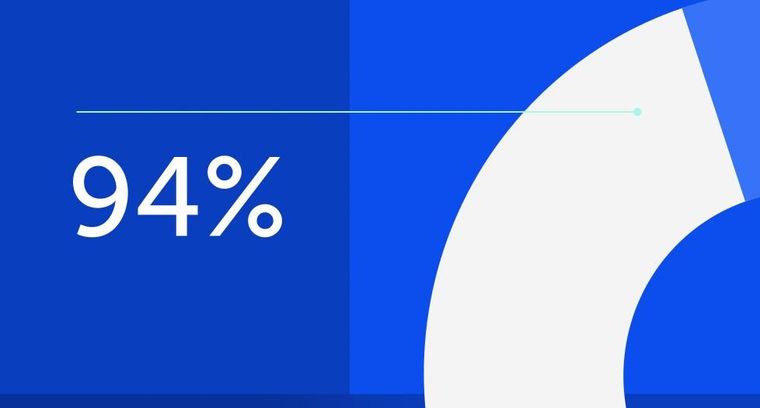
94% of researchers rate our articles as excellent or good
Learn more about the work of our research integrity team to safeguard the quality of each article we publish.
Find out more
ORIGINAL RESEARCH article
Front. Immunol., 12 January 2024
Sec. Inflammation
Volume 14 - 2023 | https://doi.org/10.3389/fimmu.2023.1310271
This article is part of the Research TopicSystemic Inflammation in Severe Infectious DiseasesView all 21 articles
Objective: The purpose of this study was to identify a panel of biomarkers for distinguishing early stage sepsis patients from non-infected trauma patients.
Background: Accurate differentiation between trauma-induced sterile inflammation and real infective sepsis poses a complex life-threatening medical challenge because of their common symptoms albeit diverging clinical implications, namely different therapies. The timely and accurate identification of sepsis in trauma patients is therefore vital to ensure prompt and tailored medical interventions (provision of adequate antimicrobial agents and if possible eradication of infective foci) that can ultimately lead to improved therapeutic management and patient outcome. The adequate withholding of antimicrobials in trauma patients without sepsis is also important in aspects of both patient and environmental perspective.
Methods: In this proof-of-concept study, we employed advanced technologies, including Matrix-Assisted Laser Desorption/Ionization (MALDI) and multiplex antibody arrays (MAA) to identify a panel of biomarkers distinguishing actual sepsis from trauma-induced sterile inflammation.
Results: By comparing patient groups (controls, infected and non-infected trauma and septic shock patients under mechanical ventilation) at different time points, we uncovered distinct protein patterns associated with early trauma-induced sterile inflammation on the one hand and sepsis on the other hand. SYT13 and IL1F10 emerged as potential early sepsis biomarkers, while reduced levels of A2M were indicative of both trauma-induced inflammation and sepsis conditions. Additionally, higher levels of TREM1 were associated at a later stage in trauma patients. Furthermore, enrichment analyses revealed differences in the inflammatory response between trauma-induced inflammation and sepsis, with proteins related to complement and coagulation cascades being elevated whereas proteins relevant to focal adhesion were diminished in sepsis.
Conclusions: Our findings, therefore, suggest that a combination of biomarkers is needed for the development of novel diagnostic approaches deciphering trauma-induced sterile inflammation from actual infective sepsis.
Trauma and sepsis are significant healthcare problems that urgently require novel diagnostic methods to enable optimal and timely medical care (1, 2). This is all the more true in trauma patients. In both acute conditions, septic shock, and trauma patients may be admitted to the intensive care unit (ICU) for initial treatment and stabilization and cared for by the same multisciplinary intensive care teams. At this stage clinicians are urged to choose the correct treatment including eradication of microbial foci and provision of antimicrobials for the septic but to refrain from such treatment in trauma patients (3). Indeed, inappropriate surgical intervention for foci removal in trauma patients may exacerbate inflammation (4) while indiscriminate use of antibiotics may promote the emergence and colonization of multidrug resistant bacteria as well as leading to wellknown side effects of such treatment, especially with broadspectrum antibiotics. Clinical score systems and routine blood sample assays may direct the clinician but there is yet no specific marker to make this distinction and thus, appropriate care remains challenging as even gene expression is very close in both conditions (5). First, trauma patients may develop profound immunosuppression making them more susceptible to severe infection (6). Second, due to the early release of Damage-Associated Molecular Patterns (DAMPs) (7), severe trauma patients develop systemic inflammation, hyperthermia, vasoplegia (8), hyperleucocytosis, and elevated inflammatory biomarkers all mirroring sepsis despite no infection (9, 10). The same release of DAMPs also promotes immune suppression (11, 12). Indiscriminate use of antibiotics in severe trauma patients may significantly worsen their outcome (13), delay the diagnosis of a subsequent infection, and promote the emergence of multi-drug resistant microbes. Because of their similar clinical symptoms, it is crucial to implement precise and efficient diagnostic tools that allow fast and reliable differentiation between sterile inflammation and microbial sepsis in trauma patients. The study by Chung et al. showed that trauma patients developing sepsis (11.85% of the total cohort of trauma patients) deteriorated at a rate significantly higher than those who did not develop sepsis, 28.0% versus 4.6%, p >0.001 (14). This distinction is also critical for the development of timely surgical interventions (removal of infective foci in wounds, laparotomy for perforated bowel) and tailored antimicrobial treatments that can help improve patient outcomes (14, 15).
Trauma is the primary cause of mortality among individuals under the age of 45, with sepsis significantly contributing to these fatalities following traumatic injuries and infections (16). The incidence of sepsis among trauma patients is an alarming concern, where it significantly increases mortality (17). The annual incidence of sepsis in hospitalized patients is estimated to be over 750,000, with a mortality rate close to 30% (15). In trauma patients, a large percentage of deaths occur before reaching the hospital or within the first few hours after admission (15). Infections related to trauma often affect multiple organs and can manifest early, within the first four days. This holds true in trauma patients with contaminated wounds and fractures (18), early aspiration pneumonia in brain-injured patients (19), or superinfection of lung contusions (20). Some trauma patients may experience systemic inflammatory response syndrome (SIRS), while others may exhibit a compensatory anti-inflammatory response syndrome (CARS) (21), both leading to a modulated inflammatory response. The risk of infection in trauma patients arises from factors such as the disruption of mechanical barriers, bacterial contamination, local wound conditions, and invasive medical procedures (e.g. invasive mechanical ventilation, central venous lines, and catheters). Additionally, host defense factors can be compromised, leading to impaired immune responses (22). Infections secondary to trauma are most often of bacterial origin but can also be caused by viruses or, more rarely, by fungi (23).
Differentiating sterile inflammation from sepsis in trauma patients is crucial for appropriate management and treatment. The usually used parameters, C-reactive protein, CRP, and Interleukin-6, IL-6, are unfortunately stimulated via common mechanisms in both sterile and septic inflammation, and even procalcitonin (PCT) shows restrictions in that it is mainly induced by bacterial and not viral or atypical bacterial sepsis. According to Pierrakos et al. these parameters may rather be used to exclude sepsis (24). Recent findings confirm that PCT cannot accurately discriminate infectious vs. noninfectious acute inflammation in critically ill patients with severe traumatic injuries (area under the receiver operating characteristics curve: 0.53; 95% CI, 0.42–0.64) (25). In addition, machine learning algorithms were used to analyze a large multi-omic database of over 8500 markers, including proteomics, metabolomics, and lipidomics, to identify prognostic biomarkers in trauma patients’ admission plasma samples for predicting outcomes like mortality and recovery. Abdelhamid et al. thus revealed that a combination of five proteins was best for discriminating critical illness resolution and 26 multi-omic features were effective in predicting 30-day survival, with the study suggesting the potential for novel prognostic biomarkers in trauma patients’ admission data (26).
Early recognition of sepsis is essential for improving clinical prognosis and reducing mortality (15, 24, 27). The definition of sepsis has evolved over time, with the latest definition emphasizing life-threatening organ dysfunction caused by a dysregulated host response to infection (28, 29). Quick Sepsis-associated Organ Failure Assessment (qSOFA) scores are used as screening tools to aid in the rapid diagnosis of sepsis (28). However, their discriminative power in surgical intensive care patients is very low (9) and the challenge to identify patients at risk has resulted in new approaches for scores e.g. SAPS3 and biomarkers (30–32). Trauma patients thus present unique challenges (one of them being the timely and discriminate diagnosis of infection), and their clinical course can be complicated by sepsis and sepsis-induced mortality (33).
Timely diagnosis and treatment of infection in these patients are associated with improved outcomes and reduced mortality (34). However, the standard methods for diagnosing pathogens during sepsis, such as blood or bronchoalveolar lavage cultures, can be limited in sensitivity and turnaround time (35, 36). New diagnostic techniques are being explored to enhance pathogen detection in sepsis cases (35, 37). For instance, mass spectrometry has emerged as a promising tool for identifying biomarkers in the metabolome and proteome, potentially enhancing the early diagnosis and prognosis of sepsis (38). Identifying new biomarkers to differentiate between damage-induced sterile inflammation and sepsis in trauma patients could provide valuable insights for early detection of infection and timely intervention (39). Diagnostic tests that rapidly and reliably identify the presence or absence of infection in the ICU population, including severe trauma patients are a pressing unmet need (40). Biomarkers can help in identifying subclinical changes before the establishment of disease, allowing for early therapeutic interventions (41). However, further research is needed to establish the utility and reliability of such biomarkers in the diagnosis and management of trauma and sepsis patients (42). In the present study, we show that advanced technologies, including MALDI and MAA, can aid in identifying biomarkers to distinguish between early stage sepsis and non-infected inflammation in ventilated trauma patients.
Informed consent was obtained from the patients or their guardians included in this study. Samples from Helsingborg Hospital ICU were approved by the Ethics committee in Lund Dnr. 2014/195, 2015/467 and 2019/04558 and samples from Charles-University Prague were approved by the Ethics Committee of the Military University Hospital Prague Dnr. 108/9-36/2016-UVN. Samples from Strasbourg University Hospital were approved by the French Agence Nationale de la Sécurité du Médicament et des Produits de Santé (ANSM, on October 5, 2018) and a National Institutional Review Board (CPP, on November 6, 2018, CPPIDF1-2018-ND51-Cat. 1; N°IRB/IORG#: IORG 0009918), which covers all participant sites. No patient identifiable information is presented.
The trauma population consisted in 23 ventilated trauma patients included in the Traumadornase multicenter study [NCT03368092 (43)] in the following study sites: Clermont-Ferrand, Reims and Strasbourg University Hospitals, all in France. As per protocol, these patients were adult severe trauma patients (Injury Severity Score [ISS (44)] > 15, under mechanical ventilation and included within the first 18 hours after admission to the trauma bay. Severe trauma patients under mechanical ventilation are more challenging than their non-ventilated counterparts as they develop more healthcare-associated infections, namely but not restricted to ventilation-associated pneumonia (VAP) and whose definitive diagnosis remains a serious challenge (45). Those patients had their blood sampled on days 1 (admission to the ICU), 3, and 5 and were screened daily throughout their ICU stay for the development of subsequent VAP according to the Center Disease Control and Prevention (46) and other infectious diseases requiring antimicrobial treatment according to the definition of the International Sepsis Forum (47). Infections in trauma patients were then adjudicated based on post-hospitalization review.
The sepsis group contained 23 patients with community acquired sepsis, included after admission to the ICU within 24 hours of arrival to the hospital in Helsingborg, Sweden. These sepsis patients were a subgroup of patients included in an earlier reported cohort (48). Inclusion criteria were; non-pregnant adults >18 years old with no surgery or blood transfusion during the 7 preceding days, and an expected ICU stay > 2 days (49). All patients with community aquired sepsis were on mechanical ventilation and received vasopressors, all had positive blood cultures, and they were all defined as having septic shock (28). According to ICU routine, they were assessed by the Simplified Acute Physiology Score 3 (SAPS3) (50). Clinical scores and blood tests were obtained daily for seven consecutive days. Day 1 was the day of arrival in the ICU.
In both trauma and sepsis patients, blood samples were drawn into EDTA tubes and after a centrifugation step stored at −80°C until use. Plasma samples from even healthy volounteers were also collected.
Using RayBiotech, Inc.’s Quantibody® Technology Array service, inflammatory proteins were analyzed using a glass slide-based quantitative antibody array (Human L-507 and L-493) as described by the manufacture’s instructions.
For measurements in plasma the following ELISA kits were used: TREM1 (Cat No: OKEH00303; Aviva Systems Biology), IL1F10 (Cat No: EKX-E3NR3R; Nordic BioSite), Serpin A9 (Cat No: MBS935459; MyBioSource), A2M (Cat No: EKX-6NKMQS; Nordic BioSite), GPI (Cat No: OKEH06383; Aviva Systems Biology), VIPR2 (Cat No: EKH3534; Nordic BioSite), S100A10 (Cat No: EKX-EWP59E; Nordic BioSite), IL3 (Cat No: EKH392; Nordic BioSite), SYT13 (Cat No: MBS9316889; MyBioSource) and TNFRSF10 (Cat No: OKEH04990; Aviva Systems Biology). Protein concentrations were measured by using human antigen specific ELISA Kits according to manufacturer’s protocols. Absorbance was measured in a VICTOR3™ Microplate Reader (Perkin Elmer) as described previously (51).
The abundant plasma proteins were depleted using the High-Select™ Top12 abundant protein depletion resin kit (Thermo Fischer Scientific, Waltham, MA, USA) according to the manufacturer’s instructions. Briefly, an aliquot of 8 µl from each sample was transferred onto the conditioned immuno depletion resin and incubated at room temperature (RT) for 20 min. Depleted proteins were collected by centrifugation at 1000 × g for 2 min. SDS was added at a concentration of 2%. The samples were digested with trypsin using the filter-aided sample preparation (FASP) method (52). Briefly, the samples were reduced with 100 mM dithiothreitol at 60°C for 30 min. The reduced samples were transferred to 30 kDa MWCO Pall Nanosep centrifugation filters (Pall Corporation), washed several times with 8 M urea and once with digestion buffer (DB, 0.5% sodium deoxycholate in 50 mM TEAB) prior to alkylation with 10 mM methyl methanethiosulfonate in digestion buffer for 20 min in room temperature. Digestions were performed by addition of Pierce MS grade Trypsin (Thermo Fisher Scientific) in DB to a trypsin:protein ratio of 1:100 and incubated overnight at 37°C. The next morning, an additional portion of trypsin was added and incubated for another three hours at 37°C. Peptides were collected by centrifugation and labeled using TMT (tandem mass tag) 10-plex isobaric mass tagging reagents (Thermo Scientific) according to the manufacturer’s instructions. Labeled samples from each sample type were combined into two sets, and sodium deoxycholate was removed by acidification with 10% TFA. The combined TMT-labeled samples were desalted using Pierce Peptide Desalting Spin Columns (Thermo Scientific) following the manufacturer’s instructions.
Each set of samples was pre-fractionated on the Dionex Ultimate 3000 UPLC system (Thermo Fischer Scientific) using the Waters XBridge BEH C18 column (3.0 mm x 150 mm, 3.5µm, Waters Corporation, Milford, USA) and the a linear gradient of solvent A and B was applied as follows - gradient from 3% to 40% solvent B over 18 min, from 40% to 100% B over 5 min, 100% B for 5 min, all at the flowrate of 0.4 ml/min. Solvent A was prepared with 10 mM ammonium formate in water at pH 10.0, solvent B was prepared with 90% acetonitrile, 10% 10 mM ammonium formate in water at pH 10.0. Solvent A was 0.2% formic acid and solvent B was 80% acetonitrile, 0.2% formic acid. The 40 primary fractions were concatenated into 20 fractions (1 + 21, 2 + 22, ..., 20 + 40), evaporated and reconstituted in 3% acetonitrile, 0.2% formic acid for nLC-MS/MS analysis. Each fraction was analyzed on an Orbitrap Fusion Tribrid mass spectrometer interfaced with an Easy-nLC 1200 nanoflow liquid chromatography system (both Thermo Fisher Scientific). Peptides were trapped on the Acclaim Pepmap 100 C18 trap column (100 μm x 2 cm, particle size 5 μm, Thermo Fischer Scientific) and separated on the in-house packed C18 analytical column (75 μm x 32 cm, particle size 3 μm) using the gradient from 5% to 32% B in 75 min, from 32% to 100% B in 5 min, and 100% B for 10 min at a flow of 300 nl/min. MS scans were performed at 120,000 resolution, m/z range 380-1200. MS/MS analysis was performed in a data-dependent manner, with a top speed cycle of 3 s for the most intense doubly or multiply charged precursor ions. Precursor ions were isolated in the quadrupole with a 0.7 m/z isolation window, with dynamic exclusion set to 10 ppm and a duration of 45 seconds. Isolated precursor ions were subjected to collision induced dissociation (CID) at 35 collision energy with a maximum injection time of 50 ms. Produced MS2 fragment ions were detected in the ion trap followed by multinotch (simultaneous) isolation of the top 7 most abundant fragment ions for further fragmentation (MS3) by higher-energy collision dissociation (HCD) at 60% and detection in the Orbitrap at 50 000 resolutions, m/z range 100-500.
MS raw data files for the TMT set were merged for relative quantification and feature identification conducted using Proteome Discoverer version 1.4 (Thermo Fisher Scientific). A database search for each set was performed with the Mascot search engine (Matrix Science) using the Homo Sapiens Swissprot database, version Mars 2017 with 553941sequences. MS peptide tolerance of 5 ppm and MS/MS tolerance for identification of 600 millimass units (mmu), tryptic peptides with zero missed cleavage and variable modifications of methionine oxidation, fixed modifications of cysteine alkylation, N-terminal TMT-label and lysine TMT-label were selected. The detected peptide threshold in the software was set to a significance of FDR 1% by searching against a reversed database and identified proteins were grouped by sharing the same sequences to minimize redundancy. For TMT quantification, the ratios of the TMT reporter ion intensities in HCD MS/MS spectra (m/z 126-131) from raw data sets were used. Ratios were derived by Proteome Discoverer using the following criteria: fragment ion tolerance as 3 mmu for the centroid peak with the smallest delta mass and a minimum intensity of 2000. Only peptides unique for a given protein were considered for relative quantitation, excluding those common to other isoforms or proteins of the same family. The quantification was normalized within Proteome Discoverer 1.4, using the global median of all proteins. Calculations of the ratios were made by using a reference sample made from a mix of 4 of the samples or the control sample as denominator.
All analyses of Matrix-Assisted Laser Desorption/Ionization (MALDI) and multiplex antibody array (MAA) data were performed in the open-source programming environment R (www.r-project.org, version 4.2.1). The same approach for data processing was followed for both datasets, generated by MALDI (777 analytes) and MAA (995 analytes) screening. First, for each analyte the ratio of protein levels between the trauma and sepsis groups was computed. The log2 of the aforementioned ratio was then used for downstream analysis and for ranking analytes. To highlight the relative abundance of the top 100 ranked proteins contrasting the trauma and sepsis groups, heatmaps were created using the ‘ComplexHeatmap’ package (version 2.12.1).Venn diagrams displaying unique and common proteins among all time points from the previously selected batch were constructed with the ‘nVennR’ package (version 0.2.3). For the data from MALDI screening, considering the log2-fold change of all given proteins, pathway enrichment analysis was done utilizing the ‘clusterProfiler’ package (version 4.7.1.3). The minimal allowed set size included in the enrichment analysis was set to 5. For reproducibility of the results, a seed was set. Enriched KEGG pathway terms as well as the associated proteins were depicted in barplots and Gene-Concept Networks with the help of the ‘enrichplot’ package (version 1.18.4).
ELISA data were processed and presented with Microsoft Excel 2021 (Microsoft, Redmond, WA) and GraphPad Prism 9.0 (GraphPad Software, San Diego, CA). All results are presented as mean values ± SEM with the number of independent experiments and patients per group indicated in the figure legends. The comparison of data was performed by one-way ANOVA, Tukey’s multiple comparison test and area under the curve (AUC) analysis were performed with 95% confidence interval, using the Wilson/Brown method.
Demographic, clinical and infectious data for the 23 included trauma patients are given in Table 1. In brief, these were predominantly young, male, severe trauma ICU patients mechanically ventilated for a median duration of 7 days. Among the trauma cohort, 3 patients developed VAP on days 1, 3 and 6, respectively (day 0 being admission day). No patient in the trauma cohort developed any infectious disease (excluding VAP) requiring antimicrobials during the first seven days. As depicted in Table 2, patients in the sepsis cohort were older, comorbid ICU patients in whom the sources of infection were mainly the respiratory tract and soft tissue. Biological workup and invasive therapeutic procedures (renal replacement therapy, invasive mechanical ventilation, vasopressor infusion) reflect the moderate severity of these septic ICU patients. SOFA scores in the trauma and sepsis populations (8 [5,5-10] and 11 [8,5-101, respectively) were non-significantly different (p=0.14). The plasma samples from 7 healthy individuals were used as controls.
The first series of experiments was performed to investigate the protein abundance in plasma samples from trauma and sepsis patients. To achieve this goal, we utilized cutting-edge technologies such as MALDI and MAA, respectively. These two methods allowed us to probe the proteomic terrain, unveiling specific biomarkers that exhibit potential for early detection and differentiation of trauma and sepsis patients. By applying comparative analysis methods, we assessed the protein levels in plasma sampled from patients with trauma and sepsis. To illustrate their expression patterns, the top 100 proteins (ranked by their log2 fold change) derived from the MALDI and MAA screenings are presented in Figures 1A, 2A. The log2-fold changes within the sepsis to trauma (S/PT) group spanned a range from approximately 5.6 to -5.4 and 4.4 to -4.0 within the 7-day time period tested, respectively. Positive numbers indicate higher protein levels in the sepsis group, while negative numbers present higher protein levels in the trauma group. Among the selected proteins, the distribution of common and unique biomarkers at different time points was depicted with Venn diagrams (Figures 1B, 2B). A total of 15 and 21 proteins were revealed to recur on all days, and their relative abundance was singled out in an additional heatmap (Figures 1C, 2C).
Figure 1 Protein abundance after MALDI screening. (A) Top 100 differentially regulated proteins at day 1, 3, 5 and 7 contrasting trauma and sepsis groups. The relative abundance of protein levels is shown as log2-fold change from sepsis to trauma (S/PT) group. Protein names that occurred only on a single day were printed in bold. (B) Venn diagram showing the common and unique proteins among all time points. (C) Heatmap highlighting the 21 recurring proteins on all days.
Figure 2 Protein abundance after MAA screening. (A) Top 100 differentially regulated proteins at day 1, 3, 5 and 7 contrasting trauma and sepsis groups. The relative abundance of protein levels is shown as log2-fold change of sepsis to trauma (S/PT) group. Protein names that occurred only on a single day were printed in bold. (B) Venn diagram showing the common and unique proteins among all time points. (C) Heatmap highlighting the 15 recurring proteins on all days.
We also performed an enrichment analysis on the MALDI data independently for each day. Figure 3 shows the top 15 enriched KEGG pathways per day, together with Gene-Concept Networks illustrating the top 5 enriched terms featuring the associated proteins and their relative abundance. While proteins belonging to the complement and coagulation cascades (see day 5) were generally raised, others, such as proteins relevant for focal adhesion (see day 1, day 7), were mostly diminished in the sepsis group compared to the trauma group. In summary, the comparative analysis of protein abundance and enrichment patterns between trauma and sepsis patients reveals distinct molecular signatures. In sepsis, elevated complement and coagulation cascade proteins contrast with diminished focal adhesion-related proteins, underscoring their potential as crucial diagnostic indicators.
Figure 3 Enrichment analysis of KEGG pathway terms for differentially regulated proteins contrasting trauma and sepsis group at various days. The top 15 KEGG pathway terms are listed for the days 1, 3, 5 and 7. Additionally, Gene-Concept Networks illustrate the relevant proteins associated with the top 5 KEGG pathway terms per time point. The relative abundance of protein levels is shown as log2-fold change of sepsis to trauma (S/PT) group.
The next series of experiments aimed at identifying potential biomarkers to distinguish sepsis patients from individuals with other inflammatory conditions. This proof-of-concept study involved comparing protein levels in various scenarios, including fever (n=6), bacteremia (n=5), trauma (n=4), and sepsis (n=6), using plasma samples from healthy individuals (n=6) as controls (Supplementary Table 1). To authenticate candidate biomarkers, we conducted a comprehensive analysis and identified IL1F10, A2M, GPI, VIPR2, S100A10, and SYT13 as potential targets (Figures 1, 2). Plasma protein levels were also assessed at various time points using ELISA (Figure 4). Additionally, we explored the measurement of other noteworthy targets, namely IL3 (55), TREM1 (56), SERPIN A9 (57), and TNFRSF10 (58) (Figure 4). Our analysis showed enhanced levels of SYT13, IL1F10, and S100A10 cytokines in early sepsis patients, while TREM1 levels in trauma patients increased gradually over time (Figure 4). Notably, A2M levels were consistently lower in inflammatory circumstances, particularly in sepsis patients. Additionally, we observed the stability of SERPIN A9, GPI, IL3, VIPR2, and TNFRSF10 levels across different conditions, revealing their limited potential as discriminatory biomarkers (Figure 4).
Figure 4 Identification of sepsis biomarkers. We assessed plasma protein levels using quantitative ELISA at various stages of disease development. Data are displayed as mean +/- SEM. Healthy individuals (n=6), fever (n=6), bacteremia (n=5), trauma (n=4), and sepsis (n=6).
To validate our findings, we extended the number of plasma samples for trauma (n = 23) and sepsis patients (n = 23) and performed quantitative ELISA to measure the contents of A2M, IL1F10, SYT13, and TREM1 in these samples at three time points (day 0, 3 and 5). Plasma samples from healthy individuals (n = 7) were used as control. This analysis spanned various time points in both trauma and sepsis cases, covering the initial (day 0) and later stages (day 5) of the ICU stay. As seen before, distinct patterns emerged with increased SYT13 and IL1F10 levels in plasma samples from sepsis patients, while A2M levels were decreased when compared to the level detected in plasma samples from trauma patients (Figure 5). TREM1 levels, however, increased only in plasma samples from trauma patients but not in those from sepsis patients over the course of time (Figure 5). These findings confirm that the identified biomarkers, A2M, IL1F10, SYT13, and TREM1, hold significant potential in distinguishing trauma and sepsis patients. In addition, the data was further tested using ROC curves (Figure 5, right). For early detection of sepsis patients from trauma, the AUC for SYT13 is 0.8913 and for IL1F10 is 0.9058. As a result of the findings, SYT13 and IL1F10 can be employed as diagnostic biomarkers with high prediction efficiency.
Figure 5 Potential biomarker panel to distinguish trauma and sepsis patients. Quantitative ELISA was used to determine the levels of A2M, IL1F10, SYT13, and TREM1 in trauma and sepsis patients at various time periods. The comparison of data was performed by one-way ANOVA, Tukey’s multiple comparison test and area under the curve (AUC) analysis were performed with 95% confidence interval, using Wilson/Brown method. Healthy (n=7); trauma ans sepsis (n=23).
To validate our results, we compared the relative expression levels of the four proteins with samples from healthy individuals at both early (day 0) and later time points (day 5). We set a minimum relative 2-fold shift in the expression levels as an assessment threshold. Using this setting, we measured clear distinctions between the biomarker profiles of trauma and sepsis patients. At the early time point, SYT13 and IL1F10 displayed a minimum 2-fold shift increase in expression levels in sepsis patients when compared to trauma patients (Figure 6A). Additionally, A2M showed a minimum 2-fold shift decrease in sepsis patients (Figure 6A). Further analysis focused on the correlation of these biomarkers within early sepsis samples. We observed that SYT13-positive samples showed a positive correlation with IL1F10 (Figure 6B left). Interestingly, IL1F10 positivity was also observed in SYT13-negative samples (Figure 4C right), suggesting the complexity of biomarker interactions in sepsis cases.
Figure 6 SYT13 and IL1F10 differentiate trauma and sepsis patients. (A) The levels of the proteins A2M, IL1F10, SYT13, and TREM1 at the early (day 0) and later stage (day 5) of the infection. (B) A comparison of the protein levels of SYT13 at high and low concentrations [+Ve; positive; from (B)] with those of A2M, IL1F10, and TREM1. (C) Comparison of the A2M, SYT13, and TREM1 and protein levels with the high and low protein levels of IL1F10 [+Ve; positive; from (B)]. Data are displayed as mean +/- SEM. Healthy (n=7); trauma and sepsis (n=23).
A similar analysis compared early sepsis samples with positive and negative IL1F10 2-fold shift outcomes. Our results showed that IL1F10-positive samples correlated positively with A2M while displaying limited associations with SYT13 or TREM1 (Figure 6C left). Conversely, TREM1-negative samples exhibited negligible correlations with other biomarkers (Figure 6C right).
To conclude, our research emphasizes the potential of A2M, IL1F10, SYT13, and TREM1 as a composite set of biomarkers for distinguishing between non-infected trauma and sepsis patients. Detailed information about the biological functions of the four proteins is provided in Table 3. The clear distinctions in their expression patterns offer a pathway for accurate and early diagnosis, necessitating further investigation to validate their clinical applicability.
In this study, we aimed at identifying a potential biomarker panel that can differentiate trauma-induced sterile inflammation from sepsis. Both emergencies constitute severe medical conditions with overlapping clinical manifestations, making their early and accurate differentiation challenging. To address this diagnostic problem, we employed advanced technologies, including Matrix-Assisted Laser Desorption/Ionization (MALDI) and multiplex antibody arrays (MAA), to profile the protein abundance in trauma and sepsis patients at different time points.
Our results revealed distinct protein patterns associated with the initial stages of trauma and sepsis. A2M, IL1F10, SYT13, and TREM1 emerge as compelling biomarkers for sepsis and trauma based on their distinct roles in immune response modulation. A2M is identified as a biomarker in trauma and sepsis cases, emphasizing its involvement in anti-inflammatory responses and tissue repair, making it a promising indicator for trauma-related diagnostics (60). IL1F10 belongs to the interleukin-1 (IL-1) family of cytokines (75) and in particular IL1F10 has been suggested being of diagnostic and prognostic value as clinical sepsis biomarker (76). The role of SYT13 in sepsis still unknown, whereas TREM1 is a crucial mediator of septic shock that acts by synergizing with Toll-like receptors (TLRs) to amplify the inflammatory responses to pathogens, thus promoting sepsis-induced immune dysregulation and organ dysfunction (74). While the levels of TREM1 are found up-regulated during the course of the disease we found a negative correlation for SYT13 and IL1F10 which implicate that their downregulation during the course of infection may negatively impact the host response to infection.
In trauma and sepsis patients, the inflammatory response appeared to differ, and complement and coagulation cascades were generally increased in sepsis patients compared to trauma patients. On the other hand, proteins involved in focal adhesion were mostly diminished in sepsis patients, indicating a possible difference in immune response between the two conditions.
Our findings suggest that a combination of multiple biomarkers will be more effective in distinguishing sepsis patients from those with trauma-induced sterile inflammation. Indeed, multidimensional variability reduces the accuracy of single biomarker assays (77). Utilizing a panel of biomarkers may enhance diagnostic accuracy and provide valuable insights for early prediction of infection and timely and discriminate use of antimicrobials in trauma patients. The identified biomarkers could aid in the development of novel diagnostic methods to enable rapid and accurate identification of trauma-induced sterile inflammation and sepsis, facilitating early intervention and improving patient outcomes.
Deciphering infection from non-infective Systemic Inflammatory Response Syndrome (SIRS) remains a challenging task. This is all the more true as infections contributes to tissue injury and injury predisposes to infection (78). It is therefore crucial to acknowledge that our study has specific limitations associated with the patient populations under investigation. Even if our patient sample size compares favorably with previous publications in the field (25, 79, 80), its modest number warrants further confirmation in larger, at best multicentric, cohorts of both trauma and sepsis patients.
While our study provides a promising proof of concept, further research is needed to validate these findings in larger patient cohorts and across different healthcare settings. The use of additional patient samples and validation studies will be crucial to establishing the utility and reliability of the identified biomarker panel. The aspect of gene polymorphism in patients reflects upon the response to infection, e.g interferon γ (81), making diagnostic and therapeutic considerations even more complex, though this has not been an aim of the present study.
It is worth noting that the trauma cohort included in our study consisted only of patients requiring mechanical ventilation for at least 18 hours (L21). This inclusion criterion may introduce a level of selection bias, as it focuses on a subset of trauma patients with more severe respiratory compromise. Therefore, the generalizability of our findings to the broader trauma patient population may be limited.
Also all septic patients in our study suffered from septic shock (L40,41), representing a more severe form of sepsis. While this selection facilitates the identification of septic patients, including those with a more critical condition, it may limit the extrapolation of our results to less severe septic cases. The biomarkers and mechanisms identified in patients with septic shock might differ from those in patients with milder forms of sepsis.
Moreover, it is essential to note that our primary focus was on identifying biomarkers in trauma patients who developed sepsis. The mechanisms and biomarkers associated with sepsis development following trauma may differ from those in patients who develop sepsis due to other underlying diseases, as described elsewhere in this manuscript. This distinction is crucial, as the heterogeneity of sepsis etiology could impact the generalizability of our findings to diverse septic patient populations.
Despite these specific patient population considerations, our study offers a valuable approach to distinguish trauma patients who develop sepsis from those who do not. The identified biomarkers show promise in aiding the early detection of infection in trauma patients. However, further research, including validation studies in larger and more diverse patient cohorts, is warranted to confirm the clinical utility and generalizability of the identified biomarker panel.
In conclusion, our proof-of-concept study highlights the potential of employing a biomarker panel for early differentiation of severely ill sepsis patients from those with trauma-induced sterile inflammation. By utilizing advanced technologies and exploring the inflammatory response in these patients, we identified specific proteins with diagnostic relevance. Implementing such a biomarker panel in clinical practice could significantly improve the early detection and management of infection in trauma patients, leading to better patient outcomes.
The datasets presented in this study can be found in online repositories. The names of the repository/repositories and accession number(s) can be found in the article/Supplementary Material.
The studies involving humans were approved by Ethics committee in Lund Ethics Committee of the Military University Hospital Prague French Agence Nationale de la Sécurité du Médicament et des Produits de Santé. The studies were conducted in accordance with the local legislation and institutional requirements. Written informed consent for participation was not required from the participants or the participants’ legal guardians/next of kin in accordance with the national legislation and institutional requirements. The studies were conducted in accordance with the local legislation and institutional requirements. The human samples used in this study were acquired from some of the co-authors involved in this study. Written informed consent for participation was not required from the participants or the participants’ legal guardians/next of kin in accordance with the national legislation and institutional requirements.
PP: Conceptualization, Data curation, Formal analysis, Funding acquisition, Investigation, Methodology, Project administration, Resources, Software, Validation, Writing – original draft, Writing – review & editing. MS: Formal analysis, Software, Validation, Writing – review & editing. NP: Resources, Writing – review & editing. VL: Resources, Writing – review & editing. BR: Resources, Writing – review & editing. JO: Resources, Writing – review & editing. CR: Resources, Writing – review & editing. EB: Resources, Writing – review & editing. MH: Resources, Writing – review & editing. KJ: Conceptualization, Writing – original draft, Writing – review & editing, Resources, Validation. JP: Conceptualization, Formal analysis, Resources, Validation, Writing – original draft, Writing – review & editing. HH: Conceptualization, Funding acquisition, Project administration, Supervision, Writing – original draft, Writing – review & editing.
The author(s) declare financial support was received for the research, authorship, and/or publication of this article. This work was supported in part by the Alfred Österlund Foundation (to PP and HH), the Crafoord Foundation (grant number 20180506 and 20210908 to PP), the Medical Faculty at Lund University (to HH), the Swedish Research Council (grant number 2019-01086 to HH), the Stig and Ragna Gorthon Foundation, Helsingborg to CR and JO). The funders had no role in study design, data collection and analysis, decision to publish, or preparation of the manuscript.
The Proteomics Core Facility at Sahlgrenska Academy, Gothenburg University, performed the analysis for protein identification. We are grateful to Inga-Britt and Arne Lundbergs Forskningsstiftlese for the donation of the Orbitrap Fusion Tribrid MS instrument.
The authors declare that the research was conducted in the absence of any commercial or financial relationships that could be construed as a potential conflict of interest.
The author(s) declared that they were an editorial board member of Frontiers, at the time of submission. This had no impact on the peer review process and the final decision.
All claims expressed in this article are solely those of the authors and do not necessarily represent those of their affiliated organizations, or those of the publisher, the editors and the reviewers. Any product that may be evaluated in this article, or claim that may be made by its manufacturer, is not guaranteed or endorsed by the publisher.
The Supplementary Material for this article can be found online at: https://www.frontiersin.org/articles/10.3389/fimmu.2023.1310271/full#supplementary-material
Supplementary Table 1 | Demographic and clinical data.
Supplementary Table 2 | Antibody microarray analysis data.
Supplementary Table 3 | MALDI-Tandem Mass Tag (TMT) analysis data.
1. Reinhart K, Bauer M, Riedemann NC, Hartog CS. New approaches to sepsis: molecular diagnostics and biomarkers. Clin Microbiol Rev (2012) 25:609–34. doi: 10.1128/CMR.00016-12
2. Allott NEH, Banger MS, Mcgregor AH. Evaluating the diagnostic pathway for acute ACL injuries in trauma centres: a systematic review. BMC Musculoskelet Disord (2022) 23:649. doi: 10.1186/s12891-022-05595-0
3. Raymond SL, Holden DC, Mira JC, Stortz JA, Loftus TJ, Mohr AM, et al. Microbial recognition and danger signals in sepsis and trauma. Biochim Biophys Acta Mol Basis Dis (2017) 1863:2564–73. doi: 10.1016/j.bbadis.2017.01.013
4. Balogh ZJ, Reumann MK, Gruen RL, Mayer-Kuckuk P, Schuetz MA, Harris IA, et al. Advances and future directions for management of trauma patients with musculoskeletal injuries. Lancet (2012) 380:1109–19. doi: 10.1016/S0140-6736(12)60991-X
5. Xiao W, Mindrinos MN, Seok J, Cuschieri J, Cuenca AG, Gao H, et al. A genomic storm in critically injured humans. J Exp Med (2011) 208:2581–90. doi: 10.1084/jem.20111354
6. Dobson GP, Morris JL, Letson HL. Immune dysfunction following severe trauma: A systems failure from the central nervous system to mitochondria. Front Med (Lausanne) (2022) 9:968453. doi: 10.3389/fmed.2022.968453
7. Calfee CS, Matthay MA. Clinical immunology: Culprits with evolutionary ties. Nature (2010) 464:41–2. doi: 10.1038/464041a
8. Wenceslau CF, Mccarthy CG, Szasz T, Goulopoulou S, Webb RC. Mitochondrial N-formyl peptides induce cardiovascular collapse and sepsis-like syndrome. Am J Physiol Heart Circ Physiol (2015) 308:H768–777. doi: 10.1152/ajpheart.00779.2014
9. Krebs ED, Hassinger TE, Guidry CA, Berry PS, Elwood NR, Sawyer RG. Non-utility of sepsis scores for identifying infection in surgical intensive care unit patients. Am J Surg (2019) 218:243–7. doi: 10.1016/j.amjsurg.2018.11.044
10. O'dell JC, Halimeh BN, Johnston J, Mccoy CC, Winfield RD, Guidry CA. Antibiotic initiation timing and mortality in trauma patients with ventilator-associated pneumonia. Am Surg (2022) 89:4740–6. doi: 10.1177/00031348221129518
11. Lee GR, Gallo D, Alves De Souza RW, Tiwari-Heckler S, Csizmadia E, Harbison JD, et al. Trauma-induced heme release increases susceptibility to bacterial infection. JCI Insight (2021) 6:e150813. doi: 10.1172/jci.insight.150813
12. Kim HI, Park J, Gallo D, Shankar S, Konecna B, Han Y, et al. DANGER signals activate G-protein receptor kinases suppressing neutrophil function and predisposing to infection after tissue trauma. Ann Surg (2023) 278:e1277-e1288. doi: 10.1097/SLA.0000000000005898
13. Hranjec T, Rosenberger LH, Swenson B, Metzger R, Flohr TR, Politano AD, et al. Aggressive versus conservative initiation of antimicrobial treatment in critically ill surgical patients with suspected intensive-care-unit-acquired infection: a quasi-experimental, before and after observational cohort study. Lancet Infect Dis (2012) 12:774–80. doi: 10.1016/S1473-3099(12)70151-2
14. Chung S, Choi D, Cho J, Huh Y, Moon J, Kwon J, et al. Timing and associated factors for sepsis-3 in severe trauma patients: A 3-year single trauma center experience. Acute Crit Care (2018) 33:130–4. doi: 10.4266/acc.2018.00122
15. Mas-Celis F, Olea-Lopez J, Parroquin-Maldonado JA. Sepsis in trauma: A deadly complication. Arch Med Res (2021) 52:808–16. doi: 10.1016/j.arcmed.2021.10.007
16. Raju R. Immune and metabolic alterations following trauma and sepsis - An overview. Biochim Biophys Acta Mol Basis Dis (2017) 1863:2523–5. doi: 10.1016/j.bbadis.2017.08.008
17. Tong Y, Zhang J, Fu Y, He X, Feng Q. Risk factors and outcome of sepsis in traumatic patients and pathogen detection using metagenomic next-generation sequencing. Can J Infect Dis Med Microbiol (2022) 2022:2549413. doi: 10.1155/2022/2549413
18. Renninger CH, Tedesco NS, Strelzow J. American academy of orthopaedic surgeons appropriate use criteria: prevention of surgical site infections after major extremity trauma. J Am Acad Orthop Surg (2023) 31:497–504. doi: 10.5435/JAAOS-D-22-00969
19. Harrell KN, Lee WB, Rooks HJ, Briscoe WE, Capote W, Dart BWT, et al. Early pneumonia diagnosis decreases ventilator-associated pneumonia rates in trauma population. J Trauma Acute Care Surg (2023) 94:30–5. doi: 10.1097/TA.0000000000003808
20. Prunet B, Bourenne J, David JS, Bouzat P, Boutonnet M, Cordier PY, et al. Patterns of invasive mechanical ventilation in patients with severe blunt chest trauma and lung contusion: A French multicentric evaluation of practices. J Intensive Care Soc (2019) 20:46–52. doi: 10.1177/1751143718767060
21. Yang YW, Wu CH, Tsai HT, Chen YR, Chang YP, Han YY, et al. Dynamics of immune responses are inconsistent when trauma patients are grouped by injury severity score and clinical outcomes. Sci Rep (2023) 13:1391. doi: 10.1038/s41598-023-27969-7
22. Ahmad HI, Jabbar A, Mushtaq N, Javed Z, Hayyat MU, Bashir J, et al. Immune tolerance vs. Immune resistance: the interaction between host and pathogens in infectious diseases. Front Vet Sci (2022) 9:827407. doi: 10.3389/fvets.2022.827407
23. Tsurumi A, Flaherty PJ, Que YA, Ryan CM, Mendoza AE, Almpani M, et al. Multi-biomarker prediction models for multiple infection episodes following blunt trauma. iScience (2020) 23:101659. doi: 10.1016/j.isci.2020.101659
24. Pierrakos C, Velissaris D, Bisdorff M, Marshall JC, Vincent JL. Biomarkers of sepsis: time for a reappraisal. Crit Care (2020) 24:287. doi: 10.1186/s13054-020-02993-5
25. Thair S, Mewes C, Hinz J, Bergmann I, Buttner B, Sehmisch S, et al. Gene expression-based diagnosis of infections in critically ill patients-prospective validation of the sepsisMetaScore in a longitudinal severe trauma cohort. Crit Care Med (2021) 49:e751–60. doi: 10.1097/CCM.0000000000005027
26. Abdelhamid SS, Scioscia J, Vodovotz Y, Wu J, Rosengart A, Sung E, et al. Multi-omic admission-based prognostic biomarkers identified by machine learning algorithms predict patient recovery and 30-day survival in trauma patients. Metabolites (2022) 12. doi: 10.3390/metabo12090774
27. Vincent JL, Pereira AJ, Gleeson J, Backer D. Early management of sepsis. Clin Exp Emerg Med (2014) 1:3–7. doi: 10.15441/ceem.14.005
28. Singer M, Deutschman CS, Seymour CW, Shankar-Hari M, Annane D, Bauer M, et al. The third international consensus definitions for sepsis and septic shock (Sepsis-3). JAMA (2016) 315:801–10. doi: 10.1001/jama.2016.0287
29. Gul F, Arslantas MK, Cinel I, Kumar A. Changing definitions of sepsis. Turk J Anaesthesiol Reanim (2017) 45:129–38. doi: 10.5152/TJAR.2017.93753
30. Taniguchi LU, Pires EMC, Vieira JM Jr., Azevedo LCP. Systemic inflammatory response syndrome criteria and the prediction of hospital mortality in critically ill patients: a retrospective cohort study. Rev Bras Ter Intensiva (2017) 29:317–24. doi: 10.5935/0103-507X.20170047
31. Lu HX, Du J, Wen DL, Sun JH, Chen MJ, Zhang AQ, et al. Development and validation of a novel predictive score for sepsis risk among trauma patients. World J Emerg Surg (2019) 14:11. doi: 10.1186/s13017-019-0231-8
32. Schefzik R, Hahn B, Schneider-Lindner V. Dissecting contributions of individual systemic inflammatory response syndrome criteria from a prospective algorithm to the prediction and diagnosis of sepsis in a polytrauma cohort. Front Med (Lausanne) (2023) 10:1227031. doi: 10.3389/fmed.2023.1227031
33. Iyengar KP, Venkatesan AS, Jain VK, Shashidhara MK, Elbana H, Botchu R. Risks in the management of polytrauma patients: clinical insights. Orthop Res Rev (2023) 15:27–38. doi: 10.2147/ORR.S340532
34. Ward PA. New approaches to the study of sepsis. EMBO Mol Med (2012) 4:1234–43. doi: 10.1002/emmm.201201375
35. Liesenfeld O, Lehman L, Hunfeld KP, Kost G. Molecular diagnosis of sepsis: New aspects and recent developments. Eur J Microbiol Immunol (Bp) (2014) 4:1–25. doi: 10.1556/EuJMI.4.2014.1.1
36. Duncan CF, Youngstein T, Kirrane MD, Lonsdale DO. Diagnostic challenges in sepsis. Curr Infect Dis Rep (2021) 23:22. doi: 10.1007/s11908-021-00765-y
37. Lakbar I, Singer M, Leone M. 2030: will we still need our microbiologist? Intensive Care Med (2023) 49:1232–4. doi: 10.1007/s00134-023-07186-6
38. Ludwig KR, Hummon AB. Mass spectrometry for the discovery of biomarkers of sepsis. Mol Biosyst (2017) 13:648–64. doi: 10.1039/C6MB00656F
39. Von Groote T, Meersch-Dini M. Biomarkers for the Prediction and Judgement of Sepsis and Sepsis Complications: A Step towards precision medicine? J Clin Med (2022) 11. doi: 10.3390/jcm11195782
40. Denny KJ, De Waele J, Laupland KB, Harris PNA, Lipman J. When not to start antibiotics: avoiding antibiotic overuse in the intensive care unit. Clin Microbiol Infect (2020) 26:35–40. doi: 10.1016/j.cmi.2019.07.007
41. Povoa P, Coelho L, Dal-Pizzol F, Ferrer R, Huttner A, Conway Morris A, et al. How to use biomarkers of infection or sepsis at the bedside: guide to clinicians. Intensive Care Med (2023) 49:142–53. doi: 10.1007/s00134-022-06956-y
42. Barichello T, Generoso JS, Singer M, Dal-Pizzol F. Biomarkers for sepsis: more than just fever and leukocytosis-a narrative review. Crit Care (2022) 26:14. doi: 10.1186/s13054-021-03862-5
43. Pottecher J, Noll E, Borel M, Audibert G, Gette S, Meyer C, et al. Protocol for TRAUMADORNASE: a prospective, randomized, multicentre, double-blinded, placebo-controlled clinical trial of aerosolized dornase alfa to reduce the incidence of moderate-to-severe hypoxaemia in ventilated trauma patients. Trials (2020) 21:274. doi: 10.1186/s13063-020-4141-6
44. Copes WS, Champion HR, Sacco WJ, Lawnick MM, Keast SL, Bain LW. The injury severity score revisited. J Trauma (1988) 28:69–77. doi: 10.1097/00005373-198801000-00010
45. Li Y, Liu C, Xiao W, Song T, Wang S. Incidence, risk factors, and outcomes of ventilator-associated pneumonia in traumatic brain injury: A meta-analysis. Neurocrit Care (2020) 32:272–85. doi: 10.1007/s12028-019-00773-w
46. Klompas M. Complications of mechanical ventilation–the CDC's new surveillance paradigm. N Engl J Med (2013) 368:1472–5. doi: 10.1056/NEJMp1300633
47. Calandra T, Cohen J, International Sepsis Forum Definition of Infection in The, I.C.U.C.C. The international sepsis forum consensus conference on definitions of infection in the intensive care unit. Crit Care Med (2005) 33:1538–48. doi: 10.1097/01.CCM.0000168253.91200.83
48. Olinder J, Borjesson A, Norrman J, West T, Carlstrom J, Gustafsson A, et al. Hepcidin discriminates sepsis from other critical illness at admission to intensive care. Sci Rep (2022) 12:14857. doi: 10.1038/s41598-022-18826-0
49. Olinder J, Ehinger D, Liljenborg E, Herwald H, Ryden C. Plasma levels of hepcidin and reticulocyte haemoglobin during septic shock. J Innate Immun (2020) 12:1232–4. doi: 10.1159/000508561
50. Moreno RP, Metnitz PG, Almeida E, Jordan B, Bauer P, Campos RA, et al. SAPS 3–From evaluation of the patient to evaluation of the intensive care unit. Part 2: Development of a prognostic model for hospital mortality at ICU admission. Intensive Care Med (2005) 31:1345–55. doi: 10.1007/s00134-005-2763-5
51. Papareddy P, Rossnagel M, Doreen Hollwedel F, Kilic G, Veerla S, Naudin C, et al. A human antithrombin isoform dampens inflammatory responses and protects from organ damage during bacterial infection. Nat Microbiol (2019) 4:2442–55. doi: 10.1038/s41564-019-0559-6
52. Wisniewski JR, Zougman A, Nagaraj N, Mann M. Universal sample preparation method for proteome analysis. Nat Methods (2009) 6:359–62. doi: 10.1038/nmeth.1322
53. Moreno R, Vincent JL, Matos R, Mendonca A, Cantraine F, Thijs L, et al. The use of maximum SOFA score to quantify organ dysfunction/failure in intensive care. Results of a prospective, multicentre study. Working Group on Sepsis related Problems of the ESICM. Intensive Care Med (1999) 25:686–96. doi: 10.1007/s001340050931
54. Innerhofer P, Fries D, Mittermayr M, Innerhofer N, Von Langen D, Hell T, et al. Reversal of trauma-induced coagulopathy using first-line coagulation factor concentrates or fresh frozen plasma (RETIC): a single-centre, parallel-group, open-label, randomised trial. Lancet Haematol (2017) 4:e258–71. doi: 10.1016/S2352-3026(17)30077-7
55. Weber GF, Chousterman BG, He S, Fenn AM, Nairz M, Anzai A, et al. Interleukin-3 amplifies acute inflammation and is a potential therapeutic target in sepsis. Science (2015) 347:1260–5. doi: 10.1126/science.aaa4268
56. Siskind S, Brenner M, Wang P. TREM-1 modulation strategies for sepsis. Front Immunol (2022) 13:907387. doi: 10.3389/fimmu.2022.907387
57. Wu F, Zhang YT, Teng F, Li HH, Guo SB. S100a8/a9 contributes to sepsis-induced cardiomyopathy by activating ERK1/2-Drp1-mediated mitochondrial fission and respiratory dysfunction. Int Immunopharmacol (2023) 115:109716. doi: 10.1016/j.intimp.2023.109716
58. Gyurkovska V, Ivanovska N. Distinct roles of TNF-related apoptosis-inducing ligand (TRAIL) in viral and bacterial infections: from pathogenesis to pathogen clearance. Inflammation Res (2016) 65:427–37. doi: 10.1007/s00011-016-0934-1
59. Dalli J, Norling LV, Montero-Melendez T, Federici Canova D, Lashin H, Pavlov AM, et al. Microparticle alpha-2-macroglobulin enhances pro-resolving responses and promotes survival in sepsis. EMBO Mol Med (2014) 6:27–42. doi: 10.1002/emmm.201303503
60. Kelly BJ, Lautenbach E, Nachamkin I, Coffin SE, Gerber JS, Fuchs BD, et al. Combined biomarkers discriminate a low likelihood of bacterial infection among surgical intensive care unit patients with suspected sepsis. Diagn Microbiol Infect Dis (2016) 85:109–15. doi: 10.1016/j.diagmicrobio.2016.01.003
61. Vandooren J, Itoh Y. Alpha-2-macroglobulin in inflammation, immunity and infections. Front Immunol (2021) 12:803244. doi: 10.3389/fimmu.2021.803244
62. Vlasakova K, Bourque J, Bailey WJ, Patel S, Besteman EG, Gonzalez RJ, et al. Universal accessible biomarkers of drug-induced tissue injury and systemic inflammation in rat: performance assessment of TIMP-1, A2M, AGP, NGAL, and albumin. Toxicol Sci (2022) 187:219–33. doi: 10.1093/toxsci/kfac030
63. Xu F, Lin S, Yan X, Wang C, Tu H, Yin Y, et al. Interleukin 38 protects against lethal sepsis. J Infect Dis (2018) 218:1175–84. doi: 10.1093/infdis/jiy289
64. Chai YS, Lin SH, Zhang M, Deng L, Chen Y, Xie K, et al. IL-38 is a biomarker for acute respiratory distress syndrome in humans and down-regulates Th17 differentiation in vivo. Clin Immunol (2020) 210:108315. doi: 10.1016/j.clim.2019.108315
65. Diaz-Barreiro A, Huard A, Palmer G. Multifaceted roles of IL-38 in inflammation and cancer. Cytokine (2022) 151:155808. doi: 10.1016/j.cyto.2022.155808
66. Fazeli P, Saeidnia M, Erfani M, Kalani M. An overview of the biological and multifunctional roles of IL-38 in different infectious diseases and COVID-19. Immunol Res (2022) 70:316–24. doi: 10.1007/s12026-022-09275-y
67. Tarquis-Medina M, Scheibner K, Gonzalez-Garcia I, Bastidas-Ponce A, Sterr M, Jaki J, et al. Synaptotagmin-13 is a neuroendocrine marker in brain, intestine and pancreas. Int J Mol Sci (2021) 22. doi: 10.3390/ijms222212526
68. Bakhti M, Bastidas-Ponce A, Tritschler S, Czarnecki O, Tarquis-Medina M, Nedvedova E, et al. Synaptotagmin-13 orchestrates pancreatic endocrine cell egression and islet morphogenesis. Nat Commun (2022) 13:4540. doi: 10.1038/s41467-022-31862-8
69. Zhang YD, Zhong R, Liu JQ, Sun ZX, Wang T, Liu JT. Role of synaptotagmin 13 (SYT13) in promoting breast cancer and signaling pathways. Clin Transl Oncol (2023) 25:1629–40. doi: 10.1007/s12094-022-03058-5
70. Bosco MC, Raggi F, Varesio L. Therapeutic potential of targeting TREM-1 in inflammatory diseases and cancer. Curr Pharm Des (2016) 22:6209–33. doi: 10.2174/1381612822666160826110539
71. Brenner T, Uhle F, Fleming T, Wieland M, Schmoch T, Schmitt F, et al. Soluble TREM-1 as a diagnostic and prognostic biomarker in patients with septic shock: an observational clinical study. Biomarkers (2017) 22:63–9. doi: 10.1080/1354750X.2016.1204005
72. Jolly L, Carrasco K, Salcedo-Magguilli M, Garaud JJ, Lambden S, van der Poll T, et al. sTREM-1 is a specific biomarker of TREM-1 pathway activation. Cell Mol Immunol (2021) 18:2054–6. doi: 10.1038/s41423-021-00733-5
73. Colonna M. The biology of TREM receptors. Nat Rev Immunol (2023) 23:580–94. doi: 10.1038/s41577-023-00837-1
74. Francois B, Lambden S, Fivez T, Gibot S, Derive M, Grouin JM, et al. Prospective evaluation of the efficacy, safety, and optimal biomarker enrichment strategy for nangibotide, a TREM-1 inhibitor, in patients with septic shock (ASTONISH): a double-blind, randomised, controlled, phase 2b trial. Lancet Respir Med (2023) 11:894–904. doi: 10.1016/S2213-2600(23)00158-3
75. Dinarello CA. Overview of the IL-1 family in innate inflammation and acquired immunity. Immunol Rev (2018) 281:8–27. doi: 10.1111/imr.12621
76. Ge Y, Huang M, Yao YM. Recent advances in the biology of IL-1 family cytokines and their potential roles in development of sepsis. Cytokine Growth Factor Rev (2019) 45:24–34. doi: 10.1016/j.cytogfr.2018.12.004
77. Cahill LA, Joughin BA, Kwon WY, Itagaki K, Kirk CH, Shapiro NI, et al. Multiplexed plasma immune mediator signatures can differentiate sepsis from nonInfective SIRS: american surgical association 2020 annual meeting paper. Ann Surg (2020) 272:604–10. doi: 10.1097/SLA.0000000000004379
78. Lei M, Han Z, Wang S, Guo C, Zhang X, Song Y, et al. Biological signatures and prediction of an immunosuppressive status-persistent critical illness-among orthopedic trauma patients using machine learning techniques. Front Immunol (2022) 13:979877. doi: 10.3389/fimmu.2022.979877
79. Wang J, Wen D, Zeng S, Du J, Cui L, Sun J, et al. Cytokine biomarker phenotype for early prediction and triage of sepsis in blunt trauma patients. J Surg Res (2023) 283:824–32. doi: 10.1016/j.jss.2022.10.059
80. Yang B, Wang X, Liu Z, Lu Z, Fang G, Xue X, et al. Endothelial-related biomarkers in evaluation of vascular function during progression of sepsis after severe trauma: new potential diagnostic tools in sepsis. J Inflammation Res (2023) 16:2773–82. doi: 10.2147/JIR.S418697
Keywords: sepsis, trauma, bacteremia, systemic inflammatory response syndrome, biomarkers, A2M, IL1F10, SYT13
Citation: Papareddy P, Selle M, Partouche N, Legros V, Rieu B, Olinder J, Ryden C, Bartakova E, Holub M, Jung K, Pottecher J and Herwald H (2024) Identifying biomarkers deciphering sepsis from trauma-induced sterile inflammation and trauma-induced sepsis. Front. Immunol. 14:1310271. doi: 10.3389/fimmu.2023.1310271
Received: 09 October 2023; Accepted: 22 December 2023;
Published: 12 January 2024.
Edited by:
Beate E. Kehrel, University Hospital Münster, GermanyReviewed by:
Karen Elizabeth Kempsell, UK Health Security Agency (UKHSA), United KingdomCopyright © 2024 Papareddy, Selle, Partouche, Legros, Rieu, Olinder, Ryden, Bartakova, Holub, Jung, Pottecher and Herwald. This is an open-access article distributed under the terms of the Creative Commons Attribution License (CC BY). The use, distribution or reproduction in other forums is permitted, provided the original author(s) and the copyright owner(s) are credited and that the original publication in this journal is cited, in accordance with accepted academic practice. No use, distribution or reproduction is permitted which does not comply with these terms.
*Correspondence: Heiko Herwald, aGVpa28uaGV3YWxkQG1lZC5sdS5zZQ==
Disclaimer: All claims expressed in this article are solely those of the authors and do not necessarily represent those of their affiliated organizations, or those of the publisher, the editors and the reviewers. Any product that may be evaluated in this article or claim that may be made by its manufacturer is not guaranteed or endorsed by the publisher.
Research integrity at Frontiers
Learn more about the work of our research integrity team to safeguard the quality of each article we publish.