- 1Department of Neurology, Xiangya Hospital, Central South University, Changsha, Hunan, China
- 2National Clinical Medical Research Center for Geriatric Diseases (Xiangya Hospital), Central South University, Changsha, Hunan, China
- 3Department of Pathology, First Hospital of Changsha, Changsha, Hunan, China
Background: Myasthenia gravis (MG) is an autoimmune disease observed to have connections with gut microbiome. We aimed to systematically assess the causal relationships between gut microbiome, gut microbiome-derived metabolites, and MG using Mendelian randomization (MR) approach.
Methods: Summary-level genetic datasets from large-scale genome-wide association studies regarding 196 gut microbial taxa from the MiBioGen consortium (n=18,340), 72 derived metabolites from the TwinsUK and KORA studies (n=7,824), and antiacetylcholine receptor (AChR) antibody-positive MG (case=1,873, control=36,370) were employed for MR causal estimates. The inverse-variance weighted (IVW) method was utilized as the main analysis with MR-Egger, maximum likelihood, simple mode, and weighted median as complements. The tests of Cochran’s Q, MR-Egger intercept, Steiger, MR-PRESSO and leave-one-out were implemented for sensitivity analyses.
Results: The forward MR estimates of IVW revealed significant causal associations of the abundance of phylum Actinobacteria, class Gammaproteobacteria, family Defluviitaleac, family Family XIII, and family Peptococcaceae with a reduced risk of MG. Conversely, the abundance of phylum Lentisphaerae, order Mollicutes RF9, order Victivallales, and genus Faecalibacterium was causally associated with an increased risk of MG. The reversed MR analysis proved negative causal correlations between the MG and the abundance of family Peptostreptococcaceae, genus Romboutsia, and genus Subdoligranulum. Regarding the derived metabolites, the IVW estimates revealed that elevated levels of beta-hydroxyisovalerate and methionine were causally associated with a decreased risk of MG, while increased levels of choline and kynurenine were linked to an increased risk of MG. Furthermore, genetically predicted MG was associated with a decreased level of cholesterol. The results obtained from complementary MR methods were similar. These findings remained robust in all sensitivity analyses.
Conclusion: Our MR findings support the causal effects of specific gut microbiome taxa and derived metabolites on AChR antibody-positive MG, and vice versa, yielding novel insights into prevention and therapy targets of MG. Future studies may be warranted for validation and pursuing the precise mechanisms.
Introduction
Myasthenia gravis (MG) is an autoimmune disorder characterized by muscle weakness and fatigue. It is mediated by B-cells and associated with the presence of antibodies targeting the acetylcholine receptor (AChR), muscle-specific kinase (MUSK), lipoprotein-related protein 4 (LRP4), or agrin in the postsynaptic membrane at the neuromuscular junction (1). MG has a reported worldwide prevalence ranging from 40 to 180 per million people, with an annual incidence of 4 to 12 per million people (2). The overall in-hospital mortality rate in a cohort from the United States was 2.2% (3). In European studies, the incidence rates of MG ranged from 0.63 to 2.9 per 100,000 person-years, and prevalence rates varied between 11.17 and 36.1 per 100,000 person-years (4). Over the years, there has been a notable increase in the prevalence rate, which can be attributed to factors such as improved diagnostic precision and treatments, prolonged survival, and an aging population (5).
The patients of MG may experience muscle weakness in the oculomotor, limb, pharyngeal, and respiratory muscles. In severe cases, it can result in respiratory failure (6). The pathogenesis of MG depends on the target and isotype of the autoantibodies directed against components of the postsynaptic muscular membrane. In most cases, autoantibodies against the AChR are recognized as diagnostic markers and pathogenic factors. These autoantibodies lead to complement-mediated attack and an increase in the rate of AChR turnover, causing the loss of AChR from the postsynaptic membrane and impairing neuromuscular transmission (7, 8).
While antibodies against components of the neuromuscular junction have been identified, the exact pathogenesis of MG still remains unclear, despite its well-known multifactorial nature. The disease’s occurrence and development depend on a complex interplay of genetic and environmental factors. Notably, disruptions in gut microbiome (dysbiosis) have been a subject of particular interest in recent years, especially with advancements in human microbiome research. Growing evidence suggests that the host microbiome and its metabolites play a significant role in the pathogenesis of autoimmune diseases, including MG (9–12).
The gut microbiome plays a crucial role in maintaining the integrity of the intestinal epithelial tight junctions, thus regulating intestinal permeability. Disruptions in its composition or function can often result in increased intestinal permeability, heightening the risk of pathogenic infections and affecting Foxp3+CD4+Treg cells, thereby contributing to the development of MG (13–15). Furthermore, the gut microbiome produces a variety of metabolites, including short-chain fatty acids (SCFAs), which can modulate the T cell-proinflammatory Th17 cell axis for immune regulation and pathogen protection (13, 16). Lower levels of SCFAs are considered to be a contributing factor to the observed immune response in MG patients (17–19).
The connections between gut microbiome and MG have been established, yet the precise causal links remain elusive. Numerous studies have delved into the causality between gut microbiome and MG, primarily through case-control investigations (9). However, due to the impact of environmental factors, demographic differences and so on, the resulting conclusions exhibit variability, posing a challenge in ascertaining whether alterations in gut microbiome composition precede the onset of MG or vice versa. The genome-wide association study (GWAS) approach has demonstrated remarkable success in the identification of novel associations between genetic variants and traits, thereby facilitating the exploration of previously unknown biological mechanisms. Recently, Chia R et al. have undertaken a GWAS that included 1,873 individuals diagnosed with AChR antibody-positive MG and 36,370 healthy controls to pinpoint genetic loci influencing the risk of MG and contributing to disease susceptibility, bringing novel genetic evidence to the pathogenesis of MG (20).
Mendelian randomization(MR) is a robust and effective method using genetic variants of single-nucleotide polymorphisms (SNPs) to explore the causal effects between exposure and outcome (21). Based on the stochastic principles governing meiosis, SNPs undergo random assortment during zygote formation throughout gestation. Consequently, the outcomes of MR analyses remain impervious to reverse causation and confounding effects (22). Previous investigations utilizing MR approach have established causal links between the gut microbiome and various autoimmune and neurological disorders, encompassing type 1 diabetes, Graves’ disease, IgA nephropathy, Sjogren’s syndrome, ischemic stroke, Parkinson’s Disease, epilepsy, and migraine (23–30). However, the causal associations between gut microbiome, gut microbiome-derived metabolites, and MG have yet to be firmly established. Hence, we have undertaken a bidirectional MR study to systematically assess the underlying causalities.
Methods
Study design
Summary-level genetic datasets from large-scale GWASs of gut microbiome, gut microbiome-derived metabolites, and MG were employed for MR causal estimates. To ensure the validity of genetic variants as instrumental variables (IVs), three fundamental conditions of MR analysis must be met: firstly, the selected IVs should exhibit robust associations with the exposure; secondly, they should not be associated with any confounders of exposure or outcome; finally, the selected IVs should solely influence the outcome through their impact on exposure (21). Initially, we assessed the causal impact of gut microbiome and derived metabolites on MG in the forward analysis. Subsequently, we performed a reverse evaluation regarding the causal effect of MG on alterations in gut microbiome and derived metabolites. The flowchart of the study is depicted in Figure 1.
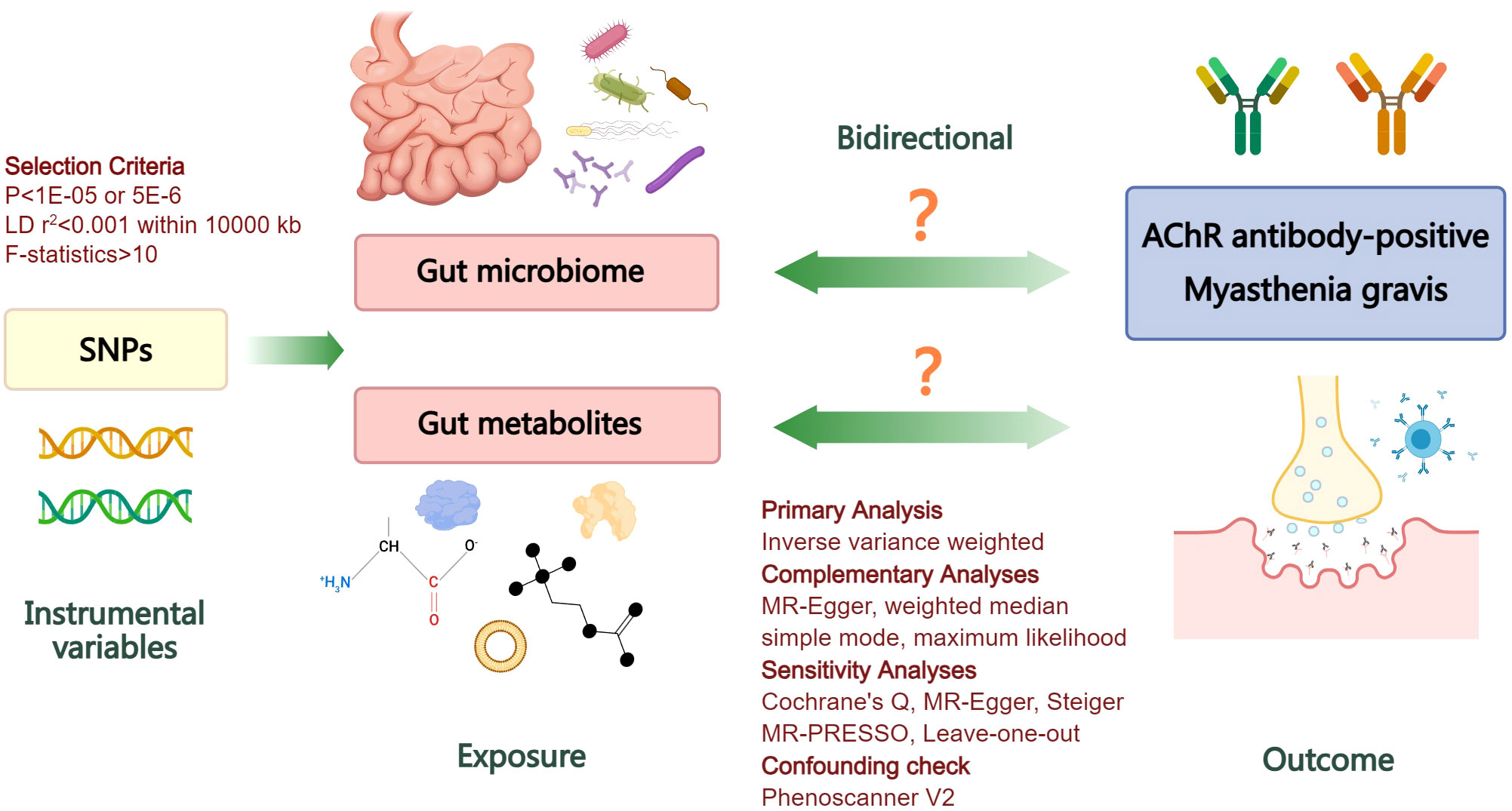
Figure 1 Flowchart illustrating the bidirectional MR study design for investigating the causal associations between gut microbiome, derived metabolites, and myasthenia gravis. LD, linkage disequilibrium; SNPs, single nucleotide polymorphisms; AChR, acetylcholine receptor; MR, Mendelian randomization.
Data source of gut microbiome
The summary-level dataset for the gut microbiome was obtained from a large-scale GWAS conducted by the MiBioGen consortium (31). This dataset, which is currently the largest genetics research of the human gut microbiome, comprises 18,340 samples collected from 24 diverse cohorts with specifical sequencing of the 16S ribosomal RNA gene. The majority of participants in these cohorts were of European ancestry. To ensure reproducibility, the microbial taxa were profiled by targeting three distinct variable regions of the 16S rRNA gene including V4 from 10,413 samples, 13 cohorts; V3-V4 from 4,211 samples, 6 cohorts; and V1-V2 from 3,716 samples, 5 cohorts. All gut microbial taxa datasets were rarefied to a standardized read count of 10,000 reads per sample. The data encompassed 211 taxa with mean abundance over 1%, including 131 genera, 35 families, 20 orders, 16 classes, and 9 phyla. Covariates such as sex, age, technical variables, and principal components were adjusted in all cohorts. For more accuracy, a total of 196 microbial taxa were included in our MR analyses, with the exclusion of 15 unidentified taxa (12 genera and 3 families) (Supplementary Table S1). More detailed information about this GWAS can be found in the original literature (31). The GWAS data could be obtained at https://mibiogen.gcc.rug.nl.
Data source of gut microbiome-derived metabolites
Considering the crucial roles of metabolites in microbiome-host communication, we further employed summary-level data from a GWAS that investigated the human blood metabolome among 7,824 European participants from the TwinsUK and KORA cohorts in our current investigation (32). We conducted a manual screening for gut microbiome-derived metabolites utilizing the Human Metabolome Database (HMDB, https://hmdb.ca/). Overall, our MR analyses incorporated a comprehensive list of 72 derived metabolite traits identified through HMDB (Supplementary Table S2).
Data source of myasthenia gravis
The dataset for MG was obtained from the largest recently published GWAS (20), which was conducted in cohorts from the United States and Italy, and subsequently replicated in an independent cohort from the United Kingdom. The diagnosis of MG was based on standard clinical criteria of characteristic fluctuating weakness and electrophysiological and/or pharmacological abnormalities. The presence of AChR antibodies was confirmed in all enrolled cases, as it can be detected in about 90% of patients with generalized MG. For more accuracy, patients positive for MUSK antibodies were excluded. This GWAS included a total of 1,873 cases and 36,370 controls. Among the cases, there were 989 males and 884 females with MG, while the control cohorts were well matched to the case cohorts based on ethnic group. More details of demographic profiles could be found in the original GWAS. The dataset for MG provided access to 23,679,120 SNPs, which could be obtained from IEU OpenGWAS project at https://gwas.mrcieu.ac.uk/.
Instrumental variables selection
SNPs genetically predisposed to gut microbiome, derived metabolites, and MG were selected as IVs. The IVs had to meet specific criteria, including being free of linkage disequilibrium (pairwise r2<0.001 within 10000 kb), using the European population as a reference. We used a threshold of P<1E-05 for IV selection of gut microbiome and derived metabolites, and P<5E-06 for IV selection of MG to increase the number of SNPs available for sensitivity analyses (30). SNPs with a minor allele frequency less than 0.01 were excluded due to potentially low confidence. In order to mitigate the impact of weak genetic instruments, all SNPs selected as IVs analyzed in this study had a minimum F-statistic of 10. The directions of effects of SNPs on exposures and outcomes were then harmonized, with the exception of palindromic SNPs with intermediate allele frequencies. To minimize the correlation between SNPs and confounders, the PhenoScanner was utilized to check all IVs and the SNPs associated with potential confounding factors were removed (The detailed information of all IVs is listed in Supplementary Tables S3-S9).
Mendelian randomization analyses and statistics
For the forward MR analysis, the gut microbiome and derived metabolites were chosen as the exposure traits for IVs selection with MG being considered as the outcome. Conversely, reverse MR analysis utilizing SNPs correlated with MG was performed to examine whether MG had causal impacts on gut microbial taxa and derived metabolites. The inverse-variance weighted (IVW) method, widely recognized as the most robust approach in MR studies for generating reliable causal estimates, was employed as the primary analytical tool to estimate the odds ratio (OR) and P-value in both forward and reverse directions of MR analyses. Additionally, complementary methods such as MR-Egger, maximum likelihood, simple mode, and weighted median were utilized (33). All statistical analyses were performed using the TwoSampleMR package (Version 0.5.7) in the R studio (Version 2023.06.0). Considering the exploratory nature of the study, we adopted not to perform Bonferroni correction. The significance threshold was set at a level of P<0.05.
Sensitivity analyses
To test the robustness of the MR analyses in both forward and reverse directions. Cochran’s Q and MR-Egger intercept tests were employed to assess the presence of heterogeneity and pleiotropy among the IVs. The Steiger test was utilized to validate the accurate directions of SNPs. The Mendelian randomization pleiotropy residual sum and outlier (MR-PRESSO) in conjunction with leave-one-out analysis, were performed to identify potential outliers among the IVs. If any SNP outliers were detected, they were removed and the MR and sensitivity analyses would be re-executed (34).
Results
Causal effects of the gut microbiome on myasthenia gravis
The IVW estimates in forward MR analysis revealed significant causal associations between specific microbial taxa and a diminished risk of MG. These associations encompassed the phylum Actinobacteria (OR=0.60, 95% CI: 0.40-0.90, P=0.012), class Gammaproteobacteria (OR=0.59, 95% CI: 0.36-0.97, P=0.037), family Defluviitaleaceae (OR=0.70, 95% CI: 0.49-1.00, P=0.047), family Family XIII (OR=0.61, 95% CI: 0.41-0.92, P=0.017), and family Peptococcaceae (OR=0.70, 95% CI: 0.51-0.96, P=0.029). The application of the maximum likelihood method yielded consistent results across all five microbial taxa. Specifically, the phylum Actinobacteria (OR=0.61, 95% CI: 0.40-0.91, P=0.016), class Gammaproteobacteria (OR=0.58, 95% CI: 0.35-0.98, P=0.040), family Defluviitaleaceae (OR=0.69, 95% CI: 0.49-0.96, P=0.028), family Family XIII (OR=0.61, 95% CI: 0.41-0.92, P=0.018), and family Peptococcaceae (OR=0.69, 95% CI: 0.49-0.97, P=0.034) exhibited analogous causal associations with MG as IVW. Additionally, the weighted median method provided evidence supporting a causal association between Family XIII and MG (OR=0.54, 95% CI: 0.32-0.92, P=0.023).
Conversely, certain microbial taxa, including the phylum Lentisphaerae (OR=1.32, 95% CI: 1.03-1.68, P=0.026), order Mollicutes RF9 (OR=1.42, 95% CI: 1.01-2.00, P=0.041), order Victivallales (OR=1.31, 95% CI: 1.01-1.69, P=0.044), and genus Faecalibacterium (OR=1.76, 95% CI: 1.22-2.55, P=0.003), were identified as risk-increasing factors for MG according to the IVW estimates. The maximum likelihood estimates confirmed the consistent correlations between these microbial taxa and the risk of MG, including the phylum Lentisphaerae (OR=1.33, 95% CI: 1.03-1.71, P=0.028), order Mollicutes RF9 (OR=1.45, 95% CI: 1.02-2.05, P=0.039), order Victivallales (OR=1.31, 95% CI: 1.01-1.72, P=0.045), and genus Faecalibacterium (OR=1.80, 95% CI: 1.22-2.67, P=0.003). Notably, the simple mode method also further supported an elevated risk for the genus Faecalibacterium (OR=3.02, 95% CI: 1.39-6.57, P=0.023) with MG. Significant MR results are presented in Table 1. The summary of causal estimates regarding the impact of all gut microbial taxa on MG is presented in Figure 2. Scatter plots illustrating the significant causal effects are shown in Figure 3.
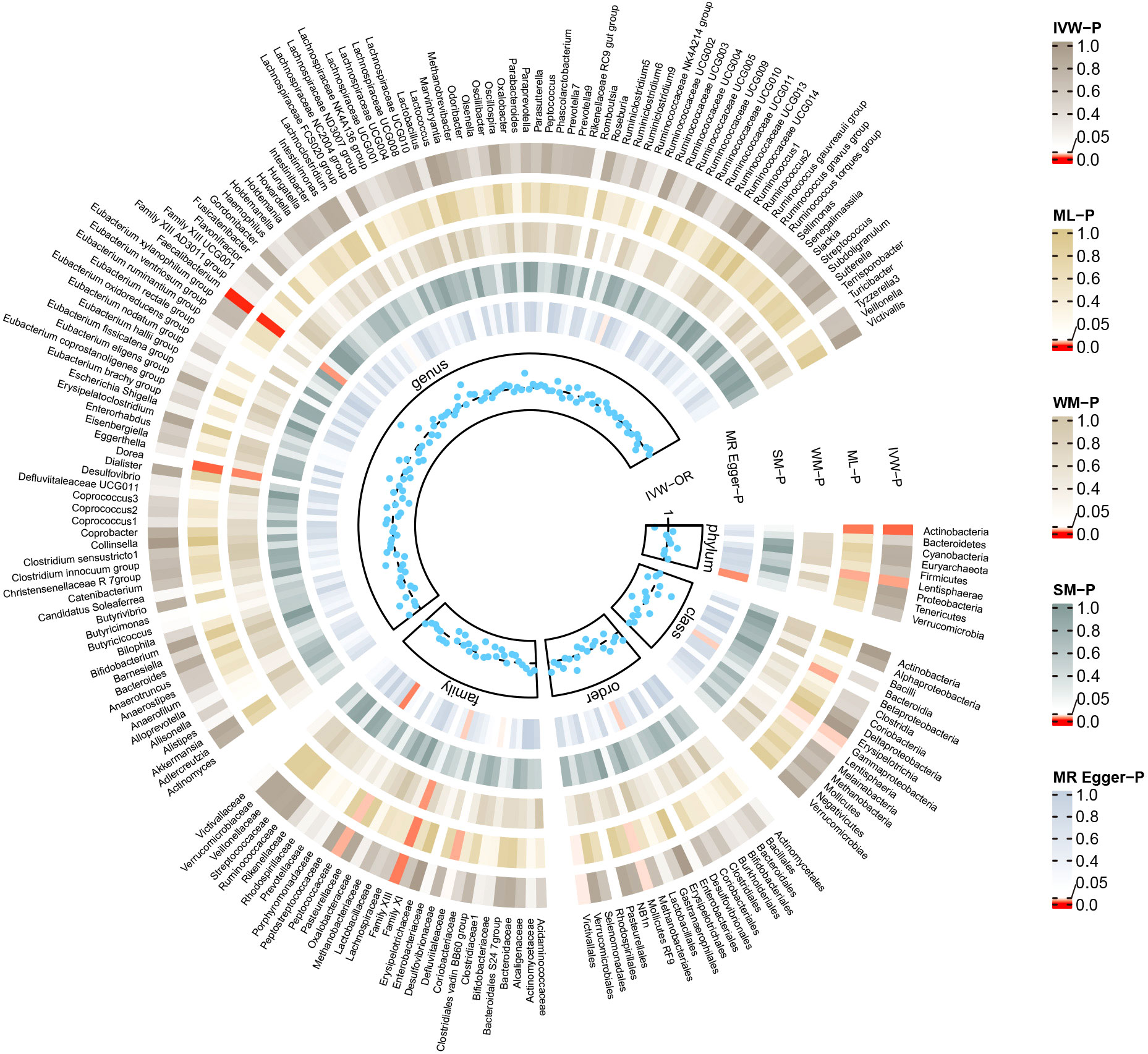
Figure 2 Summary of causal estimates regarding the impact of all gut microbiome on myasthenia gravis in forward MR analysis. From outside to inside, the corresponding P-values of IVW, ML, WM, SM, MR-Egger are represented, respectively. MR, mendelian randomization; IVW, inverse-variance weighted; ML, maximum likelihood; WM, weighted median; SM, simple mode; OR, odds ratio.
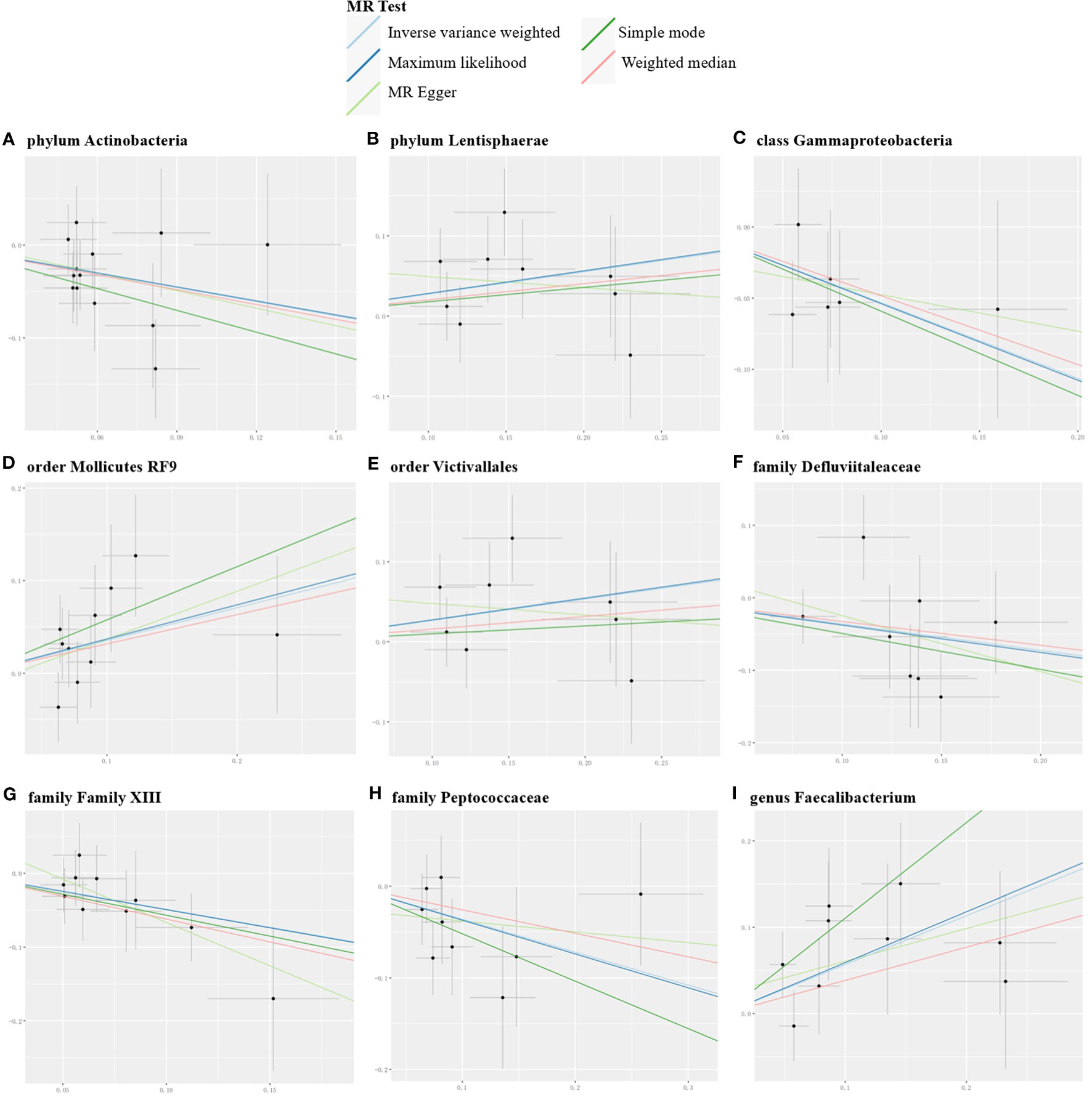
Figure 3 Scatter plots for the significant causal effects of gut microbiome (abscissas) on myasthenia gravis (ordinate) in forward MR analysis (A–I). The presence of a positive slope signifies a positive causal association, and vice versa.
Causal effects of the myasthenia gravis on gut microbiome
The reverse MR analysis revealed causal effects of MG on alterations in multiple gut microbiome compositions. According to the IVW estimates, the family Peptostreptococcaceae (OR=0.97, 95%CI: 0.95-0.99, P=0.015), genus Romboutsia (OR=0.97, 95%CI: 0.95-1.00, P=0.044), and genus Subdoligranulum (OR=0.97, 95%CI: 0.95-0.99, P=0.015) exhibited inverse causal associations with the onset of MG. Maximum likelihood estimates in the family Peptostreptococcaceae (OR=0.97, 95%CI: 0.95-0.99, P=0.015), genus Romboutsia (OR=0.97, 95%CI: 0.95-1.00, P=0.044), and genus Subdoligranulum (OR=0.97, 95%CI: 0.95-0.99, P=0.014) further supported these causalities. The weighted median method also demonstrated a consistent trend with IVW in the family Peptostreptococcaceae (OR=0.96, 95%CI: 0.93-1.00, P=0.035). Significant MR results are presented in Table 2. The summary of causal estimates regarding the impact of MG on all gut microbial taxa is presented in Figure 4. Scatter plots illustrating the significant causal effects are shown in Figure 5. Forest plots illustrating the significant causal estimates of the five methods in bidirectional MR are presented in Figure 6.
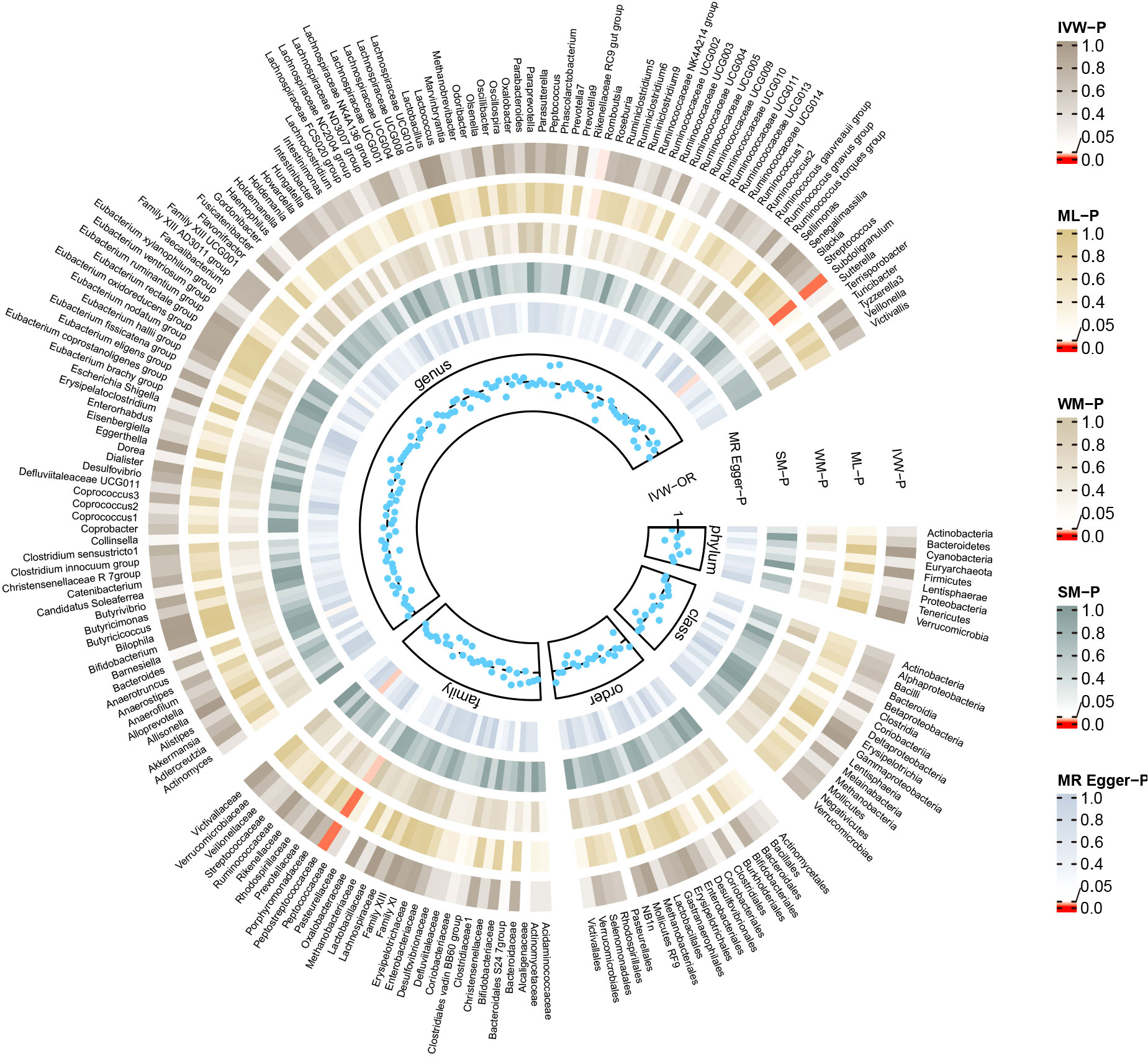
Figure 4 Summary of causal estimates regarding the impact of myasthenia gravis on all gut microbiome in reverse MR analysis. From outside to inside, the corresponding P-values of IVW, ML, WM, SM, MR-Egger are represented, respectively. MR, mendelian randomization; IVW, inverse-variance weighted; ML, maximum likelihood; WM, weighted median; SM, simple mode; OR, odds ratio.
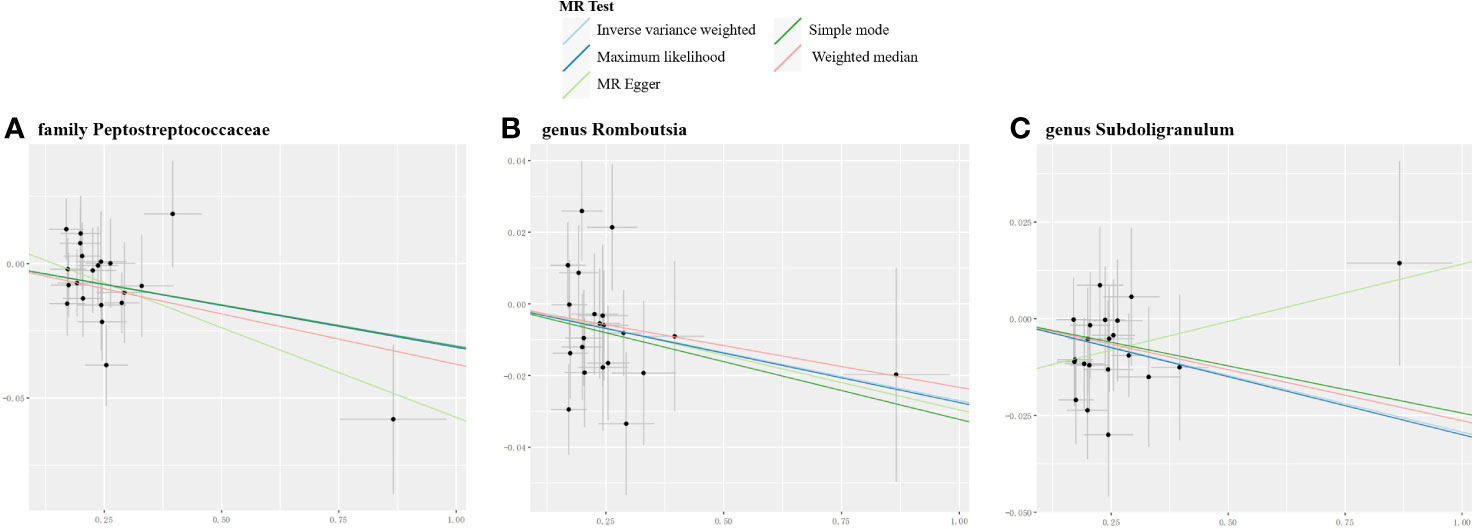
Figure 5 Scatter plots for the significant causal effects of myasthenia gravis (abscissas) on gut microbiome (A–C) (ordinate) in reverse MR analysis. The presence of a positive slope signifies a positive causal association, and vice versa.
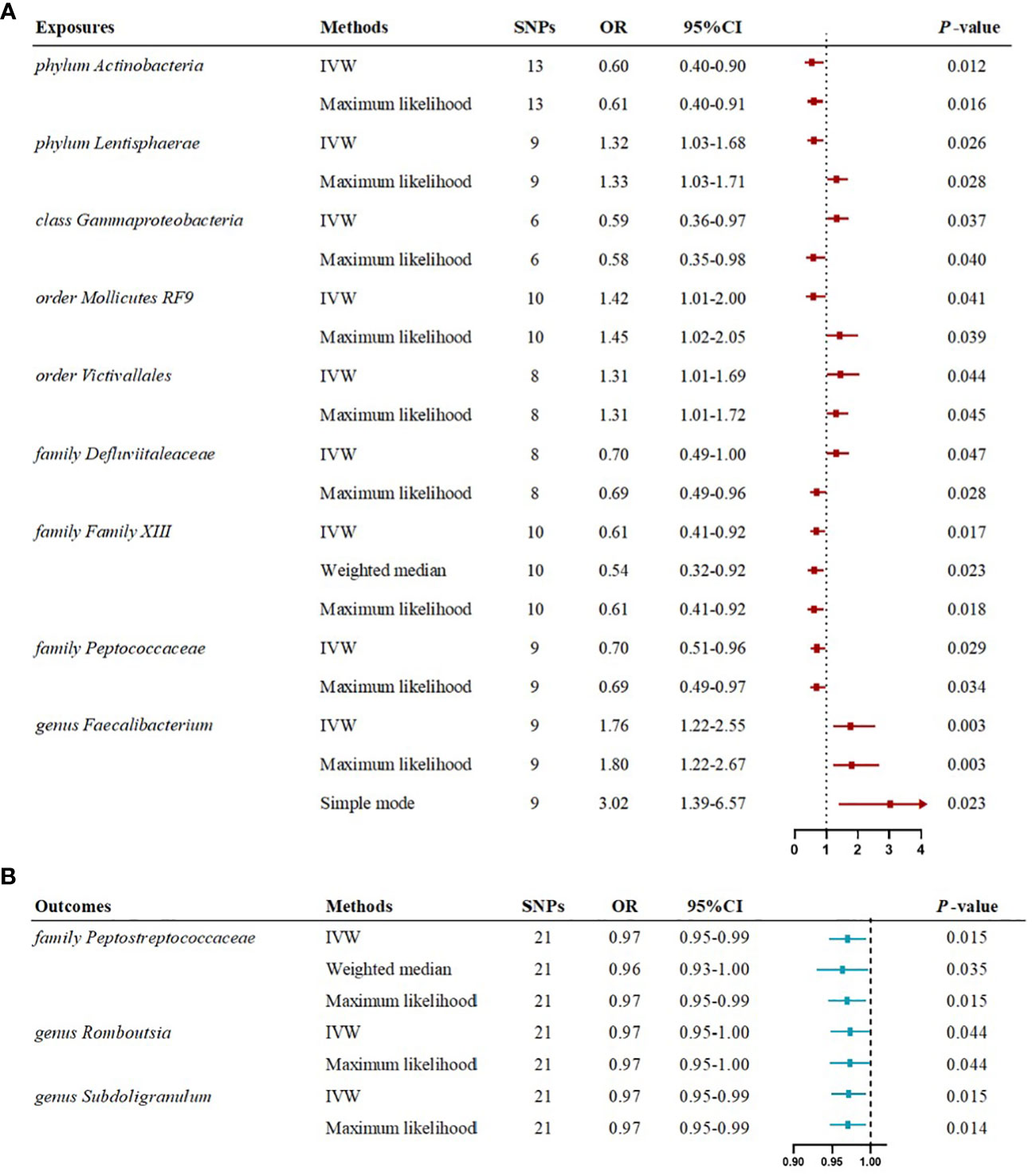
Figure 6 Forest plots illustrating the significant causal estimates of gut microbiome on myasthenia gravis (A), and myasthenia gravis on gut microbiome (B) are presented. nSNPs, number of single nucleotide polymorphisms; OR, odds ratio; IVW, inverse-variance weighted.
Causal effects of gut microbiome-derived metabolites on myasthenia gravis
For derived metabolites, the IVW estimates in forward MR analysis revealed that the levels of beta-hydroxyisovalerate (OR=0.18, 95%CI: 0.06-0.52, P=0.002) and methionine (OR=0.04, 95%CI: 0.00-0.53, P=0.014) were inversely correlated with the risk of MG, while choline (OR=50.01, 95%CI: 2.82-885.73, P=0.008) and kynurenine (OR=5.91, 95%CI: 1.55-22.62, P=0.009) were positively correlated with the risk of MG. Consistent results were obtained using the maximum likelihood method: beta-hydroxyisovalerate (OR=0.18, 95%CI: 0.06-0.57, P=0.003), methionine (OR=0.04, 95%CI: 0.00-0.63, P=0.022), choline (OR=59.20, 95%CI: 4.33-808.53, P=0.002), and kynurenine (OR=6.33, 95%CI: 1.80-22.27, P=0.004). Weighted median estimates also indicated negative correlations for methionine (OR=0.02, 95%CI: 0.00-0.86, P=0.041) and a positive correlation for kynurenine (OR=8.72, 95%CI: 1.38-55.32, P=0.022) with the risk of MG. Significant MR results are presented in Table 3. Scatter plots illustrating the aforementioned significant causal effects are shown in Figures 7A–D.
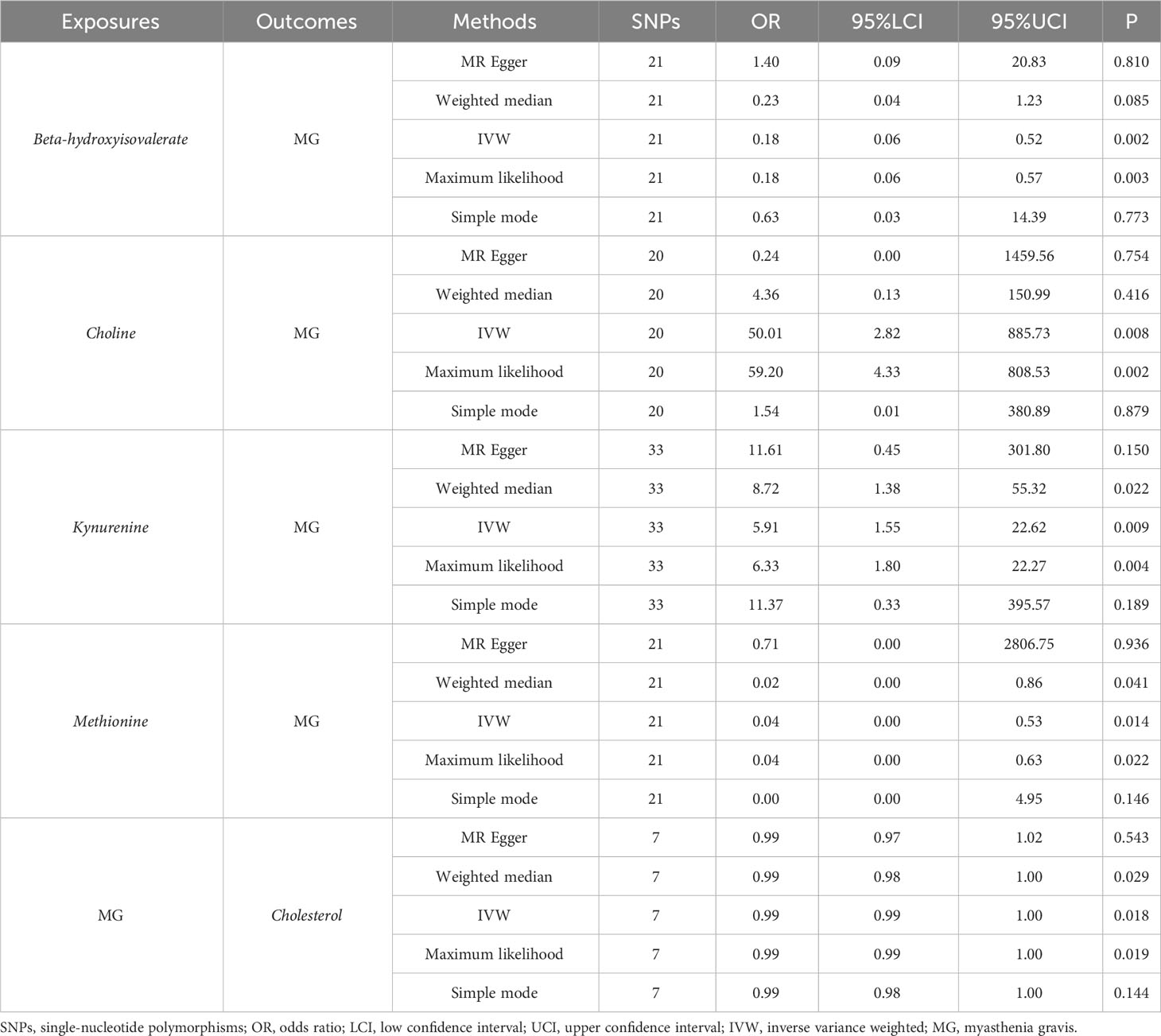
Table 3 Significant MR results elucidating the causal effects between gut-derived metabolites and MG.
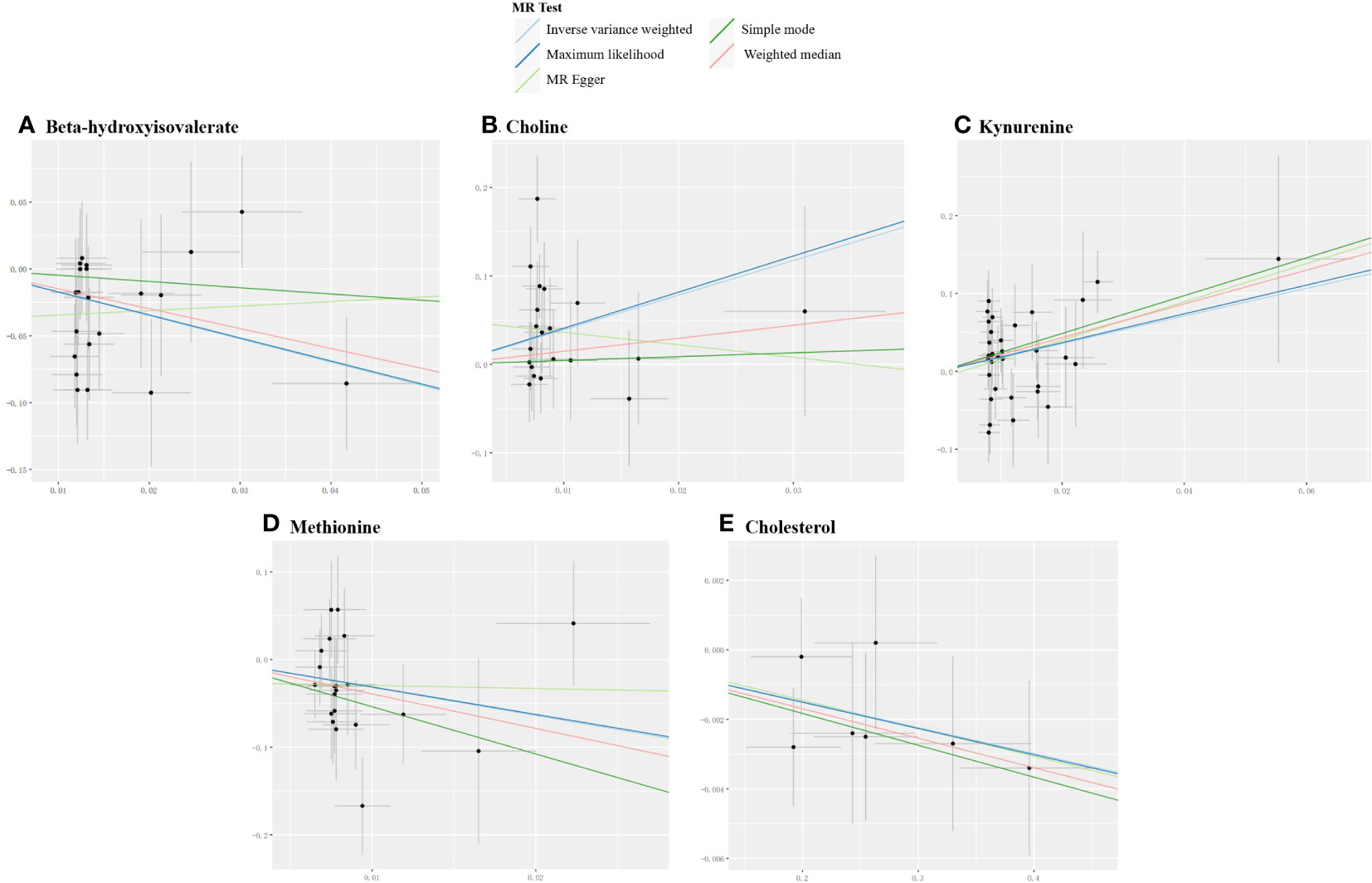
Figure 7 Scatter plots for the significant causal effects between gut microbiome-derived metabolites and myasthenia gravis. (A–D) depict the forward MR analysis, with metabolites as the exposures (abscissas) and myasthenia gravis as the outcome (ordinate). (E) presents the reverse MR analysis, with myasthenia gravis as the exposure (abscissas) and cholesterol as the outcome (ordinate). The presence of a positive slope signifies a positive causal association, and vice versa.
Causal effects of myasthenia gravis on gut microbiome-derived metabolites
In the reverse IVW estimates, it was observed that MG was associated with a decreased level of cholesterol (OR=0.99, 95%CI: 0.99-1.00, P=0.018). This finding was consistent when employing the maximum likelihood (OR=0.99, 95%CI: 0.99-1.00, P=0.019) and weighted median (OR=0.99, 95%CI: 0.98-1.00, P=0.029) methods. The result is presented in Table 3 and Figure 7E.
Sensitivity analyses
To conduct sensitivity analyses, we confirmed the validity of IVs and MR model assumptions using Cochran’s Q and MR-Egger tests. Both the forward and reverse analyses revealed no evidence of heterogeneity or pleiotropy among IVs. The Steiger test provided evidence supporting the correctness of SNP directions. Furthermore, both MR-PRESSO and leave-one-out analyses provided robust support for our results by detecting no potential instrumental outliers. The summary of sensitivity analyses can be found in Supplementary Tables S10-S12. The plots illustrating leave-one-out analysis are presented in Supplementary Figures S1-S3.
Discussion
We have conducted a comprehensive MR investigation to unravel the bidirectional causal association between the gut microbiome, derived metabolites, and AChR antibody-positive MG. Our findings unveiled a reciprocal interplay between the gut microbiome, derived metabolites and MG aligning with prior observational studies and emphasizing the pathological and physiological dynamics between them, presenting innovative insights, and indicating distinctive interactions between specific gut microbial taxa, derived metabolites and MG.
Our results underscored notable inverse causal effects on MG for the phylum Actinobacteria, class Gammaproteobacteria, family Defluviitaleaceae, Family XIII, and Peptococcaceae, implying a protective influence. Conversely, the phylum Lentisphaerae, order Victivallales, order Mollicutes RF9, and genus Faecalibacterium exerted significant positive causal impacts on MG, signifying potential risk factors.
The study conducted by Zheng et al. (35) demonstrated a reduced relative abundance of Actinobacteria in patients with MG compared to the healthy control group. Actinobacteria, one of the four major phyla of the gut microbiome, predominantly include Bifidobacteria in the human gut. Bifidobacteria are known for their beneficial effects on maintaining gut barrier integrity, attributed to their substantial production of SCFAs (4). Reduced levels of Bifidobacteria have been linked to increased gut permeability, leading to the translocation of lipopolysaccharide into the bloodstream. This event triggers immune system activation and contributes to the sustenance of chronic inflammatory conditions (36). In the older population, probiotics containing Bifidobacteria have been shown to improve constipation and enhance cellular immune activity (37), aligning with the findings of our study. Our research results are also in line with those of Sun et al. (38), who observed that nuciferine could enhance intestinal barrier functions, regulate bile acids metabolism, and alleviate inflammation in non-alcoholic fatty liver disease patients. The proposed mechanism of nuciferine metabolism involves the contribution of Family XIII, which was closely associated with specific bile acids monomers by Spearman rank correlation analysis. Interestingly, our study revealed a negative association between Gammaproteobacteria and the risk of MG. This finding contrasts with the results of Qiu et al. (18), who identified a significant enrichment in Proteobacteria. Furthermore, our study first highlighted the protective roles of the bacterial families Defluviitaleaceae and Peptococcaceae, expanding the spectrum of gut microbiome that warrants investigation in the context of MG.
The MYBIOM study, conducted in Germany, revealed an elevated abundance of Faecalibacterium in the fecal microbiome of individuals with MG compared to controls with non-inflammatory diseases, aligning with our findings (39). Faecalibacterium is known for its capacity to generate a substantial proportion of the SCFA butyrate in the intestinal milieu (40). This particular fatty acid elicits anti-inflammatory effects by activating Treg cells and enhancing the secretion of anti-inflammatory cytokines. Noteworthy is the paradoxical higher relative abundance of Faecalibacterium in MG patients, despite the disorder’s characteristic reduction in Treg cells. This may be due to the fact that the vast majority of the MG population ingests corticosteroid medications, which may affect the gut microbiome. The underlying mechanisms of this observation necessitate further exploration in subsequent research endeavors. In this MR study, intriguing associations have surfaced. Specifically, the abundance of phylum Lentisphaerae, order Mollicutes RF9, and order Victivallales exhibited correlations with the risk of MG. While prior investigations have linked Mollicutes RF9 to serum lipid levels and blood glucose in stroke patients (41), there is a dearth of research exploring the direct relationship between the phylum Lentisphaerae, order Victivallales, and MG. Subsequent research endeavors should delve into elucidating the mechanistic impact of these microbial taxa on MG, providing promising avenues for future insights.
Similarly, the onset of MG led to notable changes in gut microbiome composition. Following genetically predicted MG onset, there was a significant reduction in the abundance of the family Peptostreptococcaceae, genus Romboutsia, and genus Subdoligranulum. Notably, Chriswell ME et al.’s research established an association between a specific intestinal bacterium in the genus Subdoligranulum and various immunological phenomena, including local intestinal isolated lymphoid follicle formation, T cell and rheumatoid arthritis-associated autoantibody development, as well as paw swelling characterized by IgG, IgA, and C3 deposition in mice models (42). The family Peptostreptococcaceae has correlations with various malignancies, such as non-small cell lung cancer (43), and oral squamous cell carcinoma (44). Conversely, the genus Romboutsia is associated with metabolic disorders, particularly obesity, showing a positive correlation with parameters indicative of body weight and serum lipid levels (45). These potential mechanisms are linked to bacterial metabolism, such as producing SCFAs like butyrate and propionate, thereby fostering Treg development and functionality.
We have taken a step further to explore the causal associations between gut microbiome-derived metabolites and MG. The findings revealed that genetically predicted beta-hydroxyisovalerate and methionine demonstrated significant inverse causal effects on MG, suggesting protective factors. Conversely, choline and kynurenine exerted notable causal influences on MG, representing risk factors. A randomized controlled trial revealed that diets rich in phytochemicals and dietary fiber could enhance the abundance of beta-hydroxyisovalerate in stool samples from colorectal cancer survivors, positively modulating the patients’ gut metabolome (46). This modulation may play a significant role in MG as well. Methionine-choline deficiency can lead to abnormal mitochondrial β-oxidation function, a decrease in very low-density lipoprotein synthesis, exacerbation of inflammation, and promotion of early liver fibrosis (47). However, our MR study found that choline, which contradicts the previous consensus in nonalcoholic steatohepatitis, represented a risk factor for MG. A recent literature demonstrated that elevated concentrations of choline and the resulting increased plasma levels of trimethylamine N-oxide have been proven to be associated with heightened abundance of trimethylamine-producing bacteria and the development of cardiovascular and neurological diseases (48). Moreover, our study aligns with existing literature on the kynurenine pathway of tryptophan degradation, which was implicated in various neuropathological features presented in amyotrophic lateral sclerosis, including neuroinflammation, excitotoxicity, oxidative stress, immune system activation, and dysregulation of energy metabolism (49). Our MR findings further revealed a potentially lower level of cholesterol associated with the genetically predicted onset of MG, which may be explained by the alterations in the gut microbiome of MG individuals, potentially linked to impaired intestinal lipid absorption and dysregulated cholesterol metabolism. Of note, the metabolites analyzed in our MR study included 2-aminobutyrate, a derivative of SCFA, and 3-hydroxybutyrate, a typical SCFA, although both of which exhibited no causal association with MG.
The disparities between the outcomes of the aforementioned studies and those of our MR study might potentially be attributed to the synergistic interplay between the gut microbiome and gene-environment factors. Firstly, the use of non-selective immunosuppressive agents such as glucocorticoid in MG patients might lead to changes in the gut microbiome. Secondly, the composition of gut microbiome could vary between active and remission phases of MG, which many studies failed to consider when grouping patients. Thirdly, previous clinical observational studies were not exclusively limited to AChR antibody-positive MG; they may have also included other antibody-positive cases, such as MUSK, which could introduce confounding factors. Fourthly, the interactions and cross-talks among gut microbiome may be intricate and cannot be eliminated in former observational studies. Furthermore, factors like gender ratios, age disparities, ethnicity, and varying sample sizes across different studies could result in differences in the composition of gut microbiome. The presence of these uncertainties contributes to incongruent research outcomes and hampers the ability to infer a precise causal link between gut microbiome and MG risk. Future randomized controlled trials and pathophysiological studies are needed to provide more evidence for their interactive causal relationships.
Our study has notable strengths. Firstly, we presented the first MR study on gut microbiome, derived metabolites, and AChR antibody-positive MG. Secondly, we incorporated the latest extensive GWAS summary data, utilizing genetic information from a substantial sample size encompassing the largest cohort of MG cases. This enhances the reliability of our findings compared to small scale randomized controlled trials. To ensure robustness, bidirectional MR analyses were conducted. Furthermore, the strict inclusion criteria for the study population have made the results more convincing.
It is important to note that our study had some limitations: Firstly, the MR study did not ascertain whether there was data overlap within the included GWAS summary data. However, we have taken measures to minimize participant overlap deviation and discarding weak IVs through the utilization of F statistics (F>10). Secondly, the relatively low 10,000 read count of gene sequencing in terms of gut microbiome may be indicative of bias inherent in the original GWAS; if it was conducted using more advanced shotgun metagenomic sequencing analysis, the results would likely be more specific and accurate. Thirdly, because the majority of participants were of European ancestry, there is a possibility that the findings of this study may not be applicable to citizens in other regions. Therefore, future analyses should be conducted on a larger scale and employ more advanced methodologies to assess the interrelationships between gut microbiome features such as species and MG using high-resolution data, which may shed more light on supplementation of probiotics or prebiotics, fecal microbiota transplantation and other manners of gut microbiome regulation for MG prevention and treatment.
Conclusions
In conclusion, our MR findings support the genetically predicted causal effects of specific gut microbiome taxa and derived metabolites on AChR antibody-positive MG, and vice versa, yielding novel insights into prevention and therapy targets of MG. Future studies may be warranted for validation and pursuing the precise mechanisms.
Data availability statement
The original contributions presented in the study are included in the article/Supplementary Material. Further inquiries can be directed to the corresponding author.
Ethics statement
This MR study did not necessitate ethical approval, as it was conducted using summarized data obtained exclusively from publicly accessible sources. No individual’s personal information was involved at any stage of this study.
Author contributions
DS: Methodology, Software, Writing – original draft, Writing – review & editing. SW: Methodology, Visualization, Writing – original draft, Writing – review & editing. PL: Investigation, Methodology, Writing – review & editing. JL, ZX, and HL: Software, Writing – review & editing. WL: Validation, Writing – review & editing. BX: Conceptualization, Writing – review & editing. LZ: Conceptualization, Project administration, Writing – original draft, Writing – review & editing
Funding
The author(s) declare financial support was received for the research, authorship, and/or publication of this article. This work was supported by the following funding: National Natural Science Foundation of China (Grant No. 81601139) and Natural Science Foundation of Hunan Province (Grant No. 2017JJ3500).
Acknowledgments
Our gratitude goes to the authors and researchers of the utilized GWASs for their valuable summary statistics datasets.
Conflict of interest
The authors declare that the research was conducted in the absence of any commercial or financial relationships that could be construed as a potential conflict of interest.
Publisher’s note
All claims expressed in this article are solely those of the authors and do not necessarily represent those of their affiliated organizations, or those of the publisher, the editors and the reviewers. Any product that may be evaluated in this article, or claim that may be made by its manufacturer, is not guaranteed or endorsed by the publisher.
Supplementary material
The Supplementary Material for this article can be found online at: https://www.frontiersin.org/articles/10.3389/fimmu.2023.1279845/full#supplementary-material
References
1. Gilhus NE, Verschuuren JJ. Myasthenia gravis: subgroup classification and therapeutic strategies. Lancet Neurol (2015) 14(10):1023–36. doi: 10.1016/S1474-4422(15)00145-3
2. Carr AS, Cardwell CR, McCarron PO, McConville J. A Systematic Review of Population Based Epidemiological Studies in Myasthenia Gravis. Carr et al. BMC Neurol (2010) 10:46. doi: 10.1186/1471-2377-10-46
3. Alshekhlee A, Miles MJD, Katirji PB, Preston DC, Kaminski HJ. Incidence and mortality rates of myasthenia gravis and myasthenic crisis in us hospitals. Neurology (2009) 72:1548–54. doi: 10.1212/WNL.0b013e3181a41211
4. Binda C, Lopetuso LR, Rizzatti G, Gibiino G, Cennamo V, Gasbarrini A. Actinobacteria: A relevant minority for the maintenance of gut homeostasis. Digestive Liver Dis (2018) 50(5):421–8. doi: 10.1016/j.dld.2018.02.012
5. Punga AR, Maddison P, Heckmann JM, Guptill JT, Evoli A. Epidemiology, diagnostics, and biomarkers of autoimmune neuromuscular junction disorders. Lancet Neurol (2022) 21(2):176–88. doi: 10.1016/S1474-4422(21)00297-0
6. Wong SH, Petrie A, Plant GT. Ocular myasthenia gravis: toward a risk of generalization score and sample size calculation for a randomized controlled trial of disease modification. J Neuro-Ophthalmology (2016) 36(3):252–8. doi: 10.1097/wno.0000000000000350
7. Verschuuren JJGM, Huijbers MG, Plomp JJ, Niks EH, Molenaar PC, Martinez-Martinez P, et al. Pathophysiology of myasthenia gravis with antibodies to the acetylcholine receptor, muscle-specific kinase and low-density lipoprotein receptor-related protein 4. Autoimmun Rev (2013) 12(9):918–23. doi: 10.1016/j.autrev.2013.03.001
8. Gilhus NE. Myasthenia and the neuromuscular junction. Curr Opin Neurol (2012) 25(5):523–9. doi: 10.1097/WCO.0b013e3283572588
9. Thye AY, Law JW, Tan LT, Thurairajasingam S, Chan K-G, Letchumanan V, et al. Exploring the gut microbiome in myasthenia gravis. Nutrients (2022) 14(8):1647. doi: 10.3390/nu14081647
10. Liu L, Wang H, Chen X, Xie P. Gut microbiota: A new insight into neurological diseases. Chin Med J (Engl) (2023) 136(11):1261–77. doi: 10.1097/CM9.0000000000002212
11. Kang Y, Li L, Kang X, Zhao Y, Cai Y. Gut microbiota and metabolites in myasthenia gravis: early diagnostic biomarkers and therapeutic strategies. Clin Immunol (2022) 245:109173. doi: 10.1016/j.clim.2022.109173
12. Kapoor B, Gulati M, Gupta R, Singla RK. Microbiota dysbiosis and myasthenia gravis: do all roads lead to rome? Autoimmun Rev (2023) 22(5):103313. doi: 10.1016/j.autrev.2023.103313
13. Jandhyala SM, Talukdar R, Subramanyam C, Vuyyuru H, Sasikala M, Nageshwar Reddy D. Role of the normal gut microbiota. World J Gastroenterol (2015) 21(29):8787–803. doi: 10.3748/wjg.v21.i29.8787
14. Aricha R, Mizrachi K, Fuchs S, Souroujon MC. Blocking of il-6 suppresses experimental autoimmune myasthenia gravis. J Autoimmun (2011) 36(2):135–41. doi: 10.1016/j.jaut.2010.12.001
15. Mu L, Sun B, Kong Q, Wang J, Wang G, Zhang S, et al. Disequilibrium of T helper type 1, 2 and 17 cells and regulatory T cells during the development of experimental autoimmune myasthenia gravis. Immunology (2009) 128(1 Suppl):e826–36. doi: 10.1111/j.1365-2567.2009.03089.x
16. Vijay A, Valdes AM. Role of the gut microbiome in chronic diseases: A narrative review. Eur J Clin Nutr (2022) 76(4):489–501. doi: 10.1038/s41430-021-00991-6
17. Liu P, Jiang Y, Gu S, Xue Y, Yang H, Li Y, et al. Metagenome-wide association study of gut microbiome revealed potential microbial marker set for diagnosis of pediatric myasthenia gravis. BMC Med (2021) 19(1):159. doi: 10.1186/s12916-021-02034-0
18. Qiu D, Xia Z, Jiao X, Deng J, Zhang L, Li J. Altered gut microbiota in myasthenia gravis. Front Microbiol (2018) 9:2627. doi: 10.3389/fmicb.2018.02627
19. Moris G, Arboleya S, Mancabelli L, Milani C, Ventura M, de Los Reyes-Gavilán CG, et al. Fecal microbiota profile in a group of myasthenia gravis patients. Sci Rep (2018) 8(1):14384. doi: 10.1038/s41598-018-32700-y
20. Chia R, Saez-Atienzar S, Murphy N, Chiò A, Blauwendraat C, Roda RH, et al. Identification of genetic risk loci and prioritization of genes and pathways for myasthenia gravis: A genome-wide association study. Proc Natl Acad Sci U.S.A. (2022) 119(5):e2108672119. doi: 10.1073/pnas.2108672119
21. Emdin CA, Khera AV, Kathiresan S. Mendelian randomization. JAMA (2017) 318(19):1925–6. doi: 10.1001/jama.2017.17219
22. Sekula P, Del Greco MF, Pattaro C, Kottgen A. Mendelian randomization as an approach to assess causality using observational data. J Am Soc Nephrol (2016) 27(11):3253–65. doi: 10.1681/ASN.2016010098
23. Luo M, Sun M, Wang T, Zhang S, Song X, Liu X, et al. Gut microbiota and type 1 diabetes: A two-sample bidirectional mendelian randomization study. Front Cell Infect Microbiol (2023) 13:1163898. doi: 10.3389/fcimb.2023.1163898
24. Cao J, Wang N, Luo Y, Ma C, Chen Z, Chenzhao C, et al. A cause–effect relationship between graves’ Disease and the gut microbiome contributes to the thyroid–gut axis: A bidirectional two-sample mendelian randomization study. Front Immunol (2023) 14:977587. doi: 10.3389/fimmu.2023.977587
25. Ren F, Jin Q, Liu T, Ren X, Zhan Y. Causal effects between gut microbiota and iga nephropathy: A bidirectional mendelian randomization study. Front Cell Infect Microbiol (2023) 13:1171517. doi: 10.3389/fcimb.2023.1171517
26. Cao Y, Lu H, Xu W, Zhong M. Gut microbiota and sjogren’s syndrome: A two-sample mendelian randomization study. Front Immunol (2023) 14:1187906. doi: 10.3389/fimmu.2023.1187906
27. Meng C, Deng P, Miao R, Tang H, Li Y, Wang J, et al. Gut microbiome and risk of ischaemic stroke: A comprehensive mendelian randomization study. Eur J Prev Cardiol (2023) 30(7):613–20. doi: 10.1093/eurjpc/zwad052
28. Jiang L, Li JC, Tang BS, Guo JF. Associations between gut microbiota and parkinson disease: A bidirectional mendelian randomization analysis. Eur J Neurol (2023) 30(11):3471–7. doi: 10.1111/ene.15848
29. Zeng Y, Cao S, Yang H. Roles of gut microbiome in epilepsy risk: A mendelian randomization study. Front Microbiol (2023) 14:1115014. doi: 10.3389/fmicb.2023.1115014
30. He Q, Wang W, Xiong Y, Tao C, Ma L, Ma J, et al. A causal effects of gut microbiota in the development of migraine. J Headache Pain (2023) 24(1):90. doi: 10.1186/s10194-023-01609-x
31. Kurilshikov A, Medina-Gomez C, Bacigalupe R, Radjabzadeh D, Wang J, Demirkan A, et al. Large-scale association analyses identify host factors influencing human gut microbiome composition. Nat Genet (2021) 53(2):156–65. doi: 10.1038/s41588-020-00763-1
32. Shin S-Y, Fauman EB, Petersen A-K, Krumsiek J, Santos R, Huang J, et al. An atlas of genetic influences on human blood metabolites. Nat Genet (2014) 46(6):543–50. doi: 10.1038/ng.2982
33. Yu K, Chen XF, Guo J, Wang S, Huang X-T, Guo Y, et al. Assessment of bidirectional relationships between brain imaging-derived phenotypes and stroke: A mendelian randomization study. BMC Med (2023) 21(1):271. doi: 10.1186/s12916-023-02982-9
34. Verbanck M, Chen CY, Neale B, Do R. Detection of widespread horizontal pleiotropy in causal relationships inferred from mendelian randomization between complex traits and diseases. Nat Genet (2018) 50(5):693–8. doi: 10.1038/s41588-018-0099-7
35. Zheng P, Li Y, Wu J, Zhang H, Huang Y, Tan X, et al. Perturbed microbial ecology in myasthenia gravis: evidence from the gut microbiome and fecal metabolome. Adv Sci (Weinh) (2019) 6(18):1901441. doi: 10.1002/advs.201901441
36. Hiippala K, Jouhten H, Ronkainen A, Hartikainen A, Kainulainen V, Jalanka J, et al. The potential of gut commensals in reinforcing intestinal barrier function and alleviating inflammation. Nutrients (2018) 10(8):988. doi: 10.3390/nu10080988
37. Miller LE, Lehtoranta L, Lehtinen MJ. The effect of bifidobacterium animalis ssp. Lactis hn019 on cellular immune function in healthy elderly subjects: systematic review and meta-analysis. Nutrients (2017) 9(3):191. doi: 10.3390/nu9030191
38. Sun J, Fan J, Li T, Yan X, Jiang Y. Nuciferine protects against high-fat diet-induced hepatic steatosis via modulation of gut microbiota and bile acid metabolism in rats. J Agric Food Chem (2022) 70(38):12014–28. doi: 10.1021/acs.jafc.2c04817
39. Totzeck A, Ramakrishnan E, Schlag M, Stolte B, Kizina K, Bolz S, et al. Gut bacterial microbiota in patients with myasthenia gravis: results from the mybiom study. Ther Adv Neurol Disord (2021) 14:17562864211035657. doi: 10.1177/17562864211035657
40. Qiu X, Zhang M, Yang X, Hong N, Yu C. Faecalibacterium prausnitzii upregulates regulatory T cells and anti-inflammatory cytokines in treating tnbs-induced colitis. J Crohns Colitis (2013) 7(11):e558–e68. doi: 10.1016/j.crohns.2013.04.002
41. Li N, Wang X, Sun C, Wu X, Lu M, Si Y, et al. Change of intestinal microbiota in cerebral ischemic stroke patients. BMC Microbiol (2019) 19(1):191. doi: 10.1186/s12866-019-1552-1
42. Chriswell ME, Lefferts AR, Clay MR, Hsu AR, Seifert J, Feser ML, et al. Clonal iga and igg autoantibodies from individuals at risk for rheumatoid arthritis identify an arthritogenic strain of subdoligranulum. Sci Transl Med (2022) 14(668):eabn5166. doi: 10.1126/scitranslmed.abn5166
43. Vernocchi P, Gili T, Conte F, Del Chierico F, Conta G, Miccheli A, et al. Network analysis of gut microbiome and metabolome to discover microbiota-linked biomarkers in patients affected by non-small cell lung cancer. Int J Mol Sci (2020) 21(22):8730. doi: 10.3390/ijms21228730
44. Zhang L, Liu Y, Zheng HJ, Zhang CP. The oral microbiota may have influence on oral cancer. Front Cell Infect Microbiol (2019) 9:476. doi: 10.3389/fcimb.2019.00476
45. Zeng Q, Li D, He Y, Li Y, Yang Z, Zhao X, et al. Discrepant gut microbiota markers for the classification of obesity-related metabolic abnormalities. Sci Rep (2019) 9(1):13424. doi: 10.1038/s41598-019-49462-w
46. Brown DG, Borresen EC, Brown RJ, Ryan EP. Heat-stabilised rice bran consumption by colorectal cancer survivors modulates stool metabolite profiles and metabolic networks: A randomised controlled trial. Br J Nutr (2017) 117(9):1244–56. doi: 10.1017/S0007114517001106
47. Wang Q, Zhou H, Bu Q, Wei S, Li L, Zhou J, et al. Role of xbp1 in regulating the progression of non-alcoholic steatohepatitis. J Hepatol (2022) 77(2):312–25. doi: 10.1016/j.jhep.2022.02.031
48. Hosseinkhani F, Heinken A, Thiele I, Lindenburg PW, Harms AC, Hankemeier T. The contribution of gut bacterial metabolites in the human immune signaling pathway of non-communicable diseases. Gut Microbes (2021) 13(1):1–22. doi: 10.1080/19490976.2021.1882927
Keywords: gut microbiome, myasthenia gravis, metabolites, Mendelian randomization, causal effect, neuroimmunology
Citation: Sheng D, Wang S, Li P, Li J, Xiao Z, Lv H, Liu W, Xiao B and Zhou L (2023) Evidence for genetic causal relationships between gut microbiome, metabolites, and myasthenia gravis: a bidirectional Mendelian randomization study. Front. Immunol. 14:1279845. doi: 10.3389/fimmu.2023.1279845
Received: 06 September 2023; Accepted: 11 December 2023;
Published: 21 December 2023.
Edited by:
Narendra Prasad Singh, University of South Carolina, United StatesReviewed by:
Urmi Halder, University of South Carolina, United StatesMuthanna Sultan, University of Tikrit, Iraq
Copyright © 2023 Sheng, Wang, Li, Li, Xiao, Lv, Liu, Xiao and Zhou. This is an open-access article distributed under the terms of the Creative Commons Attribution License (CC BY). The use, distribution or reproduction in other forums is permitted, provided the original author(s) and the copyright owner(s) are credited and that the original publication in this journal is cited, in accordance with accepted academic practice. No use, distribution or reproduction is permitted which does not comply with these terms.
*Correspondence: Luo Zhou, emhvdWx1b0Bjc3UuZWR1LmNu
†These authors have contributed equally to this work and share first authorship