- 1Department of Computer Science, City University of Hong Kong, Kowloon, China
- 2City University of Hong Kong Shenzhen Research Institute, Shenzhen, Guangdong, China
- 3School of Mathematical Sciences, Dalian University of Technology, Dalian, Liaoning, China
Numerous studies have shown that immune checkpoint inhibitor (ICI) immunotherapy has great potential as a cancer treatment, leading to significant clinical improvements in numerous cases. However, it benefits a minority of patients, underscoring the importance of discovering reliable biomarkers that can be used to screen for potential beneficiaries and ultimately reduce the risk of overtreatment. Our comprehensive review focuses on the latest advancements in predictive biomarkers for ICI therapy, particularly emphasizing those that enhance the efficacy of programmed cell death protein 1 (PD-1)/programmed cell death-ligand 1 (PD-L1) inhibitors and cytotoxic T-lymphocyte antigen-4 (CTLA-4) inhibitors immunotherapies. We explore biomarkers derived from various sources, including tumor cells, the tumor immune microenvironment (TIME), body fluids, gut microbes, and metabolites. Among them, tumor cells-derived biomarkers include tumor mutational burden (TMB) biomarker, tumor neoantigen burden (TNB) biomarker, microsatellite instability (MSI) biomarker, PD-L1 expression biomarker, mutated gene biomarkers in pathways, and epigenetic biomarkers. TIME-derived biomarkers include immune landscape of TIME biomarkers, inhibitory checkpoints biomarkers, and immune repertoire biomarkers. We also discuss various techniques used to detect and assess these biomarkers, detailing their respective datasets, strengths, weaknesses, and evaluative metrics. Furthermore, we present a comprehensive review of computer models for predicting the response to ICI therapy. The computer models include knowledge-based mechanistic models and data-based machine learning (ML) models. Among the knowledge-based mechanistic models are pharmacokinetic/pharmacodynamic (PK/PD) models, partial differential equation (PDE) models, signal networks-based models, quantitative systems pharmacology (QSP) models, and agent-based models (ABMs). ML models include linear regression models, logistic regression models, support vector machine (SVM)/random forest/extra trees/k-nearest neighbors (KNN) models, artificial neural network (ANN) and deep learning models. Additionally, there are hybrid models of systems biology and ML. We summarized the details of these models, outlining the datasets they utilize, their evaluation methods/metrics, and their respective strengths and limitations. By summarizing the major advances in the research on predictive biomarkers and computer models for the therapeutic effect and clinical utility of tumor ICI, we aim to assist researchers in choosing appropriate biomarkers or computer models for research exploration and help clinicians conduct precision medicine by selecting the best biomarkers.
1 Introduction
In the last few years, tumor immunotherapy research has advanced rapidly due to the emergence of innovative therapies such as immune checkpoint inhibitor (ICI) immunotherapy, cell immunotherapy, and cancer vaccine (1). Compared to conventional treatments, tumor immunotherapy has exhibited longer-lasting effects (2–4). ICI therapy is a leading research direction of tumor immunotherapies, with the most representative ICI being programmed cell death protein 1 (PD-1)/programmed cell death-ligand 1 (PD-L1) inhibitors and cytotoxic T-lymphocyte antigen-4 (CTLA-4) inhibitors. These inhibitors restart the human immune system to fight cancer, such as liver, lung, colon, breast, skin, etc. (5). Yet, only a subset of patients achieves enduring efficacy and survival with ICI therapy. Determining biomarkers that effectively identify patients that will benefit from ICI would minimize unnecessary therapy costs and severe toxicity for the non-responders. Though biomarkers have guided ICI precision therapy to some extent, they still only benefit a restricted number of patients (6). Computer models can simulate and reproduce dynamic changes in the immune system during immunotherapy, conferring unparalleled advantages in inferring the law of immunotherapy and predicting tumor ICI immunotherapy response (7, 8).
In this review, we focused on reviewing predictive biomarkers that impact the effectiveness of ICI therapy from the perspective of tumor cells, TIME, and other dimensions (Figure 1). We also discussed the various detection techniques for these biomarkers. Moreover, we summarized the detailed information of these biomarkers, outlining the datasets they adopt, their advantages and disadvantages, and the methods or indicators for evaluation (Supplementary Table S1). We also explored the limitations and challenges of biomarker research for ICI therapy, such as the impact of tumor heterogeneity, the lack of standardization in biomarker detection and analysis, and the difficulty in translating research findings into clinical practice.
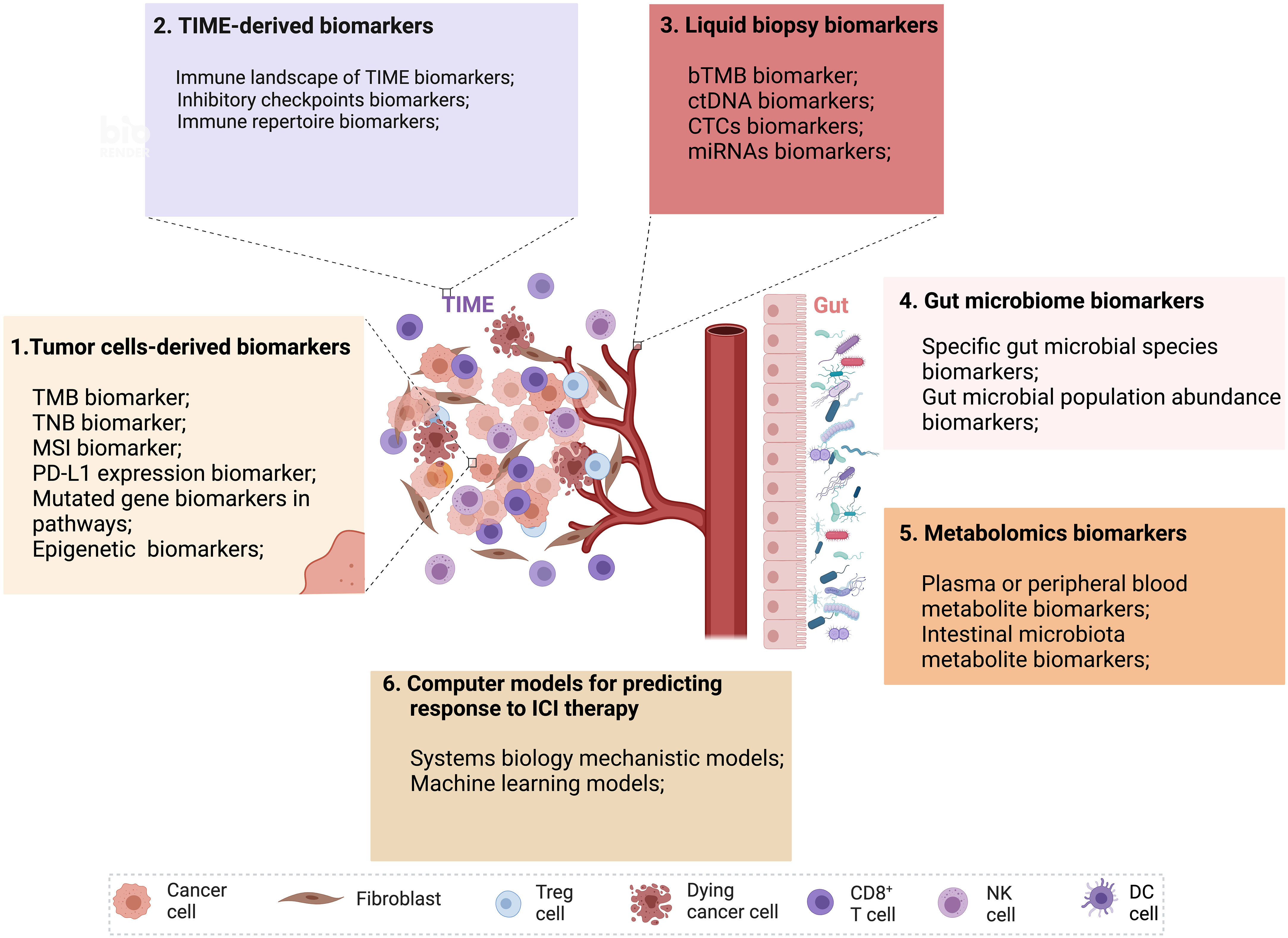
Figure 1 Multidimensional biomarkers and computer models to predict the response to ICI tumor immunotherapy. Multidimensional biomarkers include tumor cells-derived biomarkers and TIME-derived biomarkers. 1. Tumor cells-derived biomarkers include TMB, TNB, MSI, PD-L1 expression, mutated gene biomarkers in pathways, and epigenetic biomarkers. 2. TIME-derived biomarkers include the immune landscape of TIME biomarkers, inhibitory checkpoint biomarkers, and immune repertoire biomarkers. 3. Liquid biopsy biomarkers (bTMB, ctDNA, CTCs, miRNAs). 4. Gut microbiome biomarkers (Specific gut microbial signatures biomarkers, gut microbial population abundance biomarkers). 5. Metabolomics biomarkers (Plasma or peripheral blood metabolite biomarkers, intestinal microbiota metabolite biomarkers). 6. Computer models for predicting response to ICI therapy (Systems biology mechanistic models and machine learning models) (Created with Biorender). bTMB, blood-based tumor mutational burden; CTCs, circulating tumor cells; ctDNA, circulating tumor DNA; miRNAs, microRNAs; MSI, microsatellite instability; TIME, tumor immune microenvironment; TMB, tumor mutational burden; TNB, tumor neoantigen burden.
Furthermore, we comprehensively reviewed computational models for predicting the response to ICI treatment, including mechanistic models based on bottom-up prior knowledge-based systems biology and top-down data-driven ML models and hybrids of both (Figure 1). We also summarized the details of these models, outlining the datasets they adopt, their advantages and disadvantages, and the methods or indicators for evaluation. A summary of validated biomarkers and computer models, along with their predictive performance and clinical utility, has been incorporated to assist readers in quickly identifying the most relevant biomarkers and models for their research or clinical practice.
2 Biomarkers in immune checkpoint inhibitor (ICI) Immunotherapy
2.1 Tumor cells-derived biomarkers
2.1.1 Tumor mutational burden (TMB) biomarker
TMB measures the quantity of somatic gene-coding mutations, mainly consisting of single nucleotide variants (SNVs) and insertions and deletions (INDELs) per DNA megabase (Mb). TMB values positively correlate with the response to ICI therapy in various cancer types, including bladder cancer, non-small cell lung cancer (NSCLC), head and neck cancer and melanoma (9–12). However, a mere small proportion of mutations may translate into neoantigens that activate T cell responses (13). There are also other causes of the lack of efficacy of ICI in TMB high tumors, such as the immunosuppressive environment of the tumor (14), or poor infiltration of immune cells (15). Hence, TMB-high may not accurately predict the effectiveness of ICI therapy in all types of cancer (16), whereas some patients with lower TMB may still benefit from PD-1/PD-L1 blockade therapy (17). These cases show that TMB cannot accurately distinguish tumor patients who respond effectively to ICI therapy.
TMB detection technologies primarily consist of whole genome sequencing (WGS), whole exome sequencing (WES), and gene panels (Figure 2A). Although WGS offers a comprehensive view for TMB detection, its usage is restricted due to high costs, long processing times, and intensive data analysis needs. On the other hand, while WES is typically regarded as the most accurate method for TMB detection, it is often too costly and complex for routine clinical use. As TMB values calculated based on gene panels are generally considered reliable due to their consistency with TMB results measured from WES data, gene panel-based technologies are frequently employed in clinical practice (18). In practical applications, the cutoff value of TMB is influenced by multiple factors such as tumor types, sequencing platforms, number of genes detected, and algorithms, making it difficult to achieve consistency (19). And there is no clear optimal TMB value to maximize efficacy in the pan-tumor population. Nevertheless, the combination of TMB and other practical markers such as PD-L1 expression, microsatellite instability (MSI) status can optimize the stratification of responders and non-responders of patients receiving ICI therapy (20, 21).
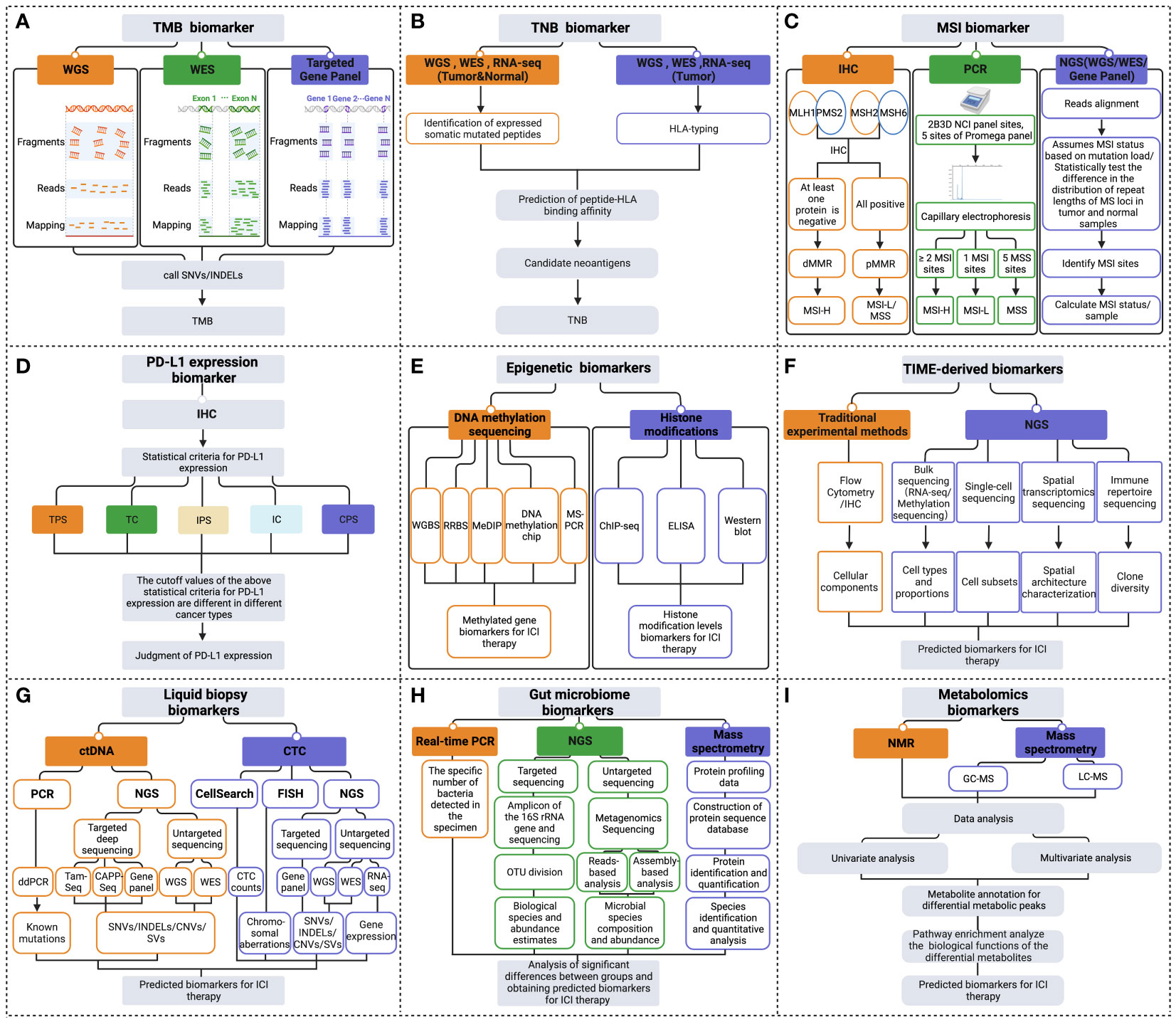
Figure 2 Multidimensional biomarkers detection methods to predict the response to ICI immunotherapy. The detection methods for tumor cells-derived biomarkers include (A) TMB biomarker detection methods; (B) TNB biomarker detection methods; (C) MSI biomarker detection methods; (D) PD-L1 expression biomarker detection methods; (E) Epigenetic biomarkers detection methods. (F) Detection methods of TIME-derived biomarkers. (G) Liquid biopsy biomarkers detection methods. (H) Gut microbiome biomarkers detection methods. (I) Metabolomics biomarkers detection methods (Created with Biorender). ChIP-seq, chromatin immunoprecipitation followed by sequencing; CNVs, copy number variations; CPS, combined positive score; ddPCR, droplet digital PCR; dMMR, deficient MMR; ELISA, enzyme-linked immunosorbent assay; FISH, fluorescence in situ hybridization; GC-MS, gas chromatography- mass spectrometry; HLA, human leukocyte antigen; IC, immune cell; IHC, immunohistochemistry; INDELs, insertions and deletions; IPS, immune proportion score; LC-MS, liquid chromatography- mass spectrometry; MeDIP, methylated DNA immunoprecipitation; MSI-H, MSI-high; MSI-L/MSS, MSI-low/microsatellite-stable; MS-PCR, methylation-specific polymerase chain reaction; NGS, next-generation sequencing; NMR, nuclear magnetic resonance; OUT, operational taxonomic units; PCR, polymerase chain reaction; pMMR, proficient MMR; RNA-seq, RNA-sequencing; RRBS, reduced representation bisulfite sequencing; SNVs, single nucleotide variant; SVs, structural variation; TC, tumor cell; TPS, tumor proportion score; WES, whole exome sequencing; WGBS, whole genome bisulfite sequencing; WGS, whole genome sequencing.
2.1.2 Tumor neoantigen burden (TNB) biomarker
Tumor neoantigens, as the primary target recognized by cytotoxic T cells, play a crucial role in the success of immunotherapy. The number of tumor neoantigens per megabase of the tumor genome is known as TNB (22, 23). In clinical trials of PD-1/PD-L1 antibody therapy, patients with a higher TNB had a more prolonged overall survival (OS) (24, 25). However, resistance to ICI treatment has also been reported in patients with high TNB (26), indicating the need for further optimization of TNB as a biomarker to predict ICI immunotherapy (27).
Many studies that evaluate TNB focus on predicting tumor neoantigens, which are traditionally assessed based on the prediction of binding affinity between the peptide and human leukocyte antigen (HLA) (28) (Figure 2B). However, this method has limited accuracy due to factors such as sequencing data quality, the accuracy of biological information tools, and the complexity of tumor genetics. Therefore TNB inferred from identified neoantigens does not have higher accuracy than TMB in predicting ICI immunotherapy (29). To improve the accuracy of neoantigen prediction, neoantigen heterogeneity should be considered, including truncal and subclonal mutations and changes in neoantigen immunogenicity resulting from immunoediting. Recent studies have developed novel algorithms such as the Cauchy-Schwarz index neoantigen (CSiN) score and the immune-editing-optimized tumor neoantigen load (ioTNL) algorithm to account for neoantigen heterogeneity and predict the response to ICI therapy in various cancers (30, 31). Additionally, multi-omics data, including tumor genomics, transcriptomics, epigenomics, and proteomics, can provide more molecular information for detecting neoantigen load and predicting immunotherapy efficacy (13, 32). Tumor genomics serves as the foundation for predicting neoantigen load (33), yet the integration of multi-omics data can further refine this prediction. For example, transcriptomic analysis can reveal which mutated genes are actually being expressed at the RNA level (34), thus identifying potential neoantigens that can be transcribed and translated into proteins, essential for recognition by the immune system. Epigenomic data, such as DNA methylation analysis, aids in understanding the regulatory mechanisms of gene expression, pinpointing which neoantigens might not be effectively presented due to epigenetic modifications (35). Proteomics, by directly measuring proteins on the tumor cell surface and inside, can confirm which neoantigen peptides are processed and presented by tumor cells to the immune system (36). The combined utilization of these multi-omics data not only enhances the accuracy of neoantigen load detection but also assists in more accurately predicting patients’ responses to immunotherapy.
2.1.3 Microsatellite instability (MSI) biomarker
MSI is a reliable biomarker for predicting the effectiveness of ICI immunotherapy in clinical practice.MSI refers to INDELs mutations in repeating microsatellites units due to a functional defect in the mismatch repair (MMR) system that impairs the repair of DNA replication errors (37). Loss of MMR protein function caused by MMR gene mutations leads to MSI-high (MSI-H) (38). The food and drug administration (FDA) approved anti-PD1 blocking antibody pembrolizumab to be used in the therapy of unresectable or metastatic solid tumors in patients with MSI-H/deficient MMR (dMMR) in, 2017. This was the first time the FDA had approved an antitumor treatment based on biomarkers rather than tumor origin (39). However, MSI-H is relatively rare in most cancer types, except for endometrial and colorectal cancer, with a prevalence ranging from 0% to 16.5% across different cancer types (40). Although the available evidence suggests that MSI-H/dMMR is an effective biomarker to predict the effectiveness of ICI immunotherapy in specific cancer types, more comprehensive data is necessary to confirm the overall value of this biomarker.
Various MSI assays have been developed, such as immunohistochemistry (IHC), multiplex fluorescent polymerase chain reaction (PCR) capillary electrophoresis, and next-generation sequencing (NGS) (41) (Figure 2C). The IHC method primarily detects the expression of MMR proteins in the nucleus, including MutL homologue 1 (MLH1), postmeiotic segregation 2 (PMS2), MutS homologue 2 (MSH2), and MutS homologue 6 (MSH6). If any of these MMR proteins are absent, it is considered dMMR, which is thought to cause high MSI. Conversely, when all four MMR proteins are expressed, it is considered low MSI or microsatellite-stable (MSS), indicating proficient MMR (42, 43). PCR detection of MSI mainly adopts the method of multiplex fluorescent PCR combined with capillary electrophoresis (44). The detection sites used to determine MSI status include the 2B3D National Cancer Institute (NCI) panel sites (including two mononucleotide repeat unit sites and three dinucleotide repeat unit sites) and five mononucleotide repeat unit sites of the Promega panel (45, 46). NGS commonly detects MSI, including WGS, WES, or gene panel (47). NGS detection of MSI includes two main methods. The first method assumes MSI status based on mutation load. The second method measures the status of MSI by statistically examining differences in the distribution of read numbers of a set of microsatellite loci with different repeat lengths in tumor and standard samples (48). Overall, the various assays available for detecting MSI status in tumors offer clinicians a range of options. Therefore, it is essential to evaluate and choose the appropriate method for clinical use carefully.
2.1.4 PD-L1 expression biomarker
PD-L1 expression is the first clinically validated biomarker to predict the curative effectiveness of PD-1/PD-L1 antibodies (49). However, there are certain limitations to its accuracy. One retrospective clinical study revealed that just 28.9% of patients could be predicted for ICI treatment efficacy using PD-L1 expression as a useful biomarker (50). Furthermore, in recent studies, treatment with PD-1/PD-L1 blockade is still effective for some patients with negative PD-L1 expression (51, 52). The efficacy of PD-L1 as a reliable biomarker may be influenced by the heterogeneity of tumors and the tumor microenvironment (TME). The TME is defined as the complex milieu surrounding a tumor, which includes a variety of cell types, blood vessels, immune cells, and extracellular components. This definition also takes into account the dynamic nature of patients’ immune responses, highlighting the intricate interactions that could affect biomarker reliability (53, 54).
PD-L1 expression is mainly detected in clinical settings using IHC methods. PD-L1 expression status can be assessed by measuring various scoring metrics, including tumor proportion score (TPS), tumor cell (TC) score, immune proportion score (IPS), immune cell (IC) score, as well as combined positive score (CPS) (55–57). These scores are methods to quantify the level of PD-L1 expression on tumor and immune cells. The statistical thresholds for PD-L1 expression assessment vary across different cancer types (Figure 2D). Establishing a standardized benchmark for expression assessment is necessary to improve the accuracy of using PD-L1 expression as a predictor in guiding ICI treatment.
2.1.5 Mutated gene biomarkers in pathways
Oncogenic mutations or gene expression changes in cancer cells can activate or inhibit various signaling pathways, such as the interferon-γ (IFN-γ), mitogen-activated protein kinase (MAPK), phosphatidylinositol 3-kinase (PI3K), transforming growth factor-β (TGF-β), and tumor necrosis factor-α (TNF-α) pathways, which can affect PD-L1 expression (Figure 3) and the TME, ultimately impacting ICI therapy efficacy. These pathway-related genetic mutations or gene expression changes may be potential biomarkers for predicting ICI immunotherapy efficacy (58). These biomarkers are presently subject to clinical and preclinical investigations. To date, they have not received approval from the FDA for clinical application (59).
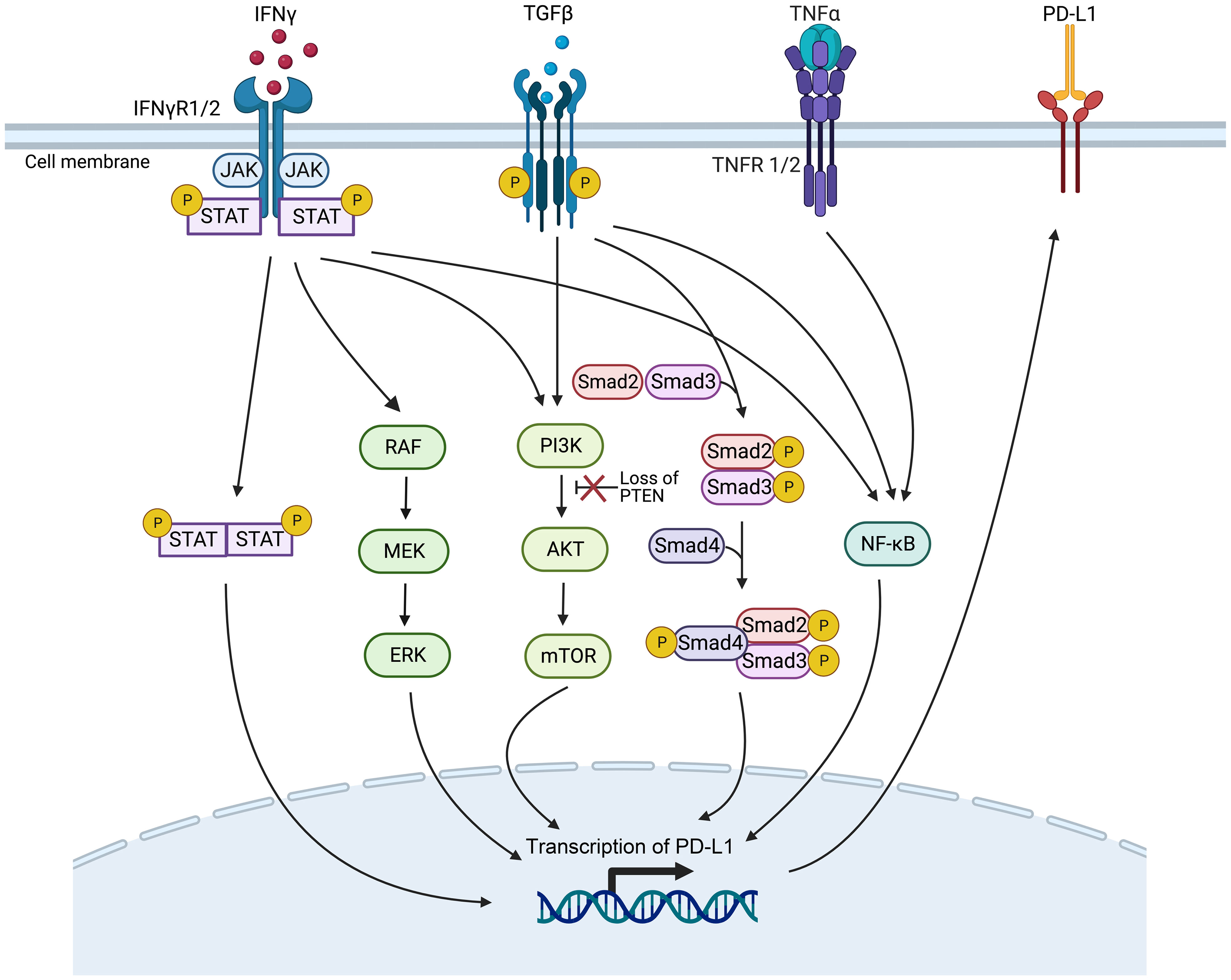
Figure 3 Genetic mutations or gene expression changes in various signaling pathways affect PD-L1 expression. The signaling ways include IFN-γ, MAPK, PI3K, TGF-β, and the TNF-α signaling pathways (Created with Biorender). JAK/STAT, Janus kinase/signal transducers and activators of transcription; mTOR, mammalian target of rapamycin; NF-κB, nuclear factor κB; PTEN, phosphatase with tensin homology.
The IFN-γ pathway is activated when IFN-γ receptor 1/2 (IFNγR1/2) on tumor cells are recognized by IFN-γ, activating the Janus kinase/signal transducers and activators of transcription (JAK/STAT) pathway, then inducing the expression of PD-L1 to inhibit the ICI therapy (60). Gene mutations in proteins related to the IFN-γ pathway, such as IFNγR1/2 and signal transducer and activator of transcription 1 (STAT1), can lead to resistance to ICI therapy, making them potential predictive biomarkers of efficacy (61, 62).
Gene mutations that activate or inhibit the MAPK pathway may also serve as practical biomarkers to predict PD-1/PD-L1 blockade therapy. In lung adenocarcinoma, growth factors and IFN-γ can up-regulate PD-L1 expression levels through MAPK signaling and affect response to immunotherapy (63). In addition, inhibition of the mitogen-activated protein kinase kinase (MEK) and B-Raf proto-oncogene, serine/threonine kinase (BRAF) mutations can enhance the curative efficacy of PD-1/PD-L1 inhibitors (64).
Deletion of phosphatase and tensin homolog (PTEN), a tumor suppressor gene, in tumor cells causes the serine/threonine kinase Akt phosphorylation, enhancing the PI3K/Akt signaling pathway and ultimately up-regulating the expression of PD-L1, leading to T cell inactivation (65). PTEN-deficient metastatic melanoma patients have lower levels of inflammation-related gene expression and tumor-infiltrating lymphocytes, reducing anti-PD-1 therapy response (66).
High expression levels of TGF-β ligand TGFB1 and its receptor TGFBR2, two crucial genes of the TGF-β signaling pathway, are associated with non-response and decreased OS in tumor patients treated with ICI immunotherapy (67). It has been demonstrated that TGF-β can boost the expression of PD-L1 in a Smad2-dependent manner (68). TGF-β can activate the PI3K/Akt/mammalian target of rapamycin (mTOR) and Smad3 pathways to induce epithelial-mesenchymal transition (EMT) (69), which has been associated with the up-regulated expression of PD-L1 (70). Furthermore, TGF-β up-regulates the presentation of the myocardin related transcription factor-A (MRTF-A), promoting the combination of nuclear factor kappa-light-chain-enhancer of activated B cells/p65 subunit (NF-κB/p65) and the PD-L1 promoter, ultimately leading to the activation of PD-L1 expression (71). In addition, TNF-α can activate the NF-κB pathway, leading to the demethylation of the cluster of differentiation 274 (CD274) promoter and the promotion of PD-L1 expression (72). However, high levels of PD-L1 expression after ICI treatment have been associated with poorer treatment outcomes (73).
In the identification of mutated gene biomarkers within signaling pathways, a suite of advanced detection technologies are currently employed, with NGS playing a pivotal role. NGS facilitates whole-genome sequencing, whole-exome sequencing, and targeted gene panel sequencing, not only identifying known genetic mutations but also uncovering novel mutation events, thereby significantly expanding the scope of mutated gene biomarker detection (74). Additionally, quantitative real-time PCR (qPCR) and digital PCR (dPCR), renowned for their exceptional sensitivity and rapid response, have become the methods of choice in both clinical and research settings for detecting specific known mutations. fluorescence in situ hybridization (FISH) and microarray technologies also provide precise capabilities for mutation gene localization and expression level analysis. With the continuous advancements in biotechnology, emerging techniques such as single-cell sequencing and RNA sequencing are being employed in mutation detection, offering more accurate tools for cellular-level variant analysis (75–77). The integrated application of these advanced technologies, coupled with robust bioinformatics analysis, now enables us to more comprehensively and deeply unravel mutated genes within signaling pathways, thereby enhancing the precision in detecting predictive biomarkers for cancer ICI immunotherapy efficacy.
2.1.6 Epigenetic biomarkers
Epigenetic variation in tumor cells has been linked to tumor progression and changes in immune response, suggesting that it can serve as a valuable biomarker for predicting the effectiveness of ICI therapy (78). This variation includes DNA methylation, modifications of histones, and other chromatin remodeling activities (79). Guerreiro et al. investigated that the combination of DNA repair gene RAD51B promoter methylation RAD51Bme+ with PD-L1+ was more effective in predicting the response to ICI therapy than either marker alone in NSCLC (80). Darvin et al. found that histone acetylase overexpression is involved in the expression of PD-L1 induced by EMT, contributing to immune evasion (81). Loss of function in polybromo 1 (PBRM1), a subunit of the switch/sucrose‐non‐fermentable (SWI/SNF) chromatin remodeling complex, is related to improved clinical outcomes in patients receiving PD-1 antibody therapy (82).
Recent advancements in DNA methylation sequencing technology have enabled the identification of differentially methylated genes or methylation signatures as predictive biomarkers of the efficacy of ICI treatment (83). This technology includes whole genome bisulfite sequencing (WGBS), reduced representation bisulfite sequencing (RRBS), methylated DNA immunoprecipitation (MeDIP), DNA methylation chips, and methylation-specific polymerase chain reaction (MS-PCR), among others (Figure 2E). MS-PCR is commonly used in clinical testing to analyze site-specific methylation of individual CpG islands (84). Chromatin immunoprecipitation followed by sequencing (ChIP-seq) is usually used to investigate histone-specific modifications at specific locations throughout the genome (85). Additionally, the overall levels of histone modifications can be assessed through enzyme-linked immunosorbent assay (ELISA) or western blot techniques (86) (Figure 2E).
2.2 Tumor immune microenvironment (TIME)-derived biomarkers
2.2.1 Immune landscape of TIME biomarkers
Understanding the immune landscape of the TIME is critical in predicting the efficacy of immunotherapy, as an immunosuppressive TIME can hinder its effectiveness. Cell type, abundance, location, and function within the TIME may impact tumor immunotherapy efficacy (58). A range of methods is available to quantify the different cellular components of tumor tissue. Traditional experimental techniques, such as flow cytometry and IHC, may have limited utility due to low tissue availability or high costs (87). NGS data for analyzing immune cells primarily come from bulk sequencing, such as transcriptome and methylation data, single-cell sequencing data, and spatial transcriptome sequencing data (Figure 2F). The transcriptome and methylation data analysis can be divided into three methods: a non-reference strategy and two reference-based methods - deconvolution and scoring (88). More recently, single-cell sequencing has enabled the characterization of immune cell status within the TIME at a single-cell level, allowing for identifying novel tumor-associated immune cell subsets at a finer resolution (89). The development of spatial transcriptomics sequencing has allowed the study of spatial distribution characteristics within the TIME, thereby obtaining spatial biomarkers to predict the effect of immunotherapy (90). In the tumor microenvironment (TME), fibrosis, marked by an excessive buildup of the extracellular matrix, particularly collagen, leads to hardened and thickened tissue structures. This condition can hinder immune cell infiltration and functionality, thereby impacting the efficacy of ICI therapy. As such, fibrosis within the TME is identified as a significant biomarker, aiding in the prediction of ICI therapeutic outcomes. The influence of fibrosis on both the TME structure and immune cell functions within the TIME highlights its critical role in modulating tumor immune responses, underscoring the importance of further investigation into fibrosis for enhancing immunotherapy strategies (91, 92).
2.2.2 Inhibitory checkpoints biomarkers
In addition to CTLA-4 and PD-1/PD-L1, the TIME contains other inhibitory checkpoints such as T-cell immunoglobulin and mucin-domain-containing molecule 3 (TIM-3) plus TIM-3 ligands, lymphocyte-activation gene 3 (LAG-3). TIM-3 up-regulated by T cells has also been proposed as a candidate biomarker to track the progress of ICI therapy. During treatment with PD-1/PD-L1 inhibitors, TIM-3 upregulation may suppress T helper cell type 1 (Th1) cell responses and decrease the expression of cytokines like TNF-α and IFN-γ, leading to drug resistance in cancer patients (61, 93). In addition, LAG-3 has been shown to up-regulate Treg activity and inhibit Teff activity, which can create a TIME that suppresses immune responses (94). Therefore, monitoring the expression of LAG-3 is crucial for predicting ICI therapy response, but the expression of LAG-3 is not routinely used in the clinic (59).
2.2.3 Immune repertoire biomarkers
The immune repertoire consists of all the T cell receptors (TCRs) and B cell receptors (BCRs) in an individual. These receptors bind to self-antigens or foreign antigens, such as external viruses and bacteria, triggering an immune response. Therefore, TCRs and BCRs are crucial in immunotherapy (95, 96). For example, patients receiving anti-CTLA-4 therapy demonstrate longer survival when exhibiting low baseline TCR clonality and the presence of clones with large expansion after treatment. In contrast, these factors do not exist in patients receiving anti-PD-1 therapy (97). The finding suggests that TCR clones may be biomarkers for predicting the response to ICI therapy.
The clonal diversity of the immune repertoire is assessed by analyzing the sequence characteristics of the V(D)J recombination fragments of the complementarity-determining region 3 (CDR3) region from the receptor gene, which can be used as a biomarker to predict the therapeutic effect of ICI (Figure 2F). Early tools for sequencing analysis of immune repertoires include IgBLAST (98), iHMMune-align (99), and IMGT/V-QUEST (100). MiXCR can correct PCR errors and precisely analyze sequencing data for BCR and TCR (101). IGoR can accurately identify V(D)J rearrangements (102). TRUST4 finds many CDR3s with high precision and sensitivity (103). Integrating artificial intelligence algorithms with BCR and TCR sequencing data may lead to more accurate identification of patient subgroups likely to respond positively to ICI therapy.
2.3 Liquid biopsy biomarkers
In the past decade, the development of non-invasive techniques for tumor diagnosis, such as liquid biopsy, has dramatically accelerated the pace of tumor research (104). Potential sources for the development of fluid biopsy biomarkers for tumor ICI therapy include blood-based TMB (bTMB), circulating tumor DNA (ctDNA), circulating tumor cells (CTCs), and microRNAs (miRNAs), among others (105). As a non-invasive and replicable biomarker for forecasting ICI treatment efficacy, bTMB offers tremendous advantages in predicting clinical outcomes for cancer patients (106). Identifying alterations in ctDNA levels can predict a patient’s response to immunotherapy sooner than traditional imaging evaluations (107). In addition, positive PD-L1 expression status in CTCs can expect a response to immunotherapy (108). Incorvaia et al. reported that directly identified miRNA expression profiles of patients before and after treatment could be used to assess the dynamic molecular changes underlying PD-1 inhibitor nivolumab treatment and predict the response to treatment (109).
Detection of ctDNA in liquid biopsy includes quantitative PCR and NGS (110) (Figure 2G). Droplet digital PCR (ddPCR) is an advanced form of quantitative PCR and provides exceptional sensitivity and precision for nucleic acid quantification (111). NGS detects ctDNA mutations using non-targeted sequencing and targeted deep sequencing methods. Non-targeted sequencing encompasses WGS and WES. Leary et al. used the PARE method to detect ctDNA genomic rearrangement breakpoints in plasma samples via whole genome analysis (112). Targeted deep sequencing, such as tagged-amplicon deep sequencing (Tam-Seq), cancer personalized profiling by deep sequencing (CAPP-Seq), and gene panel methods, captures specific genomic regions enriched in ctDNA by PCR or hybridization (113). NGS can identify ctDNA mutations, such as SNVs, INDELs, copy number variations (CNVs), structural variations (SVs), etc. Nevertheless, the sensitivity and specificity of ctDNA detection by NGS depend on sequencing depth, bioinformatic analysis pipelines, and parameters (113, 114). CTC enrichment and detection can be achieved through biophysical and antibodies-based methods. Antibody-based methods detect cell surface markers, such as epithelial cell adhesion molecule (EpCAM) on CTCs. For example, the CellSearch® system, an FDA-approved automated CTC counting system, uses EpCAM-based detection (115). FISH can also detect CTCs, where chromosomal aberrations can be observed (116). NGS detects CTCs by non-targeted sequencing (WGS, WES, RNA-sequencing (RNA-seq)) and targeted sequencing gene panel methods. WGS, WES, and gene panels can detect CTC mutations, including SNVs, INDELs, CNVs, SVs, etc. (117–120), and RNA-seq allows for gene expression detection (121). Sequencing techniques enable the identification of differentially mutated or expressed genes in ICI treatment-responsive and non-responsive patients, making liquid biopsies a valuable source of biomarkers for ICI therapy (Figure 2G).
2.4 Gut microbiome biomarkers
Regulation of the intestinal flora on human immunity directly affects the effect of tumor immunotherapy (122–124). The impact of PD-1/PD-L1 blocking treatment is compromised in antibiotic-experienced cancer patients, and survival is significantly reduced (125–127). Melanoma patients exhibited distinct microbial signatures in their intestines, with Lachnospiraceae spp. being associated with a favorable clinical response, while Streptococcaceae spp. was associated with an unfavorable clinical response (128). Zheng et al. found that patients with good responses to treatment had a higher population richness of the gut microbiota and gene counts than cancer patients with poor answers. The compositional richness of the intestinal microbiota is closely related to the effect of immunotherapy (129). Therefore, the gut microbiota can affect and predict the clinical impact of immunotherapy on cancer.
Various molecular detection methods are available for analyzing intestinal microbes, including quantitative real-time PCR, targeted NGS such as 16S ribosomal RNA (rRNA) gene amplicon analysis, non-targeted NGS sequencing, and mass spectrometry (MS) (130) (Figure 2H). Quantitative fluorescence PCR in real-time is a simple and effective method for detecting the specific number of bacteria in the sample, with strong specificity (131). The data analysis of 16S amplicon sequencing includes operational taxonomic units (OTUs) classification and estimation of biological species and abundance, enabling significant difference analysis between the response and non-response groups to obtain biomarkers for efficacy prediction of ICI treatment (132, 133). Metagenomics extracts the total DNA of environmental microorganisms for non-targeted sequencing (134). Two analysis methods are commonly used for metagenomic sequencing to obtain the composition and abundance of microbial species. The first method directly compares the reads to reference genomes or feature gene sets. The second method involves getting high-quality bacterial genomes based on assembly and then using genome sequence annotation and alignment to obtain species composition and abundance. Combining these two methods usually yields more accurate results (135). MS technology is used for metaproteomics detection. It can accurately and efficiently identify the distinct protein components in human gut microbiota, enabling species identification and quantitative analysis (136, 137). Predictive biomarkers of ICI therapy can be identified by analyzing the significant differences in gut microbiome species or abundance between patients who respond to ICI treatment and those who do not.
2.5 Metabolomics biomarkers
Metabolites are crucial in exploring new predictive biomarkers for ICI therapy in cancer patients (138). In one investigation, the analysis of metabolites in plasma from NSCLC patients before and after nivolumab treatment using liquid chromatography-MS (LC-MS) or gas chromatography-MS (GC-MS) showed that a combination of four metabolites could effectively predict the therapeutic effect of the PD-1 inhibitor (139). Higher cholesterol levels in the bloodstream have been linked to longer OS and progression-free survival (PFS) in cancer patients treated with ICI (140). Short-chain fatty acids (SCFAs), metabolites from the host intestinal microbiota, are critical in regulating immune cell response. SCFAs-focused microbial metabolites may be a new biomarker to predict the immunotherapy response (141).
Nuclear magnetic resonance (NMR) spectroscopy and MS are widely used techniques for directly detecting metabolites in metabolomics research (142, 143). MS is divided into LC-MS and GC-MS (144) (Figure 2I). The spectrum produced by NMR is linearly related to the compound concentration. However, due to its low sensitivity, NMR is often used to identify and analyze simple or purified samples. On the other hand, combining MS and chromatographic separation offers high sensitivity and specificity. The MS raw data analysis tools include XCMS (145), MZmine (146), and IP4M (147). Statistical analysis, including univariate and multivariate analysis, is primarily used to identify differential metabolite peaks between responders and non-responders to ICI therapy (148). Then, the annotation of metabolites is performed on the differential metabolic peaks, and the biological functions of the differential metabolites are analyzed through pathway enrichment, which is used to predict the therapeutic effect of ICI.
2.6 Challenges, limitations, and future perspectives
It’s essential to acknowledge the challenges and limitations of this field, such as the impact of tumor heterogeneity, the lack of standardization in biomarker detection, and the complexities of translating research into clinical practice. Further research into these areas will likely yield more nuanced insights and refinements in biomarker discovery and utilization. At the same time, we are confronted with the dual challenge of integrating diverse omics data without substantially increasing costs and identifying the minimal biomarker combination that can accurately predict responses to immunotherapy.
Tumor heterogeneity serves as a principal source of variability in treatment responses, a phenomenon not only observed between distinct tumors but also within individual tumors. This directly impacts the expression and detection of biomarkers, thereby influencing the prediction of treatment outcomes. Future research should delve deeper into the essence of tumor heterogeneity and explore strategies for overcoming this challenge through precise biomarker combinations.
Moreover, the lack of standardization in biomarker detection warrants attention. Variabilities in detection methodologies and standards across different laboratories compromise the comparability of results, adding layers of complexity to the translation of research findings into clinical practice. Thus, establishing unified detection standards and methodologies is imperative for enhancing the accuracy and reliability of biomarker detection.
The complexity of translating research outcomes into clinical applications is equally critical. Despite the potential of research to provide valuable biomarker insights, applying these discoveries in a clinical setting necessitates overcoming several hurdles, including cost, feasibility of technology transfer, and applicability across diverse patient populations.
Looking forward, the integration of various omics data, such as genomics, transcriptomics, and metabolomics, promises to offer a more comprehensive view of the tumor and its microenvironment. Although such integrative research requires sophisticated modeling techniques to process and analyze extensive datasets, it also poses significant cost challenges. Therefore, balancing the enhancement of predictive accuracy with cost control emerges as a pressing issue that demands resolution.
Identifying the minimal combination of biomarkers for effective prediction of immunotherapy responses is both complex and crucial. This task involves not only assessing the dependency of biomarkers on the tumor’s histological type but also considering the use of advanced technologies like machine learning and artificial intelligence to discern the most promising biomarker combinations from data. Strategies to address tumor heterogeneity might include the development of new biomarkers that reflect various aspects of the tumor, such as gene expression, metabolic state, and immune environment. Additionally, customizing treatment plans based on specific tumor characteristics of patients could improve therapeutic effectiveness and precision.
Overall, future research requires not only technological and methodological innovation but also conceptual breakthroughs to tackle the current challenges and limitations. By integrating and analyzing multiple types of omics data, developing novel biomarkers, and formulating precise treatment strategies, we can advance toward more accurately predicting responses to immunotherapy. Interdisciplinary collaboration, involving the concerted efforts of biologists, data scientists, clinicians, and patients, will be key to achieving this goal. Through such collaboration, we anticipate overcoming existing challenges and providing patients with more effective and personalized treatment options.
3 Computer models to predict the response to ICI therapy
While the administration of ICI antibodies via intravenous infusion is a straightforward process, the real complexity in tumor immunotherapy lies in understanding the biological mechanisms of the treatment and accurately predicting patient responses. This challenge is exacerbated by the intricate interplay of immunosuppressive and immunostimulatory components within the tumor microenvironment, which significantly complicates the prediction of therapeutic outcomes (149, 150). A system-level framework is necessary to comprehend the dynamic temporal and spatial relationships between cellular and molecular types. Mechanistic modeling in systems biology can help achieve this by modeling multivariate biomarkers with dynamic characteristics and extracting essential parameters based on prior knowledge, allowing for systematic evaluation of immunotherapy predictive biomarkers (151). Various mechanistic models, including pharmacokinetic/pharmacodynamic (PK/PD) models, partial differential equations (PDE) models, signal network-based models, quantitative systems pharmacology (QSP) models, and agent-based models (ABMs), can be used for predicting the efficacy of ICI therapy. Supplementary Table S2 describes the detailed information of these mechanistic models, including the advantages and disadvantages of the models, the datasets used, evaluation methods/metrics, biomarkers, etc. Data-driven ML models capture rules from complex cancer changes, resulting in good prediction results even without a complete understanding of the underlying biological mechanisms. Furthermore, the features and parameters of ML models contain a wealth of latent information that can be utilized to explore new biomarkers (152, 153) (Figure 4). Additionally, Hybrid models, by combining prior knowledge with multimodal data using computer models, help to discover more accurate biomarkers of systematic dynamics.
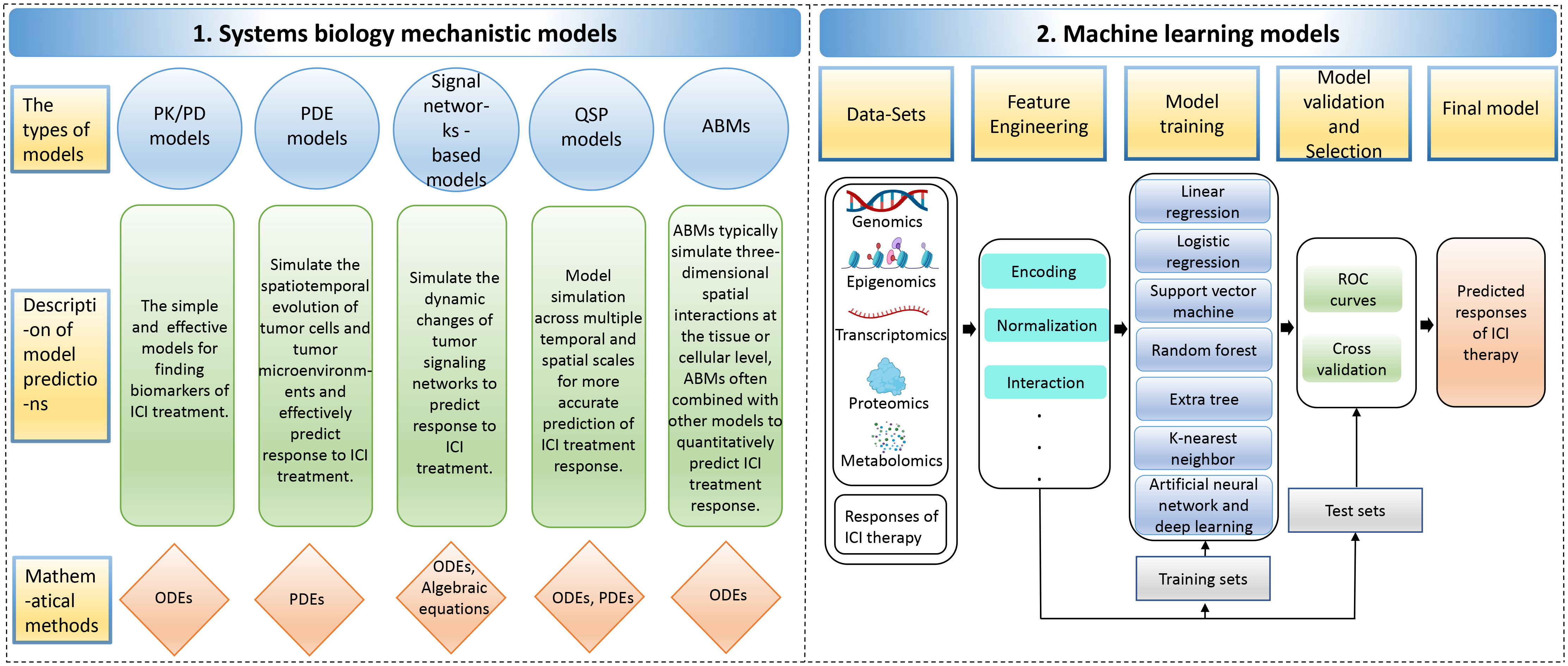
Figure 4 The computer models for predicting responses to immune checkpoint inhibitor therapy. The computer models include 1. Systems biology mechanistic models; 2. Machine learning models. ABMs, agent-based models; ODEs, ordinary differential equations; PDEs, partial differential equations; PK/PD, pharmacokinetic/pharmacodynamic; QSP, quantitative systems pharmacology; ROC, receiver operating characteristic.
3.1 Knowledge-based mechanistic models
3.1.1 Pharmacokinetic/pharmacodynamic (PK/PD) Models
PK/PD models are typically created using ordinary differential equations (ODEs), which involve abstracting data into dynamic systems (154). PK/PD models can be used to identify biomarkers for predicting the curative effects of anti-PD-1/PD-L1 (7). For instance, Netterberg et al. developed a PK/PD quantitative model to explore circulating biomarkers capable of predicting early tumor response to PD-L1 inhibitor atezolizumab in NSCLC (155). In addition, Liu et al. developed population pharmacokinetic (PPK) models to describe the PK characteristics of the PD-1 antibody nivolumab. It has been reported that the change in clearance rate of nivolumab is associated with disease state after treatment. Specifically, patients with better disease response and survival showed greater reductions in baseline clearance, leading to higher exposure to drugs during stable conditions (156).
3.1.2 Partial differential equations (PDE) models
In addition to using ODEs, partial differential equations (PDEs) can also be applied to simulate spatiotemporal tumor growth and predict the effectiveness of ICI therapy (157). For example, Siewe et al. utilized the PDE system to create a mathematical model of cancer cells, immune cells, and cytokines to explain the primary drug resistance of PD-1 antibody therapy. This model also identified two cancer parameters that could potentially be biomarkers to predict the effectiveness of combination therapy using both anti-PD-1 and anti-TGF-β treatments (158).
3.1.3 Signal networks-based models
The dynamics of tumor signaling networks in cancer patients treated with ICIs can be modeled using ODEs, which predict their response to immunotherapy. For instance, Brogden et al. used ODEs to model protein-protein interactions at each step in the signaling pathway and modeled the reaction at each specific node as the Michaelis-Menten equation. They constructed a patient-specific predictive calculation model based on annotating a patient-specific spectrum of genetically deleterious mutations in cancer networks. This model was utilized to predict the expression of various chemotactic and immunosuppressive factors, which can help predict the effectiveness of PD-1 blockade therapy in tumor patients (159).
3.1.4 Quantitative systems pharmacology (QSP) models
Compared to clinical trials, QSP models have advantages in exploring biomarkers that can predict the response of PD-1/PD-L1 blocked therapy by spanning multiple temporal and spatial scales (160). Zhang et al. developed a personalized prediction model for the curative response to ICI in triple-negative breast cancer by integrating single-cell tumor data and a spatial QSP model. Their model, consisting of a four-compartment QSP model representing the entire patient and an ABM that simulated spatiotemporal cellular and molecular interactions within the tumor compartment’s three-dimensional space, accounted for antigen burden and TME heterogeneity (161).
3.1.5 Agent-based models (ABMs)
ABMs are commonly used to simulate three-dimensional spatial interactions at the tissue or cell level and have been widely used to evaluate cancer drug resistance and efficacy (162). ABM combines other models to build multiscale agent models to simulate ICI treatment and achieve quantitative prediction of combinations of therapeutic biomarkers (163). Storey et al. constructed a multiscale model that combined ABM and PDE models to simulate the response of glioblastoma to combined treatment with PD-1 inhibitor therapy and oncolytic virus therapy. According to the model simulations, the level of tumor antigenicity, which is determined by the tumor-mediated proliferation rate of T cells, has a more significant impact on therapeutic outcomes than the killing efficiency of T cells (164). These studies emphasize the significance and practical value of using ABMs in conjunction with other models for deeply understanding and predicting cancer treatment responses, particularly in the context of complex dynamics at the tissue and cellular levels.
3.2 Machine learning (ML) models
ML approaches can extract multi-dimensional latent features from biological multi-omics sequencing data, including genomic, epigenomic, transcriptomic, proteomic, and metabolomic data, to explore predictive markers of efficacy in ICI therapy (165, 166). These ML approaches generally entail data collection and selection, feature engineering, model building, validation, optimization, and evaluation. Various ML algorithms have been employed in predicting the effectiveness of cancer immunotherapy, including linear regression, logistic regression, support vector machine (SVM), random forest, extra tree, k-nearest neighbor (KNN), artificial neural network (ANN) and deep learning, among others (167). In addition, hybrid models of systems biology and ML are explained (Supplementary Table S3).
3.2.1 Linear regression models
A linear regression model can capture the potential linear relationship between ICI treatment response levels and tumor patients’ cell molecular profiles (168). Common linear regression models include the ridge regression model, the least absolute shrinkage and selection operator (lasso) regression model, and the elastic net regression model. Elastic net regression can learn a sparse model similar to lasso regression but also has the stability of ridge regression (169). Xue et al. initially identified CpG probes linked to the objective response rate (ORR) of PD-1/PD-L1 blockade therapy, and then developed a lasso regression model using these probes for predicting the treatment’s efficacy (170). Sun et al. created an elastic net regularized regression method to obtain a radiomic signature-based non-invasive predictor of tumor-infiltrating CD8 cells to predict the curative response of PD-1/PD-L1 antibodies (171).
3.2.2 Logistic regression models
Compared to the linear regression model, the logistic regression model introduces nonlinear factors by introducing the signature mapping function. It estimates model parameters using the maximum likelihood method and gradient descent for classification tasks (172–174). For instance, Park et al. developed a multivariate logistic regression model to predict ICI treatment response in metastatic urothelial carcinoma, with high-risk groups predicted by the model associated with worse survival outcomes (175). Refae et al. utilized elastic net penalty logistic regression to predict the therapeutic effect of anti-PD-1/anti-PD-L1 based on single nucleotide polymorphisms (SNPs) data related to immunogenetics in advanced cancer patients. They identified gene SNPs related to TME associated with ICI treatment efficacy (176). Zhang et al. used lasso logistic regression to select dynamic serum markers for metastatic or recurrent nasopharyngeal carcinoma and developed a risk score prediction model for PD-1 inhibitor therapy. The model’s predicted probability of efficacy was consistent with the observed probability of prediction (177).
3.2.3 Support vector machine (SVM)/random forest/extra tree/k-nearest neighbor (KNN) models
Other ML models, such as SVM, random forest, extra tree, and KNN, have been applied to predict response to ICI treatment (178, 179). SVM finds the maximum margin hyperplanes among different data classes, while random forest algorithms perform classification or regression tasks by constructing many decision trees (180). One advantage of random forest is the ability to evaluate the importance of features while training a highly accurate model, and the learning process is fast (181, 182). The extra tree uses a series of decision trees to make final predictions about the class or category to which the data point belongs, but it uses the entire original sample rather than sub-sampling and replacing the data like a random forest (183). KNN is a simple and effective algorithm that finds the K nearest neighbors of a test sample in the feature space and classifies the sample based on the majority class of its neighbors. However, KNN requires high computational time costs and memory requirements, making it less suitable for large datasets (184).
Multiple ML models are often constructed to predict cancer ICI therapy response, and the best-performing model is then chosen to identify potential biomarkers. For example, Shang et al. developed a prediction model for immunotherapy response based on DNA methylation immune scores using lasso regression, SVM, and random forest models, with the lasso regression model performing best (178). Peng et al. developed several classification models based on the gut microbiome dataset from gastrointestinal cancer patients, including elastic net, SVM, random forest, extra tree, and KNN. The accuracy of all models except the SVM model was above 0.9, suggesting that the microbiome has excellent potential as a biomarker to predict the curative effect of PD-1/PD-L1 blockade (185). ML-based approaches have demonstrated outstanding potential in guiding clinical treatment.
3.2.4 Artificial neural network (ANN) and deep learning models
ANN imitates the behavioral characteristics of the biological neural network and uses artificial neurons to form a nonlinear data modeling system. It’s an adaptive information processing system with a learning capacity that can change its internal structure in response to external inputs (186). Multilayer perceptron (MLP) is a forward-structured ANN that utilizes multiple layers of artificial neurons to learn complex nonlinear relationships between input and output data (187, 188). MLP is well-suited for predicting ICI therapy response as it can effectively capture the complex relationship between multiple features and treatment response. Yang et al. constructed an MLP deep learning model that distinguishes treatment responders from non-responders by combining multi-omics data from advanced NSCLC patients treated with PD-1/PD-L1 inhibitors, showing an excellent differentiated performance (165).
In addition to MLP, deep neural networks (DNNs) with two or more hidden layers have better feature representation and complex mapping modeling capabilities than shallow neural networks (189). Peng et al. developed a DNN model that predicts the response to ICI therapy in lung adenocarcinoma patients using somatic mutations. This approach is feasible because there is a strong association between two classifications - durable clinical benefit (DCB) and no durable benefit (NDB) - and various factors such as TMB, neoantigen counts, PD-L1 expression, and the level of immune cell infiltration (190). Arbour et al. constructed a deep natural language processing model to estimate response evaluation criteria in solid tumors that have blocking treatment with PD-1/PD-L1. The model accurately predicted the onset and date of cancer progression and the best overall response (191).
The convolutional neural network (CNN), another type of DNN, is a feedforward neural network that uses convolution calculations and is widely used in computer vision (192, 193). CNN can achieve good data fitting by extracting features through convolutional layers, sharing information in convolutional kernels, and effectively reducing parameters through pooling layers (194). Hu et al. developed a CNN model to predict the impact of PD-1 inhibitor therapy in cancer patients based on hematoxylin and eosin (H&E)-stained images, yielding promising results (195). These findings suggest that deep learning-based models could be integrated into routine clinical practice to screen patients who may benefit from ICI treatment.
3.2.5 Hybrid models of systems biology and machine learning (ML)
The novel integrative computational approach that combines systems biology and ML offers a powerful tool for predicting the therapeutic effects of ICI at the individual level. Przedborski et al. developed a systems biology-informed neural network model that combines both approaches. The model generates simulated clinical data from a systems biology model and extracts patient features to distinguish treatment responders from non-responders using a classification neural network model. The transfer learning strategy is then applied to enhance the model’s predictive ability using actual clinical data (196). The advantage of hybrid models is that they bridge the gap between the two disciplines. Systems biology can generate infinitely simulated data to scale up small clinical datasets, while ML can process multi-omics high-throughput data. Moreover, systems biology models can simultaneously provide mechanistic explanations from a biological perspective that cannot be explained by ML (197).
4 Conclusion
Although ICIs have significantly improved cancer treatment, their clinical efficacy remains limited. This underscores the importance of identifying biomarkers to predict patients’ responses to ICI therapy. This review summarizes the advances in biomarker research for ICI treatment, providing a comprehensive classification and overview of these markers. These include tumor cells-derived biomarkers such as TMB, TNB, MSI, and PD-L1 expression, mutated gene biomarkers in pathways, and epigenetic biomarkers. Additionally, the scope encompasses TIME-derived biomarkers, including the immune landscape of TIME biomarkers, inhibitory checkpoints biomarkers, and immune repertoire biomarkers. We also discuss liquid biopsy biomarkers, gut microbiome biomarkers, and metabolomics biomarkers. Currently, clinically used biomarkers include TMB, MSI, and PD-L1 expression. However, these biomarkers often do not effectively predict ICI therapeutic responses in the majority of tumor patients, leading researchers to move beyond the use of a single biomarker and expand the spectrum of explored biomarkers. The article not only elaborates on various biomarkers used to predict tumor ICI treatment response or tolerance and their respective pros and cons but also introduces various laboratory and clinical methods for their detection. Advances in multi-omics sequencing, single-cell, and spatial biology techniques offer a holistic understanding of coordinated anti-tumor systemic immunity. Owing to these advancements, more multi-dimensional ICI response biomarkers have been identified and validated (198–200). Multi-omics sequencing offers a comprehensive insight, allowing a multifaceted evaluation and understanding of the interplay between tumors and the immune system. Single-cell technologies enable the resolution of different types of biomarkers at the individual cell level. Spatial biology techniques reveal cellular spatial distribution within tissue structures, aiding in understanding how biomarkers function within the tumor microenvironment. However, these techniques come with high costs, stringent sample preparation requirements, and complex data analysis. Translating multi-dimensional ICI response biomarker data into clinically meaningful interpretations and applications remains challenging.
Computational prediction models are transforming the way we use clinical biomarkers, a change that is evident not only in current applications but also in ongoing research. These models, by integrating multimodal data related to the immune system and historical knowledge, have established mechanistic models within systems biology aimed at revealing the mechanisms of immunotherapy and identifying key factors for its efficacy (201, 202). Their main advantage lies in the ability to integrate data from different disciplines and previous knowledge, aiding in the explanation and understanding of complex biological system mechanisms. This interdisciplinary integration offers the possibility to discover and validate new biomarkers, as well as to optimize the application of existing ones, thereby advancing personalized medicine. However, challenges such as the complexity of these computational models, the significant demand for computational resources, and the difficulty in accurately estimating model parameters still persist. ML methods have become crucial in the research and clinical application of immunotherapy due to their exceptional data-fitting capabilities and feature engineering. Yet, their predictive logic often lacks transparency, limiting their wider adoption. To address this, there is a push for developing interpretable ML models, which would enhance the explainability and credibility of model predictions and serve as an important research direction. To delve deeper into the dynamic nature of cancer and immune responses, it is necessary to combine mechanistic models in systems biology with advanced computational tools like neural networks and deep learning. The fusion of these approaches can reveal the intrinsic mechanisms of cancer immunotherapy, extracting valuable information and patterns from vast datasets to provide a more comprehensive perspective. This not only aids in making smarter and more adaptive predictions of tumor ICI treatment outcomes, ensuring greater precision, but also provides cancer patients with more accurate and comprehensive dynamic biomarkers, fostering more personalized immunotherapy (160).
Beyond the challenges of model complexity, computational demands, and ML transparency, effectively harnessing vast datasets from diverse sources and ensuring their security pose significant challenges. Addressing the challenge necessitates the establishment of a robust data management platform. Such a platform must ensure the standardization of datasets to facilitate interoperability and maintain stringent privacy safeguards to protect patient information. This infrastructure would serve as a pivotal nexus between the theoretical models developed through research and their practical deployment in clinical settings. By providing a standardized and secure environment for data handling, the platform would not only streamline the adoption of computational models in clinical workflows but also promote the reproducibility of research findings across different institutions.
To successfully establish a data management platform, it’s crucial to coordinate the expertise of professionals from diverse disciplines. Specialists in biology, computing, medicine, and data analysis should collaborate not only to collect and annotate data but also to process and do data quality control, enhancing the utility of the data. Such teamwork is essential for building predictive models for ICI therapies that are meaningful in a real-world medical context. In refining these tools and models, transparency and interpretability are key. The objective is to make both the data and the models understandable, which will increase the credibility of in silico analyses and support their clinical translation. This clarity is essential to ensure that the insights derived from computational research can be confidently applied to inform treatment decisions, thereby bridging the gap between research and clinical application.
Through these measures, we can fully harness the potential of biomarkers and computational models to provide more accurate and personalized treatment options for cancer patients, ultimately achieving the goal of precision medicine. With continuous technological advancements and strengthened interdisciplinary collaboration, the future holds greater breakthroughs in the field of cancer treatment.
Author contributions
QY: Conceptualization, Investigation, Methodology, Project administration, Writing – original draft, Writing – review & editing. HM: Writing – review & editing. LX: Resources, Writing – review & editing. LS: Funding acquisition, Resources, Supervision, Validation, Writing – review & editing.
Funding
The author(s) declare that financial support was received for the research, authorship, and/or publication of this article. This research was funded by The National Natural Science Foundation of China (Grant No. 62072433) and The Science Technology and Innovation Committee of Shenzhen Municipality (Project No. JCYJ20200109143216036).
Conflict of interest
The authors declare that the research was conducted in the absence of any commercial or financial relationships that could be construed as a potential conflict of interest.
Publisher’s note
All claims expressed in this article are solely those of the authors and do not necessarily represent those of their affiliated organizations, or those of the publisher, the editors and the reviewers. Any product that may be evaluated in this article, or claim that may be made by its manufacturer, is not guaranteed or endorsed by the publisher.
Supplementary material
The Supplementary Material for this article can be found online at: https://www.frontiersin.org/articles/10.3389/fimmu.2024.1368749/full#supplementary-material
References
1. Mishra AK, Ali A, Dutta S, Banday S, Malonia SK. Emerging trends in immunotherapy for cancer. Diseases. (2022) 10:60. doi: 10.3390/diseases10030060
2. Schuster SJ, Bishop MR, Tam CS, Waller EK, Borchmann P, McGuirk JP, et al. Tisagenlecleucel in adult relapsed or refractory diffuse large B-cell lymphoma. N Engl J Med. (2019) 380:45–56. doi: 10.1056/NEJMoa1804980
3. Garon EB, Rizvi NA, Hui R, Leighl N, Balmanoukian AS, Eder JP, et al. Pembrolizumab for the treatment of non–small-cell lung cancer. N Engl J Med. (2015) 372:2018–28. doi: 10.1056/NEJMoa1501824
4. Larkin J, Chiarion-Sileni V, Gonzalez R, Grob J-J, Rutkowski P, Lao CD, et al. Five-year survival with combined nivolumab and ipilimumab in advanced melanoma. N Engl J Med. (2019) 381:1535–46. doi: 10.1056/NEJMoa1910836
5. Wojtukiewicz MZ, Rek MM, Karpowicz K, Górska M, Polityńska B, Wojtukiewicz AM, et al. Inhibitors of immune checkpoints—Pd-1, Pd-L1, Ctla-4—new opportunities for cancer patients and a new challenge for internists and general practitioners. Cancer Metastasis Rev. (2021) 40:949–82. doi: 10.1007/s10555-021-09976-0
6. Havel JJ, Chowell D, Chan TA. The evolving landscape of biomarkers for checkpoint inhibitor immunotherapy. Nat Rev Cancer. (2019) 19:133–50. doi: 10.1038/s41568-019-0116-x
7. Valentinuzzi D, Jeraj R. Computational modelling of modern cancer immunotherapy. Phys Med Biol. (2020) 65:24TR01. doi: 10.1088/1361-6560/abc3fc
8. Yang Y, Zhao Y, Liu X, Huang J. Artificial intelligence for prediction of response to cancer immunotherapy. Semin Cancer Biol. (2022) 87:137–47. doi: 10.1016/j.semcancer.2022.11.008
9. Yarchoan M, Hopkins A, Jaffee EM. Tumor mutational burden and response rate to Pd-1 inhibition. N Engl J Med. (2017) 377:2500. doi: 10.1056/NEJMc1713444
10. Samstein RM, Lee CH, Shoushtari AN, Hellmann MD, Shen R, Janjigian YY, et al. Tumor mutational load predicts survival after immunotherapy across multiple cancer types. Nat Genet. (2019) 51:202–6. doi: 10.1038/s41588-018-0312-8
11. Marabelle A, Fakih M, Lopez J, Shah M, Shapira-Frommer R, Nakagawa K, et al. Association of tumour mutational burden with outcomes in patients with advanced solid tumours treated with pembrolizumab: prospective biomarker analysis of the multicohort, open-label, phase 2 keynote-158 study. Lancet Oncol. (2020) 21:1353–65. doi: 10.1016/S1470-2045(20)30445-9
12. Valero C, Lee M, Hoen D, Zehir A, Berger MF, Seshan VE, et al. Response rates to anti-Pd-1 immunotherapy in microsatellite-stable solid tumors with 10 or more mutations per megabase. JAMA Oncol. (2021) 7:739–43. doi: 10.1001/jamaoncol.2020.7684
13. Schumacher TN, Schreiber RD. Neoantigens in cancer immunotherapy. Science. (2015) 348:69–74. doi: 10.1126/science.aaa4971
14. Wang S, Xie K, Liu T. Cancer immunotherapies: from efficacy to resistance mechanisms – not only checkpoint matters. Front Immunol. (2021) 12:690112. doi: 10.3389/fimmu.2021.690112
15. Yuan C, Xiang L, Cao K, Zhang J, Luo Y, Sun W, et al. The prognostic value of tumor mutational burden and immune cell infiltration in esophageal cancer patients with or without radiotherapy. Aging (Albany NY). (2020) 12:4603–16. doi: 10.18632/aging.102917
16. McGrail DJ, Pilié PG, Rashid NU, Voorwerk L, Slagter M, Kok M, et al. High tumor mutation burden fails to predict immune checkpoint blockade response across all cancer types. Ann Oncol. (2021) 32:661–72. doi: 10.1016/j.annonc.2021.02.006
17. Gromeier M, Brown MC, Zhang G, Lin X, Chen YQ, Wei Z, et al. Very low mutation burden is a feature of inflamed recurrent glioblastomas responsive to cancer immunotherapy. Nat Commun. (2021) 12:352. doi: 10.1038/s41467-020-20469-6
18. Noskova H, Kyr M, Pal K, Merta T, Mudry P, Polaskova K, et al. Assessment of tumor mutational burden in pediatric tumors by real-life whole-exome sequencing and in silico simulation of targeted gene panels: how the choice of method could affect the clinical decision? Cancers. (2020) 12:230. doi: 10.3390/cancers12010230
19. Klempner SJ, Fabrizio D, Bane S, Reinhart M, Peoples T, Ali SM, et al. Tumor mutational burden as a predictive biomarker for response to immune checkpoint inhibitors: a review of current evidence. Oncologist. (2020) 25:E147–E59. doi: 10.1634/theoncologist.2019-0244
20. Liu L, Bai X, Wang J, Tang XR, Wu DH, Du SS, et al. Combination of TMB and CNA stratifies prognostic and predictive responses to immunotherapy across metastatic cancer. Clin Cancer Res. (2019) 25:7413–23. doi: 10.1158/1078-0432.CCR-19-0558
21. Galsky MD, Saci A, Szabo PM, Han GC, Grossfeld G, Collette S, et al. Nivolumab in patients with advanced platinum-resistant urothelial carcinoma: efficacy, safety, and biomarker analyses with extended follow-up from Checkmate 275. Clin Cancer Res. (2020) 26:5120–8. doi: 10.1158/1078-0432.CCR-19-4162
22. Feng F, Sun H, Zhao Z, Sun C, Zhao Y, Lin H, et al. Identification of APC mutation as a potential predictor for immunotherapy in colorectal cancer. J Oncol. (2022) 2022:6567998. doi: 10.1155/2022/6567998
23. Wang P, Chen Y, Wang C. Beyond tumor mutation burden: tumor neoantigen burden as a biomarker for immunotherapy and other types of therapy. Front Oncol. (2021) 11:672677. doi: 10.3389/fonc.2021.672677
24. Zhu Y, Meng X, Ruan X, Lu X, Yan F, Wang F. Characterization of neoantigen load subgroups in gynecologic and breast cancers. Front Bioeng Biotechnol. (2020) 8:702. doi: 10.3389/fbioe.2020.00702
25. McGranahan N, Furness AJ, Rosenthal R, Ramskov S, Lyngaa R, Saini SK, et al. Clonal neoantigens elicit T cell immunoreactivity and sensitivity to immune checkpoint blockade. Science. (2016) 351:1463–9. doi: 10.1126/science.aaf1490
26. Wu D, Liu Y, Li X, Liu Y, Yang Q, Liu Y, et al. Identification of clonal neoantigens derived from driver mutations in an EGFR-mutated lung cancer patient benefitting from Anti-PD-1. Front Immunol. (2020) 11:1366. doi: 10.3389/fimmu.2020.01366
27. Mauriello A, Zeuli R, Cavalluzzo B, Petrizzo A, Tornesello ML, Buonaguro FM, et al. High somatic mutation and neoantigen burden do not correlate with decreased progression-free survival in HCC patients not undergoing immunotherapy. Cancers. (2019) 11:1824. doi: 10.3390/cancers11121824
28. Rizvi NA, Hellmann MD, Snyder A, Kvistborg P, Makarov V, Havel JJ, et al. Mutational landscape determines sensitivity to PD-1 blockade in non-small cell lung cancer. Science. (2015) 348:124–8. doi: 10.1126/science.aaa1348
29. Bai R, Lv Z, Xu D, Cui J. Predictive Predictive biomarkers for cancer immunotherapy with immune checkpoint inhibitors. biomark Res. (2020) 8:34. doi: 10.1186/s40364-020-00209-0
30. Lu T, Wang S, Xu L, Zhou Q, Singla N, Gao J, et al. Tumor neoantigenicity assessment with CSiN score incorporates clonality and immunogenicity to predict immunotherapy outcomes. Sci Immunol. (2020) 5:eaaz3199. doi: 10.1126/sciimmunol.aaz3199
31. Su X, Jin H, Wang J, Lu H, Gu T, Gao Z, et al. Construction and validation of an immunoediting-based optimized neoantigen load (Iotnl) model to predict the response and prognosis of immune checkpoint therapy in various cancers. Aging. (2022) 14:4586–605. doi: 10.18632/aging.204101
32. Löffler MW, Mohr C, Bichmann L, Freudenmann LK, Walzer M, Schroeder CM, et al. Multi-omics discovery of exome-derived neoantigens in hepatocellular carcinoma. Genome Med. (2019) 11:28. doi: 10.1186/s13073-019-0636-8
33. Rooney MS, Shukla SA, Wu CJ, Getz G, Hacohen N. Molecular and genetic properties of tumors associated with local immune cytolytic activity. Cell. (2015) 160:48–61. doi: 10.1016/j.cell.2014.12.033
34. Battaglia S. Chapter seventeen - neoantigen prediction from genomic and transcriptomic data. Methods Enzymol. (2020) 635:267–81. doi: 10.1016/bs.mie.2019.10.003
35. Siebenkäs C, Chiappinelli KB, Guzzetta AA, Sharma A, Jeschke J, Vatapalli R, et al. Inhibiting DNA methylation activates cancer testis antigens and expression of the antigen processing and presentation machinery in colon and ovarian cancer cells. PLoS One. (2017) 12:e0179501. doi: 10.1371/journal.pone.0179501
36. Laumont CM, Vincent K, Hesnard L, Audemard É, Bonneil É, Laverdure J-P, et al. Noncoding regions are the main source of targetable tumor-specific antigens. Sci Transl Med. (2018) 10:eaau5516. doi: 10.1126/scitranslmed.aau5516
37. Németh E, Lovrics A, Gervai JZ, Seki M, Rospo G, Bardelli A, et al. Two main mutational processes operate in the absence of DNA mismatch repair. DNA Repair. (2020) 89:102827. doi: 10.1016/j.dnarep.2020.102827
38. Le DT, Durham JN, Smith KN, Wang H, Bartlett BR, Aulakh LK, et al. Mismatch repair deficiency predicts response of solid tumors to PD-1 blockade. Science. (2017) 357:409–13. doi: 10.1126/science.aan6733
39. Hirano H, Takashima A, Hamaguchi T, Shida D, Kanemitsu Y, JCOG JCOG. Current status and perspectives of immune checkpoint inhibitors for colorectal cancer. Jpn J Clin Oncol. (2021) 51:10–9. doi: 10.1093/jjco/hyaa200
40. Trabucco SE, Gowen K, Maund SL, Sanford E, Fabrizio DA, Hall MJ, et al. A novel next-generation sequencing approach to detecting microsatellite instability and pan-tumor characterization of 1000 microsatellite instability-high cases in 67,000 patient samples. J Mol Diagn. (2019) 21:1053–66. doi: 10.1016/j.jmoldx.2019.06.011
41. Li K, Luo H, Huang L, Luo H, Zhu X. Microsatellite instability: A review of what the oncologist should know. Cancer Cell Int. (2020) 20:16. doi: 10.1186/s12935-019-1091-8
42. Cheah P-L, Jing L, Lai-Meng L, Cing-Chai K, Tze-Pheng L, Chang S-W, et al. Screening for microsatellite instability in colorectal carcinoma: practical utility of immunohistochemistry and PCR with fragment analysis in a diagnostic histopathology setting. Malays J Pathol. (2019) 41:91–100.
43. Bai H, Wang R, Cheng W, Shen Y, Li H, Xia W, et al. Evaluation of concordance between deficient mismatch repair and microsatellite instability testing and their association with clinicopathological features in colorectal cancer. Cancer Manag Res. (2020) 12:2863–73. doi: 10.2147/cmar.S248069
44. Trojan J, Stintzing S, Haase O, Koch C, Ziegler P, Demes M, et al. Complete pathological response after neoadjuvant short-course immunotherapy with ipilimumab and nivolumab in locally advanced MSI-H/dMMR rectal cancer. Oncologist. (2021) 26:e2110–e4. doi: 10.1002/onco.13955
45. Zhang CJ, Ding HG, Sun SJ, Luan ZH, Liu GY, Li Z. Incidence and detection of high microsatellite instability in colorectal cancer in a Chinese population: a meta-analysis. J Gastrointest Oncol. (2020) 11:1155–63. doi: 10.21037/jgo-20-487
46. Takamochi K, Takahashi F, Suehara Y, Sato E, Kohsaka S, Hayashi T, et al. DNA mismatch repair deficiency in surgically resected lung adenocarcinoma: Microsatellite instability analysis using the Promega panel. Lung Cancer. (2017) 110:26–31. doi: 10.1016/j.lungcan.2017.05.016
47. Bonneville R, Krook MA, Chen HZ, Smith A, Samorodnitsky E, Wing MR, et al. Detection of microsatellite instability biomarkers via next-generation sequencing. Methods Mol Biol. (2020) . 2055:119–32. doi: 10.1007/978-1-4939-9773-2_5
48. Zhu L, Huang Y, Fang X, Liu C, Deng W, Zhong C, et al. A novel and reliable method to detect microsatellite instability in colorectal cancer by next-generation sequencing. J Mol Diagn. (2018) 20:225–31. doi: 10.1016/j.jmoldx.2017.11.007
49. Savic Prince S, Bubendorf L. Predictive potential and need for standardization of PD-L1 immunohistochemistry. Virchows Arch. (2019) 474:475–84. doi: 10.1007/s00428-018-2445-7
50. Davis AA, Patel VG. The role of PD-L1 expression as a predictive biomarker: an analysis of all Us food and drug administration (FDA) approvals of immune checkpoint inhibitors. J Immunother Cancer. (2019) 7:278. doi: 10.1186/s40425-019-0768-9
51. Shen X, Zhao B. Efficacy of PD-1 or PD-L1 inhibitors and PD-L1 expression status in cancer: Meta-analysis. BMJ. (2018) 362:k3529. doi: 10.1136/bmj.k3529
52. Dafni U, Tsourti Z, Vervita K, Peters S. Immune checkpoint inhibitors, alone or in combination with chemotherapy, as first-line treatment for advanced non-small cell lung cancer. A systematic review and network meta-analysis. Lung cancer. (2019) 134:127–40. doi: 10.1016/j.lungcan.2019.05.029
53. Zhou KI, Peterson B, Serritella A, Thomas J, Reizine N, Moya S, et al. Spatial and temporal heterogeneity of PD-L1 expression and tumor mutational burden in Gastroesophageal Adenocarcinoma at baseline diagnosis and after chemotherapy. Clin Cancer Res. (2020) 26:6453–63. doi: 10.1158/1078-0432.CCR-20-2085
54. Torlakovic E, Lim HJ, Adam J, Barnes P, Bigras G, Chan AW, et al. “Interchangeability” of PD-L1 immunohistochemistry assays: a meta-analysis of diagnostic accuracy. Mod Pathol. (2020) 33:4–17. doi: 10.1038/s41379-019-0327-4
55. Doroshow DB, Bhalla S, Beasley MB, Sholl LM, Kerr KM, Gnjatic S, et al. PD-L1 as a biomarker of response to immune-checkpoint inhibitors. Nat Rev Clin Oncol. (2021) 18:345–62. doi: 10.1038/s41571-021-00473-5
56. Witte HM, Gebauer N, Lappöhn D, Umathum VG, Riecke A, Arndt A, et al. Prognostic impact of PD-L1 expression in Malignant Salivary Gland Tumors as assessed by established scoring criteria: Tumor proportion score (TPS), combined positivity score (CPS), and immune cell (IC) infiltrate. Cancers. (2020) 12:873. doi: 10.3390/cancers12040873
57. Falk AT, Yazbeck N, Guibert N, Chamorey E, Paquet A, Ribeyre L, et al. Effect of mutant variants of the KRAS Gene on PD-L1 expression and on the immune microenvironment and association with clinical outcome in lung adenocarcinoma patients. Lung Cancer. (2018) 121:70–5. doi: 10.1016/j.lungcan.2018.05.009
58. Lapuente-Santana O, Eduati F. Toward systems biomarkers of response to immune checkpoint blockers. Front Oncol. (2020) 10:1027. doi: 10.3389/fonc.2020.01027
59. Goodman RS, Jung S, Balko JM, Johnson DB. Biomarkers of immune checkpoint inhibitor response and toxicity: Challenges and opportunities. Immunol Rev. (2023) 318:157–66. doi: 10.1111/imr.13249
60. Fenton SE, Saleiro D, Platanias LC. Type I and II interferons in the anti-tumor immune response. Cancers. (2021) 13:1037. doi: 10.3390/cancers13051037
61. Chen Q, Li TH, Yue WT. Drug response to PD-1/PD-L1 blockade: Based on biomarkers. Onco Targets Ther. (2018) 11:4673–83. doi: 10.2147/OTT.S168313
62. Jhunjhunwala S, Hammer C, Delamarre L. Antigen presentation in cancer: Insights into tumour immunogenicity and immune evasion. Nat Rev Cancer. (2021) 21:298–312. doi: 10.1038/s41568-021-00339-z
63. Stutvoet TS, Kol A, de Vries EGE, de Bruyn M, Fehrmann RSN, van Scheltinga AGTT, et al. MAPK pathway activity plays a key role in PD-L1 expression of lung adenocarcinoma cells. J Pathol. (2019) 249:52–64. doi: 10.1002/path.5280
64. Diggs LP, Hsueh EC. Utility of PD-L1 immunohistochemistry assays for predicting PD-1/PD-L1 inhibitor response. biomark Res. (2017) 5:12. doi: 10.1186/s40364-017-0093-8
65. Escors D, Gato-Canas M, Zuazo M, Arasanz H, Garcia-Granda MJ, Vera R, et al. The intracellular signalosome of PD-L1 in cancer cells. Signal Transduct Target Ther. (2018) 3:26. doi: 10.1038/s41392-018-0022-9
66. Trujillo JA, Sweis RF, Bao R, Luke JJ. T cell-inflamed versus non-T cell-inflamed tumors: A conceptual framework for cancer immunotherapy drug development and combination therapy selection. Cancer Immunol Res. (2018) 6:990–1000. doi: 10.1158/2326-6066.CIR-18-0277
67. Benjamin DJ, Lyou Y. Advances in immunotherapy and the TGF-β resistance pathway in metastatic bladder cancer. Cancers. (2021) 13:5724. doi: 10.3390/cancers13225724
68. David JM, Dominguez C, McCampbell KK, Gulley JL, Schlom J, Palena C. A novel bifunctional anti-PD-L1/TGF-β trap fusion protein (M7824) efficiently reverts mesenchymalization of human lung cancer cells. OncoImmunology. (2017) 6:e1349589. doi: 10.1080/2162402X.2017.1349589
69. Kurimoto R, Iwasawa S, Ebata T, Ishiwata T, Sekine I, Tada Y, et al. Drug resistance originating from a TGF-β/FGF-2-driven epithelial-to-mesenchymal transition and its reversion in human lung adenocarcinoma cell lines harboring an EGFR mutation. Int J Oncol. (2016) 48:1825–36. doi: 10.3892/ijo.2016.3419
70. Jiang Y, Zhan H. Communication between EMT and PD-L1 signaling: new insights into tumor immune evasion. Cancer Lett. (2020) 468:72–81. doi: 10.1016/j.canlet.2019.10.013
71. Du F, Qi X, Zhang A, Sui F, Wang X, Proud CG, et al. MRTF-A-NF-κB /p65 axis-mediated PD-L1 transcription and expression contributes to immune evasion of non-small-cell lung cancer via TGF-β. Exp Mol Med. (2021) 53:1366–78. doi: 10.1038/s12276-021-00670-3
72. Asgarova A, Asgarov K, Godet Y, Peixoto P, Nadaradjane A, Boyer-Guittaut M, et al. PD-L1 expression is regulated by both DNA methylation and NF-κB during EMT signaling in non-small cell lung carcinoma. Oncoimmunology. (2018) 7:e1423170. doi: 10.1080/2162402X.2017.1423170
73. Ni Y, Soliman A, Joehlin-Price A, Rose PG, Vlad A, Edwards RP, et al. High TGF-β signature predicts immunotherapy resistance in gynecologic cancer patients treated with immune checkpoint inhibition. NPJ Precis Oncol. (2021) 5:101. doi: 10.1038/s41698-021-00242-8
74. Singh RR. Next-generation sequencing in high-sensitive detection of mutations in tumors: challenges, advances, and applications. J Mol Diagn. (2020) 22:994–1007. doi: 10.1016/j.jmoldx.2020.04.213
75. Lei Y, Tang R, Xu J, Wang W, Zhang B, Liu J, et al. Applications of single-cell sequencing in cancer research: progress and perspectives. J Hematol Oncol. (2021) 14:91. doi: 10.1186/s13045-021-01105-2
76. Petti AA, Williams SR, Miller CA, Fiddes IT, Srivatsan SN, Chen DY, et al. A general approach for detecting expressed mutations in AML cells using single cell RNA-sequencing. Nat Commun. (2019) 10:3660. doi: 10.1038/s41467-019-11591-1
77. Byron SA, Van Keuren-Jensen KR, Engelthaler DM, Carpten JD, Craig DW. Translating RNA sequencing into clinical diagnostics: Opportunities and challenges. Nat Rev Genet. (2016) 17:257–71. doi: 10.1038/nrg.2016.10
78. Jung G, Hernández-Illán E, Moreira L, Balaguer F, Goel A. Epigenetics of colorectal cancer: biomarker and therapeutic potential. Nat Rev Gastroenterol Hepatol. (2020) 17:111–30. doi: 10.1038/s41575-019-0230-y
79. Yoshikawa Y, Kuribayashi K, Minami T, Ohmuraya M, Kijima T. Epigenetic alterations and biomarkers for immune checkpoint inhibitors-current standards and future perspectives in Malignant pleural mesothelioma treatment. Front Oncol. (2020) 10:554570. doi: 10.3389/fonc.2020.554570
80. Guerreiro IM, Barros-Silva D, Lopes P, Cantante M, Cunha AL, Lobo J, et al. RAD51Bme levels as a potential predictive biomarker for PD-1 blockade response in non-small cell lung cancer. J Clin Med. (2020) 9:1000. doi: 10.3390/jcm9041000
81. Darvin P, Sasidharan Nair V, Elkord E. PD-L1 expression in human breast cancer stem cells is epigenetically regulated through posttranslational histone modifications. J Oncol. (2019) 2019:3958908. doi: 10.1155/2019/3958908
82. Pan D, Kobayashi A, Jiang P, Ferrari de Andrade L, Tay RE, Luoma AM, et al. A major chromatin regulator determines resistance of tumor cells to T cell–mediated killing. Science. (2018) 359:770–5. doi: 10.1126/science.aao1710
83. Heller G. DNA methylation as predictive marker of response to immunotherapy? Memo-Mag Eur Med Onc. (2021) 14:150–3. doi: 10.1007/s12254-021-00696-3
84. Thomas ML, Marcato P. Epigenetic modifications as biomarkers of tumor development, therapy response, and recurrence across the cancer care continuum. Cancers. (2018) 10:101. doi: 10.3390/cancers10040101
85. Park PJ. Chip-seq: Advantages and challenges of a maturing technology. Nat Rev Genet. (2009) 10:669–80. doi: 10.1038/nrg2641
86. Dai B, Giardina C, Rasmussen TP. Quantitation of nucleosome acetylation and other histone posttranslational modifications using microscale NU-ELISA. Methods Mol Biol. (2013) 981:167–76. doi: 10.1007/978-1-62703-305-3_13
87. Xu Y, Su G-H, Ma D, Xiao Y, Shao Z-M, Jiang Y-Z. Technological advances in cancer immunity: from immunogenomics to single-cell analysis and artificial intelligence. Signal Transduct Target Ther. (2021) 6:312. doi: 10.1038/s41392-021-00729-7
88. Chen Z, Wu A. Progress and challenge for computational quantification of tissue immune cells. Brief Bioinform. (2021) 22:bbaa358. doi: 10.1093/bib/bbaa358
89. Zheng B, Wang D, Qiu X, Luo G, Wu T, Yang S, et al. Trajectory and functional analysis of PD-1high CD4+ CD8+ T Cells in Hepatocellular Carcinoma by single-cell cytometry and transcriptome sequencing. Adv Sci. (2020) 7:2000224. doi: 10.1002/advs.202000224
90. Qi J, Sun H, Zhang Y, Wang Z, Xun Z, Li Z, et al. Single-cell and spatial analysis reveal interaction of FAP+ fibroblasts and SPP1+ macrophages in colorectal cancer. Nat Commun. (2022) 13:1742. doi: 10.1038/s41467-022-29366-6
91. Tajaldini M, Poorkhani A, Amiriani T, Amiriani A, Javid H, Aref P, et al. Strategy of targeting the tumor microenvironment via inhibition of fibroblast/fibrosis remodeling new era to cancer chemo-immunotherapy resistance. Eur J Pharmacol. (2023) 957:175991. doi: 10.1016/j.ejphar.2023.175991
92. Jensen C, Nissen NI, Von Arenstorff CS, Karsdal MA, Willumsen N. Serological assessment of collagen fragments and tumor fibrosis may guide immune checkpoint inhibitor therapy. J Exp Clin Canc Res. (2021) 40:326. doi: 10.1186/s13046-021-02133-z
93. Das M, Zhu C, Kuchroo VK. Tim-3 and its role in regulating anti-tumor immunity. Immunol Rev. (2017) 276:97–111. doi: 10.1111/imr.12520
94. Murciano-Goroff YR, Warner AB, Wolchok JD. The future of cancer immunotherapy: microenvironment-targeting combinations. Cell Res. (2020) 30:507–19. doi: 10.1038/s41422-020-0337-2
95. Liu X, Wu J. History, applications, and challenges of immune repertoire research. Cell Biol Toxicol. (2018) 34:441–57. doi: 10.1007/s10565-018-9426-0
96. Aversa I, Malanga D, Fiume G, Palmieri C. Molecular T-cell repertoire analysis as source of prognostic and predictive biomarkers for checkpoint blockade immunotherapy. Int J Mol Sci. (2020) 21:2378. doi: 10.3390/ijms21072378
97. Hopkins AC, Yarchoan M, Durham JN, Yusko EC, Rytlewski JA, Robins HS, et al. T cell receptor repertoire features associated with survival in immunotherapy-treated pancreatic ductal adenocarcinoma. JCI Insight. (2018) 3:e122092. doi: 10.1172/jci.insight.122092
98. Ye J, Ma N, Madden TL, Ostell JM. gBLAST: an immunoglobulin variable domain sequence analysis tool. Nucleic Acids Res. (2013) 41:W34–40. doi: 10.1093/nar/gkt382
99. Gaëta BA, Malming HR, Jackson KJL, Bain ME, Wilson P, Collins AM. iHMMune-align: Hidden Markov model-based alignment and identification of germline genes in rearranged immunoglobulin gene sequences. Bioinformatics. (2007) 23:1580–7. doi: 10.1093/bioinformatics/btm147
100. Brochet X, Lefranc M-P, Giudicelli V. IMGT/V-QUEST: the highly customized and integrated system for IG and TR standardized V-J and V-D-J sequence analysis. Nucleic Acids Res. (2008) 36:W503–W8. doi: 10.1093/nar/gkn316
101. Bolotin DA, Poslavsky S, Mitrophanov I, Shugay M, Mamedov IZ, Putintseva EV, et al. MiXCR: software for comprehensive adaptive immunity profiling. Nat Methods. (2015) 12:380–1. doi: 10.1038/nmeth.3364
102. Marcou Q, Mora T, Walczak AM. High-throughput immune repertoire analysis with IGoR. Nat Commun. (2018) 9:561. doi: 10.1038/s41467-018-02832-w
103. Song L, Cohen D, Ouyang Z, Cao Y, Hu X, Liu XS. TRUST4: Immune repertoire reconstruction from bulk and single-cell RNA-seq data. Nat Methods. (2021) 18:627–30. doi: 10.1038/s41592-021-01142-2
104. Alix-Panabieres C. Perspective: The future of liquid biopsy. Nature. (2020) 579:S9–S. doi: 10.1038/d41586-020-00844-5
105. Honrubia-Peris B, Garde-Noguera J, García-Sánchez J, Piera-Molons N, Llombart-Cussac A, Fernández-Murga ML. Soluble biomarkers with prognostic and predictive value in advanced non-small cell lung cancer treated with immunotherapy. Cancers. (2021) 13:4280. doi: 10.3390/cancers13174280
106. Bai Y, Zhao HT. Liquid biopsy in tumors: opportunities and challenges. Ann Transl Med. (2018) 6:S89. doi: 10.21037/atm.2018.11.31
107. Bratman SV, Yang S, Iafolla MA, Liu Z, Hansen AR, Bedard PL, et al. Personalized circulating tumor DNA analysis as a predictive biomarker in solid tumor patients treated with pembrolizumab. Nat Cancer. (2020) 1:873–81. doi: 10.1038/s43018-020-0096-5
108. Nicolazzo C, Raimondi C, Mancini M, Caponnetto S, Gradilone A, Gandini O, et al. Monitoring PD-L1 positive circulating tumor cells in non-small cell lung cancer patients treated with the PD-1 inhibitor Nivolumab. Sci Rep. (2016) 6:31726. doi: 10.1038/srep31726
109. Incorvaia L, Fanale D, Badalamenti G, Brando C, Bono M, De Luca I, et al. A “lymphocyte microrna signature” as predictive biomarker of immunotherapy response and plasma PD-1/PD-L1 expression levels in patients with metastatic Renal Cell Carcinoma: pointing towards epigenetic reprogramming. Cancers. (2020) 12:3396. doi: 10.3390/cancers12113396
110. Postel M, Roosen A, Laurent-Puig P, Taly V, Wang-Renault S-F. Droplet-based digital PCR and next generation sequencing for monitoring circulating tumor DNA: a cancer diagnostic perspective. Expert Rev Mol Diagn. (2018) 18:7–17. doi: 10.1080/14737159.2018.1400384
111. Wu X, Li J, Gassa A, Buchner D, Alakus H, Dong Q, et al. Circulating tumor DNA as an emerging liquid biopsy biomarker for early diagnosis and therapeutic monitoring in hepatocellular carcinoma. Int J Biol Sci. (2020) 16:1551–62. doi: 10.7150/ijbs.44024
112. Leary RJ, Sausen M, Kinde I, Papadopoulos N, Carpten JD, Craig D, et al. Detection of chromosomal alterations in the circulation of cancer patients with whole-genome sequencing. Sci Transl Med. (2012) 4:162ra154. doi: 10.1126/scitranslmed.3004742
113. Gorgannezhad L, Umer M, Islam MN, Nguyen N-T, Shiddiky MJ. Circulating tumor DNA and liquid biopsy: opportunities, challenges, and recent advances in detection technologies. Lab Chip. (2018) 18:1174–96. doi: 10.1039/C8LC00100F
114. Maron SB, Chase LM, Lomnicki S, Kochanny S, Moore KL, Joshi SS, et al. Circulating tumor DNA sequencing analysis of gastroesophageal adenocarcinoma. Clin Cancer Res. (2019) 25:7098–112. doi: 10.1158/1078-0432.CCR-19-1704
115. Maravelia P, Silva DN, Rovesti G, Chrobok M, Stål P, Lu Y-C, et al. Liquid biopsy in hepatocellular carcinoma: opportunities and challenges for immunotherapy. Cancers. (2021) 13:4334. doi: 10.3390/cancers13174334
116. Swennenhuis JF, Tibbe AG, Levink R, Sipkema RC, Terstappen LW. Characterization of circulating tumor cells by fluorescence in situ hybridization. Cytometry. (2009) 75:520–7. doi: 10.1002/cyto.a.20718
117. Jiang R, Lu YT, Ho H, Li B, Chen JF, Lin M, et al. A comparison of isolated circulating tumor cells and tissue biopsies using whole-genome sequencing in prostate cancer. Oncotarget. (2015) 6:44781–93. doi: 10.18632/oncotarget.6330
118. Fernandez-Garcia D, Nteliopoulos G, Hastings RK, Rushton A, Page K, Allsopp RC, et al. Shallow WGS of individual CTCs identifies actionable targets for informing treatment decisions in metastatic breast cancer. Br J Cancer. (2022) 127:1858–64. doi: 10.1038/s41416-022-01962-9
119. Manier S, Park J, Capelletti M, Bustoros M, Freeman SS, Ha G, et al. Whole-exome sequencing of cell-free DNA and circulating tumor cells in multiple myeloma. Nat Commun. (2018) 9:1691. doi: 10.1038/s41467-018-04001-5
120. Liu HE, Triboulet M, Zia A, Vuppalapaty M, Kidess-Sigal E, Coller J, et al. Workflow optimization of whole genome amplification and targeted panel sequencing for CTC mutation detection. NPJ Genom Med. (2017) 2:34. doi: 10.1038/s41525-017-0034-3
121. Lang JE, Ring A, Porras T, Kaur P, Forte VA, Mineyev N, et al. RNA-seq of circulating tumor cells in stage II–III breast cancer. Ann Surg Oncol. (2018) 25:2261–70. doi: 10.1245/s10434-018-6540-4
122. Gopalakrishnan V, Spencer CN, Nezi L, Reuben A, Andrews MC, Karpinets TV, et al. Gut microbiome modulates response to anti-PD-1 immunotherapy in melanoma patients. Science. (2018) 359:97–103. doi: 10.1126/science.aan4236
123. Davar D, Dzutsev AK, McCulloch JA, Rodrigues RR, Chauvin J-M, Morrison RM, et al. Fecal microbiota transplant overcomes resistance to anti-PD-1 therapy in melanoma patients. Science. (2021) 371:595–602. doi: 10.1126/science.abf3363
124. Wang Y, Ma R, Liu F, Lee SA, Zhang L. Modulation of gut microbiota: A novel paradigm of enhancing the efficacy of programmed death-1 and programmed death ligand-1 blockade therapy. Front Immunol. (2018) 9:374. doi: 10.3389/fimmu.2018.00374
125. Routy B, Le Chatelier E, Derosa L, Duong CPM, Alou MT, Daillère R, et al. Gut microbiome influences efficacy of PD-1-based immunotherapy against epithelial tumors. Science. (2018) 359:91–7. doi: 10.1126/science.aan3706
126. Ouaknine Krief J, Helly de Tauriers P, Dumenil C, Neveux N, Dumoulin J, Giraud V, et al. Role of antibiotic use, plasma citrulline and blood microbiome in advanced non-small cell lung cancer patients treated with nivolumab. J Immunother Cancer. (2019) 7:176. doi: 10.1186/s40425-019-0658-1
127. Hakozaki T, Okuma Y, Omori M, Hosomi Y. Impact of prior antibiotic use on the efficacy of nivolumab for non-small cell lung cancer. Oncol Lett. (2019) 17:2946–52. doi: 10.3892/ol.2019.9899
128. McCulloch JA, Davar D, Rodrigues RR, Badger JH, Fang JR, Cole AM, et al. Intestinal microbiota signatures of clinical response and immune-related adverse events in melanoma patients treated with anti-PD-1. Nat Med. (2022) 28:545–56. doi: 10.1038/s41591-022-01698-2
129. Zheng Y, Wang T, Tu X, Huang Y, Zhang H, Tan D, et al. Gut microbiome affects the response to anti-PD-1 immunotherapy in patients with hepatocellular carcinoma. J Immunother cancer. (2019) 7:193. doi: 10.1186/s40425-019-0650-9
130. Buchan BW, Ledeboer NA. Emerging technologies for the clinical microbiology laboratory. Clin Microbiol Rev. (2014) 27:783–822. doi: 10.1128/CMR.00003-14
131. Carey CM, Kirk JL, Ojha S, Kostrzynska M. Current and future uses of real-time polymerase chain reaction and microarrays in the study of intestinal microbiota, and probiotic use and effectiveness. Can J Microbiol. (2007) 53:537–50. doi: 10.1139/W07-039
132. Sanschagrin S, Yergeau E. Next-generation sequencing of 16S ribosomal RNA gene amplicons. J Vis Exp. (2014) 90):51709. doi: 10.3791/51709
133. Derosa L, Routy B, Kroemer G, Zitvogel L. The intestinal microbiota determines the clinical efficacy of immune checkpoint blockers targeting PD-1/PD-L1. Oncoimmunology. (2018) 7:e1434468. doi: 10.1080/2162402X.2018.1434468
134. Quince C, Walker AW, Simpson JT, Loman NJ, Segata N. Shotgun metagenomics, from sampling to analysis. Nat Biotechnol. (2017) 35:833–44. doi: 10.1038/nbt.3935
135. Gao B, Chi L, Zhu Y, Shi X, Tu P, Li B, et al. An introduction to next generation sequencing bioinformatic analysis in gut microbiome studies. Biomolecules. (2021) 11:530. doi: 10.3390/biom11040530
136. Isaac NI, Philippe D, Nicholas A, Raoult D, Eric C. Metaproteomics of the human gut microbiota: challenges and contributions to other OMICS. Clin Mass Spectrom. (2019) 14:18–30. doi: 10.1016/j.clinms.2019.06.001
137. Bauermeister A, Mannochio-Russo H, Costa-Lotufo LV, Jarmusch AK, Dorrestein PC. Mass spectrometry-based metabolomics in microbiome investigations. Nat Rev Microbiol. (2022) 20:143–60. doi: 10.1038/s41579-021-00621-9
138. Kumar A, Chamoto K. Immune metabolism in PD-1 blockade-based cancer immunotherapy. Int Immunol. (2021) 33:17–26. doi: 10.1093/intimm/dxaa046
139. Hatae R, Chamoto K, Kim YH, Sonomura K, Taneishi K, Kawaguchi S, et al. Combination of host immune metabolic biomarkers for the PD-1 blockade cancer immunotherapy. JCI Insight. (2020) 5:e133501. doi: 10.1172/jci.insight.133501
140. Zhang H, Zhao W, Li X, He Y. Cholesterol metabolism as a potential therapeutic target and a prognostic biomarker for cancer immunotherapy. Onco Targets Ther. (2021) 14:3803–12. doi: 10.2147/ott.S315998
141. Nomura M, Nagatomo R, Doi K, Shimizu J, Baba K, Saito T, et al. Association of short-chain fatty acids in the gut microbiome with clinical response to treatment with nivolumab or pembrolizumab in patients with solid cancer tumors. JAMA Netw Open. (2020) 3:e202895. doi: 10.1001/jamanetworkopen.2020.2895
142. Su K-Y, Lee W-L. Fourier transform infrared spectroscopy as a cancer screening and diagnostic tool: a review and prospects. Cancers. (2020) 12:115. doi: 10.3390/cancers12010115
143. Letertre MP, Dervilly G, Giraudeau P. Combined nuclear magnetic resonance spectroscopy and mass spectrometry approaches for metabolomics. Anal Chem. (2021) 93:500–18. doi: 10.1021/acs.analchem.0c04371
144. Yoon SJ, Lee CB, Chae SU, Jo SJ, Bae SK. The comprehensive “Omics” approach from metabolomics to advanced omics for development of immune checkpoint inhibitors: Potential strategies for next generation of cancer immunotherapy. Int J Mol Sci. (2021) 22:6932. doi: 10.3390/ijms22136932
145. Smith CA, Want EJ, O'Maille G, Abagyan R, Siuzdak G. XCMS: processing mass spectrometry data for metabolite profiling using nonlinear peak alignment, matching, and identification. Anal Chem. (2006) 78:779–87. doi: 10.1021/ac051437y
146. Katajamaa M, Miettinen J, Orešič M. MZmine: Toolbox for processing and visualization of mass spectrometry based molecular profile data. Bioinformatics. (2006) 22:634–6. doi: 10.1093/bioinformatics/btk039
147. Liang D, Liu Q, Zhou K, Jia W, Xie G, Chen T. IP4M: An integrated platform for mass spectrometry-based metabolomics data mining. BMC Bioinf. (2020) 21:444. doi: 10.1186/s12859-020-03786-x
148. Shen X, Zhu Z-J. MetFlow: an interactive and integrated workflow for metabolomics data cleaning and differential metabolite discovery. Bioinformatics. (2019) 35:2870–2. doi: 10.1093/bioinformatics/bty1066
149. Tormoen GW, Crittenden MR, Gough MJ. Role of the immunosuppressive microenvironment in immunotherapy. Adv Radiat Oncol. (2018) 3:520–6. doi: 10.1016/j.adro.2018.08.018
150. Vitale I, Shema E, Loi S, Galluzzi L. Intratumoral heterogeneity in cancer progression and response to immunotherapy. Nat Med. (2021) 27:212–24. doi: 10.1038/s41591-021-01233-9
151. Peskov K, Azarov I, Chu L, Voronova V, Kosinsky Y, Helmlinger G. Quantitative mechanistic modeling in support of pharmacological therapeutics development in immuno-oncology. Front Immunol. (2019) 10:924. doi: 10.3389/fimmu.2019.00924
152. Benzekry S, Grangeon M, Karlsen M, Alexa M, Bicalho-Frazeto I, Chaleat S, et al. Machine learning for prediction of immunotherapy efficacy in non-small cell lung cancer from simple clinical and biological data. Cancers. (2021) 13:6210. doi: 10.3390/cancers13246210
153. Whalen S, Schreiber J, Noble WS, Pollard KS. Navigating the pitfalls of applying machine learning in genomics. Nat Rev Genet. (2022) 23:169–81. doi: 10.1038/s41576-021-00434-9
154. Loisios-Konstantinidis I, Mavroudis PD, Macheras P. Dynamical aspects of pharmacokinetic/pharmacodynamic & quantitative systems pharmacology models. In: Approaching Complex Diseases: Network-Based Pharmacology and Systems Approach in Bio-Medicine Cham: Springer International Publishing. (2020). p. 35–61. doi: 10.1007/978-3-030-32857-3_2
155. Netterberg I, Li CC, Molinero L, Budha N, Sukumaran S, Stroh M, et al. A PK/PD analysis of circulating biomarkers and their relationship to tumor response in Atezolizumab-treated non-small cell lung cancer patients. Clin Pharmacol Ther. (2019) 105:486–95. doi: 10.1002/cpt.1198
156. Liu C, Yu J, Li H, Liu J, Xu Y, Song P, et al. Association of time-varying clearance of nivolumab with disease dynamics and its implications on exposure response analysis. Clin Pharmacol Ther. (2017) 101:657–66. doi: 10.1002/cpt.656
157. Zhang Z, Liu L, Ma C, Cui X, Lam RHW, Chen W. An in silico glioblastoma microenvironment model dissects the immunological mechanisms of resistance to PD-1 checkpoint blockade immunotherapy. Small Methods. (2021) 5:2100197. doi: 10.1002/smtd.202100197
158. Siewe N, Friedman A. TGF-β inhibition can overcome cancer primary resistance to PD-1 blockade: A mathematical model. PLoS One. (2021) 16:e0252620. doi: 10.1371/journal.pone.0252620
159. Brogden KA, Parashar D, Hallier AR, Braun T, Qian F, Rizvi NA, et al. Genomics of NSCLC patients both affirm PD-L1 expression and predict their clinical responses to anti-PD-1 immunotherapy. BMC Cancer. (2018) 18:225. doi: 10.1186/s12885-018-4134-y
160. Aghamiri SS, Amin R, Helikar T. Recent applications of quantitative systems pharmacology and machine learning models across diseases. J Pharmacokinet Phar. (2022) 49:19–37. doi: 10.1007/s10928-021-09790-9
161. Zhang S, Gong C, Ruiz-Martinez A, Wang H, Davis-Marcisak E, Deshpande A, et al. Integrating single cell sequencing with a spatial quantitative systems pharmacology model spQSP for personalized prediction of triple-negative breast cancer immunotherapy response. ImmunoInformatics. (2021) 1-2:100002. doi: 10.1016/j.immuno.2021.100002
162. Sun X, Hu B. Mathematical modeling and computational prediction of cancer drug resistance. Brief Bioinform. (2018) 19:1382–99. doi: 10.1093/bib/bbx065
163. Norton K-A, Gong C, Jamalian S, Popel AS. Multiscale agent-based and hybrid modeling of the tumor immune microenvironment. Processes. (2019) 7:37. doi: 10.3390/pr7010037
164. Storey KM, Jackson TL. An agent-based model of combination oncolytic viral therapy and anti-PD-1 immunotherapy reveals the importance of spatial location when treating glioblastoma. Cancers. (2021) 13:5314. doi: 10.3390/cancers13215314
165. Yang Y, Yang J, Shen L, Chen J, Xia L, Ni B, et al. A multi-omics-based serial deep learning approach to predict clinical outcomes of single-agent anti-PD-1/PD-L1 immunotherapy in advanced stage non-small-cell lung cancer. Am J Transl Res. (2021) 13:743–56.
166. Bourbonne V, Geier M, Schick U, Lucia F. Multi-omics approaches for the prediction of clinical endpoints after immunotherapy in non-small cell lung cancer: a comprehensive review. Biomedicines. (2022) 10:1237. doi: 10.3390/biomedicines10061237
167. Jin W, Luo Q. When artificial intelligence meets PD-1/PD-L1 inhibitors: Population screening, response prediction and efficacy evaluation. Comput Biol Med. (2022) 145:105499. doi: 10.1016/j.compbiomed.2022.105499
168. Chen J, Zhang L. A survey and systematic assessment of computational methods for drug response prediction. Brief Bioinform. (2021) 22:232–46. doi: 10.1093/bib/bbz164
169. Firoozbakht F, Yousefi B, Schwikowski B. An overview of machine learning methods for monotherapy drug response prediction. Brief Bioinform. (2022) 23:bbab408. doi: 10.1093/bib/bbab408
170. Xue G, Cui Z-J, Zhou X-H, Zhu Y-X, Chen Y, Liang F-J, et al. DNA methylation biomarkers predict objective responses to PD-1/PD-L1 inhibition blockade. Front Genet. (2019) 10:724. doi: 10.3389/fgene.2019.00724
171. Sun R, Limkin EJ, Vakalopoulou M, Dercle L, Champiat S, Han SR, et al. A radiomics approach to assess tumour-infiltrating CD8 cells and response to anti-PD-1 or anti-PD-L1 immunotherapy: An imaging biomarker, retrospective multicohort study. Lancet Oncol. (2018) 19:1180–91. doi: 10.1016/S1470-2045(18)30413-3
172. Khairunnahar L, Hasib MA, Rezanur RHB, Islam MR, Hosain MK. Classification of Malignant and benign tissue with logistic regression. Inf Med Unlocked. (2019) 16:100189. doi: 10.1016/j.imu.2019.100189
173. Kleinbaum DG, Klein M. Maximum likelihood techniques: An overview. In: Logistic regression. New York, NY: Springer. (2010). p. 103–27. doi: 10.1007/978-1-4419-1742-3_4
174. Khandezamin Z, Naderan M, Rashti MJ. Detection and classification of breast cancer using logistic regression feature selection and GMDH classifier. J BioMed Inf. (2020) 111:103591. doi: 10.1016/j.jbi.2020.103591
175. Park KJ, Lee J-L, Yoon S-K, Heo C, Park BW, Kim JK. Radiomics-based prediction model for outcomes of PD-1/PD-L1 immunotherapy in metastatic urothelial carcinoma. Eur Radiol. (2020) 30:5392–403. doi: 10.1007/s00330-020-06847-0
176. Refae S, Gal J, Ebran N, Otto J, Borchiellini D, Peyrade F, et al. Germinal immunogenetics predict treatment outcome for PD-1/PD-L1 checkpoint inhibitors. Invest New Drugs. (2020) 38:160–71. doi: 10.1007/s10637-019-00845-w
177. Zhang A, Zhong G, Wang L, Cai R, Han R, Xu C, et al. Dynamic serum biomarkers to predict the efficacy of PD-1 in patients with nasopharyngeal carcinoma. Cancer Cell Int. (2021) 21:518. doi: 10.1186/s12935-021-02217-y
178. Shang S, Li X, Gao Y, Guo S, Sun D, Zhou H, et al. MeImmS: predict clinical benefit of Anti-PD-1/PD-L1 treatments based on DNA methylation in non-small cell lung cancer. Front Genet. (2021) 12:676449. doi: 10.3389/fgene.2021.676449
179. Lu Z, Chen H, Jiao X, Zhou W, Han W, Li S, et al. Prediction of immune checkpoint inhibition with immune oncology-related gene expression in gastrointestinal cancer using a machine learning classifier. J Immunother Cancer. (2020) 8:e000631. doi: 10.1136/jitc-2020-000631
180. Huang S, Cai N, Pacheco PP, Narrandes S, Wang Y, Xu W. Applications of support vector machine (SVM) learning in cancer genomics. Cancer Genom Proteom. (2018) 15:41–51. doi: 10.21873/cgp.20063
181. Fawagreh K, Gaber MM, Elyan E. Random forests: from early developments to recent advancements. Syst Sci Control Engineering: Open Access J. (2014) 2:602–9. doi: 10.1080/21642583.2014.956265
182. Schonlau M, Zou RY. The random forest algorithm for statistical learning. Stata J. (2020) 20:3–29. doi: 10.1177/1536867X20909688
183. Chaurasia V, Pal S. Applications of machine learning techniques to predict diagnostic breast cancer. SN Comput Sci. (2020) 1:270. doi: 10.1007/s42979-020-00296-8
184. Abu Alfeilat HA, Hassanat AB, Lasassmeh O, Tarawneh AS, Alhasanat MB, Eyal Salman HS, et al. Effects of distance measure choice on k-nearest neighbor classifier performance: A review. Big data. (2019) 7:221–48. doi: 10.1089/big.2018.0175
185. Peng Z, Cheng S, Kou Y, Wang Z, Jin R, Hu H, et al. The gut microbiome is associated with clinical response to anti–PD-1/PD-L1 immunotherapy in gastrointestinal cancer. Cancer Immunol Res. (2020) 8:1251–61. doi: 10.1158/2326-6066.cir-19-1014
186. Wu Y-C, Feng J-W. Development and application of artificial neural network. Wireless Pers Commun. (2018) 102:1645–56. doi: 10.1007/s11277-017-5224-x
187. Feng G, He N, Xia HHX, Mi M, Wang K, Byrne CD, et al. Machine learning algorithms based on proteomic data mining accurately predicting the recurrence of hepatitis B-related hepatocellular carcinoma. J Gastroen Hepatol. (2022) 37:2145–53. doi: 10.1111/jgh.15940
188. Akermi S, Sahoo S, Panesar R, Bernard J, Dey A, Biswas R, et al. Drug response prediction using machine learning. In: Computational Intelligence in Oncology. Singapore: Springer (2022). p. 349–68. doi: 10.1007/978-981-16-9221-5_20
189. Huang Y, Sun S, Duan X, Chen Z. A study on deep neural networks framework. 2016 IEEE Advanced Information Management, Communicates, Electronic and Automation Control Conference (IMCEC), Xi'an, China. (2016) pp. 1519–22. doi: 10.1109/IMCEC.2016.7867471
190. Peng J, Zou D, Gong W, Kang S, Han L. Deep neural network classification based on somatic mutations potentially predicts clinical benefit of immune checkpoint blockade in lung adenocarcinoma. OncoImmunology. (2020) 9:1734156. doi: 10.1080/2162402X.2020.1734156
191. Arbour KC, Luu AT, Luo J, Rizvi H, Plodkowski AJ, Sakhi M, et al. Deep learning to estimate RECIST in patients with NSCLC treated with PD-1 blockade. Cancer Discovery. (2021) 11:59–67. doi: 10.1158/2159-8290.cd-20-0419
192. Alzubaidi L, Zhang J, Humaidi AJ, Al-Dujaili A, Duan Y, Al-Shamma O, et al. Review of deep learning: concepts, CNN architectures, challenges, applications, future directions. J Big Data. (2021) 8:53. doi: 10.1186/s40537-021-00444-8
193. Arora D, Garg M, Gupta M. Diving deep in deep convolutional neural network. 2020 2nd International Conference on Advances in Computing, Communication Control and Networking (ICACCCN), Greater Noida, India. (2020) pp. 749–51. doi: 10.1109/ICACCCN51052.2020.9362907
194. Tian Y. Artificial intelligence image recognition method based on convolutional neural network algorithm. IEEE Access. (2020) 8:125731–44. doi: 10.1109/ACCESS.2020.3006097
195. Hu J, Cui C, Yang W, Huang L, Yu R, Liu S, et al. Using deep learning to predict anti-PD-1 response in melanoma and lung cancer patients from histopathology images. Transl Oncol. (2021) 14:100921. doi: 10.1016/j.tranon.2020.100921
196. Przedborski M, Smalley M, Thiyagarajan S, Goldman A, Kohandel M. Systems biology informed neural networks (SBINN) predict response and novel combinations for PD-1 checkpoint blockade. Commun Biol. (2021) 4:877. doi: 10.1038/s42003-021-02393-7
197. Benzekry S. Artificial intelligence and mechanistic modeling for clinical decision making in oncology. Clin Pharmacol Ther. (2020) 108:471–86. doi: 10.1002/cpt.1951
198. Kim KH, Kim CG, Shin E-C. Peripheral blood immune cell-based biomarkers in anti-PD-1/PD-L1 therapy. Immune Netw. (2020) 20:e8. doi: 10.4110/in.2020.20.e8
199. Hayase E, Jenq RR. Role of the intestinal microbiome and microbial-derived metabolites in immune checkpoint blockade immunotherapy of cancer. Genome Med. (2021) 13:107. doi: 10.1186/s13073-021-00923-w
200. Lee JY, Kannan B, Lim BY, Li Z, Lim AH, Loh JW, et al. The multi-dimensional biomarker landscape in cancer immunotherapy. Int J Mol Sci. (2022) 23:7839. doi: 10.3390/ijms23147839
201. Sancho-Araiz A, Mangas-Sanjuan V, Trocóniz IF. The role of mathematical models in immuno-oncology: challenges and future perspectives. Pharmaceutics. (2021) 13:1016. doi: 10.3390/pharmaceutics13071016
Keywords: tumor, ICI immunotherapy, biomarkers, computational models, prediction of treatment effectiveness
Citation: Qin Y, Huo M, Liu X and Li SC (2024) Biomarkers and computational models for predicting efficacy to tumor ICI immunotherapy. Front. Immunol. 15:1368749. doi: 10.3389/fimmu.2024.1368749
Received: 11 January 2024; Accepted: 27 February 2024;
Published: 08 March 2024.
Edited by:
Jehad Charo, Roche (Switzerland), SwitzerlandReviewed by:
Priscilla S. Briquez, University of Freiburg Medical Center, GermanyKosuke Kawaguchi, Kyoto University, Japan
Copyright © 2024 Qin, Huo, Liu and Li. This is an open-access article distributed under the terms of the Creative Commons Attribution License (CC BY). The use, distribution or reproduction in other forums is permitted, provided the original author(s) and the copyright owner(s) are credited and that the original publication in this journal is cited, in accordance with accepted academic practice. No use, distribution or reproduction is permitted which does not comply with these terms.
*Correspondence: Xingwu Liu, bGl1eGluZ3d1QGRsdXQuZWR1LmNu; Shuai Cheng Li, c2MubGlAY2l0eXUuZWR1Lmhr