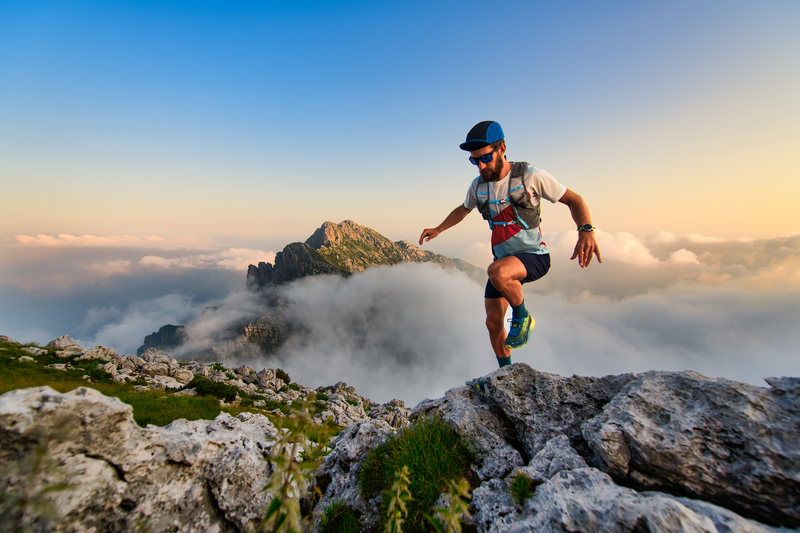
94% of researchers rate our articles as excellent or good
Learn more about the work of our research integrity team to safeguard the quality of each article we publish.
Find out more
ORIGINAL RESEARCH article
Front. Immunol. , 28 February 2024
Sec. Autoimmune and Autoinflammatory Disorders: Autoinflammatory Disorders
Volume 15 - 2024 | https://doi.org/10.3389/fimmu.2024.1289049
Background: Inflammatory bowel disease (IBD) and ankylosing spondylitis (AS) share common traits of chronic recurrent inflammation affecting both the intestines and joints. Epidemiological studies have revealed that the incidence of AS has jumped from 0.3% to 3% among patients with IBD. However, these findings do not definitively establish a causal relationship whereby IBD directly leads to the development of AS. Moreover, whether the activity of IBD will have an impact on this process remains a pending question.
Methods: Two-sample Mendelian randomization (MR) analyses were employed across multiple datasets to investigate the potential of IBD as a risk factor for AS. The pathogenic genes of AS were identified by MR analysis of expression quantitative trait locus. Risk scores for active and inactive patients were calculated by single-sample gene set enrichment analysis. Comparative assessments encompassing alterations in risk transcription factor activity, shifts in signaling pathways, and variances in immune cell profiles were conducted between active and inactive patients. Moreover, the correlation of immune cells and risk genes was quantified.
Results: A total of 6 MR analyses, conducted across 3 exposure datasets and 2 outcome datasets, consistently revealed that IBD substantially elevates the risk of AS development. The MR analysis of the two outcome datasets identified 66 and 54 risk genes, respectively. Notably, both the risk scores computed from the two distinct sets of risk genes were notably higher in active patients compared to their inactive counterparts. Discernible variations in the activity of risk-associated transcription factors were observed between active and inactive patients. In addition, three inflammatory pathways exhibited marked activation in active patients. Moreover, seven specific immune cell types, closely linked to disease activity, exhibited statistically significant correlations with the identified risk genes.
Conclusion: By combining Mendelian randomization with transcriptome analysis, this study postulates IBD as a significant risk factor for AS, and further presents innovative evidence for the impact of IBD activity on the progression of AS.
Inflammatory bowel disease (IBD), including Crohn’s disease (CD) and ulcerative colitis (UC), is a chronic immune-mediated disorder that affects the gastrointestinal system (1). Ankylosing spondylitis (AS), also termed as radiographic axial spondyloarthritis, is a chronic inflammatory disease affecting the joints and spine (2). Both IBD and AS are characterized by recurrent inflammation with intestinal and joint involvement (3, 4). A substantial body of evidence from clinical manifestations, epidemiology, and pathogenesis has collectively substantiated their interconnectedness. Arthritis and spondyloarthropathy stand as classic extraintestinal manifestations of IBD, while IBD also constitutes a prevalent extra-articular feature of AS (5). Inflammatory bowel disease arthritis and AS belong to the broader concept of spondyloarthritis (3). The concurrent effectiveness of TNF-α inhibitors in both diseases also hints at their shared underlying mechanisms (6, 7). Particularly noteworthy is the fact that while the incidence of AS ranges from 0.09% to 0.3% in healthy populations, its prevalence surges to approximately 3% (95% CI 2-4%) among IBD patients, marking a more than tenfold increase (8, 9).
However, little is known about the intricate mechanism underpinning the connection between IBD and AS. Moreover, the observed epidemiological associations, reflecting an elevated AS incidence within the IBD population, were limited, leaving us with a compelling query: Does IBD function as a causative risk factor, driving the increased incidence of AS? Alternatively, could these conditions share upstream co-pathogenic elements that predispose them to co-occurrence, lacking a direct causal link? Furthermore, if IBD does indeed contribute to the development of AS, would the activity of IBD have an impact on this process? Given the recurrent and relatively low-incidence nature of IBD and AS, conventional clinical investigations face challenges in addressing these inquiries effectively.
Mendelian randomization (MR) analysis utilizes genetic variants as instrumental variables to simulate natural experiments, enabling the exploration of causal relationships between exposures and outcomes (10). This study uniquely employed a combination of MR analysis and transcriptome analysis to investigate the effect of gene expression levels on disease risk, providing a novel molecular-level approach for unraveling these complex clinical inquiries.
The overall design of the study were depicted in Figure 1.
The genome-wide association studies (GWAS) data of IBD and AS was acquired from IEU OpenGWAS project (https://gwas.mrcieu.ac.uk/). To ensure result validation, various datasets from distinct consortiums meeting a threshold of over 9,000,000 SNPs were employed as both exposures and outcomes. MR Analysis was performed separately across each exposure and outcome dataset. The selection criteria for instrumental variable (IV) were as follows: (1)The IV was associated with the risk factor. (2) The IV was not associated with confounders. (3) The IV influenced outcome only through the risk factor. The selection of IV was based on a threshold of P< 5x10-8 and r = 0.001. However if fewer than 20 IVs were available, the threshold was adjusted to P< 5x10^-6 and r = 0.01 (11). SNPs that were directly related to the outcome (Poutcome< 5x10-6) were removed first. The F statistic was used to evaluate weak instrumental variables and was calculated by the following formula: (12). IVs with F<10 were excluded. Then, the Phenoscanner database (http://www.phenoscanner.medschl.cam.ac.uk/) was used to screen out IVs that could potentially be associated with confounding factors or strongly correlated with outcomes. The confounding factors included in this study referred to the possible pathogenic factors, which mainly encompassed mechanical stress, infections and psoriasis (2). The degree of heterogeneity was assessed using Cochran’s Q statistic. To detect horizontal pleiotropy within inferred causal relationships from MR analysis, the MR-PRESSO test and MR Egger were employed. The outcome from the inverse-variance weighted (IVW) regression model was adopted as the primary result, with significance set at P-value< 0.05. The leave-one-out analysis was performed to illustrate the individual impact of each SNP on the overall outcomes. R packages “TwoSampleMR” (https://mrcieu.github.io/TwoSampleMR/) and “MR-PRESSO” (https://github.com/rondolab/MR-PRESSO) were applied for MR analysis (13, 14).
Gene expressions were obtained from Gene Expression Omnibus (GEO) database (http://www.ncbi.nlm.nih.gov/geo/) under the accession number GSE94648 (15). The “Lmfit” function within the “limma” package (http://bioconductor.org/packages/release/bioc/html/limma.html) was used to fit linear model for each gene in the given array series (16). The function “contrasts.fit” was used to calculate estimated coefficients and standard errors. Genes with adjusted P-values< 0.05 were identified as differentially expressed genes (DEGs) in IBD. The corresponding expression quantitative trait locus (eQTLs) were obtained from eQTLGen database (https://eqtlgen.org). Each eQTL was treated as an exposure, and a rigorously defined AS GWAS dataset (finn-b-M13_ANKYLOSPON_STRICT) was selected as the primary outcome, while another dataset, ukb-a-88, was chosen as the validation outcome. The criteria for IVs included meeting the threshold of P< 5x10^-8 and r = 0.001. If only a single SNP was available, the Wald ratio was utilized as the primary result. With a binary outcome and a dichotomous IV, log risk ratio estimate (dichotomous IV) = , where is commonly the log of the probability of an event, or the log odds of an event, in genetic subgroup j (17). In cases of multiple SNPs, the IVW regression model was adopted as the primary result. The was estimated by (17).
Significance in the main result was determined by a P-value< 0.05. Considering that this study focused on changes in general rather than specific genes, the original P-value rather than the adjusted P-value was chosen as a screening criterion to avoid excessive false negative rates. The remaining procedures of MR analysis were consistent with those detailed in the previous section. Genes corresponding to eQTLs derived from MR analysis were identified as key genes within IBD contributing to the development of AS. The protein quantitative trait loci (pQTL) data were derived from a large scale studies on circulating plasma proteins and genetic associations from deCODE, which provided data of 4,907 proteins (18). The causal relationship between protein expression and AS risk was further verified by MR analysis with pQTL data. The parameters of pQTL analysis were consistent with those of eQTL.
Genes were categorized based on the odds ratio (OR) determined by MR analysis. Those with an OR greater than 1 were designated as risk genes, while those with an OR less than 1 were categorized as protective genes. Subsequently, utilizing the lists of risk and protective genes, the enrichment score was computed through single-sample gene set enrichment analysis (ssGSEA) to generate the risk and protective scores (19). The ssGSEA method started by rank-normalizing all the genes in a given sample in order of the expression, from largest to smallest. Enrichment scores were then generated based on the Empirical Cumulative Distribution Functions (ECDF) of the given gene set (in this study, the risk gene set of AS) and the remaining genes. The enrichment score was obtained by an integration of the difference between the ECDFs. This algorithm was executed using the R package “GSVA” (http://bioconductor.org/packages/release/bioc/html/GSVA.html). The comparison of scores between the two groups was conducted employing the Wilcoxon test.
The R package “RTN” was imported to analyze the transcription factors (TFs) in risk genes (20). This package offers a systematic approach to establish Transcriptional Network Inference (TNI) through a sequence of three steps: (i) computing mutual information between a regulator and all potential targets, and removing non-significant associations by permutation analysis; (ii) removing unstable interactions by bootstrapping; and (iii) applying the algorithm for the reconstruction of accurate cellular networks. The set of genes controlled by a given TF forms a regulon. The activity score of regulon corresponding to each transcription factor was determined using TNI and presented in a heatmap. One-tailed gene set enrichment analysis was utilized to ascertain the potential association of the regulon with a specific phenotype. In parallel, a two-tailed GSEA was employed to investigate whether the regulon demonstrates either a positive or negative correlation with the phenotype.
Gene set enrichment analysis (GSEA) was used to examine and clarify coordinated alterations at the pathway level across distinct phenotypes (21). The analysis centered on discerning pathway variations between active patients and inactive patients.
The “xCell” package (https://github.com/dviraran/xCell), built on a spillover compensation technique, offered methodologies to predict 64 immune and stromal cell types with precision (22). This approach involved input of a gene expression dataset (such as FPKM or TPM) normalized to gene length. The ssGSEA scores were calculated for each of the 489 gene signatures and the scores of all signatures corresponding to a cell type were then averaged. Then the spillover compensation was performed for each row using linear least squares. All the values were then combined to create the final xCell score. Using this package, enrichment scores for 34 distinct immune cell types were computed. Univariate regression analysis and least absolute shrinkage and selection operator (LASSO) regression analysis were conducted on these 34 immune cell types, aiming to identify those most strongly linked to disease activity. The “cor” function within the “stats” package was employed to assess the correlation between risk genes and immune cell populations.
The IBD datasets “ieu-a-31”, “ebi-a-GCST004131”, and “finn-b-K11_IBD_STRICT”, along with the AS datasets “ukb-a-88” and “finn-b-M13_ANKYLOSPON_STRICT” were utilized for MR analysis (23–25). The IBD datasets encompassed 308,662 samples, including 41,677 patients, while the AS dataset comprised 555,189 samples, with 1,567 being patients. A total of 6 MR analyses were conducted across 3 exposure datasets and 2 outcome datasets. All analyses underwent scrutiny via the MR-PRESSO test, MR-EGGER test, and Cochran’s Q test, consistently showing no evidence of pleiotropy or heterogeneity. The results of the 6 MR analyses, based on datasets from various consortiums, yielded significant outcomes. While these results indicated variations in ORs stemming from diverse exposures and outcomes, all ORs were greater than 1, supporting the notion of IBD as a risk factor for AS. Table 1 and Figure 2 showed the main results of the 6 sets of MR analyses. More details were provided in the Supplementary Materials.
Figure 2 Mendelian randomization analyses between IBD and AS (A) exposure:ieu-a-31; outcome: ukb-a-88 (B) exposure: ieu-a-31; outcome: finn-b-M13_ANKYLOSPON_STRICT (C) exposure: ebi-a-GCST004131; outcome: ukb-a-88 (D) exposure: ebi-a-GCST004131; outcome: finn-b-M13_ANKYLOSPON_STRICT (E) exposure: finn-b-K11_IBD_STRICT; outcome: ukb-a-88 (F) exposure: finn-b-K11_IBD_STRICT; outcome: finn-b-M13_ANKYLOSPON_STRICT.
The analysis revealed 2761 significant DEGs between IBD and healthy controls. In the analysis of primary outcomes, 140 genes were identified to be involved in the development of AS. Of these 140 key genes, 66 exhibited OR values exceeding 1, classifying them as risk genes, while 74 displayed OR values below 1, denoting protective genes. However, not all risk genes were up-regulated in IBD. In fact, only 30 risk genes demonstrated up-regulation, and 44 protective genes exhibited down-regulation within the study. In the analysis of validation outcome, 112 genes were identified as contributors to AS development, with 54 being risk genes and 58 protective genes. Intriguingly, only five risk genes (TINF2, IMMT, ZNF408, NEU1, and APOM) and three protective genes (ANKRD13C, KDELR2, and ZKSCAN8) held significance in both analyses. Figure 3 showed the risk and protective effects of key DGEs with |logFC| > 0.3. The OR, P-values, LogFC and other information for these genes were provided in the Supplementary Materials.
Figure 3 Risk and protective effects of key DGEs with |logFC| > 0.3 (A) The key DEGs of outcome ukb-a-88 (B) The key DEGs of outcome finn-b-M13_ANKYLOSPON_STRICT.
As for the protein levels, due to limited availability of pQTL data sources, verification was only conducted for a subset of the risk genes. In the primary outcome analysis, proteomic data were accessible for 19 out of the 66 risk genes. Among these, APOM and TP53I11 exhibited a positive causal association with AS at the protein level. For the validation outcome analysis, pQTL data were found for 16 out of the 54 risk genes. Among these genes, only DYNLL2 demonstrated positive results on protein levels that aligned with eQTL analysis findings. However, considering the limitations of MR Analysis, the possibility that these proteins are involved in the pathogenesis of AS cannot be completely ruled out even in the absence of positive results. Detailed results of MR Analysis of pQTL were provided in Supplementary Materials.
Given the occurrence of both up-regulation and down-regulation among risk and protective genes, and considering the limited number of genes that were significant in both outcomes, the study then shifted its focus towards comprehending the overall regulatory patterns of these genes within active and inactive patients. The set of 140 pivotal genes were categorized as 66 risk genes and 74 protective genes based on their respective OR values. Subsequently, individual ssGSEA scores were calculated to examine the enrichment profiles of these two gene categories across distinct phenotypic samples. Likewise the 112 genes assessed in the validation outcome analysis were categorized as 54 risk genes and 58 protective genes, and their corresponding scores were calculated.
Notably, across both outcome analyses, active patients consistently exhibited significantly higher risk scores compared to their inactive counterparts (as depicted in Figure 4). However, inconsistencies were observed in the protective scores between the two outcome analyses. These findings suggested that alterations in risk genes might hold greater significance than those in protective genes in the process of how IBD leading to AS. Moreover, the results indicated that active patients might face an elevated risk compared to inactive patients, emphasizing the clinical implications of disease activity.
Figure 4 Risk scores between active and inactive patients (A) Risk scores in outcome ukb-a-88 (B) Risk scores in outcome finn-b-M13_ANKYLOSPON_STRICT.
Among the 66 risk genes of the primary outcome, 9 TFs were identified, while 7 TFs were identified among the 54 risk genes of the validation outcome, culminating in a total of 15 distinct nonredundant risk TFs. Through one-tailed gene set enrichment analysis of these transcription factors, it became evident that 9 out of the 15 risk TFs exhibited significant disparities in their regulatory networks between active and inactive patients (as illustrated in the Figure 5B).
Figure 5 Transcription factor analyses (A) Clustering heat map of risk transcription factor activity (B) TFs with significant disparities in their regulatory networks between active and inactive patients (one-tailed GSEA) (C) Two-tailed GSEA of TFs with significant disparities between active and inactive patients.
Expanding on this, a two-tailed GSEA was performed on the 9 transcription factors that were identified as significant in the previous analysis. The results revealed a divergence in the effects of these transcription factors. Specifically, genes positively correlated with TRIM23, ZNF460, POGZ, PLSCR1, and BBX were activated, while genes negatively linked to them were suppressed in active patients compared to inactive patients. Conversely, the remaining four genes—TULP3, IKZF2, SPIB and DIP2C—exhibited contrasting changes in expression patterns (as shown in the Figure 5C).
Based on the clustering analysis of transcription factor activity, a noticeable pattern emerged in which active and inactive patients were clearly separated, as illustrated in Figure 5A. Analyses of risk TFs hinted at potential disparities between active and inactive IBD patients in the progression leading to AS.
According to the results of GSEA analysis, this investigation directed its attention towards three inflammation-associated signaling pathways that demonstrated substantial discrepancies between active and inactive patients. These pathways included the “Nod-like receptor signaling pathway”, “neutrophil extracellular trap (NET) formation,” and “TNF signaling pathway”, all of which exhibited significant activation in active patients compared to their inactive counterparts (as shown in the Figure 6A). The combination of these GSEA findings pointed towards a profound interplay between the disease activity of IBD and the heightened activation of inflammatory pathways, potentially exerting an influence on the pathogenesis of AS.
Figure 6 GSEA and Immune cell analyses (A) GSEA of “Nod-like receptor signaling pathway,” “neutrophil extracellular trap (NET) formation,” and “TNF signaling pathway,” between active and inactive patients active and inactive patients (B) LASSO regression screens key immune cells (C) Correlation coefficients between key immune cells and risk genes (left: ukb-a-88; right: finn-b-M13_ANKYLOSPON_STRICT).
Enrichment scores were computed for 34 immune cell types, and subsequently univariate regression analysis was conducted. The results highlighted a total of 14 immune cell types exhibiting significant correlations with disease activity. Out of these 14 cell types, a more comprehensive analysis was performed using LASSO regression (as shown in the Figure 6B), resulting in the identification of the 7 most pertinent immune cell types. These included CD4+ naive T-cells, CD4+ Tcm, CD8+ T-cells, Mast cells, Memory B-cells, Th1 cells, and Th2 cells.
Further analysis delved into the association between these seven immune cell types and risk genes, revealing that 63 out of the 66 risk genes exhibited significant correlations with at least one immune cell type. Among these, 39 genes displayed significant associations with CD8+ T-cells, followed by 31 genes with Th1 cells, and 30 genes with CD4+ naive T-cells and CD4+ Tcms each. Memory B-cells were associated with 28 genes, while Mast cells and Th2 cells were linked with 9 and 19 genes, respectively. These findings were consistent in the validation data as well. Out of the 54 risk genes analyzed, 52 were significantly associated with at least one immune cell type. Specifically, CD8+ T-cells were associated with 32 genes, CD4+ naive T-cells with 29 genes, Th1 cells with 27 genes, CD4+ Tcms with 25 genes, memory B-cells with 24 genes, and mast cells with 7 genes. The correlation coefficients between these genes and immune cells were depicted in the Figure 6C. Detailed P-values were available in the Supplementary Materials.
The analyses of immune cells not only unveiled discrepancies between active and inactive patients but also underscored the close relationship between these immune cell types and risk genes, indicating their potential involvement in the pathogenesis of AS.
This study addressed two pivotal clinical inquiries: does IBD directly lead to the development of AS, and does the activity of IBD exert an influence in this context? Previous studies have not provided sufficient answers due to multiple factors. On one hand, the challenge arose from the inherent difficulty in establishing causal relationships within previous study designs. On the other hand, the intermittent nature of IBD flares and the insidious onset of AS, making it challenging to precisely determine the onset of disease, further complicated the demonstration of the impact of IBD activity on the development of AS.
This study forged a novel approach, the conjunction of Mendelian randomization analysis and transcriptomic data, thereby establishing a linkage between the genetic level and macroscopic phenotypic interactions. This endeavor not only unveiled IBD as an influencing factor in the development of AS but also provided evidence suggesting that active IBD might have an increased potential to precipitate the onset of AS.
Six sets of Mendelian randomization analyses provided relatively robust evidence for IBD being a risk factor for AS. The shared underlying mechanisms of IBD and AS may help elucidate why individuals with IBD are more susceptible to developing AS. Certain genes related to these diseases overlap, with IL23R being a notable example. However, IL23A is associated with IBD but not AS (26, 27). “Gut-synovial axis” hypothesis, implicating environmental and host factors, was an attempt to establish a connection between the intestine and the joint (28). Intestinal dysbiosis and the disruption of epithelial barrier could trigger the activation of innate cells, innate-like cells and specific subsets of T cells, contributing to the pathogenesis of spondyloarthritis (29). Furthermore, immune cell migration initiated in the gut and directed towards other locations was observed in patients with AS. CX3CR1+CD59+ mononuclear phagocytes and type 3 innate lymphoid cells (ILC3s) expanded not only within inflamed gut tissue but also in the peripheral blood, synovial fluid, and bone marrow of patients with AS. The presence of CCR9 in non-intestinal CX3CR1+CD59+ cells and the expression of the α4β7 integrin in ILC3s probably indicate their intestinal origin (30, 31).
The analysis of pathogenic genes presented vastly different landscapes depending on the outcome chosen. Only 8 genes showed consistent results across the analyses of the two outcomes, which could be attributed to the differences in population samples encompassed by the distinct outcomes. Among the five common risk genes, TINF2 is one of six components of the telomere protein complex, also known as shelterin (32). It participates in multiple protein-protein interactions crucial for maintaining the protective function of shelterin at the telomeric ends (33). IMMT constitutes a fundamental element of the mitochondrial contact site complex, which governs the stability of mitochondrial cristae (34). ZNF408 encodes a transcription factor equipped with 10 predicted C2H2-type zinc fingers, believed to be involved in DNA binding (35) and its mutation showed a great impact on retinal vascular development (36, 37). NEU1, a neurophysin, specifically binds to oxytocin and functions as a carrier protein facilitating the transport of hormones from the hypothalamus, where they are synthesized, to the storage site in the pituitary nerve lobe (38). APOM, a prominent member of the apolipoprotein family, serves as a principal carrier of sphingosine-1-phosphate (39). However, APOM’s role extends beyond metabolic and cardiovascular disorders, encompassing contributions to autoimmunity and inflammation (40–42). Among the three shared down-regulated protective genes, ANKRD13C operates as a molecular chaperone for G protein-coupled receptors, overseeing their biogenesis and exit from the endoplasmic reticulum (43). KDELR2, located on the Golgi apparatus, can bind to the K-D-E-L sequence motif and prevent secretion of soluble endoplasmic reticulum-resident proteins (44). ZKSCAN8 encodes a zinc finger protein with KRAB and SCAN domains with little experimental study of its functions. These eight genes exhibit diverse localization and functions, and most importantly, their exploration in IBD and AS remains largely unexplored.
The differences in results of pathogenic genes arising from the selection of distinct outcome data, coupled with the scarcity of research on shared pathogenic genes in IBD and AS, hinted at the need for caution when attributing disease causality to a single gene. Therefore, this study shifted its focus to investigating the broader expression patterns. Through ssGSEA analysis, it was revealed that despite differing sets of risk genes, the overall risk scores for active patients were significantly higher than those for inactive patients. Further analysis of risk transcription factors also supported the distinctions between active and inactive patients.
Subsequently, a question arose: which pathways and immune cell types exhibited significant differences between active and inactive patients? The GSEA analysis presented compelling findings, demonstrating that three crucial inflammatory pathways were significantly activated in active patients, whereas they were not pronounced in inactive patients. The NOD-like receptor (NLR) family of proteins comprises a group of pattern recognition receptors recognized for their role in initiating the initial innate immune response to cellular injury and stress. Several NLRs participate in the formation of inflammasomes (45), with NLRP3 being the most extensively studied among them. NLRP3 and IL-1β were found to be up-regulated in active UC and CD patients compared to those in a quiescent state (46). In addition, the NLRP3 inflammasome was activated in CD patients and in UC patients with long-standing disease (47). However, in animal studies, NLRP3 has demonstrated a bidirectional effect, both promoting inflammation and safeguarding gut integrity. On one hand, NEK7 interacted with NLRP3 to regulate NLRP3 inflammasome activation, thereby modulating the pyroptosis in MODE-K cells and DSS-induced chronic colitis in mice (48). Conversely, Nlrp3(-/-) mice displayed heightened susceptibility to experimental colitis, underscoring its vital role in maintaining intestinal homeostasis (49). There were also treatments that work by inhibiting the NLRP3 inflammasomes (50, 51). Apart from NLRP3, NLRP6 and NLRP1 inflammasomes were also reported to contribute to IBD pathogenesis (52, 53). Meanwhile, an increase of NLRP3 inflammasomes and NLRP3 inflammasome-derived proinflammatory cytokines was observed in peripheral blood mononuclear cells of patients with AS (54). Remarkably, the inflammasome activation in AS was reported to be associated with gut dysbiosis (55), suggesting the potential impact of IBD on AS.
The role of the TNF pathway in both IBD and AS is undeniable, as the remarkable success of anti-TNF therapy serves as compelling evidence that TNF is a pivotal inflammatory factor in both diseases. This also underscores the association between TNF and disease activity in IBD, aligning with the observations of this study.
NETs are intricate extracellular structures, resembling web-like formations, composed of cytosolic and granule proteins assembled on a framework of decondensed chromatin. NETs serve a dual role, offering protection against various pathogens such as bacteria, fungi, viruses, and parasites, while also contributing to the development of immune-related disorders (56). The active involvement of NETs in IBD has become increasingly evident, indicating their capability to exacerbate inflammatory responses and impair intestinal barrier (57–59). Numerous studies substantiated a positive correlation between NET abundance and active disease, with density linked to histopathological severity in IBD cases (58–61). Moreover, several therapeutic approaches exhibited efficacy in ameliorating IBD by targeting NETs (62, 63). As for AS, it was reported that neutrophils from AS patients exhibited heightened formation of NETs containing bioactive IL-17A (64). Elevated IL-17 levels was observed in AS patients, with serum concentrations correlating with disease activity (65, 66). Furthermore, anti-IL-17 therapy has demonstrated significant effectiveness in AS treatment (67). This suggests the possibility that NETs contribute to AS pathogenesis through IL-17, although other potential mechanisms remain inadequately explored.
The study revealed a captivating pattern, with significantly heightened activation of inflammatory pathways in active IBD patients as compared to inactive ones. Considering that AS is also characterized by chronic recurring autoinflammation and is believed to be triggered by infections, this raises a conjecture that the distinct inflammation arising from IBD, separate from infections, may potentially serve as a factor in initiating or exacerbating AS.
The analysis of immune cells revealed that CD8+ T cells and CD4+ T cells (including CD4+ naive T-cells, Th1 cells, and CD4+ Tcms) were likely to be significant contributors, as they exhibited a strong correlation with disease activity. While the role of CD8+ T cells in IBD was controversial, possibly stemming from variations in sources and subsets, it is suggested that activated cytotoxic CD8+ T cells could initiate and contribute to the progression of IBD (68). On the other hand, CD4+ naive T cells can be induced to differentiate into various subpopulations, with Th1 being particularly relevant to this study. Th1 cells release TNF-α and IFN-γ, triggering responses in other immune cells and fostering inflammation. Excessive Th1 responses were observed in IBD patients, with both UC and CD showcasing activated effector Th1 cells (69, 70).
Moreover, CD8+T cells and CD4+T cells are also involved in the pathogenesis of AS. It has been reported that human leukocyte antigen B*27 (HLA-B27), a crucial marker for AS, plays a role in presenting pathogenic peptides to CD8+ T cells (71). Additionally, the autoreactivity of CD8(+) T cells towards HLA-B27-restricted self-epitopes was reported to be associated with AS (72). Comparative data from serum and synovial fluid suggested that cytotoxic phenotype CD8+ T cells are recruited to the joints where they exhibit an activated phenotype (73). A meta-analysis revealed significant increases in the proportions of CD4+ T cells as well as Th1/Th2 ratio in AS patients (74). Furthermore, Th1 cells were found to respond more frequently to conserved E. coli proteins in peripheral blood mononuclear cells and synovial fluid mononuclear cells in AS patients compared to RA patients (75). Additionally, our findings also highlighted a significant association between risk genes and CD8+ T cells as well as CD4+ T cells, thereby suggesting their potential pathogenic role.
In this study, we observed a higher score of AS risk genes in active IBD patients compared to non-active patients, indicating the potential association between disease activity of IBD and the development of AS. Moreover, the clustering analysis revealed that these critical genes primarily contributed to inflammatory pathways, suggesting the potential therapeutic benefits of managing inflammation in individuals with IBD to prevent the onset of AS.
The main constraint of this study lay in the limited data sample source, which introduced potential biases that were challenging to precisely estimate. In addition, the application process of MR analysis also has limitations. The selection of appropriate genetic variation as an IV is crucial to the results of Mendelian randomization analysis, and genetic variants may have horizontal pleiotropy, leading to misjudgments about causation. Additionally, MR Analysis relies on certain assumptions such as strong correlation and independence, which if not met can result in misestimates. To mitigate these potential risks, this study utilized three distinct IBD GWAS datasets as exposure and two separate AS GWAS datasets as outcomes to enhance outcome reliability. IVs that might be associated with confounders or strongly associated with results were also carefully screened out. Furthermore, pleiotropy and heterogeneity were tested by multiple methods. However, despite these efforts, the possibility of errors cannot be entirely dismissed.
In conclusion, this study, through the integration of Mendelian randomization and transcriptome analysis, not only suggested IBD as a potential risk factor for AS but also employed AS-associated risk genes as a bridge, providing novel evidence for the influence of IBD activity on the development of AS.
All data are publicly available. Detailed information for these datasets is summarized in Supplementary Material.
This study was approved by the ethics board of Yueyang Hospital of Integrated Traditional Chinese and Western Medicine. All methods were performed in accordance with the relevant guidelines and regulations.
YD: Data curation, Formal analysis, Methodology, Writing – original draft. JC: Methodology, Writing – review & editing. RL: Validation, Writing – review & editing. LX: Funding acquisition, Supervision, Validation, Writing – review & editing.
The author(s) declare financial support was received for the research, authorship, and/or publication of this article. This work was supported by the National Natural Science Foundation of China (Award Number: 82374318).
We want to acknowledge the participants and investigators of the FinnGen study, Neale Lab and deCODE for data sharing. We also appreciate the data shared by Jimmy Z Liu et al, Katrina M de Lange et al. and Núria Planell et al. In addition, want to acknowledge GEO database and openGWAS database for providing data sharing platform.
The authors declare that the research was conducted in the absence of any commercial or financial relationships that could be construed as a potential conflict of interest.
All claims expressed in this article are solely those of the authors and do not necessarily represent those of their affiliated organizations, or those of the publisher, the editors and the reviewers. Any product that may be evaluated in this article, or claim that may be made by its manufacturer, is not guaranteed or endorsed by the publisher.
The Supplementary Material for this article can be found online at: https://www.frontiersin.org/articles/10.3389/fimmu.2024.1289049/full#supplementary-material
Additional File 1 | Table S1 Datasets Information (.doc).
Additional File 2 | Detailed information of MR analysis between IBD and AS (.pdf).
Additional File 3 | Detailed information of key DEGs (.pdf).
Additional File 4 | P-values of the correlation analysis between risk gene and immune cell types (.pdf).
Additional File 5 | Detailed information of MR analysis of pQTL (.pdf).
IBD, inflammatory bowel disease; AS, ankylosing spondylitis; MR, Mendelian randomization; eQTL, expression quantitative trait loci; pQTL, protein quantitative trait loci; GWAS, genome-wide association studies; IV, instrumental variable; IVW, inverse-variance weighted; DEG, differentially expressed genes; OR, odds ratio; GSEA, gene set enrichment analysis; ssGSEA, single-sample gene set enrichment analysis; TF, transcription factor; LASSO, least absolute shrinkage and selection operator; NET, neutrophil extracellular trap; ILC3, type 3 innate lymphoid cell; NLR, NOD-like receptor; UC, ulcerative colitis; CD, Crohn’s disease; HLA-B27, human leukocyte antigen B*27.
1. Núñez FP, Krugliak CN, Quera R, Rubin DT. Evolving role of endoscopy in inflammatory bowel disease: going beyond diagnosis. World J Gastroenterol. (2021) 27:2521–30. doi: 10.3748/wjg.v27.i20.2521
2. Sieper J, Poddubnyy D. Axial spondyloarthritis. Lancet. (2017) 390:73–84. doi: 10.1016/S0140-6736(16)31591-4
3. Dougados M, Baeten D. Spondyloarthritis. Lancet. (2011) 377:2127–37. doi: 10.1016/S0140-6736(11)60071-8
4. Khor B, Gardet A, Xavier RJ. Genetics and pathogenesis of inflammatory bowel disease. Nature. (2011) 474:307–17. doi: 10.1038/nature10209
5. Greuter T, Vavricka SR. Extraintestinal manifestations in inflammatory bowel disease - epidemiology, genetics, and pathogenesis. Expert Rev Gastroenterol Hepatol. (2019) 13:307–17. doi: 10.1080/17474124.2019.1574569
6. Ahmed W, Galati J, Kumar A, Christos PJ, Longman R, Lukin DJ, et al. Dual biologic or small molecule therapy for treatment of inflammatory bowel disease: a systematic review and meta-analysis. Clin Gastroenterol Hepatol. (2022) 20:e361–79. doi: 10.1016/j.cgh.2021.03.034
7. MaChado MA, Barbosa MM, Almeida AM, de Araújo VE, Kakehasi AM, Andrade EI, et al. Treatment of ankylosing spondylitis with tnf blockers: a meta-analysis. Rheumatol Int. (2013) 33:2199–213. doi: 10.1007/s00296-013-2772-6
8. Karreman MC, Luime JJ, Hazes J, Weel A. The prevalence and incidence of axial and peripheral spondyloarthritis in inflammatory bowel disease: a systematic review and meta-analysis. J Crohns Colitis. (2017) 11:631–42. doi: 10.1093/ecco-jcc/jjw199
9. Wang R, Ward MM. Epidemiology of axial spondyloarthritis: an update. Curr Opin Rheumatol. (2018) 30:137–43. doi: 10.1097/BOR.0000000000000475
10. Davies NM, Holmes MV, Davey SG. Reading mendelian randomisation studies: a guide, glossary, and checklist for clinicians. Bmj. (2018) 362:k601. doi: 10.1136/bmj.k601
11. Shi X, Wei T, Hu Y, Wang M, Tang Y. The associations between plasma soluble trem1 and neurological diseases: a mendelian randomization study. J Neuroinflamm. (2022) 19:218. doi: 10.1186/s12974-022-02582-z
12. Burgess S, Thompson SG. Mendelian randomization: methods for using genetic variants in causal estimation. Boca Raton, FL, USA: CRC Press (2021).
13. Hemani G, Zheng J, Elsworth B, Wade KH, Haberland V, Baird D, et al. The mr-base platform supports systematic causal inference across the human phenome. Elife. (2018) 7:e34408. doi: 10.7554/eLife.34408
14. Verbanck M, Chen CY, Neale B, Do R. Detection of widespread horizontal pleiotropy in causal relationships inferred from mendelian randomization between complex traits and diseases. Nat Genet. (2018) 50:693–8. doi: 10.1038/s41588-018-0099-7
15. Planell N, Masamunt MC, Leal RF, Rodríguez L, Esteller M, Lozano JJ, et al. Usefulness of transcriptional blood biomarkers as a non-invasive surrogate marker of mucosal healing and endoscopic response in ulcerative colitis. J Crohns Colitis. (2017) 11:1335–46. doi: 10.1093/ecco-jcc/jjx091
16. Law CW, Chen Y, Shi W, Smyth GK. Voom: precision weights unlock linear model analysis tools for rna-seq read counts. Genome Biol. (2014) 15:R29. doi: 10.1186/gb-2014-15-2-r29
17. Stephen B, TS G. Mendelian randomization : methods for causal inference using genetic variants. Boca Raton, FL, USA: Chapman & Hall/CRC (2021).
18. Ferkingstad E, Sulem P, Atlason BA, Sveinbjornsson G, Magnusson MI, Styrmisdottir EL, et al. Large-scale integration of the plasma proteome with genetics and disease. Nat Genet. (2021) 53:1712–21. doi: 10.1038/s41588-021-00978-w
19. Barbie DA, Tamayo P, Boehm JS, Kim SY, Moody SE, Dunn IF, et al. Systematic rna interference reveals that oncogenic kras-driven cancers require tbk1. Nature. (2009) 462:108–12. doi: 10.1038/nature08460
20. Fletcher MN, Castro MA, Wang X, de Santiago I, O'Reilly M, Chin SF, et al. Master regulators of fgfr2 signalling and breast cancer risk. Nat Commun. (2013) 4:2464. doi: 10.1038/ncomms3464
21. Subramanian A, Tamayo P, Mootha VK, Mukherjee S, Ebert BL, Gillette MA, et al. Gene set enrichment analysis: a knowledge-based approach for interpreting genome-wide expression profiles. Proc Natl Acad Sci U.S.A. (2005) 102:15545–50. doi: 10.1073/pnas.0506580102
22. Aran D, Hu Z, Butte AJ. Xcell: digitally portraying the tissue cellular heterogeneity landscape. Genome Biol. (2017) 18:220. doi: 10.1186/s13059-017-1349-1
23. Liu JZ, van Sommeren S, Huang H, Ng SC, Alberts R, Takahashi A, et al. Association analyses identify 38 susceptibility loci for inflammatory bowel disease and highlight shared genetic risk across populations. Nat Genet. (2015) 47:979–86. doi: 10.1038/ng.3359
24. de Lange KM, Moutsianas L, Lee JC, Lamb CA, Luo Y, Kennedy NA, et al. Genome-wide association study implicates immune activation of multiple integrin genes in inflammatory bowel disease. Nat Genet. (2017) 49:256–61. doi: 10.1038/ng.3760
25. Kurki MI, Karjalainen J, Palta P, Sipilä TP, Kristiansson K, Donner KM, et al. Finngen provides genetic insights from a well-phenotyped isolated population. Nature. (2023) 613:508–18. doi: 10.1038/s41586-022-05473-8
26. Rahman P, Inman RD, Gladman DD, Reeve JP, Peddle L, Maksymowych WP. Association of interleukin-23 receptor variants with ankylosing spondylitis. Arthritis Rheum. (2008) 58:1020–5. doi: 10.1002/art.23389
27. Evans DM, Spencer CC, Pointon JJ, Su Z, Harvey D, Kochan G, et al. Interaction between erap1 and hla-b27 in ankylosing spondylitis implicates peptide handling in the mechanism for hla-b27 in disease susceptibility. Nat Genet. (2011) 43:761–7. doi: 10.1038/ng.873
28. Fragoulis GE, Liava C, Daoussis D, Akriviadis E, Garyfallos A, Dimitroulas T. Inflammatory bowel diseases and spondyloarthropathies: from pathogenesis to treatment. World J Gastroenterol. (2019) 25:2162–76. doi: 10.3748/wjg.v25.i18.2162
29. Mauro D, Thomas R, Guggino G, Lories R, Brown MA, Ciccia F. Ankylosing spondylitis: an autoimmune or autoinflammatory disease? Nat Rev Rheumatol. (2021) 17:387–404. doi: 10.1038/s41584-021-00625-y
30. Ciccia F, Guggino G, Zeng M, Thomas R, Ranganathan V, Rahman A, et al. Proinflammatory cx3cr1+cd59+tumor necrosis factor-like molecule 1a+interleukin-23+ monocytes are expanded in patients with ankylosing spondylitis and modulate innate lymphoid cell 3 immune functions. Arthritis Rheumatol. (2018) 70:2003–13. doi: 10.1002/art.40582
31. Ciccia F, Guggino G, Rizzo A, Saieva L, Peralta S, Giardina A, et al. Type 3 innate lymphoid cells producing il-17 and il-22 are expanded in the gut, in the peripheral blood, synovial fluid and bone marrow of patients with ankylosing spondylitis. Ann Rheum Dis. (2015) 74:1739–47. doi: 10.1136/annrheumdis-2014-206323
32. O'Connor MS, Safari A, Xin H, Liu D, Songyang Z. A critical role for tpp1 and tin2 interaction in high-order telomeric complex assembly. Proc Natl Acad Sci U.S.A. (2006) 103:11874–9. doi: 10.1073/pnas.0605303103
33. Smith EM, Pendlebury DF, Nandakumar J. Structural biology of telomeres and telomerase. Cell Mol Life Sci. (2020) 77:61–79. doi: 10.1007/s00018-019-03369-x
34. Ott C, Dorsch E, Fraunholz M, Straub S, Kozjak-Pavlovic V. Detailed analysis of the human mitochondrial contact site complex indicate a hierarchy of subunits. PloS One. (2015) 10:e0120213. doi: 10.1371/journal.pone.0120213
35. Avila-Fernandez A, Perez-Carro R, Corton M, Lopez-Molina MI, Campello L, Garanto A, et al. Whole-exome sequencing reveals znf408 as a new gene associated with autosomal recessive retinitis pigmentosa with vitreal alterations. Hum Mol Genet. (2015) 24:4037–48. doi: 10.1093/hmg/ddv140
36. Collin RW, Nikopoulos K, Dona M, Gilissen C, Hoischen A, Boonstra FN, et al. Znf408 is mutated in familial exudative vitreoretinopathy and is crucial for the development of zebrafish retinal vasculature. Proc Natl Acad Sci U.S.A. (2013) 110:9856–61. doi: 10.1073/pnas.1220864110
37. Karjosukarso DW, Ali Z, Peters TA, Zhang J, Hoogendoorn A, Garanto A, et al. Modeling znf408-associated fevr in zebrafish results in abnormal retinal vasculature. Invest Ophthalmol Vis Sci. (2020) 61:39. doi: 10.1167/iovs.61.2.39
38. Pickering BT, Jones CW, Burford GD, Mcpherson M, Swann RW, Heap PF, et al. The role of neurophysin proteins: suggestions from the study of their transport and turnover. Ann N Y Acad Sci. (1975) 248:15–35. doi: 10.1111/j.1749-6632.1975.tb34174.x
39. Chen Z, Hu M. The apom-s1p axis in hepatic diseases. Clin Chim Acta. (2020) 511:235–42. doi: 10.1016/j.cca.2020.10.023
40. Shi Y, Lam SM, Liu H, Luo G, Zhang J, Yao S, et al. Comprehensive lipidomics in apom(-/-) mice reveals an overall state of metabolic distress and attenuated hepatic lipid secretion into the circulation. J Genet Genomics. (2020) 47:523–34. doi: 10.1016/j.jgg.2020.08.003
41. Del GI, Rubinelli L, Sasset L, Wadsack C, Hla T, Di Lorenzo A. Endothelial spns2 and apom regulation of vascular tone and hypertension via sphingosine-1-phosphate. J Am Heart Assoc. (2021) 10:e021261. doi: 10.1161/JAHA.121.021261
42. Tsai HC, Han MH. Sphingosine-1-phosphate (s1p) and s1p signaling pathway: therapeutic targets in autoimmunity and inflammation. Drugs. (2016) 76:1067–79. doi: 10.1007/s40265-016-0603-2
43. Parent A, Roy SJ, Iorio-Morin C, Lépine MC, Labrecque P, Gallant MA, et al. Ankrd13c acts as a molecular chaperone for g protein-coupled receptors. J Biol Chem. (2010) 285:40838–51. doi: 10.1074/jbc.M110.142257
44. Raykhel I, Alanen H, Salo K, Jurvansuu J, Nguyen VD, Latva-Ranta M, et al. A molecular specificity code for the three mammalian kdel receptors. J Cell Biol. (2007) 179:1193–204. doi: 10.1083/jcb.200705180
45. Platnich JM, Muruve DA. Nod-like receptors and inflammasomes: a review of their canonical and non-canonical signaling pathways. Arch Biochem Biophys. (2019) 670:4–14. doi: 10.1016/j.abb.2019.02.008
46. Ranson N, Veldhuis M, Mitchell B, Fanning S, Cook AL, Kunde D, et al. Nlrp3-dependent and -independent processing of interleukin (il)-1β in active ulcerative colitis. Int J Mol Sci. (2018) 20(1):57. doi: 10.3390/ijms20010057
47. Lazaridis LD, Pistiki A, Giamarellos-Bourboulis EJ, Georgitsi M, Damoraki G, Polymeros D, et al. Activation of nlrp3 inflammasome in inflammatory bowel disease: differences between crohn's disease and ulcerative colitis. Dig Dis Sci. (2017) 62:2348–56. doi: 10.1007/s10620-017-4609-8
48. Chen X, Liu G, Yuan Y, Wu G, Wang S, Yuan L. Nek7 interacts with nlrp3 to modulate the pyroptosis in inflammatory bowel disease via nf-κb signaling. Cell Death Dis. (2019) 10:906. doi: 10.1038/s41419-019-2157-1
49. Hirota SA, Ng J, Lueng A, Khajah M, Parhar K, Li Y, et al. Nlrp3 inflammasome plays a key role in the regulation of intestinal homeostasis. Inflammation Bowel Dis. (2011) 17:1359–72. doi: 10.1002/ibd.21478
50. Ma X, Di Q, Li X, Zhao X, Zhang R, Xiao Y, et al. Munronoid i ameliorates dss-induced mouse colitis by inhibiting nlrp3 inflammasome activation and pyroptosis via modulation of nlrp3. Front Immunol. (2022) 13:853194. doi: 10.3389/fimmu.2022.853194
51. Zhang Y, Tao M, Chen C, Zhao X, Feng Q, Chen G, et al. Baff blockade attenuates dss-induced chronic colitis via inhibiting nlrp3 inflammasome and nf-κb activation. Front Immunol. (2022) 13:783254. doi: 10.3389/fimmu.2022.783254
52. Elinav E, Strowig T, Kau AL, Henao-Mejia J, Thaiss CA, Booth CJ, et al. Nlrp6 inflammasome regulates colonic microbial ecology and risk for colitis. Cell. (2011) 145:745–57. doi: 10.1016/j.cell.2011.04.022
53. Tye H, Yu CH, Simms LA, de Zoete MR, Kim ML, Zakrzewski M, et al. Nlrp1 restricts butyrate producing commensals to exacerbate inflammatory bowel disease. Nat Commun. (2018) 9:3728. doi: 10.1038/s41467-018-06125-0
54. Kim SK, Cho YJ, Choe JY. Nlrp3 inflammasomes and nlrp3 inflammasome-derived proinflammatory cytokines in peripheral blood mononuclear cells of patients with ankylosing spondylitis. Clin Chim Acta. (2018) 486:269–74. doi: 10.1016/j.cca.2018.08.022
55. Guggino G, Mauro D, Rizzo A, Alessandro R, Raimondo S, Bergot AS, et al. Inflammasome activation in ankylosing spondylitis is associated with gut dysbiosis. Arthritis Rheumatol. (2021) 73:1189–99. doi: 10.1002/art.41644
56. Papayannopoulos V. Neutrophil extracellular traps in immunity and disease. Nat Rev Immunol. (2018) 18:134–47. doi: 10.1038/nri.2017.105
57. Lin EY, Lai HJ, Cheng YK, Leong KQ, Cheng LC, Chou YC, et al. Neutrophil extracellular traps impair intestinal barrier function during experimental colitis. Biomedicines. (2020) 8(8):275. doi: 10.3390/biomedicines8080275
58. Cao M, Yu M, Zhang Y, Tong D, Shi J. Neutrophil extracellular traps exacerbate inflammatory responses and thrombotic tendency in both a murine colitis model and patients with inflammatory bowel disease. Blood. (2017) 130:994–4. doi: 10.1182/blood.V130.Suppl_1.994.994
59. Li T, Wang C, Liu Y, Li B, Zhang W, Wang L, et al. Neutrophil extracellular traps induce intestinal damage and thrombotic tendency in inflammatory bowel disease. J Crohns Colitis. (2020) 14:240–53. doi: 10.1093/ecco-jcc/jjz132
60. Dinallo V, Marafini I, Di Fusco D, Laudisi F, Franzè E, Di Grazia A, et al. Neutrophil extracellular traps sustain inflammatory signals in ulcerative colitis. J Crohns Colitis. (2019) 13:772–84. doi: 10.1093/ecco-jcc/jjy215
61. Schroder AL, Chami B, Liu Y, Doyle CM, El KM, Ahlenstiel G, et al. Neutrophil extracellular trap density increases with increasing histopathological severity of Crohn's disease. Inflammation Bowel Dis. (2022) 28:586–98. doi: 10.1093/ibd/izab239
62. Li G, Lin J, Zhang C, Gao H, Lu H, Gao X, et al. Microbiota metabolite butyrate constrains neutrophil functions and ameliorates mucosal inflammation in inflammatory bowel disease. Gut Microbes. (2021) 13:1968257. doi: 10.1080/19490976.2021.1968257
63. Dong W, Liu D, Zhang T, You Q, Huang F, Wu J. Oral delivery of staphylococcal nuclease ameliorates dss induced ulcerative colitis in mice via degrading intestinal neutrophil extracellular traps. Ecotoxicol Environ Saf. (2021) 215:112161. doi: 10.1016/j.ecoenv.2021.112161
64. Papagoras C, Chrysanthopoulou A, Mitsios A, Ntinopoulou M, Tsironidou V, Batsali AK, et al. Il-17a expressed on neutrophil extracellular traps promotes mesenchymal stem cell differentiation toward bone-forming cells in ankylosing spondylitis. Eur J Immunol. (2021) 51:930–42. doi: 10.1002/eji.202048878
65. Chen WS, Chang YS, Lin KC, Lai CC, Wang SH, Hsiao KH, et al. Association of serum interleukin-17 and interleukin-23 levels with disease activity in chinese patients with ankylosing spondylitis. J Chin Med Assoc. (2012) 75:303–8. doi: 10.1016/j.jcma.2012.05.006
66. Romero-Sanchez C, Jaimes DA, Londoño J, De Avila J, Castellanos JE, Bello JM, et al. Association between th-17 cytokine profile and clinical features in patients with spondyloarthritis. Clin Exp Rheumatol. (2011) 29:828–34.
67. Ward MM, Deodhar A, Gensler LS, Dubreuil M, Yu D, Khan MA, et al. update of the american college of rheumatology/spondylitis association of america/spondyloarthritis research and treatment network recommendations for the treatment of ankylosing spondylitis and nonradiographic axial spondyloarthritis. Arthritis Care Res (Hoboken). (2019) 71:1285–99. doi: 10.1002/acr.24025
68. Casalegno GR, Däbritz J. New insights on cd8(+) t cells in inflammatory bowel disease and therapeutic approaches. Front Immunol. (2021) 12:738762. doi: 10.3389/fimmu.2021.738762
69. Leppkes M, Neurath MF. Cytokines in inflammatory bowel diseases - update 2020. Pharmacol Res. (2020) 158:104835. doi: 10.1016/j.phrs.2020.104835
70. Ma C, Wu W, Lin R, Ge Y, Zhang C, Sun S, et al. Critical role of cd6highcd4+ t cells in driving th1/th17 cell immune responses and mucosal inflammation in ibd. J Crohns Colitis. (2019) 13:510–24. doi: 10.1093/ecco-jcc/jjy179
71. Yang X, Garner LI, Zvyagin IV, Paley MA, Komech EA, Jude KM, et al. Autoimmunity-associated t cell receptors recognize hla-b*27-bound peptides. Nature. (2022) 612:771–7. doi: 10.1038/s41586-022-05501-7
72. Fiorillo MT, Maragno M, Butler R, Dupuis ML, Sorrentino R. Cd8(+) t-cell autoreactivity to an hla-b27-restricted self-epitope correlates with ankylosing spondylitis. J Clin Invest. (2000) 106:47–53. doi: 10.1172/JCI9295
73. Gracey E, Yao Y, Qaiyum Z, Lim M, Tang M, Inman RD. Altered cytotoxicity profile of cd8+ t cells in ankylosing spondylitis. Arthritis Rheumatol. (2020) 72:428–34. doi: 10.1002/art.41129
74. Liu D, Liu B, Lin C, Gu J. Imbalance of peripheral lymphocyte subsets in patients with ankylosing spondylitis: a meta-analysis. Front Immunol. (2021) 12:696973. doi: 10.3389/fimmu.2021.696973
Keywords: inflammatory bowel disease, ankylosing spondylitis, Mendelian randomization, disease activity, transcriptome analysis
Citation: Ding Y, Chen J, Li R and Xue L (2024) Inflammatory bowel disease activity threatens ankylosing spondylitis: implications from Mendelian randomization combined with transcriptome analysis. Front. Immunol. 15:1289049. doi: 10.3389/fimmu.2024.1289049
Received: 05 September 2023; Accepted: 14 February 2024;
Published: 28 February 2024.
Edited by:
Xiaolin Sun, Peking University People’s Hospital, ChinaReviewed by:
HuangHsi Chen, Chung Shan Medical University Hospital, TaiwanCopyright © 2024 Ding, Chen, Li and Xue. This is an open-access article distributed under the terms of the Creative Commons Attribution License (CC BY). The use, distribution or reproduction in other forums is permitted, provided the original author(s) and the copyright owner(s) are credited and that the original publication in this journal is cited, in accordance with accepted academic practice. No use, distribution or reproduction is permitted which does not comply with these terms.
*Correspondence: Luan Xue, eGVsY29AMTYzLmNvbQ==
†These authors have contributed equally to this work
Disclaimer: All claims expressed in this article are solely those of the authors and do not necessarily represent those of their affiliated organizations, or those of the publisher, the editors and the reviewers. Any product that may be evaluated in this article or claim that may be made by its manufacturer is not guaranteed or endorsed by the publisher.
Research integrity at Frontiers
Learn more about the work of our research integrity team to safeguard the quality of each article we publish.