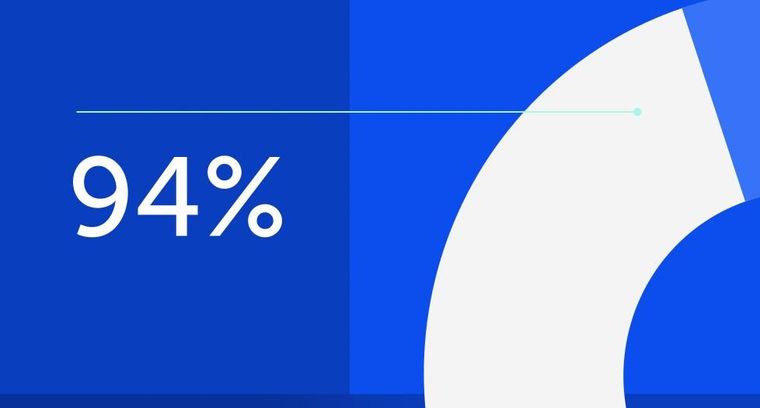
94% of researchers rate our articles as excellent or good
Learn more about the work of our research integrity team to safeguard the quality of each article we publish.
Find out more
ORIGINAL RESEARCH article
Front. Immunol., 07 February 2024
Sec. Alloimmunity and Transplantation
Volume 15 - 2024 | https://doi.org/10.3389/fimmu.2024.1280876
This article is part of the Research TopicImproving Stem Cell Transplantation Delivery Using Computational ModellingView all 6 articles
Introduction: Data on genomic susceptibility for adverse outcomes after hematopoietic stem cell transplantation (HSCT) for recipients are scarce.
Methods: We performed a genome wide association study (GWAS) to identify genes associated with survival/mortality, relapse, and severe graft-versus-host disease (sGvHD), fitting proportional hazard and subdistributional models to data of n=1,392 recipients of European ancestry from three centres.
Results: The single nucleotide polymorphism (SNP) rs17154454, intronic to the neuronal growth guidant semaphorin 3C gene (SEMA3C), was genome-wide significantly associated with event-free survival (p=7.0x10-8) and sGvHD (p=7.5x10-8). Further associations were detected for SNPs in the Paxillin gene (PXN) with death without prior relapse or sGvHD, as well as for SNPs of the Plasmacytoma Variant Translocation 1 gene (PVT1, a long non-coding RNA gene), the Melanocortin 5 Receptor (MC5R) gene and the WW Domain Containing Oxidoreductase gene (WWOX), all associated with the occurrence of sGvHD. Functional considerations support the observed associations.
Discussion: Thus, new genes were identified, potentially influencing the outcome of HSCT.
Hematopoietic Stem Cell Transplantation (HSCT) was an important breakthrough in the therapy of hematologic malignancies with up to 9 HSCT per 100.000 inhabitants currently being performed in Europe each year (1, 2). More than a million HSCTs have been carried out since the first successful transplants in 1968. Transplant-related mortality (TRM) rates are decreasing from about 50% in the 1970s to as low as 10% today (2). Human leukocyte antigen (HLA) minor and major incompatibilities between HSC donors and recipients can trigger allorecognition by T-cells. Relapse and graft-versus-host disease (GvHD) are the two major reasons for transplant (Tx) failure (3). A five-year rate of relapse after HSCT can be as high as 45%-50% (2). Relapse can be treated e.g. by donor lymphocyte infusion (DLI) or novel effective pharmacological compounds, such as tyrosine kinase inhibitors (TKI), hypomethylating agents or monoclonal antibodies (4, 5).
Acute GvHD (aGvHD) results from transplanted cells attacking alloantigens on the recipient’s tissues. aGvHD affects up to 35%–45% of HLA-matched siblings and 60%–80% of partly mismatched unrelated donor transplant recipients (6). Host antigen presenting cells are activated in response to damage-associated and pathogen-associated molecular patterns (DAMPs and PAMPs) released in the course of pretransplant conditioning and induce proliferation and activation of alloreactive donor T-cells. Subsequently, these T-cells mediate local tissue injury together with soluble inflammatory agents (e.g. IFN-γ, TNF-α) (3, 6). In contrast, chronic GvHD (cGvHD) is characterized by the presence of alloreactive, dysregulatory T- and B-cells. Almost every second HSCT recipient develops cGvHD, either de novo, during progression or after aGvHD. Furthermore, cGvHD is a major cause of late non-relapse associated mortality following HSCT (7). Prophylaxis for GvHD was introduced as an effective preventive action. Unfortunately, the lower incidence of severe GvHD was offset by high rates of relapse in malignancy.
During the past decade, a series of genomic variants have been discussed to be associated with GvHD or other transplantation (Tx)-related outcomes (8, 9). A first genome-wide association study (GWAS) associated genotype mismatch at HLA-DPB1 (10) and rs17473423 (12p12.1, now listed as rs1137282, located in the Kirsten rat sarcoma virus (KRAS) gene encoding K-Ras, a part of the RAS/MAPK pathway, were associated with GvHD in a Japanese population (11). Combining GWAS on a Finnish and a Spanish cohort with gene expression studies revealed further 51 genes potentially associated with GvHD (12). However, despite plausible molecular biological explanations, replication often fails, often due to incompatibilities between patient and donor cohorts with regards to HSCT treatment regimens and patient and donor characteristics (9, 13–15).
One major challenge in searching for genetic associations with post-Tx events are the different possible clinically relevant endpoints, the competing behaviour of target events in addition to the inherent differences between transplant cohorts (16, 17). Competing post-Tx events are events which prevent the occurrence or modify the risk of each other. These include the manifestation of relapse, or GvHD and death, and need to be taken into account when assessing cause-specific hazards, cumulative incidence, event-free or overall survival (18, 19). Both previously reported GWAS used a case-control design, meaning they compare, i.e., in the simplest case, the minor allele frequency (MAF) between GvHD cases and GvHD-free controls. Such comparisons may be biased if, for example, the time since Tx of cases and controls differ, or patients are prone to both GvHD and relapse, but the considered SNP is only associated with relapse (hence in the presence of competing risks) (20).
Here, we aim to identify genomic markers, associated with any of the relevant, post-Tx key events, death, relapse and GvHD, and establish a joint GWAS using recipient DNA samples from three transplantation centres. Complex survival analysis was carried out taking competing risks into account, as previously recommended (16, 17, 19).
This observational study is a joint analysis of samples collected in the Freeman Hospital of Newcastle (UK), the University Hospital Regensburg (Germany) and the University Medical Centre Göttingen (Germany). The patients were treated according to the respective standard of clinical protocols, between the years 2001 and 2017. All recipient and donor data were collected at the participating study centres in accordance with local standards and with the approval of the local research ethics committee. Inclusion criteria are provided in the Supplementary Material. All clinical data originate from the comprehensive routine documentation of the respective study centers. Patients were given the chance to follow them for at least a year.
Prior to data analysis, all phenotype data were cleaned from incorrect, inaccurate, or inconsistent entries, and harmonised between the study sites. Missing values and obvious errors have been removed or corrected after query. All dates were considered in days relative to the day of the 1st Tx. All medical causes of death excluding relapse were considered as treatment-related. Survival times with unknown or clearly non-medical/non-mental causes of death were treated as censored observations. Missing values on covariates were imputed taking known information on patient, donor and treatment for each study centre into account. Notwithstanding subsequent harmonization of grading and staging (21), aGvHD and cGvHD were graded according to previously published criteria, as recorded in the study centres (22, 23). For this analysis, we jointly considered an acute GvHD of grade III or IV or an extensive chronic GvHD as severe GvHD (further noted as sGvHD), as the overall survival (OS) after onset of GvHD differs significantly between aGvHD grade I, II and III/IV (p<0.001, detail see Supplementary Material). We finally estimated a hazard ratios (HR) of 2.31 (95%-CI: 1.84-2.89) for sGvHD compared to aGvHD grade 1 or 2 (p<.0001), corresponding to a difference in the median survival time (after onset of GvHD) of 3100 days (~8 yrs.) versus 5664 days (~15 yrs.).
DNA samples of 1,392 patients of European ancestry were available and shipped to the Research Unit of Molecular Epidemiology of the Helmholtz Zentrum München (HGMU). Genotyping was performed using the Infinium OncoArray 500K-V1.0 (OA) from Illumina Inc. in three batches between 2015 and 2017. The genotypes were subjected to strict quality control (QC) and harmonized between batches, including gender and ethnicity checks. Further details on the genotyping, quality assurance and QC are given in the Supplementary Material.
Overall, 499,563 markers could be genotyped. In total, 94,878 markers failed at least one of the QC-criteria. The most common reasons for exclusion were a MAF<1% or a call rate<90% (fraction of unsuccessful genotyping ≥10%) in at least one batch. After quality control (QC), 404,685 markers entered the data analysis.
We used SNPnexus, dbSNP, dbGene, Ensemble, and Illumina information files to assign markers to genes and other information (24–27). Pairwise linkage disequilibrium (LD) between markers was determined from Ldlink (28).
We considered the course after Tx characterised by the following key events: death, or the clinical manifestation of a relapse episode, or the clinical diagnosis of sGvHD (18, 29). At least death and relapse are competing events. Other censoring events were a 2nd Tx or loss-to-follow-up. Any information after the first occurrence of one of these events was ignored, to prevent therapeutic measures affecting any further post-HSCT course. If the study site documentation did not record any of the key events, or the absence of these key events was documented, we considered the respective key event as “not occurred. When describing the sample, the frequency of occurrence of a key event as the first event and the cumulative incidence function (CIF) on days 90 and 180 (Kaplan-Meier nonparametric) were reported.
When evaluating time-to-event data, the hazard function is usually modelled. A hazard is understood as the instantaneous risk that an undesirable event (e.g. relapse) will occur that has not previously occurred. With competing risks, the hazards of several events (e.g. death and relapse) are correlated, because e.g. the occurrence of a relapse changes by the hazard to die. Competing risks can mutually mask the occurrence of an other event; e.g. those patients who died cannot subsequently suffer a relapse. The so-called “at-risk group” includes all study participants who are at risk for an event at time point t. How those who have experienced a competing event prior to t are treated may vary.
We considered overall survival (OS) to be the time between Tx and death, regardless of relapse or sGvHD. We defined event-free survival time (EFS) by the duration between Tx and the first occurrence of one of the key events. EFS and OS are considered as composite endpoints and therefore to be “descriptive in nature”, because key events are either ignored (OS) or undifferentiated (EFS) (16). We complemented the modelling of EFS and OS with considerations of cause-specific hazard (CSH) for sGvHD, relapse and death (as non-relapse-non-sGvHD-mortality, NRGM), as recommended (16, 19).
Cox proportional hazard regression was used as framework for the analysis. Within this we investigated cause-specific hazard ratios (HR) for all key events separately (30). In addition, we fitted Fine‐Gray’s subdistribution hazard (SDH) models, that “cure the event of interest from failure from other causes”, and allows the study of effects that interfere the incidence of the event (31–33). In cause-specific hazard (CSH) models with a key event as the outcome (e.g. death), individuals who have experienced a competing event are removed from the “risk set”. In subdistribution hazard (SDH) models, these individuals remain in the “risk set”, albeit only “partially” due to the overall survival function S(t). CSH modelling is useful for aetiological research when one wants to investigate the causal relationship between risk factors (here a SNP) and a particular outcome (e.g. sGvHD). SDH modelling is useful for prognostic research when the aim is to predict the probability of an event (e.g. sGvHD) at time t for an individual patient. The results of the two models and for all the competing key events need to be considered together in order to make a conclusive statement about a SNP. For further details see (19, 34).
Therefore, for each SNP we fitted a total of 8 models to the data (one each for OS and EFS, three each for CSH and SDH – one per key event relapse, sGvHD and NRGM). These models were fitted conditional on the study centre, adjusted for patient-, donor- or Tx-related covariates (listed in Supplementary Table S1) and for 3 principal components (PCs, to adjust for population stratification), as well as adjusted for PCs only (crude). To avoid over-adjustment, relevant covariates were selected in a data-driven manner using the Akaike information criterion (AIC). Modelling was performed using PROC PHREG of SAS 9.4® (35).
Due to intensive and time-consuming modelling, we pre-selected SNPs in a fast but limited adequate modelling step, with SurvivalGWAS_SV (36). This software tool for GWAS of “time-to-event” outcomes does not allow conditioning on study centre or fitting of SDH models; and limits the number of covariates. Therefore, covariates informative for OS and EFS were combined into three scores: a “recipient score” (gender, age, previous autologous hematopoietic cell transplantation (auto-HCT), underlying condition, stage of disease, total body irradiation), a “donor/centre score” (study centre, waiting-time, patient/donor relationship, patient/donor gender-relationship), and a “Tx score” (T-cell depletion, GvHD prophylaxis, stem cell source, conditioning, reduced intensive conditioning). Details are provided in the supplement (Supplementary Table S9). Corresponding genomic inflation factors λ are given in Supplementary Table S10; they indicate that test results are methodologically inflated if they are greater than one.
Genome-wide significant SNPs (p ≤ 10-7, gwSNPs) and blocks of suggestively significant SNPs (10-5≤p ≤ 10-7; suggSNPs), as well as SNPs assigned to the same genes as gw/suggSNPs, were then reanalysed using the core statistical model described above.
The threshold for “genome-wide significance” was set at a level of p=10−7, for “suggestive significance” at p=10−5, and for “nominal significance” at p=0.05. We additionally demanded consistency of hazard ratios (HR; same direction and similar magnitude), respectively subdistributional hazard ratios (sHR), between subgroups and between crude vs. adjusted models. Most importantly, we demanded consistency in direction of effects (HRs) between all three study sites to demonstrate implicit replication in independent samples of different source populations. The subgroup analysis was performed in subsamples of size ≤90% of the total sample, but ≥350 (e.g. by study centre, by gender, underlying condition; in total 28 subsamples).
Figure 1 displays a schematic of the research/study strategy.
Figure 1 Schematic representation of the research/study strategy. * year of Tx (transplant), QC quality control, OS overall survival time, EFS event-free survival time, sGvHD severe graft-versus-host disease, NRGM non-relapse-non-sGvHD-mortality, 3 prin. comp. Three principal components of the genomic architecture to adjust for population stratification, gwSNP genome-wide significant SNPs (p ≤ 10-7), suggSNP suggestively significant SNPs (10-5≤p ≤ 10-7).
The genotyped study cohort included n=1,392 recipients of European ancestry who underwent a HSCT between January 2001 and July 2017. Of these recipients, n=548 (39%) were treated in Göttingen, n=258 (19%) in Newcastle, and n=586 (42%) in Regensburg. At Tx, they were 15 to 78 years old (median 50 yrs.) and n=869 (63%) were male. The underlying disease was leukaemia in n=882 cases (63%) and lymphoma in n=473 cases (34%). In n=524 (38%) cases, the disease was in complete remission (CR), in n=340 (24%) cases in partial remission (PR), while n=189 (14%) recipients had progressive or resistant underlying disease. Most recipients (n=952, 68%) received stem cells from HLA-matched unrelated donors, with approximately 30% from HLA-matched related donors (mainly siblings) while HLA-mismatches were only present in 7% of the donors. In >90% the grafts were peripheral blood stem cells (PBSC), in 83% cases cytostatic drugs were used for conditioning, with the majority of cases (68%) receiving a reduced intensive conditioning (RIC). About 95% of recipients received GvHD prophylaxis, about 77% underwent T-cell depletion (ATG in vivo was applied in Regensburg und Göttingen, in Newcastle CliniMACS device® TCRαβ-Biotin system, the CliniMACS® CD34 Reagent system and ATG in vivo was used).
Survival (time Tx to death) was fully recorded in 277 patients. aGvHD occurred as the first key event in 98 patients (7%, CIF-90 days: 8%, CIF-180 days: 9%), cGvHD in 128 patients (9%, CIF-90 days: 3%, CIF-180 days: 18%), relapse in 113 patients (8%, CIF-90 days: 5%, CIF-180 days: 12%), and NRGM in 93 patients (8% of all patients, 33% among those whose survival is fully known, CIF-90 days: 9%, CIF-180 days: 13%). More details are tabled in the Supplementary Material (Supplementary Tables S1–6).
Table 1 summarises some patient and donor characteristics, more details are in the Supplementary Material.
We observed a median EFS of 172 days (~1/2 year; 95% CI: 162-181 days) and a median OS of 789 days (~2 1/6 year, 95% CI: 662-890 days). OS and EFS rates one year after therapy were 62% and 30%, respectively; five years after therapy, 39% and 13%, respectively. Survival curves are presented in the Supplementary Figure S1, survival time are listed in Supplementary Table S1. We detected no genomic subclusters within recipients. However, the first principal component appeared to cover a minimal north-south gradient (see Supplementary Figure S1). Also, survival functions differ between the study centres (pEFS=4.6 10-6; see Supplementary Figure S3). Therefore, survival models were fitted conditional to study centres to avoid batch effects and minimize residual effects due to data collection methods, definitions of clinical endpoints, and patient management between the study sites.
Overall, 511 SNPs (58 gwSNPS, 123 suggSNPS, listed in Supplementary Tables S12 and 2, and 330 SNPs near them), selected by the fast pre-modelling of limited adequacy, were subjected to a more adequate analysis (proper adjustment for selected covariables, conditioned on study centre; SDH modelling). With this we achieved one genome-wide and 10 suggestive significant signals in the total sample (see Table 2).
The marker rs17154454, located at 7q21.11 intronic of SEMA3C, was genome-wide significantly associated with sGvHD (p=7.5x10-8) and to EFS (p=7x10-8) in the crude model (see Table 3). We estimated a hazard ratio of HR=2.68 (95% CI: 1.43-5.03, p=0.002) for sGvHD, adjusted for clinical features, which was comparable but less significant. The adjusted HR for EFS was slightly smaller (1.89, 95% CI: 1.22-2.93, p=0.004). Moreover, in most considered subgroups, we achieved similar HR estimates for EFS and sGvHD (see Figure 2). However, rs17154454 is an infrequent variant (MAF=3.2%) and hence only few recipients carry a minor allele. Thus, the sHR estimate of the SDH model was lower (adjusted: 1.40, 95% CI: 0.77-2.56, p=0.262). Therefore, the prognostic content of rs17154454 for sGvHD is limited.
Figure 2 Hazard ratio (HR) estimates of rs17154454 (SEMA3C) for sGvHD by subsamples. CSH, cause-specific hazard; HR, hazard ratio, 95% CI 95% confidence interval; sGvHD, severe graft-versus-host disease; GvHD-PPX, GvHD prophylaxis; P/D, patient-to-donor relationship; FdMp, female donor-to-male recipient relationship; mDorfP, male donor-or-female recipient relationship; PBSC, peripheral blood stem cells; adjusted model adjusted for patient-, donor- or Tx-related covariates and for 3 principal components, crude adjusted for 3 principal components only.
We further achieved suggestive significant associations for markers assigned to the genes RBFOX1, STK38L, PXN, WWOX, POLR3A, MC5R, ABCB5 and GABRG3; as well as for rs17207650 (a rare SNP in the intergenic region on 6p21.33/HLA region).
As in the screening step, we observed the common SNPs rs1151832 and rs4767886, located intronic of PXN and in high LD to eachother, as (suggestively) associated to NRGM. E.g. for rs4767886 the adjusted hazard ratio was HR=2.19 (95% CI: 1.59-3.01). In general, HR estimates were comparable between these markers, adjusted and crude modelling, and between subgroups. However, the effect was smaller and not significant in the subsample of Newcastle (e.g. adjusted: HR=1.38, 95% CI: 0.27-7.00, p=0.695). The p-values of an association to EFS or OS are <0.05 for both markers, but not to sGvHD or relapse. We also observed suggestive associations of both markers in the SDH model (e.g. rs4767886 adjusted: 1.87, 95% CI: 1.41-2.46). This together with a MAF of 12.7% indicates that PXN genotypes may have some prognostic value for NRGM.
We could suggestively associate the marker rs11080686, located at 19p11.21 downstream (3’UTR) of the gene MC5R, with the appearance of sGvHD (adjusted HR 1.54, 95% CI: 1.28-1.84, p=2.3x10-6). No association was seen with NRGM, relapse or OS. All hazard ratio estimates were comparable between crude and adjusted modelling, as well as between subgroups. Interestingly, the hazard ratio estimated in lymphoma patients (1.13, 95% CI: 0.81-1.57, p=0.442) was not significant and smaller than the estimate in leukemia patients (1.67, 95% CI: 1.33-2.09, p=7.9x10-6). We also achieved a suggestive p-value for the key event sGvHD in the SDH model (p=3.7x10-6). Together with a MAF of 19.1%, this indicates that MC5R genotypes may have some prognostic value for sGvHD.
For the marker rs9927016, located at 16q23.1 (spanning FRA16D, one of the most active common fragile sites in the human genome) within the gene WWOX, we observed a suggestive significant association with sGvHD (adjusted sHR=1.59, 95% CI: 1.22-1.84) in the SDH model (37). For the crude association we achieved a p=1.7x10-6, but adjusted for clinical features of p=0.0006 only. However, the adjusted hazard ratio estimates in the subsamples of Newcastle and Regensburg (both HR=1.69) and the crude estimate of Göttingen (HR=1.41) were consistently close.
Finally, for the marker rs17137725, located at 15q12 within the GABRG3 gene, we observed a suggestive significant association with relapse (adjusted HR=3.80, 95% CI 2.18-6.65, p=2.6x10-06), but neither for sGvHD nor for NRGM. Although the direction of hazard ratio estimates is the same in all considered subgroups, there is some inhomogeneity in the magnitude between the study centres. Interestingly, larger hazard ratios were also observed within HLA-unrelated recipient/donor-pairs (HR=6.11, 95% CI 2.79-13.3) and in the subgroup “male donor or female patient” (HR=5.60, 95% CI 3.10-10.1).
The remaining findings of suggestive evidence (rs17207650 (intergenic, sGvHD); rs17142828 (RBFOX1, EFS/sGvHD); rs7959382 (STK38L, sGvHD); rs34588967 (POLR3A, NRGM); and rs138553412 (ABCB5, EFS)) belonged to rare markers and results did not prove to be robust between crude and adjusted modelling or between subgroups. They should therefore be viewed with caution (details can be seen in the Supplementary Material).
We performed GWAS on post-Tx course with sGvHD, relapse, or death as the key events. Because these events compete, it is not advisable to focus on any one of these events alone, or examine composite endpoints (like EFS) or strongly simplified endpoints (like OS). In order to understand or biologically explain an observed association of a genomic marker, one needs to know which endpoint it is associated with and which one it is not. This is not possible, if only EFS, OS or one simple event is considered. Instead, we fitted models for aetiological and prognostic research, and for all competing key events to obtain an overall view of each SNP. We did not prioritize individual SNPs, nor did we include previous findings or speculation about a SNP in our analysis.
In general, identifying genes associated with the post-Tx events remains challenging due to disease- and treatment-related confounding, competing key events, multiple tests, among others. Examination of rare markers (MAF <5%) in the post-Tx course of HSCT is difficult and prone to inexplicably low p-values.
However, we found interesting genome-wide and suggestive significant signals for several genes as described below:
The gene SEMA3C was found to be positively associated with EFS or sGvHD in the final analysis, with genome-wide significance. Many types of cancer cells express class 3 semaphorins, to inhibit tumour angiogenesis and growth. In contrast, expression of SEMA3C is potentiating tumour progression in several cancer types. Subsequently, SEMA3C displays pro- and anti-tumorgenetic characteristics (38–40). Due to its role in neurodevelopmental processes which are frequently dysregulated in cancer, inhibiting SEMA3C signalling is proposed as therapeutic strategy to improve cancer control (39). SEMA3C may contribute to drug-induced resistance of cancer cells (27, 41). Interestingly, semaphoring C has also been shown to be involved in angiogenesis, and angiogenesis has been reported as a pathogenic factor in early GvHD, and it will be of interest to see whether there is a direct interaction of semaphoring C and endothelial repair in the context of allogeneic SCT (42–46). Another semaphoring Sema4D has been linked with T cell function in the setting of GvHD, but the mechanisms may be different form our observation (47).
The gene PXN was the only one that appeared at least suggestively significant regarding NRGM at the screening step and in the refined analysis. Paxillin functions as a docking protein for binding signalling molecules to a specific cellular compartment. As a central protein of focal adhesion, it is a common target of many different oncoproteins and involved in numerous processes. PXN is considered a susceptibility gene for many types of tumours, including large B-cell lymphoma (48, 49). Upregulated PXN expression was linked to poor OS in acute myeloid leukaemia (50) and has been associated with prediction of relapse in chronic myeloid leukaemia (51). Therefore, PXN seems to play a role in the post-Tx course, but for which key event remains unclear.
We were able to detect three further genes that are associated with the occurrence of severe GvHD: Plasmacytoma Variant Translocation 1 (PVT1), WW Domain Containing Oxidoreductase (WWOX) and Melanocortin 5 receptor (MC5R).
PVT1 is considered as a candidate oncogene, and was associated e.g. with Hodgkin lymphoma (HL) (27). PVT1 is closely located in the genome to the MYC gene, an universal amplifier of transcription associated with e.g. leukaemia or Burkitt lymphoma (52). They are considered “tween players among [some] haematological malignancies.” (53) Up-regulation of PVT1, mediated by MYC, is thought to confer a proliferation advantage to malignant cells, e.g. in acute myeloid leukaemia (AML), acute promyeloid leukaemia (APL), or multiple myeloma (MM) (53). PVT1 and the circularization of the exon 2 of the PVT1 gene (circPVT1), an alternative transcript, have been reported to enhance malignant cells and hinder the immune response to the tumour during cancer progression (53). Further, two independent variants of PVT1 (rs13255292 and rs4733601) were associated with diffuse large B-cell lymphoma (DLBCL), with genome-wide significance (54). These two markers, which were significant in our cohort, are in low LD to each other (D’ ≤ 0.357).
WWOX is a gene with many faces. Being located at a common fragile site (16q23.3) at the genome, it is considered a tumor suppressor gene. An increased incidence of lymphomas was observed in mouse experiments (55). However, WWOX is involved in several biologic functions interacting with many proteins (56). WWOX is involved in, inter alia, TGFβ1- and TNF-mediated cell death. WWOX inhibits Wnt-signaling and may control genotoxic stress-induced cell death synergistically with p53/TP53 (27, 57). WWOX has already been associated with severe aGvHD in a previous GWAS (p=1.75x10−7) (58). Defects in the gene WWOX are associated further with multiple types of cancer, i.a. hematopoietic malignancies (37, 59).
The protein encoded by MC5R is a receptor for melanocyte-stimulating and adrenocorticotropic hormones. It seems to play a role in sebum generation, but little is known about its involvement of tumorigenesis (27). In a small mouse experiment, MC5R was found as the only melanocortin receptor mediating the α-melanocyte-stimulating hormone (α-MSH) that promotes myelopoiesis, which in turn has been associated with GvHD in other animal models (60, 61).
Two other markers intronic of KCNS3 and LINC02570 were of genome-wide significance with respect to OS, but only at the screening step. The lack of a reasonable functional explanation and the lack of significance in the refined analysis limit the evidence for this finding.
Finally, the gene GABRG3 appeared suggestively significant related to relapse. The protein (a gamma-aminobutyric acid receptor) encoded by GABRG3 is the major inhibitory neurotransmitter in the mammalian brain (27). It contains the benzodiazepine binding site. Benzodiazepine use during HSCT was associated with adverse effects, as is the case in non-transplant patients (62, 63).. The GABAergic system is involved in immune cell functions, inflammatory conditions and diseases in peripheral tissues (64, 65). It was found that GABA protects human islet cells against the deleterious effects of immunosuppressive drugs and exerts immunoinhibitory effects (66).
Although we used all available data collected over a long period within three transplantation centres, the sample size is rather small for a GWAS considering three competing key events, due to the problem of multiple testing. We found it necessary to fit many and rather complex models in order to adequately apply Cox’s proportional hazard models to investigate the impact of individual SNPs on the course on the post-transplant outcome. While this is time-consuming and computationally expensive, it is the only way to separately assess their impact on key events while examine them in their entirety. Appropriate adjustment for clinical covariates appears to be another key to reliable estimation results. Thus, we were unable to run a single genome-wide analysis in suitable time with feasible use of computer resources. It is likely, that potentially associated markers were not selected during the screening step. We also restricted our investigation to recipient genotypes. A further differentiation between GvHD of different stages would be desirable, but only advisable in larger samples. Although we conducted some subgroup analyses, we did not fully investigate the interaction with clinical or environmental factors.
The observed suggestive association of a GABRG3 marker and relapse could be due to the administration of benzodiazepine during HSCT. Since the use of narcotic analgesics and psychotropic drugs was not considered as clinical covariate (data not available), the association, although unlikely, may be caused by residual confounding. If this is the case, it justifies the need for an appropriate adjustment when studying associations between gene and HST outcomes.
We found the recipient SNP rs17154454 of SEMA3C to be associated with EFS and severe GvHD. In addition recipient SNPs in the genes PXN, PVT1, MC5R and WWOX potentially influence the outcome of HSCT. All discovered genes are directly or indirectly involved in molecular pathways that could affect GvHD, relapse or other key events after HSCT. However, identifying genes associated with the post-Tx events remains challenging due to disease- and treatment-related confounders, competing key events, and multiple tests.
The datasets presented in this article are not readily available because of legal and privacy restrictions. Requests to access the datasets should be directed toYXJvc2VuYkBnd2RnLmRl.
The studies involving humans were approved by the Ethical Review Committee of the University of Regensburg (approval no 02-220), the Ethical Review Committee of the University Medical Center Göttingen (approval no 6/7/10) and the Newcastle and North Tyneside Research Ethics Committee (REC Red: 14/NE/1136 and 07/H0906/131). The studies were conducted in accordance with the local legislation and institutional requirements. Written informed consent for participation in this study was provided by the participants’ legal guardians/next of kin.
AR: Formal analysis, Methodology, Software, Writing – original draft. RC: Writing – review & editing, Resources. RD: Writing – review & editing. DK: Resources, Writing – review & editing. DW: Resources, Writing – review & editing. GW: Resources, Writing – review & editing. HB: Funding acquisition, Writing – review & editing, Supervision. AD: Resources, Writing – review & editing, Conceptualization, Funding acquisition. EH: Funding acquisition, Writing – review & editing, Conceptualization, Resources.
The author(s) declare financial support was received for the research, authorship, and/or publication of this article. RD, HB and DK were supported by the Deutsche Forschungsgemeinschaft (GRK 1034). RD, AD and EH were supported by the European Commission Grant FP7-PEOPLE-2012-ITN-315963 (CELLEUROPE). RD, HB, AD, EH, RC and DW were supported by the European Cooperation in Science & Technology under the COST Action CA17138 (Integrated European Network on Chronic Graft Versus Host Disease: EUROGRAFT). RC was supported by the Newcastle Hospitals Charity (BH191620). We acknowledge support by the Open Access Publication Funds of the Göttingen University (manuscript number: 1280876).
We thank Professor Matthew Collin, Translational & Clinical Research Institute, Medical School, Newcastle University, for making patient information and data available to us. We would also like to thank Ms Kienast, Arndt and Viogt, students at the University Medical School Göttingen, and Sigrun Gleich, study coordinator at the University Hospital Regensburg, for data extraction and collection.
The authors declare that the research was conducted in the absence of any commercial or financial relationships that could be construed as a potential conflict of interest.
The author(s) declared that they were an editorial board member of Frontiers, at the time of submission. This had no impact on the peer review process and the final decision.
All claims expressed in this article are solely those of the authors and do not necessarily represent those of their affiliated organizations, or those of the publisher, the editors and the reviewers. Any product that may be evaluated in this article, or claim that may be made by its manufacturer, is not guaranteed or endorsed by the publisher.
The Supplementary Material for this article can be found online at: https://www.frontiersin.org/articles/10.3389/fimmu.2024.1280876/full#supplementary-material
GWAS, genome wide association study; HSCT, hematopoietic stem cell transplantation; Tx, transplant; GvHD, graft-versus-host disease; sGvHD, severe GvHD; cGvHD, chronic GvHD; aGvHD, acute GvHD; OS, overall survival; EFS, event-free survival time; NRGM, non-relapse-non-sGvHD-mortality; SNP, single nucleotide polymorphism; MAF, minor allele frequency CSH, cause-specific hazard (model) SDH, Fine‐Gary’s subdistribution hazard (model); HR, hazard ratios; gwSNPs, genome-wide significant SNPs (p ≤ 10-7); suggSNPs, suggestively significant SNPs (10-5≤p ≤ 10-7).
1. Eurostat. Surgical operations and procedures statistics (2022). Available at: https://ec.europa.eu/eurostat/statistics-explained/index.php?title=Surgical_operations_and_procedures_statistics (Accessed February 22, 2023).
2. Horowitz M, Schreiber H, Elder A, Heidenreich O, Vormoor J, Toffalori C, et al. Epidemiology and biology of relapse after stem cell transplantation. Bone Marrow Transplant (2018) 53:1379–89. doi: 10.1038/s41409-018-0171-z
3. Bogunia-Kubik K, Łacina P. From genetic single candidate gene studies to complex genomics of GvHD. Br J Haematol (2017) 178:661–75. doi: 10.1111/bjh.14704
4. Kreidieh F, Abou Dalle I, Moukalled N, El-Cheikh J, Brissot E, Mohty M, et al. Relapse after allogeneic hematopoietic stem cell transplantation in acute myeloid leukemia: an overview of prevention and treatment. Int J Hematol (2022) 116:330–40. doi: 10.1007/s12185-022-03416-7
5. de Lima M, Porter DL, Battiwalla M, Bishop MR, Giralt SA, Hardy NM, et al. Proceedings from the National Cancer Institute’s Second International Workshop on the Biology, Prevention, and Treatment of Relapse after Hematopoietic Stem Cell Transplantation: Part III. Prevention and Treatment of Relapse after Allogeneic Transplantation. Biol Blood Marrow Transplant (2014) 20:4–13. doi: 10.1016/j.bbmt.2013.08.012
6. Ferrara JL, Levine JE, Reddy P, Holler E. Graft-versus-host disease. Lancet (2009) 373:1550–61. doi: 10.1016/S0140-6736(09)60237-3
7. Cooke KR, Luznik L, Sarantopoulos S, Hakim FT, Jagasia M, Fowler DH, et al. The biology of chronic graft-versus-host disease: A task force report from the national institutes of health consensus development project on criteria for clinical trials in chronic graft-versus-host disease. Biol Blood Marrow Transplant (2017) 23:211–34. doi: 10.1016/j.bbmt.2016.09.023
8. Hansen JA, Chien JW, Warren EH, Zhao LP, Martin PJ. Defining genetic risk for graft-versus-host disease and mortality following allogeneic hematopoietic stem cell transplantation. Curr Opin Hematol (2010) 17:483–92. doi: 10.1097/MOH.0b013e32833eb770
9. Partanen J, Hyvärinen K, Bickeböller H, Bogunia-Kubik K, Crossland RE, Ivanova M, et al. Review of genetic variation as a predictive biomarker for chronic graft-versus-host-disease after allogeneic stem cell transplantation. Front Immunol (2020) 11:575492. doi: 10.3389/fimmu.2020.575492
10. Ogawa S, Matsubara A, Onizuka M, Kashiwase K, Sanada M, Kato M, et al. Exploration of the genetic basis of GVHD by genetic association studies. Biol Blood Marrow Transplant (2009) 15:39–41. doi: 10.1016/j.bbmt.2008.11.020
11. Sato-Otsubo A, Nannya Y, Kashiwase K, Onizuka M, Azuma F, Akatsuka Y, et al. Genome-wide surveillance of mismatched alleles for graft-versus-host disease in stem cell transplantation. Blood (2015) 126:2752–63. doi: 10.1182/blood-2015-03-630707
12. Hyvärinen K, Koskela S, Niittyvuopio R, Nihtinen A, Volin L, Salmenniemi U, et al. Meta-analysis of genome-wide association and gene expression studies implicates donor T cell function and cytokine pathways in acute GvHD. Front Immunol (2020) 11:19. doi: 10.3389/fimmu.2020.00019
13. Chien JW, Zhang XC, Fan W, Wang H, Zhao LP, Martin PJ, et al. Evaluation of published single nucleotide polymorphisms associated with acute GVHD. Blood (2012) 119:5311–9. doi: 10.1182/blood-2011-09-371153
14. Martin PJ, Levine DM, Storer BE, Nelson SC, Dong X, Hansen JA. Recipient and donor genetic variants associated with mortality after allogeneic hematopoietic cell transplantation. Blood Adv (2020) 4:3224–33. doi: 10.1182/bloodadvances.2020001927
15. Dickinson AM, Norden J. Non-HLA genomics: does it have a role in predicting haematopoietic stem cell transplantation outcome? Int J Immunogenet (2015) 42:229–38. doi: 10.1111/iji.12202
16. Freemantle N, Calvert M, Wood J, Eastaugh J, Griffin C. Composite outcomes in randomized trials: greater precision but with greater uncertainty? JAMA (2003) 289:2554–9. doi: 10.1001/jama.289.19.2554
17. Kröger ECMMCDN ed. The EBMT handbook: hematopoietic stem cell transplantation and cellular therapies. New York, NY: Springer Berlin Heidelberg (2019). 688 p. doi: 10.1007/978-3-030-02278-5
18. Pintilie M. Competing risks: a practical perspective Vol. xiii. . Chichester, England; Hoboken, NJ: John Wiley & Sons (2006). 224 p. p. Table of contents only. Available at: http://www.loc.gov/catdir/toc/ecip0617/2006023988.htmlhttp://www.loc.gov/catdir/enhancements/fy0740/2006023988-d.html.
19. Varadhan R, Weiss CO, Segal JB, Wu AW, Scharfstein D, Boyd C. Evaluating health outcomes in the presence of competing risks: a review of statistical methods and clinical applications. Med Care (2010) 48:S96–105. doi: 10.1097/MLR.0b013e3181d99107
20. Pearce N, Checkoway H, Dement J. Design and conduct of occupational epidemiology studies: III. Design aspects of case-control studies. Am J Ind Med (1989) 15:395–402. doi: 10.1002/ajim.4700150404
21. Schoemans HM, Lee SJ, Ferrara JL, Wolff D, Levine JE, Schultz KR, et al. EBMT–NIH–CIBMTR Task Force position statement on standardized terminology & guidance for graft-versus-host disease assessment. Bone Marrow Transplant (2018) 53:1401–15. doi: 10.1038/s41409-018-0204-7
22. Glucksberg H, Storb R, Fefer A, Buckner CD, Neiman PE, Clift RA, et al. Clinical manifestations of graft-versus-host disease in human recipients of marrow from HL-A-matched sibling donors. Transplantation (1974) 18:295. Available at: https://journals.lww.com/transplantjournal/abstract/1974/10000/clinical_manifestations_of_graft_versus_host.1.aspx.
23. Filipovich AH, Weisdorf D, Pavletic S, Socie G, Wingard JR, Lee SJ, et al. National Institutes of Health consensus development project on criteria for clinical trials in chronic graft-versus-host disease: I. Diagnosis and staging working group report. Biol Blood Marrow Transplant (2005) 11:945–56. doi: 10.1016/j.bbmt.2005.09.004
24. Oscanoa J, Sivapalan L, Gadaleta E, Dayem Ullah AZ, Lemoine NR, Chelala C. SNPnexus: a web server for functional annotation of human genome sequence variation (2020 update). Nucleic Acids Res (2020) 48:W185–92. doi: 10.1093/nar/gkaa420
25. Cunningham F, Amode MR, Barrell D, Beal K, Billis K, Brent S, et al. Ensembl 2015. Nucleic Acids Res (2015) 43:D662–9. doi: 10.1093/nar/gku1010
26. Sherry ST, Ward MH, Kholodov M, Baker J, Phan L, Smigielski EM, et al. dbSNP: the NCBI database of genetic variation. Nucleic Acids Res (2001) 29:308–11. doi: 10.1093/nar/29.1.308
27. dbGene . Available at: https://www.ncbi.nlm.nih.gov/gene.
28. Machiela MJ, Chanock SJ. LDlink: a web-based application for exploring population-specific haplotype structure and linking correlated alleles of possible functional variants. Bioinformatics (2015) 31:3555–7. doi: 10.1093/bioinformatics/btv402
29. Austin PC, Lee DS, Fine JP. Introduction to the analysis of survival data in the presence of competing risks. Circulation (2016) 133:601–9. doi: 10.1161/CIRCULATIONAHA.115.017719
30. Fink AK, Yanik EL, Marshall BC, Wilschanski M, Lynch CF, Austin AA, et al. Cancer risk among lung transplant recipients with cystic fibrosis. J Cyst Fibros (2017) 16:91–7. doi: 10.1016/j.jcf.2016.07.011
31. Austin PC, Fine JP. Practical recommendations for reporting Fine-Gray model analyses for competing risk data. Stat Med (2017) 36:4391–400. doi: 10.1002/sim.7501
32. Putter H, Schumacher M, van Houwelingen HC. On the relation between the cause-specific hazard and the subdistribution rate for competing risks data: The Fine–Gray model revisited. Biometrical J (2020) 62:790–807. doi: 10.1002/bimj.201800274
33. Fine JP, Gray RJ. A proportional hazards model for the subdistribution of a competing risk. J Am Stat Assoc (1999) 94:496–509. doi: 10.1080/01621459.1999.10474144
34. Noordzij M, Leffondre K, van Stralen KJ, Zoccali C, Dekker FW, Jager KJ. When do we need competing risks methods for survival analysis in nephrology? Nephrol Dial Transplant (2013) 28:2670–7. doi: 10.1093/ndt/gft355
35. Shtatland ES, Kleinman K, Cain EM. 206-30: model building in PROC PHREG with automatic variable selection and information criteria, in: SUGI 30 proceedings: SAS Users Group International conference, Philadelphia, Pennsylvania. (US: SAS Institute) (2005) pp. Paper 206–30. Available at: https://support.sas.com/en/papers/proceedings-archive/sugi2005.html.
36. Syed H, Jorgensen AL, Morris AP. SurvivalGWAS_SV: software for the analysis of genome-wide association studies of imputed genotypes with “time-to-event” outcomes. BMC Bioinf (2017) 18:265. doi: 10.1186/s12859-017-1683-z
37. Gardenswartz A, Aqeilan RI. WW domain-containing oxidoreductase’s role in myriad cancers: Clinical significance and future implications. Exp Biol Med (Maywood) (2014) 239:253–63. doi: 10.1177/1535370213519213
38. Toledano S, Nir-Zvi I, Engelman R, Kessler O, Neufeld G. Class-3 semaphorins and their receptors: potent multifunctional modulators of tumor progression. Int J Mol Sci (2019) 20:556. doi: 10.3390/ijms20030556
39. Hao J, Yu JS. Semaphorin 3C and its receptors in cancer and cancer stem-like cells. Biomedicines (2018) 6:42. doi: 10.3390/biomedicines6020042
40. Bica C, Tirpe A, Nutu A, Ciocan C, Chira S, Gurzau ES, et al. Emerging roles and mechanisms of semaphorins activity in cancer. Life Sci (2023) 318:121499. doi: 10.1016/j.lfs.2023.121499
41. Kiseleva EP, Rutto KV. Semaphorin 3A in the immune system: twenty years of study. Biochem Moscow (2022) 87:640–57. doi: 10.1134/S0006297922070069
42. Iragavarapu-Charyulu V, Wojcikiewicz E, Urdaneta A. Semaphorins in angiogenesis and autoimmune diseases: therapeutic targets? Front Immunol (2020) 11:346. doi: 10.3389/fimmu.2020.00346
43. Papic N, Zidovec Lepej S, Gorenec L, Grgic I, Gasparov S, Filipec Kanizaj T, et al. The association of semaphorins 3C, 5A and 6D with liver fibrosis stage in chronic hepatitis C. PloS One (2018) 13:e0209481. doi: 10.1371/journal.pone.0209481
44. Yang W-J, Hu J, Uemura A, Tetzlaff F, Augustin HG, Fischer A. Semaphorin-3C signals through Neuropilin-1 and PlexinD1 receptors to inhibit pathological angiogenesis. EMBO Mol Med (2015) 7:1267–84. doi: 10.15252/emmm.201404922
45. Penack O, Henke E, Suh D, King CG, Smith OM, Na I-K, et al. Inhibition of neovascularization to simultaneously ameliorate graft-vs-host disease and decrease tumor growth. J Natl Cancer Inst (2010) 102:894–908. doi: 10.1093/jnci/djq172
46. Cordes S, Mokhtari Z, Bartosova M, Mertlitz S, Riesner K, Shi Y, et al. Endothelial damage and dysfunction in acute graft-versus-host disease. Haematologica (2021) 106:2147–60. doi: 10.3324/haematol.2020.253716
47. Duran-Struuck R, Tawara I, Lowler K, Clouthier SG, Weisiger E, Rogers C, et al. A novel role for the semaphorin Sema4D in the induction of allo-responses. Biol Blood Marrow Transplant (2007) 13:1294–303. doi: 10.1016/j.bbmt.2007.07.014
48. Sattler M, Pisick E, Morrison PT, Salgia R. Role of the cytoskeletal protein paxillin in oncogenesis. Crit Rev Oncog (2000) 11:63–76. doi: 10.1016/S1040-8428(97)10031-2
49. Schaller MD. Paxillin: a focal adhesion-associated adaptor protein. Oncogene (2001) 20:6459–72. doi: 10.1038/sj.onc.1204786
50. Chen Y, Zhao H, Xiao Y, Shen P, Tan L, Zhang S, et al. Pan-cancer analysis reveals an immunological role and prognostic potential of PXN in human cancer. Aging (Albany NY) (2021) 13:16248–66. doi: 10.18632/aging.203154
51. Yeung KY, Gooley TA, Zhang A, Raftery AE, Radich JP, Oehler VG. Predicting relapse prior to transplantation in chronic myeloid leukemia by integrating expert knowledge and expression data. Bioinformatics (2012) 28:823–30. doi: 10.1093/bioinformatics/bts059
52. Das SK, Lewis BA, Levens D. MYC: a complex problem. Trends Cell Biol (2023) 33:235–46. doi: 10.1016/j.tcb.2022.07.006
53. Ghetti M, Vannini I, Storlazzi CT, Martinelli G, Simonetti G. Linear and circular PVT1 in hematological Malignancies and immune response: two faces of the same coin. Mol Cancer (2020) 19:69. doi: 10.1186/s12943-020-01187-5
54. Cerhan JR, Berndt SI, Vijai J, Ghesquières H, McKay J, Wang SS, et al. Genome-wide association study identifies multiple susceptibility loci for diffuse large B cell lymphoma. Nat Genet (2014) 46:1233–8. doi: 10.1038/ng.3105
55. Ludes-Meyers JH, Kil H, Nuñez MI, Conti CJ, Parker-Thornburg J, Bedford MT, et al. WWOX hypomorphic mice display a higher incidence of B-cell lymphomas and develop testicular atrophy. Genes Chromosomes Cancer (2007) 46:1129–36. doi: 10.1002/gcc.20497
56. Abu-Remaileh M, Joy-Dodson E, Schueler-Furman O, Aqeilan RI. Pleiotropic functions of tumor suppressor WWOX in normal and cancer cells. J Biol Chem (2015) 290:30728–35. doi: 10.1074/jbc.R115.676346
57. Khawaled S, Nigita G, Distefano R, Oster S, Suh S-S, Smith Y, et al. Pleiotropic tumor suppressor functions of WWOX antagonize metastasis. Signal Transduct Target Ther (2020) 5:43. doi: 10.1038/s41392-020-0136-8
58. Goyal RK, Lee SJ, Wang T, Trucco M, Haagenson M, Spellman SR, et al. Novel HLA-DP region susceptibility loci associated with severe acute GvHD. Bone Marrow Transplant (2017) 52:95–100. doi: 10.1038/bmt.2016.210
59. Ishii H, Furukawa Y. Alterations of common chromosome fragile sites in hematopoietic Malignancies. Int J Hematol (2004) 79:238–42. doi: 10.1532/IJH97.03145
60. Xu Y, Yan J, Tao Y, Qian X, Zhang C, Yin L, et al. Pituitary hormone α-MSH promotes tumor-induced myelopoiesis and immunosuppression. Science (2022) 377:1085–91. doi: 10.1126/science.abj2674
61. Jardine L, Cytlak U, Gunawan M, Reynolds G, Green K, Wang X-N, et al. Donor monocyte-derived macrophages promote human acute graft-versus-host disease. J Clin Invest (2020) 130:4574–86. doi: 10.1172/JCI133909
62. Iqbal M, Spaulding AC, Ahmed S, Jani P, Wood C, Niazi S, et al. Opiate and benzodiazepine use during hospitalization for hematopoietic stem cell transplantation (HSCT) is associated with adverse health related outcomes. Blood (2018) 132:5873. doi: 10.1182/blood-2018-99-119781
63. Niazi SK, Iqbal M, Spaulding AC, Wood C, Manochakian R, Paulus A, et al. Impact of benzodiazepine use on length of stay and 30-day ED visits among hospitalized hematopoietic stem cell transplant recipients. South Med J (2022) 115:936–43. doi: 10.14423/SMJ.0000000000001481
64. Barragan A, Weidner JM, Jin Z, Korpi ER, Birnir B. GABAergic signalling in the immune system. Acta Physiol (2015) 213:819–27. doi: 10.1111/apha.12467
65. Bhandage AK, Barragan A. GABAergic signaling by cells of the immune system: more the rule than the exception. Cell Mol Life Sci (2021) 78:5667–79. doi: 10.1007/s00018-021-03881-z
Keywords: HSCT, GvHD, GWAS, survival, competing risks
Citation: Rosenberger A, Crossland RE, Dressel R, Kube D, Wolff D, Wulf G, Bickeböller H, Dickinson A and Holler E (2024) A genome-wide association study on hematopoietic stem cell transplantation reveals novel genomic loci associated with transplant outcomes. Front. Immunol. 15:1280876. doi: 10.3389/fimmu.2024.1280876
Received: 21 August 2023; Accepted: 15 January 2024;
Published: 07 February 2024.
Edited by:
Pawan Kumar Raghav, University of California, San Francisco, United StatesReviewed by:
Eva Maria Weissinger, Hannover Medical School, GermanyCopyright © 2024 Rosenberger, Crossland, Dressel, Kube, Wolff, Wulf, Bickeböller, Dickinson and Holler. This is an open-access article distributed under the terms of the Creative Commons Attribution License (CC BY). The use, distribution or reproduction in other forums is permitted, provided the original author(s) and the copyright owner(s) are credited and that the original publication in this journal is cited, in accordance with accepted academic practice. No use, distribution or reproduction is permitted which does not comply with these terms.
*Correspondence: Albert Rosenberger, YXJvc2VuYkBnd2RnLmRl
†These authors have contributed equally to this work and share last authorship
Disclaimer: All claims expressed in this article are solely those of the authors and do not necessarily represent those of their affiliated organizations, or those of the publisher, the editors and the reviewers. Any product that may be evaluated in this article or claim that may be made by its manufacturer is not guaranteed or endorsed by the publisher.
Research integrity at Frontiers
Learn more about the work of our research integrity team to safeguard the quality of each article we publish.