identified 12 ferroptosis-related genes and 14 necroptosis-related genes (Figures 5A–D). The RF algorithm pinpointed 11 genes associated with iron death and 12 related to necroptosis (Figures 5E–H). Meanwhile, the SVM-RFE algorithm selected 25 ferroptosis-related genes and 22 necroptosis-related genes (Figures 5I–L). Using these algorithms, we identified 2 ferroptosis-related genes (NAMPT, ZFP36) and 8 necroptosis-related genes (C7, CARD6, CASP1, CTSD, HMOX1, NOD2, PYCARD, TNFRSF21) (Figures 5M, N) Table 2.
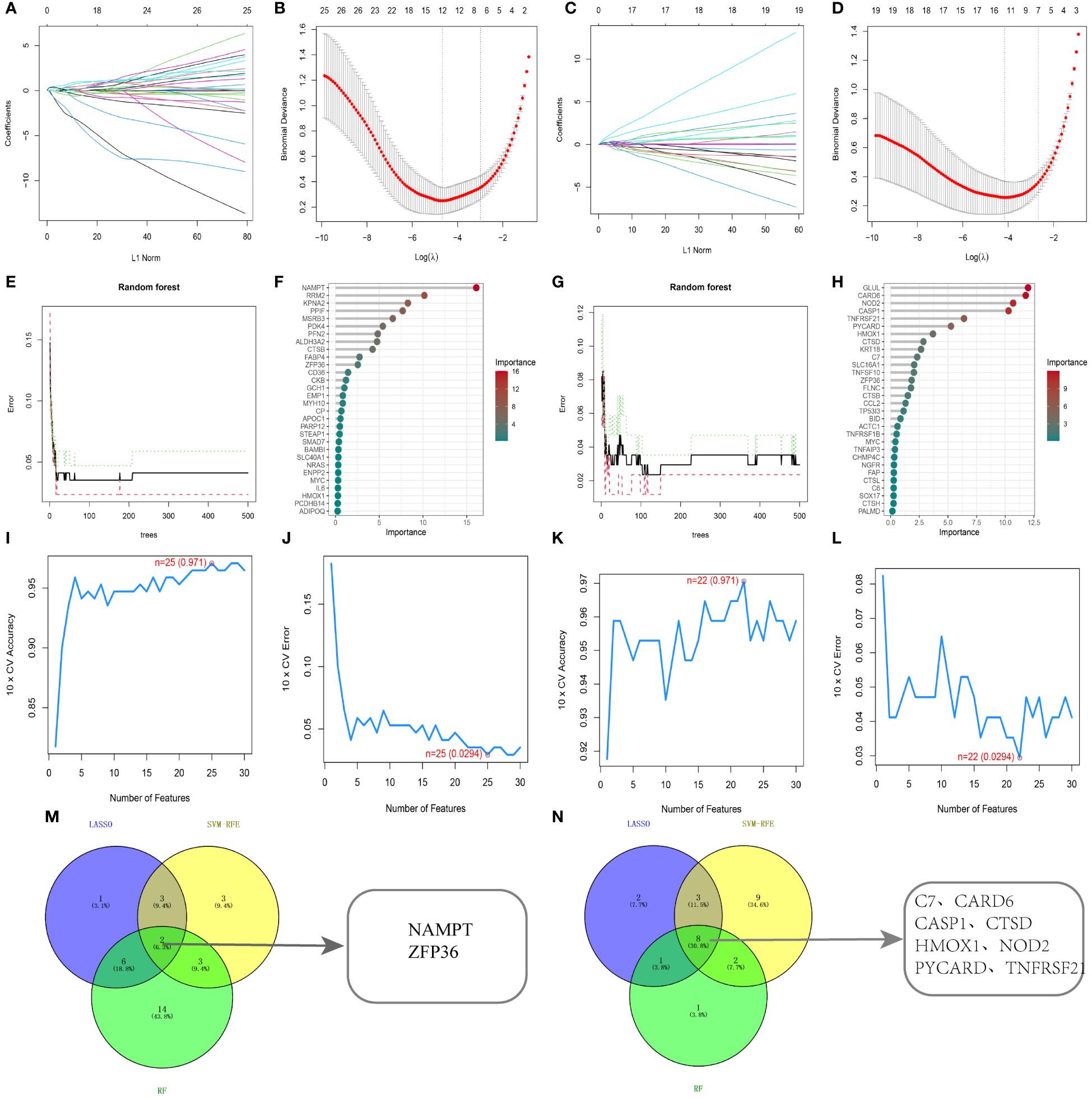
Figure 5 Identification using machine learning algorithms. (A, B) LASSO regression for selecting ferroptosis characteristic genes, with the optimal gene count (n=12) at the curve’s lowest point. (C, D) LASSO regression for selecting necroptosis characteristic genes, with the optimal gene count (n=14) at the curve’s lowest point. (E, F) Random forest algorithm for ferroptosis characteristic gene selection. (G, H) Random forest algorithm for necroptosis characteristic gene selection. (I, J) SVM-RFE algorithm for ferroptosis characteristic gene selection. (K, L) SVM-RFE algorithm for necroptosis characteristic gene selection. (M, N) Common genes identified by the three algorithms. .
3.5 Key genes were validated using the GEO database and further confirmed through cellular experiments
In our study, we used ROC curves to assess the diagnostic predictive values of hub genes in different datasets (Figures 6, 7). In the GSE28829 dataset, the following genes exhibited AUC values greater than 0.6: ZFP36 (AUC=0.707), NAMPT (AUC=0.654), C7 (AUC=0.889), CARD6 (AUC=0.865), CASP1 (AUC=0.923), CTSD (AUC=0.904), HMOX1 (AUC=0.798), NOD2 (AUC=0.918), PYCARD (AUC=0.933), TNFRSF21 (AUC=0.817). Similarly, in the GSE30999 dataset, ZFP36 (AUC=0.870), NAMPT (AUC=0.966), C7 (AUC=0.905), CARD6 (AUC=0.981), CASP1 (AUC=0.970), CTSD (AUC=0.919), HMOX1 (AUC=0.892), NOD2 (AUC=0.976), PYCARD (AUC=0.934), TNFRSF21 (AUC=0.944) also demonstrated AUC values greater than 0.6, indicating their potential as reliable diagnostic markers for AS and PsD.
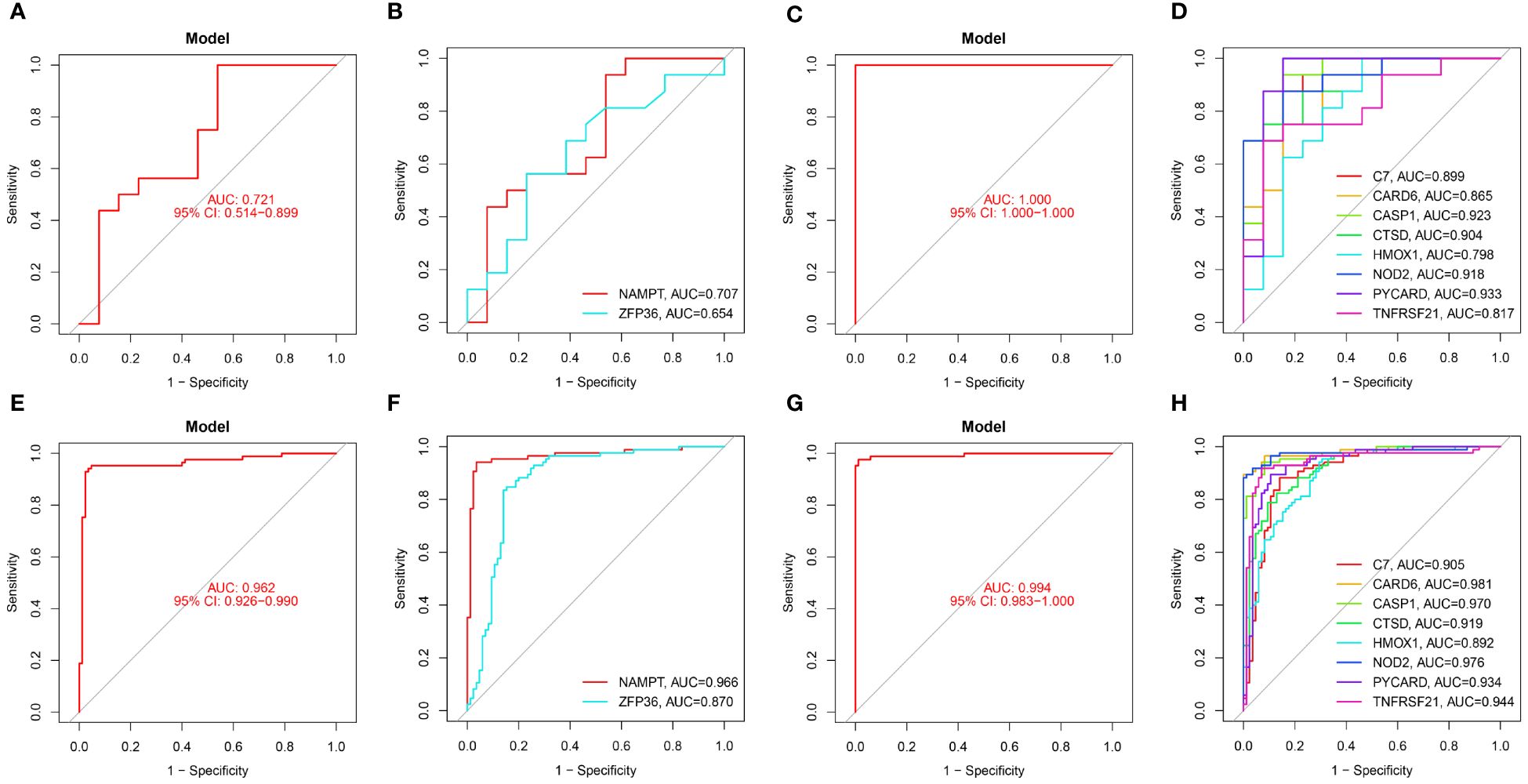
Figure 6 Diagnostic performance of candidate genes in the validation sets. (A, B) ROC curve analysis of the 2 ferroptosis genes in GSE28829. (C, D) ROC curve analysis of the 8 necroptosis genes in GSE28829. (E, F) ROC curve analysis of the 2 ferroptosis genes in GSE30999. (G, H) ROC curve analysis of the 8 necroptosis genes in GSE30999.
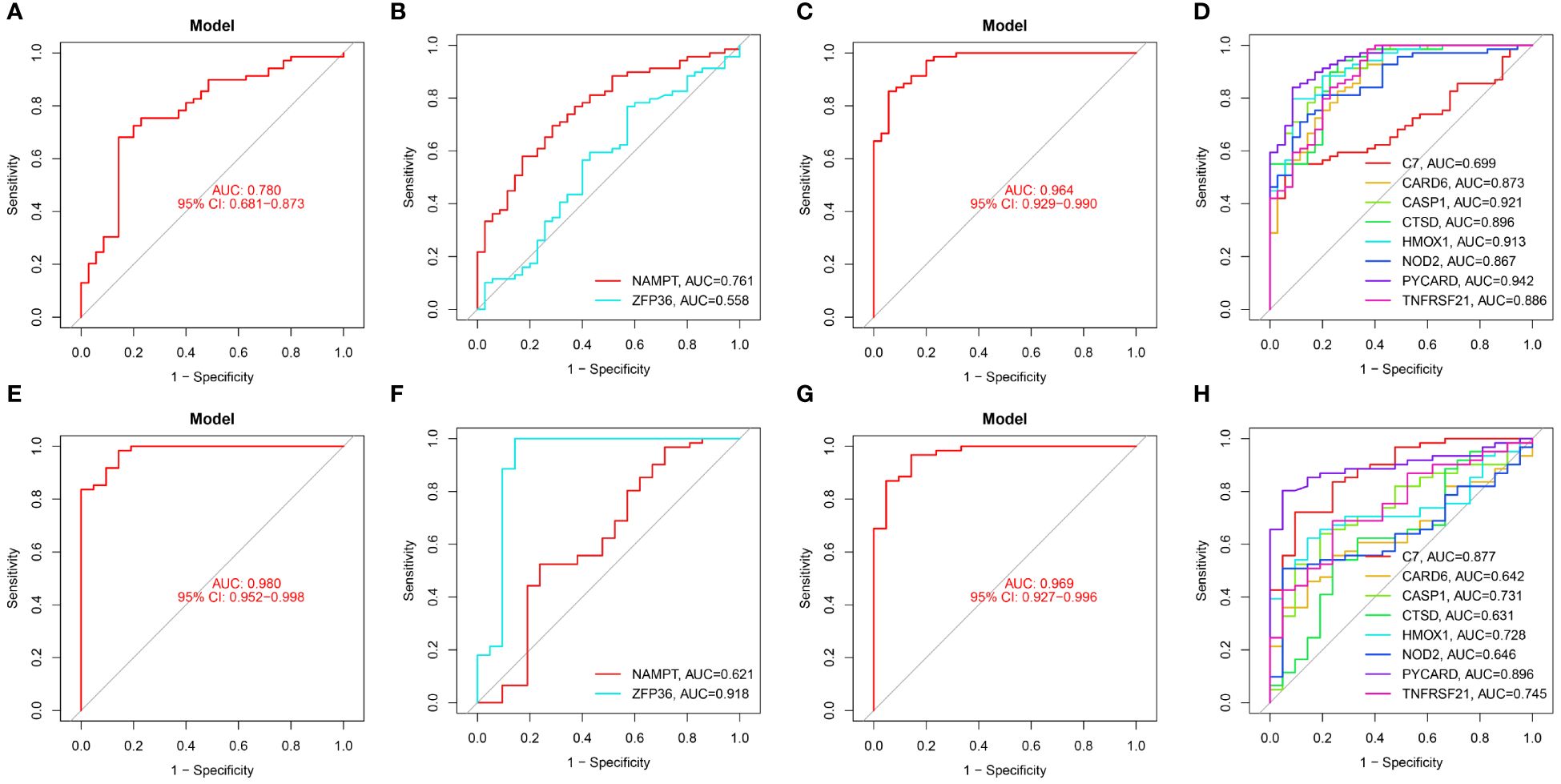
Figure 7 Diagnostic performance of candidate genes in the validation set. (A, B) ROC curve results for 2 ferroptosis genes in GSE100927. (C, D) ROC curve results for 8 necroptotic apoptosis genes in GSE100927. (E, F) ROC curve results for 2 ferroptosis genes in GSE14905. (G, H) ROC curve results for 8 necroptotic apoptosis genes in GSE14905.
In the validation sets, different cohorts also demonstrated good predictive efficacy. In the AS validation set (GSE100927), the AUC values for ZFP36, NAMPT, C7, CARD6, CASP1, CTSD, HMOX1, NOD2, PYCARD, and TNFRSF21 were 0.558, 0.761, 0.699, 0.873, 0.921, 0.896, 0.913, 0.867, 0.942, and 0.886, respectively. In the PsD validation set (GSE14905), the AUC values were 0.918, 0.621, 0.877, 0.642, 0.731, 0.631, 0.728, 0.646, 0.896, and 0.745. These results further suggest that these ten genes could serve as common diagnostic markers for AS and PsD.
We further demonstrated that the expression levels of two ferroptosis genes and eight necroptotic apoptosis genes in the training sets GSE28829 and GSE30999 (Figures 8A–J) showed significant differences between the AS group and the normal group, except for ZFP36. These genes also exhibited significant expression differences between the psoriatic disease (PsD) group and the normal group (Figures 8K–T). Additionally, as depicted in Figures 9A–J, the expression levels of the characteristic genes in GSE100927 were consistent with those in the training sets. Similarly, their expression levels in GSE14905 matched those in the training sets (Figures 9K–T). Additionally, RT-qPCR results confirmed that, compared to the control group, the mRNA expression levels of NAMPT, ZFP36, CASP1, CTSD, HMOX1, and PYCARD were elevated in AS and PsD cell models (Figure 10). Western Blot (WB) results indicated that the expression levels of NAMPT, ZFP36, CASP1, CTSD, HMOX1, and PYCARD were significantly increased in AS and PsD cell models (Figure 11). This further validates the conclusions drawn in this study.
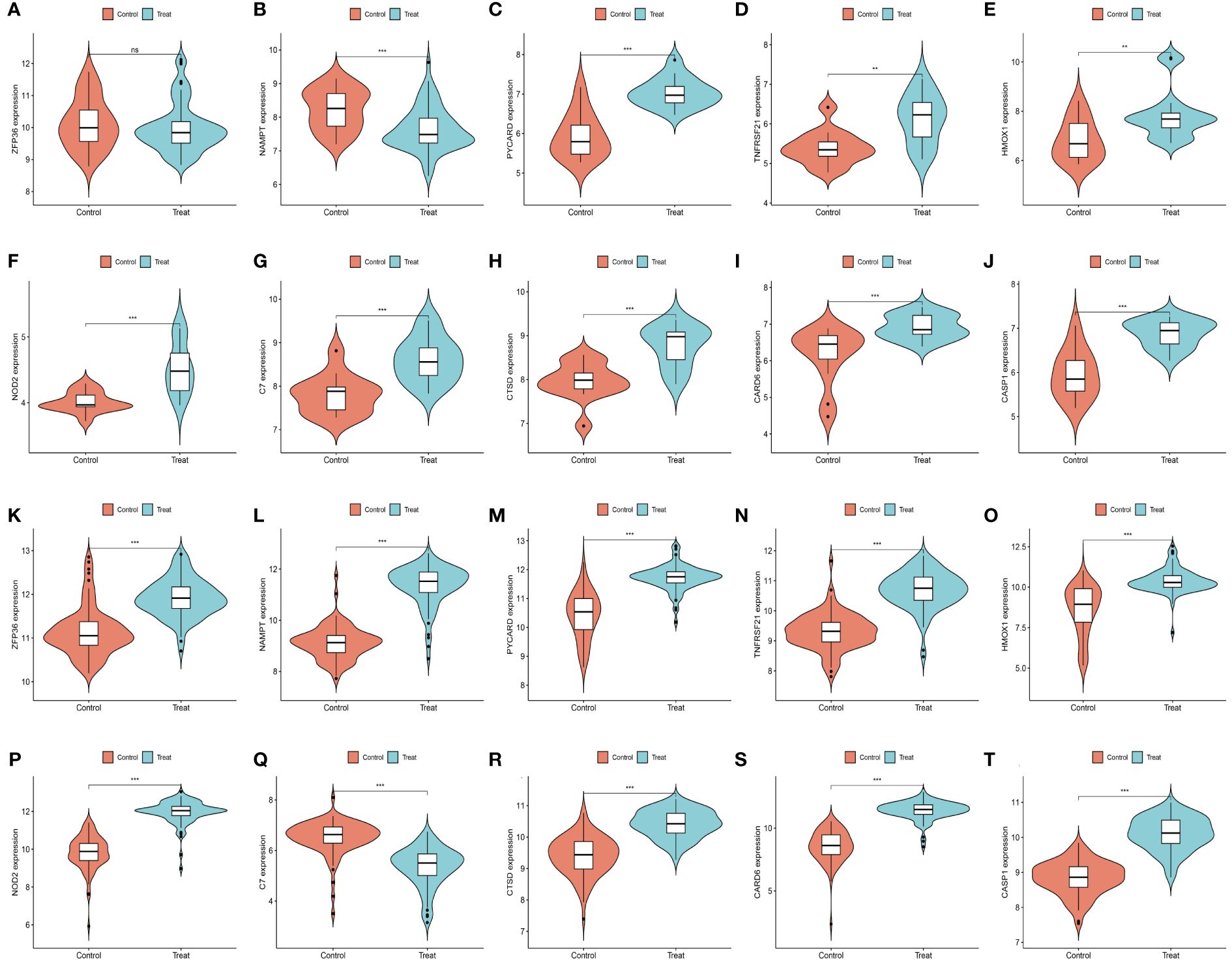
Figure 8 Expression levels of feature genes in GSE28829 and GSE30999. (A–J) Expression patterns of feature genes in GSE28829. (K–T) Expression patterns of feature genes in GSE30999. **P<0.01, ***P<0.001. ns, No statistical.
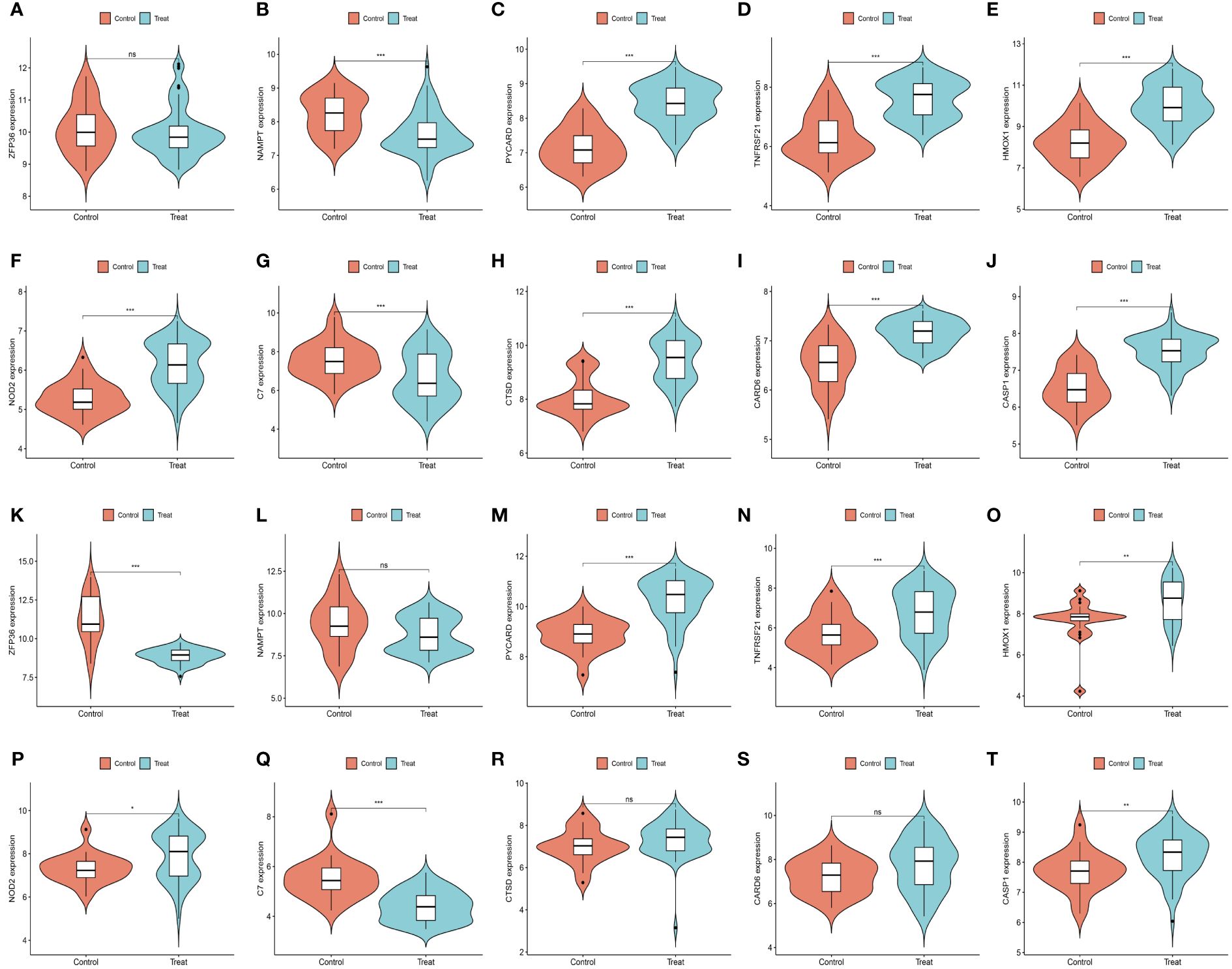
Figure 9 Expression levels of feature genes in GSE100927 and GSE14905. (A–J) Expression patterns of feature genes in GSE100927. (K–T) Expression patterns of feature genes in GSE14905. *P<0.05, **P<0.01, ***P<0.001. ns, No statistical.
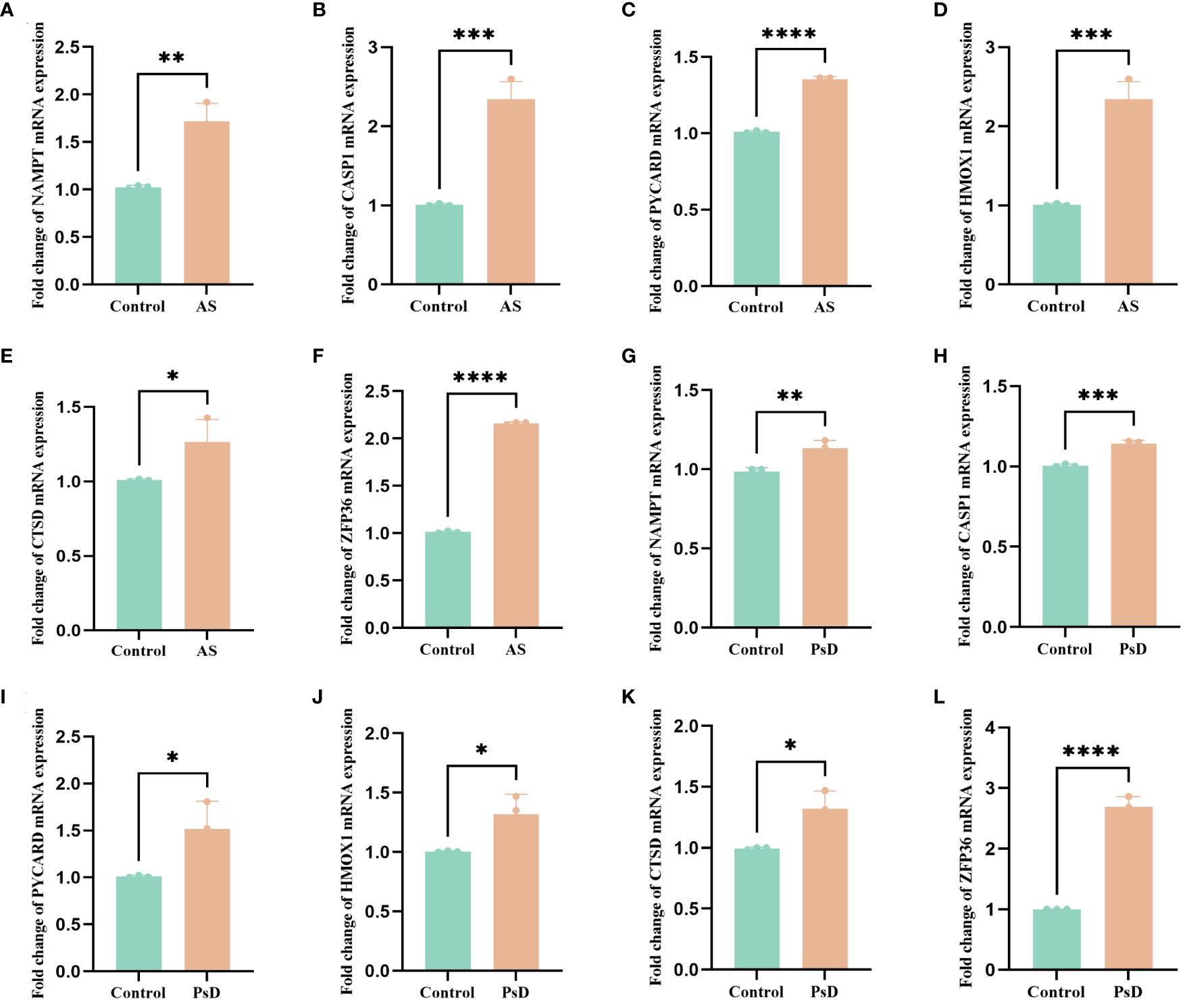
Figure 10 RT-qPCR results of six key genes in as and PsD samples. (A–F) Experimental validation of key gene expression in AS. (G–L) Experimental validation of key gene expression in PsD samples. Relative mRNA expression of NAMPT, CASP1, PYCARD, HMOX1, CTSD and ZFP36. (*p< 0.05, **p < 0.01, ***p<0.001, ****p<0.0001).
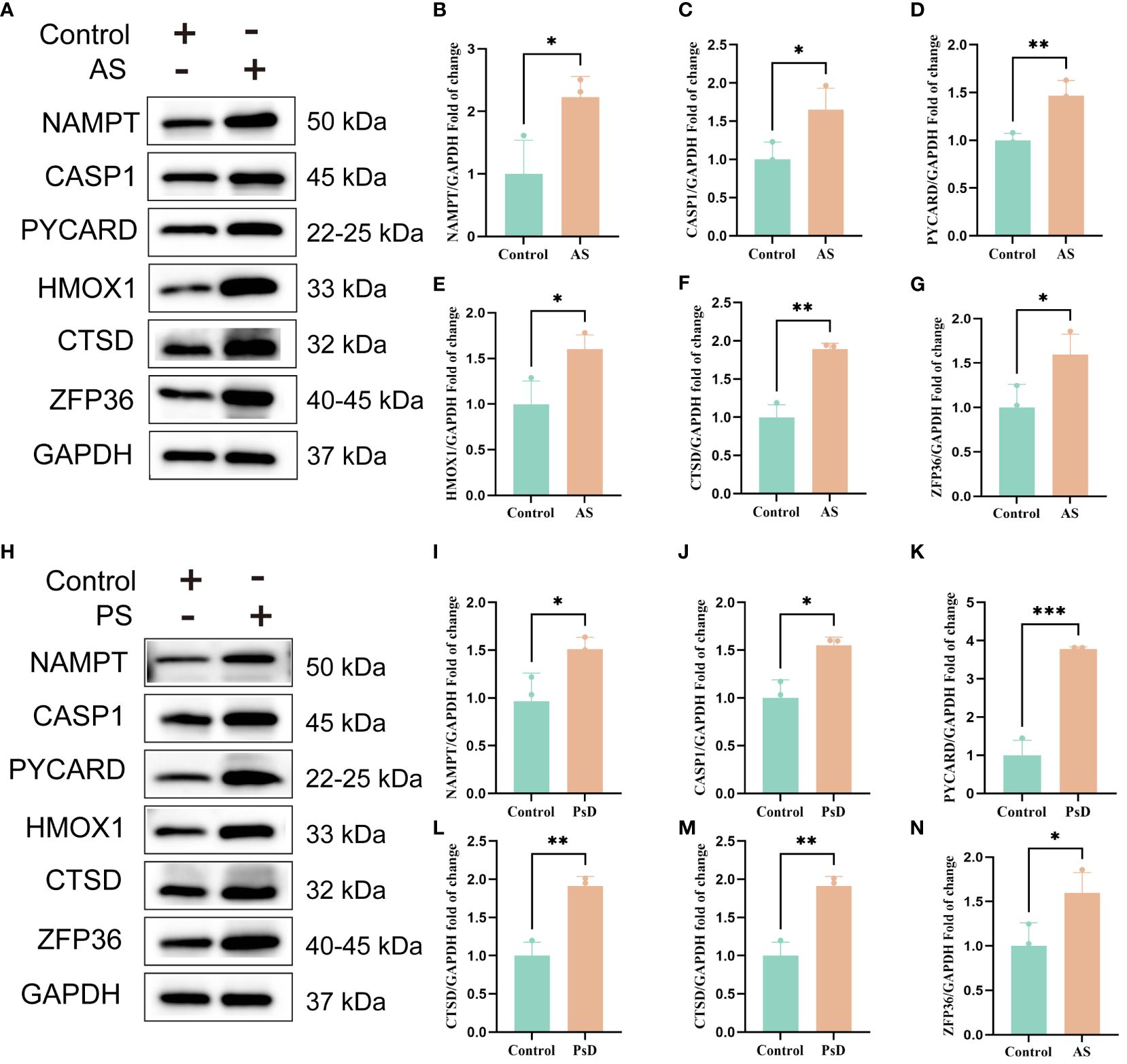
Figure 11 WB results of six key genes in AS and PsD samples. (A, H) Western blot analysis of NAMPT, CASP1, PYCARD, HMOX1, CTSD and ZFP36 protein levels in As and PsD samples. (B–G, I–N) The ratio of NAMPT, CASP1, PYCARD, HMOX1, CTSD and ZFP36 to GAPDH was quantitatively analyzed by ImageJ. (*p< 0.05, **p < 0.01, ***P<0.001).
3.6 Investigating immune cell infiltration and its correlation with key genes
The CIBERSORT algorithm was employed to analyze differences in the immune microenvironment between patients and normal samples in the GSE28829 and GSE30999 datasets. In the GSE28829 dataset, as illustrated in Figures 12A, 13A, a significant negative correlation was found between activated Mast cells and resting Mast cells (r=-0.76), while follicular helper T cells showed a significant positive correlation with naive B cells (r=0.77). In the GSE30999 dataset, naive B cells had a significant positive correlation with Plasma cells (r=-0.62), and activated Mast cells were significantly positively correlated with resting NK cells (r=0.61). Compared to normal samples, higher levels of memory B cells, gamma delta T cells, Macrophages M0, and Macrophages M2 were detected in AS samples (Figure 12B). In PsD samples, increased levels of CD4 memory activated T cells, gamma delta T cells, Macrophages M0, M1, M2, activated Dendritic cells, and Neutrophils were observed (Figure 13B). Heatmaps depicted the proportions and differences in 22 types of immune cell infiltrations between AS, PsD, and normal samples (Figures 12C, 13C). Furthermore, Spearman correlation analysis showed that in AS samples, ZFP36, TNFRSF21, PYCARD, HMOX1, and CASP1 were significantly positively correlated with Macrophages M0, while ZFP36, TNFRSF21, and CASP1 exhibited a significant negative correlation with Plasma cells (Figure 12D). In PsD samples, ZFP36, NOD2, NAMPT were significantly positively correlated with Macrophages M1, and NAMPT and CASP1 were significantly negatively correlated with resting Mast cells (Figure 13D). These results indicate a close relationship between changes in the immune microenvironment of AS and PsD patients and the expression of feature genes such as ZFP36, TNFRSF21, PYCARD, HMOX1, and CASP1.
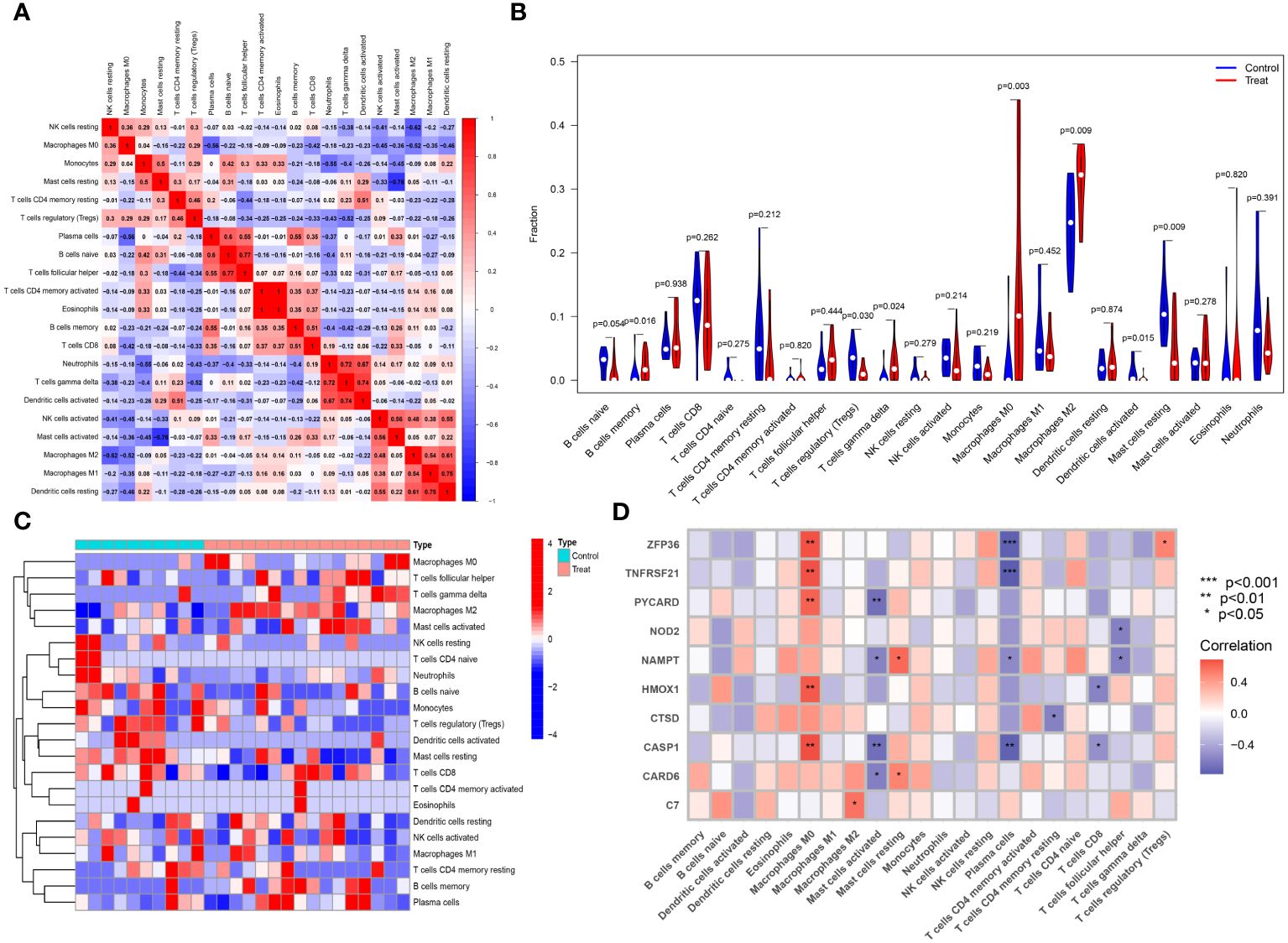
Figure 12 Immune cell infiltration composition in AS. (A) Heatmap of immune cell correlations in AS. (B) Violin plot of immune cell infiltration differences between early atherosclerotic plaques and advanced atherosclerotic plaques samples. (C) Heatmap of the proportion of various immune cells in early atherosclerotic plaques compared to advanced atherosclerotic plaques. (D) Heatmap showing correlations between immune cells and key genes.
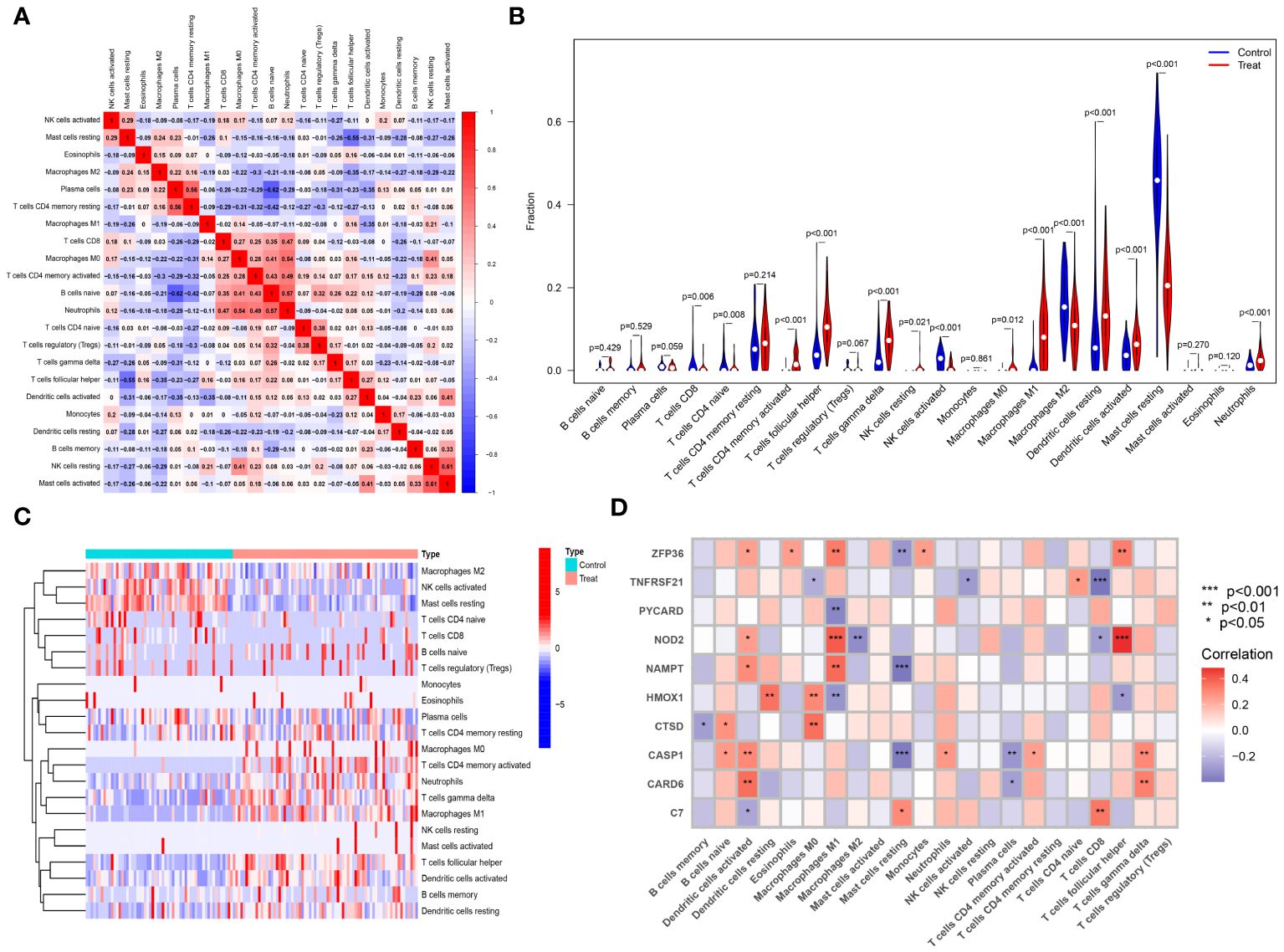
Figure 13 Immune cell infiltration composition in PsD. (A) Heatmap of immune cell correlations in PsD. (B) Violin plot of immune cell infiltration differences between skin lesions (LS) and adjacent normal tissues (NL). (C) Heatmap of the proportion of various immune cells in skin lesions (LS) and adjacent normal tissues (NL). (D) Heatmap showing correlations between immune cells and key genes.
3.7 Prediction of small molecule compounds targeting key genes for the treatment of atherosclerosis and psoriasis
Using the DGIdb database, we analyzed potential therapeutic drugs that could counteract abnormal mean gene expression. This study predicted drugs that might target the previously identified feature genes. Through DGIdb, 43 drugs targeting these genes were identified (Figure 14), including 23 drugs or molecular compounds such as NIVOCASAN, EMRICASAN, PRALNACASAN, BERKELEYAMIDE C, and CHEMBL337173, which interact with CASP1. Additionally, 12 drugs or compounds, including MIFAMURTIDE, MURABUTIDE, and CHEMBL1456848, were noted for their potential to modulate NAMPT expression. Drugs such as SORAFENIB, SUNITINIB, STANNSOPORFIN, and ASPIRIN were identified as potential targets for HMOX1. Regrettably, no drugs were found that could regulate the expression of ZFP36, C7, CARD6, PYCARD, and TNFRSF21, highlighting the need for further research in this area.
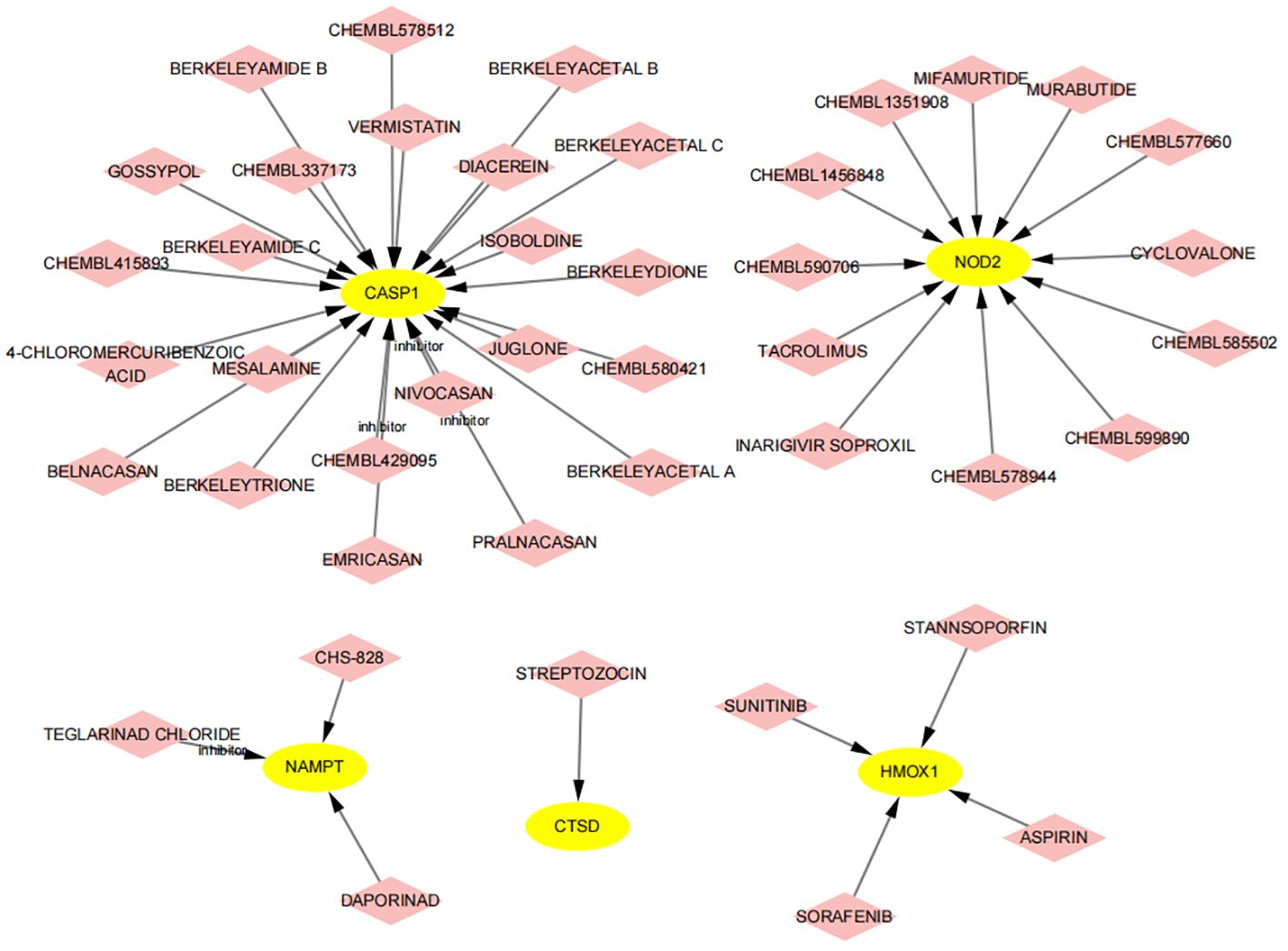
Figure 14 Prediction of drugs targeting feature genes. Yellow indicates feature genes, and pink represents drugs.
3.8 Constructing a ceRNA network based on key genes
Emerging research indicates that lncRNAs can bind competitively to target miRNAs through complementary base pairing, influencing gene expression in what is known as the ceRNA network (38). Based on this concept, we constructed a ceRNA network that includes 2 DE-FRGs and 7 DE-NRGs. The network consists of 106 nodes (9 hub genes, 76 miRNAs, and 21 lncRNAs) and 592 edges (Figure 15). According to the ceRNA hypothesis, literature review revealed that miR-142–5p is significantly expressed in the aortic plaques of AS patients and ApoE−/− mice, as well as in the skin of subjects with allergic reactions. Overexpression of miR-142–5p inhibits the expression of NAMPT. Additionally, studies have indicated that NEAT1 and XIST have putative binding sites for miR-142–5p, supporting that miR-142–5p is a direct target of NEAT1 and XIST (39, 40). Similarly, miR-192–5p is significantly elevated in the serum of patients with AS and psoriasis, and its overexpression suppresses NOD2 expression. Further research has identified specific binding sites of KCNQ1OT1 with miR-192–5p, suggesting that miR-192–5p is a direct target of KCNQ1OT1 (41–43). Consequently, we selected hsa-miR-142–5p and hsa-miR-192–5p to construct subnetworks for further analysis (Figure 15). By integrating the regulatory relationships between lncRNA, miRNA, and mRNA, the NEAT1/XIST-142–5p-NAMPT and KCNQ1OT1-miR-192–5p-NOD2 pathways may represent critical pathways in the pathogenesis of AS and PsD.
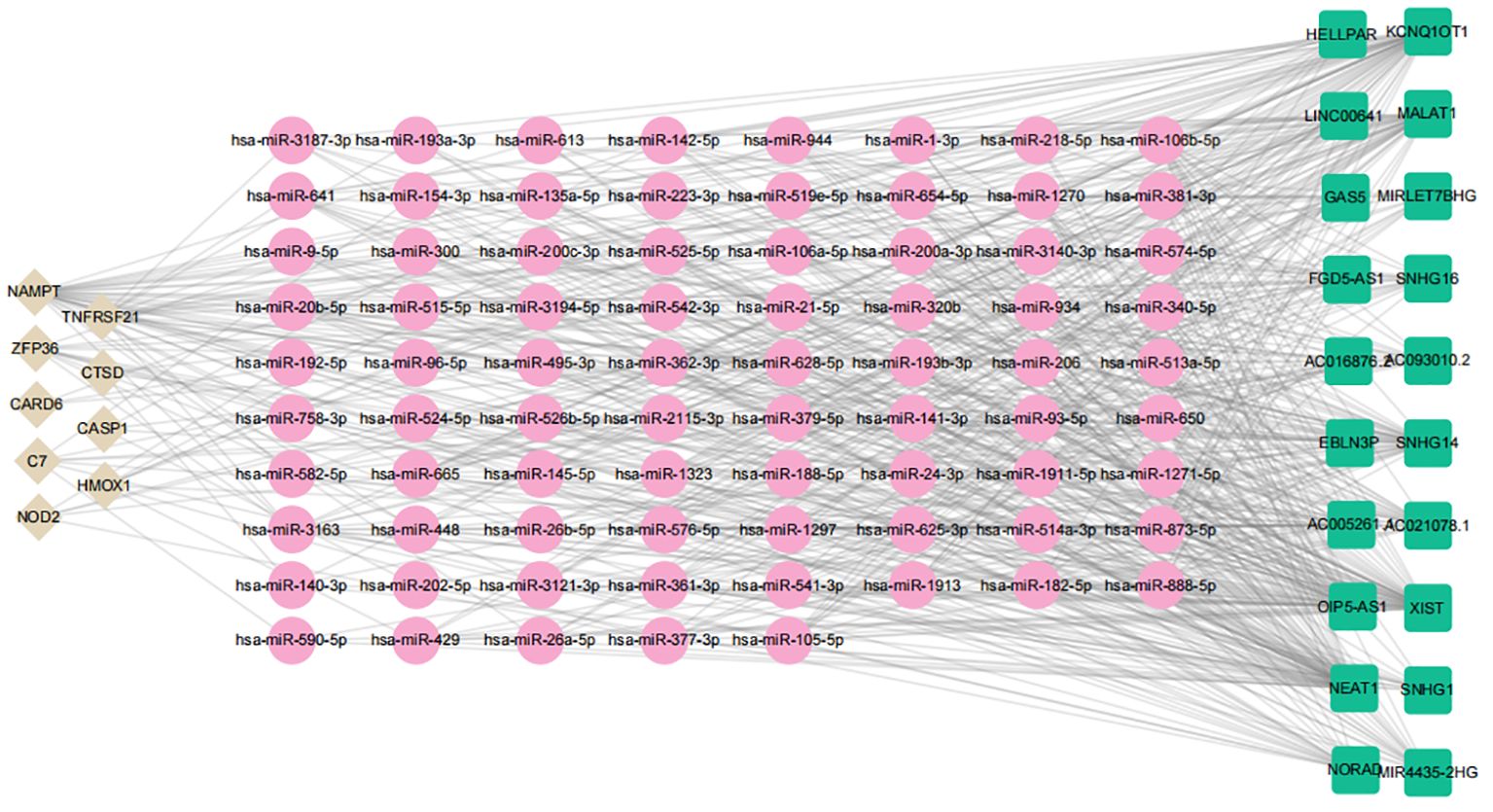
Figure 15 ceRNA regulatory network of hub genes. Brown diamonds represent hub genes; pink circles denote miRNAs, and green rectangles indicate lncRNAs.
4 Discussion
PsD and AS are prevalent diseases in clinical settings, causing significant economic losses and societal burdens worldwide (44, 45). Prior research has established a close association between PsD and systemic chronic inflammation, which also plays a critical role in AS and its complications, such as myocardial infarction and stroke (46). Chronic inflammation in PsD patients can lead to insulin resistance and endothelial cell dysfunction, thereby exacerbating AS and various cardiovascular diseases (47). Studies have demonstrated an increased risk of cardiovascular diseases in PsD patients, a risk that escalates with the severity and duration of the condition (48). Moreover, genes related to iron death and necroptotic apoptosis are implicated in the progression of both PsD and AS. However, the interplay between PsD, AS, and these apoptotic processes remains poorly understood (49–52). Consequently, our study aims to explore the shared characteristics and molecular pathways of iron death and necroptotic apoptosis genes in PsD and AS from a bioinformatics perspective, utilizing existing sequencing databases to offer novel insights into the pathogenesis of these diseases.
In this research, we identified 44 DE-FRGs and 30 DE-NRGs and conducted functional enrichment analysis. The 44 DE-FRGs were primarily enriched in pathways such as Ferroptosis, NOD-like receptor signaling, TNF signaling, PPAR signaling, and IL-17 signaling. Conversely, the 30 DE-NRGs exhibited significant enrichment in pathways such as Necroptosis, NOD-like receptor signaling, TNF signaling, and the p53 signaling pathway. These findings highlight the pivotal role of inflammation in both PsD and AS. Importantly, the NOD-like receptor signaling pathway and TNF signaling pathway were significantly prevalent in both diseases, underscoring their fundamental roles in the pathogenesis of these conditions. NOD-like receptors (NLRs), belonging to the cytoplasmic pattern recognition receptor family, are crucial to the innate immune system and are closely linked with the pathogenesis of AS (53). For example, a study found that two ferroptosis-related proteins, PTGS2 and ACSL4, and two necroptosis-related proteins, NLRP3 and caspase-1, are upregulated in the advanced stages of atherosclerosis (AS) and are positively correlated with the severity of AS. These findings support the credibility of the enriched results for ferroptosis and necroptosis in this study, suggesting that proteins involved in these processes may be potential targets for regulating AS, and their expression levels could serve as indicators of AS severity (54). Furthermore, compared to healthy individuals, NLRP3 mRNA is upregulated in the plaques and psoriatic dermatitis (PsD) epidermis of AS patients. Recent studies indicate that activation of the NLRP3 inflammasome can induce ferroptosis, highlighting that inhibiting NLRP3 activation may suppress the occurrence of ferroptosis in AS and PsD (55). Tranexamic acid (TXA) inhibits IL-17-induced ROS production, thereby suppressing IL-17-induced activation of NFκB, expression of the NLRP3 inflammasome, and Nrf2-mediated expression of keratin 17, thus exerting an anti-PsD effect (56). Moreover, NLRP3 expression levels in PsD samples are 3.5 to 4.3 times higher than in normal skin biopsy samples, indirectly indicating that inflammasome activation is closely associated with the pathogenesis of PsD (57). Additionally, TNF, a pro-inflammatory cytokine produced by activated leukocytes, targets endothelial cells and triggers a cascade of events, leading to endothelial dysfunction and accelerated development of AS (58). TNF also regulates the NF-κB signaling pathway, activates inflammatory genes, and promotes the expression of cell adhesion molecules, indirectly inducing endothelial cell apoptosis and necroptosis, thereby accelerating the progression of AS (59). The TNF signaling pathway is markedly upregulated in imiquimod-induced mouse psoriasis lesions, and TNF-α, a crucial target of this pathway, mediates inflammatory responses and is an inflammatory cytokine linked to PsD pathogenesis. Anti-TNF-α therapy has shown particular effectiveness in PsD treatment (60). These evidences show that ferroptosis-related genes and necroptosis-related genes related to AS and PsD are mainly enriched in immune and inflammatory signaling pathways, suggesting that immune-inflammatory signaling pathways mediated byferroptosis and necroptosis may be the potential mechanisms of AS and PsD.
Subsequently, we selected feature genes using three machine learning algorithms, focusing on 2 ferroptosis-related genes (NAMPT, ZFP36) and 8 necroptosis-related genes (C7, CARD6, CASP1, CTSD, HMOX1, NOD2, PYCARD, TNFRSF21). NAMPT, an enzyme involved in NAD biosynthesis, is highly expressed in the serum of neck AS patients, with significantly elevated plasma eNAMPT levels in ApoE knockout AS mice (61, 62). Additionally, eNAMPT may contribute to AS development by inducing endothelial dysfunction (63). Similarly, the NAMPT-mediated NAD salvage pathway enhances the epithelial autocrine inflammatory response in PsD, thus promoting its pathogenesis (64). This suggests that NAMPT-mediated inflammation may play a role in the development of both AS and PsD. ZFP36, minimally expressed in healthy aortas but significantly in endothelial cells and macrophages in mouse and human AS lesions, inhibits pro-inflammatory mRNA transcripts, thereby reducing vascular inflammation (65). Furthermore, ZFP36 family protein expression is diminished under chronic inflammatory conditions similar to those in PsD lesions, contributing to the onset of PsD’s inflammatory phenotype (66). Additionally, the human ZFP36 family comprises three members (ZFP36, ZFP36L1, and ZFP36L2), among which ZFP36 plays distinct roles compared to the other two. Different family members bind to varied DNA binding sites and regulate different molecular pathways. In other words, even if expressed at the same levels, ZFP36 may exert unique functions in transcriptional activity. The alternative splicing of ZFP36 could explain its higher expression levels in the PsD research cohort and lower levels in the validation cohort (65). HMOX1, highly expressed in the endothelium of AS mice, plays a role in reducing iron death in AS endothelial cells when knocked out, thus mitigating Fe2+ overload and lipid peroxidation (67). Elevated expression of HMOX1 in the skin of PsD patients is consistent with our findings (68), suggesting that inhibiting HMOX1 expression might be a shared target for AS and PsD treatment. CASP1, part of the inflammasome, is expressed at low levels in ApoE knockout mice, leading to decreased vascular inflammation and AS progression (69). Clinically, CASP1 expression is elevated in PsD patients, supporting our results (70). NOD2, pivotal in integrating endoplasmic reticulum stress and inflammation, is essential for endothelial cell apoptosis and AS progression (71). Previous research suggests that NOD2 deficiency leads to reduced lipid deposition and diminished inflammatory cell infiltration in AS plaques (72). Correspondingly, the observation of upregulated NOD2 in PsD epidermis supports these findings (73). Additionally, research data indicate that abnormalities in complement components and the resultant overactivation are associated with the formation of AS. Studies have found higher levels of complement component C7 in intimal thickening and fibrous plaques compared to normal tissues (74). However, another study confirmed that increased concentrations of C7, which is involved in the formation of the membrane attack complex, were not observed in the blood of patients with unstable plaques. This might be due to the process occurring locally within AS lesions, indirectly explaining why C7 exhibits higher expression in the AS research cohort and lower expression in the validation cohort (75). However, the roles of CARD6, CTSD, PYCARD, and TNFRSF21 in PsD and AS remain underexplored, emphasizing their significance in future research and the necessity to further investigate their specific mechanisms.
To further investigate the role of immune cells in PsD and AS, an extensive assessment of immune cell infiltration in these diseases was conducted using CIBERSORT. The findings indicated that in AS samples, memory B cells, gamma delta T cells, Macrophages M0, and Macrophages M2 were markedly present. Conversely, in PsD samples, an increase was noted in CD4 memory activated T cells, gamma delta T cells, Macrophages M0, M1, M2, activated Dendritic cells, and Neutrophils. The significant infiltration of gamma delta T cells and Macrophages in both PsD and AS led to an examination of their shared pathogenic mechanisms in these diseases. Recent studies have identified IL-23R+ gamma delta T cells to be prevalent in the aortic root of Ldlr-/- Il23rgfp/+ mice. It was found that the absence of specific gamma delta T cell subsets reduced aortic root AS lesions, and high-throughput sequencing data confirmed that gamma delta T cells predominantly express IL-23R and IL-17A in the aorta (76). Previous research has identified dermal gamma delta T cells as key sources of IL-17A, IL-22, and IL-17F, playing a crucial role in the initiation and development of PsD (77, 78). Furthermore, reports suggest that inhibiting the production of IL-17A by gamma delta T cells effectively reduces inflammatory symptoms in PsD skin tissue (79). Therefore, gamma delta T cells and their secreted cytokines are fundamental to the pathogenesis of both PsD and AS. Macrophages, vital to immune cell function in AS, efficiently remove necrotic and dead cells from lesions, thus alleviating tissue damage and the progression of AS (80). PsD patient epidermal tissues show a significant increase in macrophages, linked with hyperproliferation and inflammation. The persistence of skin inflammation in PsD is heavily dependent on macrophage recruitment and activation and the release of TNF-α (81, 82). In summary, the development of PsD and AS is intimately connected to the extent of immune cell infiltration, and understanding the underlying mechanisms of these immune cells is vital for the prevention and treatment of PsD and AS.
Furthermore, we sourced drug-gene interaction data from the DGIdb database, identifying a total of 43 potential drugs or compounds for treating PsD and AS. DIACEREIN, an anthraquinone derivative known for its anti-inflammatory, antipyretic, and analgesic properties, is commonly used in osteoarthritis treatment (83). Recent research indicates that DIACEREIN mitigates IL-1-induced skin inflammation in PsD and curbs the development of IL-1-induced AS (84). JUGLONE, a natural phenolic compound derived from walnut tree roots, stems, and leaves, has shown significant efficacy in alleviating endothelial dysfunction, oxidative stress, and NF-kB-driven inflammatory responses in diabetic mice, thus potentially preventing cardiovascular complications of diabetes (85). However, current literature does not document the use of JUGLONE in PsD treatment, emphasizing the need for further exploration of its application in PsD. Tacrolimus, extensively used as an immunosuppressant in various organ transplants, exhibits anti-inflammatory properties. Recent studies suggest that Tacrolimus may inhibit AS formation by curtailing ROS and NLRP3 inflammasome activation in macrophages, along with reducing IL-1β and IL-18 release (86). Tacrolimus is also recommended for PsD treatment, particularly in areas with thinner skin. The latest research indicates that microneedle patches laden with Tacrolimus nanocrystals can effectively ameliorate histopathological features of PsD skin and decrease TNF-α, IL-17A, and IL-23 levels (87). This study suggests that immune disorder-mediated inflammatory responses are instrumental in the onset of PsD and AS, indicating immunotherapy as a promising treatment approach for both conditions. While drug repurposing is a viable strategy for identifying therapeutic candidates, these proposed drugs and target genes require further validation through extensive animal studies and clinical trials.
To delve deeper into the mechanisms of 2 ferroptosis-related genes (NAMPT, ZFP36) and 8 necroptosis-related genes (C7, CARD6, CASP1, CTSD, HMOX1, NOD2, PYCARD, TNFRSF21) in AS and PsD, we developed a ceRNA network relevant to both conditions. This network highlighted NEAT1, XIST, KCNQ1OT as key lncRNAs, hsa-miR-3163, hsa-miR-93–5p, and hsa-miR-20b-5p as essential miRNAs, and ZFP36 and HMOX1 as critical mRNAs. NEAT1 exhibited increased expression in AS patients and ox-LDL-induced HAECs, and its downregulation significantly reduced the proliferation of ox-LDL-activated HAEC cells while promoting apoptosis (88). Similarly, XIST was upregulated in the serum of AS patients and Ox-LDL-induced VSMCs, with XIST inhibition leading to reduced cell vitality and increased apoptosis (89). Furthermore, miR-93–5p overexpression inhibited endothelial cell ferroptosis, and as previously mentioned, both ZFP36 and HMOX1 were upregulated in AS models (90). We postulate that XIST and NEAT1 may function as miR-93–5p sponges, impacting ZFP36 and HMOX1 expression and thereby contributing to AS progression. However, the regulatory influence of NEAT1, XIST, and KCNQ1OT on miRNAs in PsD remains unexplored, emphasizing the need for future research focusing on additional ncRNAs in PsD mechanisms.
In summary, we identified shared DE-FRGs and DE-NRGs in AS and PsD and performed enrichment analysis. Our findings revealed numerous common pathogenic mechanisms between AS and PsD, potentially mediated by specific hub genes. Using three machine learning algorithms, we pinpointed 2 ferroptosis-related genes (NAMPT, ZFP36) and 8 necroptosis-related genes (C7, CARD6, CASP1, CTSD, HMOX1, NOD2, PYCARD, TNFRSF21) as key biomarkers, which demonstrate positive or negative correlations with certain immune cells, potentially affecting the immune microenvironment in AS and PsD. Further investigation into these immune cells may assist in developing immunoregulatory treatments for AS and PsD. However, this study has certain limitations. Firstly, a major weakness is the insufficient sample size; in the future, we could incorporate additional datasets to expand the sample pool. Secondly, there might be bias in the selection of training and validation datasets. Although this study utilized publicly available datasets, they may not represent the broader AS or PsD patient populations. Thirdly, due to budget constraints, this study did not further explore and validate mechanisms and pathways between target organs, novel DEGs, and immune cells. Including external validation cohorts and conducting multifunctional experiments is crucial for our research. The former would enhance the robustness and generalizability of our findings, while the latter would provide deeper insights into unknown molecular mechanisms. We recommend future multidisciplinary collaborations and further basic experiments. Lastly, although our study validated the functions of NAMPT, ZFP36, CASP1, CTSD, HMOX1, and PYCARD through RT-qPCR and WB, the precise molecular regulatory mechanisms still require further investigation. More clinical samples are needed to confirm our findings. Moreover, the regulatory mechanisms of necroptosis and ferroptosis are highly complex. Although we identified NAMPT and ZFP36 as ferroptosis-related genes that may be associated with necroptosis-related genes such as CASP1, CTSD, HMOX1, and PYCARD, how they interact to influence the progression of AS and PsD warrants deeper study. We hope to construct stable cell lines with knockdown and overexpression of these genes in subsequent research to explore and validate the results obtained. Nonetheless, this study is limited as our conclusions are based solely on data analysis and lack experimental validation. Therefore, future animal studies are necessary to validate our findings. Despite these limitations, our research offers novel insights into the comorbidity of AS and PsD, providing new perspectives for exploring ferroptosis-related genes and necroptosis-related genes and molecular mechanisms in these diseases.
Data availability statement
The datasets presented in this study can be found in online repositories. The names of the repository/repositories and accession number(s) can be found in the article/supplementary material.
Ethics statement
The manuscript presents research on animals that do not require ethical approval for their study.
Author contributions
JF: Conceptualization, Data curation, Formal analysis, Methodology, Software, Validation, Writing – original draft. TZ: Project administration, Software, Writing – review & editing. XT: Methodology, Supervision, Writing – review & editing. SL: Investigation, Methodology, Supervision, Writing – original draft. S-LZ: Project administration, Writing – review & editing.
Funding
The author(s) declare that no financial support was received for the research, authorship, and/or publication of this article.
Conflict of interest
The authors declare that the research was conducted in the absence of any commercial or financial relationships that could be construed as a potential conflict of interest.
Publisher’s note
All claims expressed in this article are solely those of the authors and do not necessarily represent those of their affiliated organizations, or those of the publisher, the editors and the reviewers. Any product that may be evaluated in this article, or claim that may be made by its manufacturer, is not guaranteed or endorsed by the publisher.
References
1. Ayala-Fontánez N, Soler DC, McCormick TS. Current knowledge on psoriasis and autoimmune diseases. Psoriasis (Auckl). (2016) 6:7–32. doi: 10.2147/PTT.S64950
2. Boehncke WH. Systemic inflammation and cardiovascular comorbidity in psoriasis patients: causes and consequences. Front Immunol. (2018) 9:579. doi: 10.3389/fimmu.2018.00579
3. Piros EA, Szabo A, Rencz F, Brodszky V, Szalai K, Galajda N, et al. Impact of interleukin-17 inhibitor therapy on arterial intima-media thickness among severe psoriatic patients. Life (Basel). (2021) 11:919. doi: 10.3390/life11090919
4. Lai YC, Yew YW. Psoriasis as an independent risk factor for cardiovascular disease: an epidemiologic analysis using a national database. J Cutan Med Surg. (2016) 20:327–33. doi: 10.1177/1203475415602842
5. Armstrong AW, Harskamp CT, Ledo L, Rogers JH, Armstrong EJ. Coronary artery disease in patients with psoriasis referred for coronary angiography. Am J Cardiol. (2012) 109:976–80. doi: 10.1016/j.amjcard.2011.11.025
6. Egeberg A, Gisondi P, Carrascosa JM, Warren RB, Mrowietz U. The role of the interleukin-23/Th17 pathway in cardiometabolic comorbidity associated with psoriasis. J Eur Acad Dermatol Venereol. (2020) 34:1695–706. doi: 10.1111/jdv.16273
7. Armstrong AW, Voyles SV, Armstrong EJ, Fuller EN, Rutledge JC. A tale of two plaques: convergent mechanisms of T-cell-mediated inflammation in psoriasis and atherosclerosis. Exp Dermatol. (2011) 20:544–9. doi: 10.1111/j.1600-0625.2011.01308.x
8. Toussirot E. The IL23/Th17 pathway as a therapeutic target in chronic inflammatory diseases. Inflammation Allergy Drug Targets. (2012) 11:159–68. doi: 10.2174/187152812800392805
9. Puig L. The role of IL23 in the treatment of psoriasis. Expert Rev Clin Immunol. (2017) 13:525–34. doi: 10.1080/1744666X.2017.1292137
10. Xie LH, Fefelova N, Pamarthi SH, Gwathmey JK. Molecular mechanisms of ferroptosis and relevance to cardiovascular disease. Cells. (2022) 11:2726. doi: 10.3390/cells11172726
11. Li X, Tao X, Ding X. An integrative analysis to reveal that CLEC2B and ferroptosis may bridge the gap between psoriatic arthritis and cancer development. Sci Rep. (2022) 12:14653. doi: 10.1038/s41598-022-19135-2
12. Arbiser JL, Bonner MY, Ward N, Elsey J, Rao S. Selenium unmasks protective iron armor: A possible defense against cutaneous inflammation and cancer. Biochim Biophys Acta Gen Subj. (2018) 1862:2518–27. doi: 10.1016/j.bbagen.2018.05.018
13. Li S, Luo X, Zhang S, Su Y, Deng M, Zhu Y, et al. Ferroptosis activation contributes to the formation of skin lesions in psoriasis vulgaris. Antioxidants (Basel). (2023) 12:310. doi: 10.3390/antiox12020310
14. Bai T, Li M, Liu Y, Qiao Z, Wang Z. Inhibition of ferroptosis alleviates atherosclerosis through attenuating lipid peroxidation and endothelial dysfunction in mouse aortic endothelial cell. Free Radic Biol Med. (2020) 160:92–102. doi: 10.1016/j.freeradbiomed.2020.07.026
15. Kluck GEG, Qian AS, Sakarya EH, Quach H, Deng YD, Trigatti BL. Apolipoprotein A1 protects against necrotic core development in atherosclerotic plaques: PDZK1-dependent high-density lipoprotein suppression of necroptosis in macrophages. Arterioscler Thromb Vasc Biol. (2023) 43:45–63. doi: 10.1161/ATVBAHA.122.318062
16. Duan X, Liu X, Liu N, Huang Y, Jin Z, Zhang S, et al. Inhibition of keratinocyte necroptosis mediated by RIPK1/RIPK3/MLKL provides a protective effect against psoriatic inflammation. Cell Death Dis. (2020) 11:134. doi: 10.1038/s41419-020-2328-0
17. Zhang Y, Su SS, Zhao S, Yang Z, Zhong CQ, Chen X, et al. RIP1 autophosphorylation is promoted by mitochondrial ROS and is essential for RIP3 recruitment into necrosome. Nat Commun. (2017) 8:14329. doi: 10.1038/ncomms14329
18. Basit F, van Oppen LM, Schöckel L, Bossenbroek HM, van Emst-de Vries SE, Hermeling JC, et al. Mitochondrial complex I inhibition triggers a mitophagy-dependent ROS increase leading to necroptosis and ferroptosis in melanoma cells. Cell Death Dis. (2017) 8:e2716. doi: 10.1038/cddis.2017.133
19. Müller T, Dewitz C, Schmitz J, Schröder AS, Bräsen JH, Stockwell BR, et al. Necroptosis and ferroptosis are alternative cell death pathways that operate in acute kidney failure. Cell Mol Life Sci. (2017) 74:3631–45. doi: 10.1007/s00018-017-2547-4
20. Riegman M, Sagie L, Galed C, Levin T, Steinberg N, Dixon SJ, et al. Ferroptosis occurs through an osmotic mechanism and propagates independently of cell rupture. Nat Cell Biol. (2020) 22:1042–8. doi: 10.1038/s41556-020-0565-1
21. Su W, Zhao Y, Wei Y, Zhang X, Ji J, Yang S. Exploring the pathogenesis of psoriasis complicated with atherosclerosis via microarray data analysis. Front Immunol. (2021) 12:667690. doi: 10.3389/fimmu.2021.667690
22. Ding SA, Liu H, Zheng R, Ge Y, Fu Z, Mei J, et al. Downregulation of MYBL1 in endothelial cells contributes to atherosclerosis by repressing PLEKHM1-inducing autophagy. Cell Biol Toxicol. (2024) 40:40. doi: 10.1007/s10565-024-09873-6
23. Zou A, Chen Y, Liu T, Yang T, Zhou B. Identification and verification of three autophagy-related genes as potential biomarkers for the diagnosis of psoriasis. Sci Rep. (2023) 13:22918. doi: 10.1038/s41598-023-49764-0
24. Wang L, He W, Wang X, Wang J, Wei X, Wu D, et al. Potential diagnostic markers shared between non-alcoholic fatty liver disease and atherosclerosis determined by machine learning and bioinformatic analysis. Front Med (Lausanne). (2024) 11:1322102. doi: 10.3389/fmed.2024.1322102
25. Li Y, Li L, Tian Y, Luo J, Huang J, Zhang L, et al. Identification of novel immune subtypes and potential hub genes of patients with psoriasis. J Transl Med. (2023) 21:182. doi: 10.1186/s12967-023-03923-z
26. Ritchie ME, Phipson B, Wu D, Hu Y, Law CW, Shi W, et al. limma powers differential expression analyses for RNA-sequencing and microarray studies. Nucleic Acids Res. (2015) 43:e47. doi: 10.1093/nar/gkv007
27. Ito K, Murphy D. Application of ggplot2 to pharmacometric graphics. CPT Pharmacometrics Syst Pharmacol. (2013) 2:e79. doi: 10.1038/psp.2013.56
28. Langfelder P, Horvath S. WGCNA: an R package for weighted correlation network analysis. BMC Bioinf. (2008) 9:559. doi: 10.1186/1471-2105-9-559
29. Huang da W, Sherman BT, Lempicki RA. Bioinformatics enrichment tools: paths toward the comprehensive functional analysis of large gene lists. Nucleic Acids Res. (2009) 37:1–13. doi: 10.1093/nar/gkn923
30. Newman AM, Liu CL, Green MR, Gentles AJ, Feng W, Xu Y, et al. Robust enumeration of cell subsets from tissue expression profiles. Nat Methods. (2015) 12:453–7. doi: 10.1038/nmeth.3337
31. Friedman J, Hastie T, Tibshirani R. Regularization paths for generalized linear models via coordinate descent. J Stat Software. (2010) 33:1–22. doi: 10.18637/jss.v033.i01
32. Moradi S, Tapak L, Afshar S. Identification of novel noninvasive diagnostics biomarkers in the parkinson's diseases and improving the disease classification using support vector machine. BioMed Res Int. (2022) 2022:5009892. doi: 10.1155/2022/5009892
33. Izmirlian G. Application of the random forest classification algorithm to a SELDI-TOF proteomics study in the setting of a cancer prevention trial. Ann N Y Acad Sci. (2004) 1020:154–74. doi: 10.1196/annals.1310.015
34. Robin X, Turck N, Hainard A, Tiberti N, Lisacek F, Sanchez JC, et al. pROC: an open-source package for R and S+ to analyze and compare ROC curves. BMC Bioinf. (2011) 12:77. doi: 10.1186/1471-2105-12-77
35. Cotto KC, Wagner AH, Feng YY, Kiwala S, Coffman AC, Spies G, et al. DGIdb 3.0: a redesign and expansion of the drug-gene interaction database. Nucleic Acids Res. (2018) 46:D1068–73. doi: 10.1093/nar/gkx1143
36. Liu M, Zhang G, Wang Z, Liu X, He K, Luo R, et al. FOXE1 contributes to the development of psoriasis by regulating WNT5A. J Invest Dermatol. (2023) 143:2366–77.e7. doi: 10.1016/j.jid.2023.04.035
37. Zhu L, Bao Y, Liu Z, Liu J, Li Z, Sun X, et al. Gualou-Xiebai herb pair ameliorate atherosclerosis in HFD-induced ApoE-/- mice and inhibit the ox-LDL-induced injury of HUVECs by regulating the Nrf2-mediated ferroptosis. J Ethnopharmacol. (2024) 326:117892. doi: 10.1016/j.jep.2024.117892
38. Bhattacharjee R, Prabhakar N, Kumar L, Bhattacharjee A, Kar S, Malik S, et al. Crosstalk between long noncoding RNA and microRNA in Cancer. Cell Oncol (Dordr). (2023) 46:885–908. doi: 10.1007/s13402-023-00806-9
39. Zhang W, Wang YD, Xing YJ, Liu PJ, Yang JH. Silencing of circ-NT5C2 retards the progression of IL-1β-induced osteoarthritis in an in vitro cell model by targeting the miR-142-5p/NAMPT axis. Microbiol Immunol. (2023) 67:129–41. doi: 10.1111/1348-0421.13046
40. Tang B, Li W, Ji T, Li X, Qu X, Feng L, et al. Downregulation of XIST ameliorates acute kidney injury by sponging miR-142-5p and targeting PDCD4. J Cell Physiol. (2020) 235:8852–63. doi: 10.1002/jcp.29729
41. Ke X, Liao Z, Luo X, Chen JQ, Deng M, Huang Y, et al. Endothelial colony-forming cell-derived exosomal miR-21-5p regulates autophagic flux to promote vascular endothelial repair by inhibiting SIPL1A2 in atherosclerosis. Cell Commun Signal. (2022) 20:30. doi: 10.1186/s12964-022-00828-0
42. Zhao L, Wang B, Sun L, Sun B, Li Y. Association of miR-192-5p with Atherosclerosis and its Effect on Proliferation and Migration of Vascular Smooth Muscle Cells. Mol Biotechnol. (2021) 63:1244–51. doi: 10.1007/s12033-021-00376-x
43. Yang Z, Zeng B, Tang X, Wang H, Wang C, Yan Z, et al. MicroRNA-146a and miR-99a are potential biomarkers for disease activity and clinical efficacy assessment in psoriasis patients treated with traditional Chinese medicine. J Ethnopharmacol. (2016) 194:727–32. doi: 10.1016/j.jep.2016.08.028
44. Griffiths CEM, Armstrong AW, Gudjonsson JE, Barker JNWN. Psoriasis. Lancet. (2021) 397:1301–15. doi: 10.1016/S0140-6736(20)32549-6
45. Tang X, Huang Z, Wang F, Chen J, Qin D, Peng D, et al. Macrophage-specific deletion of MIC26 (APOO) mitigates advanced atherosclerosis by increasing efferocytosis. Atherosclerosis. (2023) 386:117374. doi: 10.1016/j.atherosclerosis.2023.117374
46. Wierzbowska-Drabik K, Lesiak A, Skibińska M, Niedźwiedź M, Kasprzak JD, Narbutt J. Psoriasis and atherosclerosis-skin, joints, and cardiovascular story of two plaques in relation to the treatment with biologics. Int J Mol Sci. (2021) 22:10402. doi: 10.3390/ijms221910402
47. Boehncke WH, Boehncke S, Tobin AM, Kirby B, et al. The 'psoriatic march': a concept of how severe psoriasis may drive cardiovascular comorbidity. Exp Dermatol. (2011) 20:303–7. doi: 10.1111/exd.2011.20.issue-4
48. Samarasekera EJ, Neilson JM, Warren RB, Parnham J, Smith CH. Incidence of cardiovascular disease in individuals with psoriasis: a systematic review and meta-analysis. J Invest Dermatol. (2013) 133:2340–6. doi: 10.1038/jid.2013.149
49. Liu L, Kang XX. ACSL4 is overexpressed in psoriasis and enhances inflammatory responses by activating ferroptosis. Biochem Biophys Res Commun. (2022) 623:1–8. doi: 10.1016/j.bbrc.2022.07.041
50. Jiang BW, Zhang WJ, Wang Y, Tan LP, Bao YL, Song ZB, et al. Convallatoxin induces HaCaT cell necroptosis and ameliorates skin lesions in psoriasis-like mouse models. BioMed Pharmacother. (2020) 121:109615. doi: 10.1016/j.biopha.2019.109615
51. Wang X, Zhang M, Mao C, Zhang C, Ma W, Tang J, et al. Icariin alleviates ferroptosis-related atherosclerosis by promoting autophagy in xo-LDL-induced vascular endothelial cell injury and atherosclerotic mice. Phytother Res. (2023) 37:3951–63. doi: 10.1002/ptr.7854
52. Karunakaran D, Geoffrion M, Wei L, Gan W, Richards L, Shangari P, et al. Targeting macrophage necroptosis for therapeutic and diagnostic interventions in atherosclerosis. Sci Adv. (2016) 2:e1600224. doi: 10.1126/sciadv.1600224
53. Kotlyarov S. Effects of atherogenic factors on endothelial cells: bioinformatics analysis of differentially expressed genes and signaling pathways. Biomedicines. (2023) 11:1216. doi: 10.3390/biomedicines11041216
54. Zhou Y, Zhou H, Hua L, Hou C, Jia Q, Chen J, et al. Verification of ferroptosis and pyroptosis and identification of PTGS2 as the hub gene in human coronary artery atherosclerosis. Free Radic Biol Med. (2021) 171:55–68. doi: 10.1016/j.freeradbiomed.2021.05.009
55. Paramel Varghese G, Folkersen L, Strawbridge RJ, Halvorsen B, Yndestad A, Ranheim T, et al. NLRP3 inflammasome expression and activation in human atherosclerosis. J Am Heart Assoc. (2016) 5:e003031. doi: 10.1161/JAHA.115.003031
56. Hseu JH, Chan CI, Vadivalagan C, Chen SJ, Yen HR, Hseu YC, et al. Tranexamic acid improves psoriasis-like skin inflammation: Evidence from in vivo and in vitro studies. BioMed Pharmacother. (2023) 166:115307. doi: 10.1016/j.biopha.2023.115307
57. Su F, Xia Y, Huang M, Zhang L, Zhang L, Chen L, et al. Expression of NLPR3 in psoriasis is associated with enhancement of interleukin-1β and caspase-1. Med Sci Monit. (2018) 24:7909–13. doi: 10.12659/MSM.911347
58. Aldokhayyil M, Gomez DH, Cook MD, Kavazis AN, Roberts MD, Geetha T, et al. Influence of race and high laminar shear stress on TNFR1 signaling in endothelial cells. Int J Mol Sci. (2023) 24:14723. doi: 10.3390/ijms241914723
59. Zhao Y, Shao C, Zhou H, Yu L, Bao Y, Mao Q, et al. Salvianolic acid B inhibits atherosclerosis and TNF-α-induced inflammation by regulating NF-κB/NLRP3 signaling pathway. Phytomedicine. (2023) 119:155002. doi: 10.1016/j.phymed.2023.155002
60. Chen Y, Wang Y, Song S, Zhang X, Wu L, Wu J, et al. Topical application of baicalin combined with eChinacoside ameliorates psoriatic skin lesions by suppressing the inflammation-related TNF signaling pathway and the angiogenesis-related VEGF signaling pathway. ACS Omega. (2023) 8:40260–76. doi: 10.1021/acsomega.3c04281
61. Zhong M, Tan HW, Gong HP, Wang SF, Zhang Y, Zhang W. Increased serum visfatin in patients with metabolic syndrome and carotid atherosclerosis. Clin Endocrinol (Oxf). (2008) 69:878–84. doi: 10.1111/j.1365-2265.2008.03248.x
62. Kong YY, Li GQ, Zhang WJ, Hua X, Zhou CC, Xu TY, et al. Nicotinamide phosphoribosyltransferase aggravates inflammation and promotes atherosclerosis in ApoE knockout mice. Acta Pharmacol Sin. (2019) 40:1184–92. doi: 10.1038/s41401-018-0207-3
63. Semerena E, Nencioni A, Masternak K. Extracellular nicotinamide phosphoribosyltransferase: role in disease pathophysiology and as a biomarker. Front Immunol. (2023) 14:1268756. doi: 10.3389/fimmu.2023.1268756
64. Mercurio L, Morelli M, Scarponi C, Scaglione GL, Pallotta S, Avitabile D, et al. Enhanced NAMPT-mediated NAD salvage pathway contributes to psoriasis pathogenesis by amplifying epithelial auto-inflammatory circuits. Int J Mol Sci. (2021) 22:6860. doi: 10.3390/ijms22136860
65. Zhang H, Taylor WR, Joseph G, Caracciolo V, Gonzales DM, Sidell N, et al. mRNA-binding protein ZFP36 is expressed in atherosclerotic lesions and reduces inflammation in aortic endothelial cells. Arterioscler Thromb Vasc Biol. (2013) 33:1212–20. doi: 10.1161/ATVBAHA.113.301496
66. Angiolilli C, Leijten EFA, Bekker CPJ, Eeftink E, Giovannone B, Nordkamp MO, et al. ZFP36 family members regulate the proinflammatory features of psoriatic dermal fibroblasts. J Invest Dermatol. (2022) 142:402–13. doi: 10.1016/j.jid.2021.06.030
67. Meng Z, Liang H, Zhao J, Gao J, Liu C, Ma X, et al. HMOX1 upregulation promotes ferroptosis in diabetic atherosclerosis. Life Sci. (2021) 284:119935. doi: 10.1016/j.lfs.2021.119935
68. Hanselmann C, Mauch C, Werner S. Haem oxygenase-1: a novel player in cutaneous wound repair and psoriasis? Biochem J. (2001) 353:459–66. doi: 10.1042/bj3530459
69. Gage J, Hasu M, Thabet M, Whitman SCl. Caspase-1 deficiency decreases atherosclerosis in apolipoprotein E-null mice. Can J Cardiol. (2012) 28:222–9. doi: 10.1016/j.cjca.2011.10.013
70. Verma D, Fekri SZ, Sigurdardottir G, Bivik Eding C, Sandin C, Enerbäck C. Enhanced inflammasome activity in patients with psoriasis promotes systemic inflammation. J Invest Dermatol. (2021) 141:586–95.e5. doi: 10.1016/j.jid.2020.07.012
71. Liu HQ, Zhang XY, Edfeldt K, Nijhuis MO, Idborg H, Bäck M, et al. NOD2-mediated innate immune signaling regulates the eicosanoids in atherosclerosis. Arterioscler Thromb Vasc Biol. (2013) 33:2193–201. doi: 10.1161/ATVBAHA.113.301715
72. Vlacil AK, Schuett J, Ruppert V, Soufi M, Oberoi R, Shahin K, et al. Deficiency of Nucleotide-binding oligomerization domain-containing proteins (NOD) 1 and 2 reduces atherosclerosis. Basic Res Cardiol. (2020) 115:47. doi: 10.1007/s00395-020-0806-2
73. Tervaniemi MH, Katayama S, Skoog T, Siitonen HA, Vuola J, Nuutila K, et al. NOD-like receptor signaling and inflammasome-related pathways are highlighted in psoriatic epidermis. Sci Rep. (2016) 6:22745. doi: 10.1038/srep22745
74. Vlaicu SI, Tatomir A, Rus V, Mekala AP, Mircea PA, Niculescu F, et al. The role of complement activation in atherogenesis: the first 40 years. Immunol Res. (2016) 64:1–13. doi: 10.1007/s12026-015-8669-6
75. Stakhneva EM, Kashtanova EV, Polonskaya YV, Striukova EV, Shramko VS, Sadovski EV, et al. The search for associations of serum proteins with the presence of unstable atherosclerotic plaque in coronary atherosclerosis. Int J Mol Sci. (2022) 23:12795. doi: 10.3390/ijms232112795
76. Gil-Pulido J, Amézaga N, Jorgacevic I, Manthey HD, Rösch M, Brand T, et al. Interleukin-23 receptor expressing γδ T cells locally promote early atherosclerotic lesion formation and plaque necrosis in mice. Cardiovasc Res. (2022) 118:2932–45. doi: 10.1093/cvr/cvab359
77. Laggner U, Di Meglio P, Perera GK, Hundhausen C, Lacy KE, Ali N, et al. Identification of a novel proinflammatory human skin-homing Vγ9Vδ2 T cell subset with a potential role in psoriasis. J Immunol. (2011) 187:2783–93. doi: 10.4049/jimmunol.1100804
78. Shibata S, Tada Y, Hau CS, Mitsui A, Kamata M, Asano Y, et al. Adiponectin regulates psoriasiform skin inflammation by suppressing IL-17 production from γδ-T cells. Nat Commun. (2015) 6:7687. doi: 10.1038/ncomms8687
79. Cai Y, Shen X, Ding C, Qi C, Li K, Li X, et al. Pivotal role of dermal IL-17-producing γδ T cells in skin inflammation. Immunity. (2011) 35:596–610. doi: 10.1016/j.immuni.2011.08.001
80. Ia D, Chen S, Bai P, Luo C, Liu J, Sun A, et al. Cardiac resident macrophage-derived legumain improves cardiac repair by promoting clearance and degradation of apoptotic cardiomyocytes after myocardial infarction. Circulation. (2022) 145:1542–56. doi: 10.1161/CIRCULATIONAHA.121.057549
81. Wang H, Peters T, Kess D, Sindrilaru A, Oreshkova T, Van Rooijen N, et al. Activated macrophages are essential in a murine model for T cell-mediated chronic psoriasiform skin inflammation. J Clin Invest. (2006) 116:2105–14. doi: 10.1172/JCI27180
82. Stratis A, Pasparakis M, Rupec RA, Markur D, Hartmann K, Scharffetter-Kochanek K, et al. Pathogenic role for skin macrophages in a mouse model of keratinocyte-induced psoriasis-like skin inflammation. J Clin Invest. (2006) 116:2094–104. doi: 10.1172/JCI27179
83. Fouad AA, Abdel-Aziz AM, Hamouda AAH. Diacerein downregulates NLRP3/caspase-1/IL-1β and IL-6/STAT3 pathways of inflammation and apoptosis in a rat model of cadmium testicular toxicity. Biol Trace Elem Res. (2020) 195:499–505. doi: 10.1007/s12011-019-01865-6
84. Mohan GC, Zhang H, Bao L, Many B, Chan LS. Diacerein inhibits the pro-atherogenic & pro-inflammatory effects of IL-1 on human keratinocytes & endothelial cells. PloS One. (2017) 12:e0173981. doi: 10.1371/journal.pone.0173981
85. Costantino S, Paneni F, Lüscher TF, Cosentino F, et al. Pin1 inhibitor Juglone prevents diabetic vascular dysfunction. Int J Cardiol. (2016) 203:702–7. doi: 10.1016/j.ijcard.2015.10.221
86. Li X, Shang X, Sun L. Tacrolimus reduces atherosclerotic plaque formation in ApoE-/- mice by inhibiting NLRP3 inflammatory corpuscles. Exp Ther Med. (2020) 19:1393–9.
87. Men Z, Su T, Tang Z, Liang J, Shen T, et al. Tacrolimus nanocrystals microneedle patch for plaque psoriasis. Int J Pharm. (2022) 627:122207. doi: 10.1016/j.ijpharm.2022.122207
88. Zhang X, Guan MX, Jiang QH, Li S, Zhang HY, Wu ZG, et al. NEAT1 knockdown suppresses endothelial cell proliferation and induces apoptosis by regulating miR−638/AKT/mTOR signaling in atherosclerosis. Oncol Rep. (2020) 44:115–25. doi: 10.3892/or
89. Yang K, Xue Y, Gao X. LncRNA XIST promotes atherosclerosis by regulating miR-599/TLR4 axis. Inflammation. (2021) 44:965–73.
Keywords: psoriasis, atherosclerosis, ferroptosis, necroptosis, ceRNA network, immune infiltration
Citation: Fan J, Zhu T, Tian X, Liu S and Zhang S-L (2024) Exploration of ferroptosis and necroptosis-related genes and potential molecular mechanisms in psoriasis and atherosclerosis. Front. Immunol. 15:1372303. doi: 10.3389/fimmu.2024.1372303
Received: 17 January 2024; Accepted: 21 June 2024;
Published: 12 July 2024.
Edited by:
Yi Wang, Sichuan Academy of Medical Sciences and Sichuan Provincial People’s Hospital, ChinaReviewed by:
Xiaodong Zou, The Chinese University of Hong Kong, Shenzhen, ChinaLintao Liu, Houston Methodist Research Institute, United States
Ku-Chi Tsao, Cornell University, United States
Copyright © 2024 Fan, Zhu, Tian, Liu and Zhang. This is an open-access article distributed under the terms of the Creative Commons Attribution License (CC BY). The use, distribution or reproduction in other forums is permitted, provided the original author(s) and the copyright owner(s) are credited and that the original publication in this journal is cited, in accordance with accepted academic practice. No use, distribution or reproduction is permitted which does not comply with these terms.
*Correspondence: Jilin Fan, ZmFuamlsaW5zZHp5eUAxNjMuY29t; Shi-Liang Zhang, emhhbmdzaGlsaWFuZ3NkQDEyNi5jb20=