- Key Laboratory of Pathobiology Ministry of Education, College of Basic Medical Sciences/China-Japan Union Hospital of Jilin University, Jilin University, Changchun, China
Tuberculosis is a major infectious disease caused by Mycobacterium tuberculosis infection. The pathogenesis and immune mechanism of tuberculosis are not clear, and it is urgent to find new drugs, diagnosis, and treatment targets. A useful tool in the quest to reveal the enigmas related to Mycobacterium tuberculosis infection and disease is the single-cell sequencing technique. By clarifying cell heterogeneity, identifying pathogenic cell groups, and finding key gene targets, the map at the single cell level enables people to better understand the cell diversity of complex organisms and the immune state of hosts during infection. Here, we briefly reviewed the development of single-cell sequencing, and emphasized the different applications and limitations of various technologies. Single-cell sequencing has been widely used in the study of the pathogenesis and immune response of tuberculosis. We review these works summarizing the most influential findings. Combined with the multi-molecular level and multi-dimensional analysis, we aim to deeply understand the blank and potential future development of the research on Mycobacterium tuberculosis infection using single-cell sequencing technology.
1 Introduction
Mycobacterium tuberculosis (Mtb) is an obligate aerobic microorganism (1). Tuberculosis (TB) is an infectious disease caused by Mtb infection and remains a major global cause of mortality (2). In 2021, there were 10.6 million new TB cases worldwide, and China is among the countries burdened with high TB incidence (3). TB primarily spreads through respiratory aerosols and can affect various organs systemically, with pulmonary tuberculosis being the most common manifestation (4). Currently, the clinical diagnosis of pulmonary tuberculosis largely relies on chest radiography, sputum smear microscopy, and bacteriological cultures (5). Rapid diagnostic techniques such as auramine-rhodamine fluorescence and the AccuProbe Mycobacterium tuberculosis complex test have also been widely utilized (6, 7). However, the ever-evolving public health challenges necessitate novel diagnostic tools and therapeutic approaches. Additionally, there is a growing need to gain deeper insights into the impact of Mtb infection on the host and the underlying immune mechanisms.
Although traditional molecular biology experimental methods have successfully revealed certain aspects of the infection and immune mechanisms of Mtb, such as surfactant protein D located in the alveoli which can inhibit Mtb infection by blocking mannose oligosaccharide residues on the bacterial cell surface (8), Mtb can secrete the virulence factor Rv0222 to escape the immune response after infecting the human body (9). However, after infection, a comprehensive panorama of the intricate host immune regulatory processes and dynamic evolution still lacks elucidation. Our understanding of the diversity and heterogeneity of immune cells, as well as their relevant physiological, pathological processes, and molecular mechanisms, remains incomplete. Therefore, there is an urgent need to explore the mechanisms of Mtb infection and the dynamic host immune processes at the single-cell level, to identify novel drug targets and new immunotherapeutic approaches.
In this section, we provide a concise overview of the development of single-cell sequencing. We place particular emphasis on the discoveries gathered through the application of single-cell sequencing in the field of tuberculosis research, as reported in the literature. Additionally, we discuss the research domains that, in our view, merit exploration through the utilization of single-cell sequencing methodologies.
2 Single-cell sequencing
Traditional transcriptomic analyses have been employed to comprehend the pathogenesis, but single-cell sequencing offers novel perspectives and significant convenience in enhancing our understanding of human cell heterogeneity, identifying susceptible cell types, and elucidating infection dynamics (10, 11). Single-cell sequencing is a high-throughput sequencing analysis performed at the individual cell level, encompassing transcriptomic, genomic, and epigenomic profiling. It bridges certain limitations of traditional sequencing techniques and molecular experiments, unraveling the genetic architecture and gene expression states of individual cells while reflecting intercellular heterogeneity (12). Consequently, single-cell sequencing technology has emerged as a highly precise, reliable, and efficient analytical approach in recent years, playing a crucial role in research domains such as oncology and virology (13, 14).
2.1 Single-cell RNA sequencing
Since the inception of single-cell transcriptomic sequencing at the individual cell level which was proposed by Professor Fuchou Tang (15), researchers have embarked on a new era of single-cell sequencing. Among these techniques, single-cell transcriptomic sequencing (scRNA-seq) stands out as the most widely applied approach. The technique has evolved from conventional transcriptomics methodologies. In conventional transcriptomics studies, the typical workflow entails RNA extraction, reverse transcription to generate cDNA, sequencing and library construction. The principle of this technology is commencing with high-throughput cellular capture and labeling, followed by transcriptome amplification to procure transcriptional information. Subsequently, comprehensive data analysis is conducted to extract meaningful insights (16).This technique enables single-cell level analysis of millions of cells within a single study. Despite the minute amount of starting material in each cell, it allows for cell classification, qualitative characterization, and discrimination at the single-cell resolution, thereby identifying rare cell populations (17). Generally, scRNA-seq steps include sample processing, single-cell capture, sequence and analysis pipelines, quality control, feature selection, cluster annotation, cell cycle, pseudotime analysis, differential expression and cell interaction (Figure 1).
In 2011, Islam et al. developed single-cell tagged reverse transcription (STRT-Seq) upon previous research. This technology enabled the identification of transcript 5′-end samples and analysis of promoter usage within single cells, thereby increasing the number of detectable cells and the diversity of cell types that could be analyzed (18). In 2012, Daniel Ramsköld et al. developed the Switching mechanism at the 5' end of the RNA transcript (Smart-Seq), which enhanced the read coverage of the transcriptome, thereby facilitating detailed analysis of alternative transcript isoforms (19). In the same year, Tamar Hashimshony et al. addressed the limitation of low starting RNA amounts in scRNA-seq by developing Cell Expression by Linear amplification and Sequencing (CEL-seq), a method that can prove beneficial for transcriptomic analysis of complex tissues containing multiple cell types (20). In 2013, building upon prior research, Simone Picelli et al. introduced Smart-seq2, a technique that significantly enhanced the sensitivity and accuracy of single-cell transcriptomic analysis, allowing for comprehensive profiling of the transcriptome at the individual cell level (21). In 2014, Islam et al. introduced an alternative quantification standard in the realm of scRNA-seq known as the unique molecular identifier(UMI). UMI is a single-strand region with a randomly synthesized nucleotide with a length ranging from 4 to 12. It is incorporated into primers before reverse transcription, enabling the unique barcoding of the 5' or 3' end of each mRNA copy of a transcript. Leveraging UMI information allows for enhanced quantification of mRNA molecules, effectively mitigating the errors introduced by PCR, and giving rise to UMI-based absolute quantification transcriptomic sequencing (22). In the subsequent years, there has been an exponential surge in related techniques for scRNA-seq, including Quartz-seq (23), MARS-seq (24), Drop-Seq (25), MARS-seq2.0 (26), inDrop (27), and others (Figure 2, Supplementary Table 1).
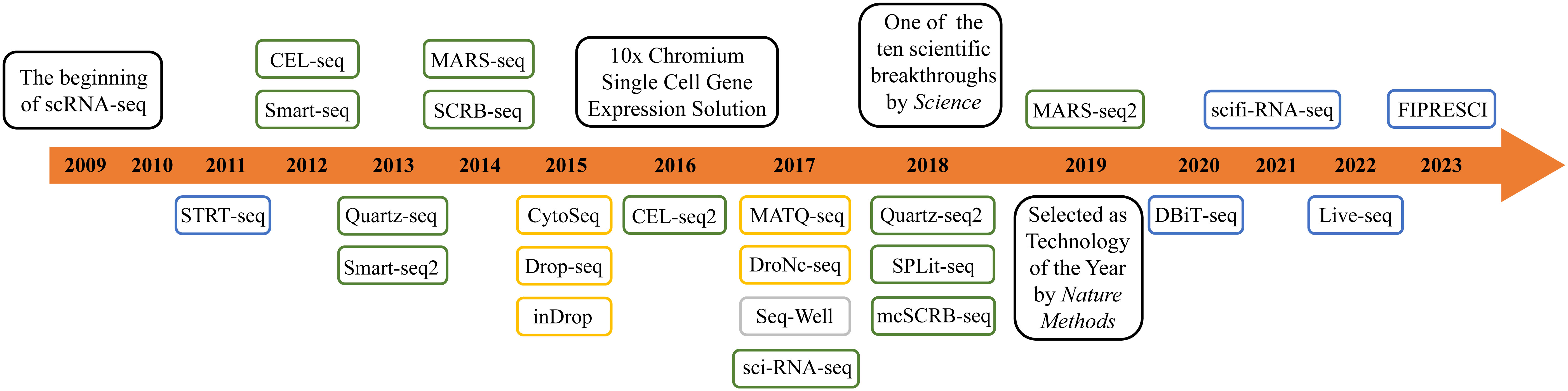
Figure 2 The development of scRNA-seq technology. The blue boxes represent microfluidics-based technology. The green boxes represent plate-based technology. The orange boxes represent microdroplet-based technology. The grey box represents nanowell-arrays-based technology.
2.2 ATAC-seq
In eukaryotes, chromatin serves as the fundamental genetic unit, typically composed of DNA, histones, non-histone proteins, and a small amount of RNA. Chromatin represents a dynamic nuclear structure that undergoes transformation into chromosomes during both mitosis and meiosis. During the interphase of the cell cycle, it exhibits transcriptional activity (28). Chromatin plays a pivotal role in regulating the expression of cell-specific genes (29, 30), and the investigation of chromatin-associated nuclear protein structures also aids in elucidating how epigenetic factors control gene expression (31). From the conventional Chromatin Immunoprecipitation (ChIP) to the chromatin immunocleavage (ChIC), research methodologies in the field of epigenetics have been continuously evolving (32, 33). As scRNA-seq has unlocked the sequencing potential at single-cell resolution, researchers have concurrently delved into the development and exploration of single-cell epigenomic sequencing techniques. Among the more commonly employed methods are MNase-seq, DNase-seq, and NOMe-seq (34, 35).
However, due to the aforementioned issues such as complex protocols, poor reproducibility, the requirement for a large number of cells, and suboptimal sequencing signals, Jason D Buenrostro and colleagues introduced the Assay for Transposase-Accessible Chromatin with high throughput sequencing (ATAC-seq) in 2013. This method utilizes engineered Tn5 transposase coupled with high-throughput sequencing technology to map nucleosome positioning and investigate chromatin accessibility at the single-cell resolution (36). ATAC-seq steps are composed of cell nuclei preparation, transposition, and amplification (37). This technique requires a relatively low sample quantity, significantly reducing the required experimental time. Moreover, due to the non-cutting nature of Tn5 transposase, it can capture complete information on the accessible regions. However, the presence of mitochondrial DNA may still introduce some errors during the detection process.
2.3 Single-cell genome sequencing
Single-cell genome sequencing is a sophisticated technique which can amplify minute quantities of the entire genome DNA at the single-cell level, followed by exome capture and sequencing. This technology enables researchers to obtain a high coverage of the complete genome, allowing for comprehensive analysis of the genetic information of each cell. The main purpose of this technique is to investigate the impact of individual cells on biological processes, uncover differences among cell populations, and reveal the evolutionary relationships between cells (38). The primary workflow of this technique involves several key steps: isolation of single cells, whole-genome amplification, detection of the amplified genome, error correction to minimize potential inaccuracies, and determination of the genetic relationships among individual cells (38). In response to the need for the isolation of individual cells, various methods have been developed, including microfluidic and bead-based methods (39) and microwell dilution (40). Currently, using droplets or micromechanical valves in microfluidic device has become more prevalent (41, 42). In the step of whole-genome amplification, various methods such as multiple displacement amplification (MDA) (43), degenerate oligonucleotide primed PCR (DOP-PCR) (44), and eMDA (45) have been utilized. However, the most commonly employed method is multiple annealing and looping-based amplification cycles (MALBAC), which was introduced in 2012 (46). This technique utilizes random primers and novel temperature cycling and it requires a smaller amount of template. Compared to other technologies, MALBAC requires a smaller amount of template, and its amplification uniformity significantly surpasses that of other amplification methods. Over 90% of loci can be successfully amplified, enabling accurate detection of copy number variations (47).
2.4 Spatial transcriptomics
The majority of techniques involve enzymatic dissociation of tissues to obtain single cells, leading to the loss of spatial positional information during the research process. To overcome this limitation, researchers have developed Spatial transcriptomics, which is based on scRNA-seq. Spatial transcriptomics aims to analyze the transcriptional profile information from different spatial locations within the sample (48). Currently, there are two main approaches for spatial transcriptomics detection: 1) imaging-based methods; 2) sequencing-based methods (49).
Imaging-based methods primarily refer to techniques that are based on imaging mRNAs in situ via microscopy. Based on the method of mRNA discrimination, two approaches can be distinguished (50). The first one is in situ hybridization (ISH), which involves the hybridization of mRNAs to fluorescently labeled, gene-specific probes. Single-molecule RNA fluorescence in situ hybridization (smFISH) is a highly classic ISH method, which allows for quantitative assessment of the target samples (51). However, this method has limitations in terms of the number of fluorescent labels it can accommodate, resulting in suboptimal performance when multiple fluorescent labels are required (52). With the advancement of technology, various techniques such as seqFISH (53), seqFISH+ (54), MERFISH (55), and enhanced electric FISH (56) have been developed and expanded upon the foundation of smFISH. These methods have increased the detectable quantity of targets and improved the stability of fluorescent signals. Another in situ approach is the in situ sequencing-based(ISS-based) technologies. This method enables direct sequencing of mRNA from fixed tissue or cellular samples, allowing the discovery of associations between sequencing information and its spatial location (e.g., subcellular localization) (52). The first ISS probe was introduced by Rongqin Ke and colleagues in 2013 and is known as padlock probes, which are single-strand molecules of DNA complementary to the target cDNA (57). In the subsequent advancements, various methods such as barcode in situ targeted sequencing (BaristaSeq) (58), fluorescence in situ sequencing (FISSEQ) (59), and STARmap (60) have been applied in spatial transcriptomics-related research.
The sequencing-based methods primarily involve the direct extraction of mRNA from tissues while preserving the associated spatial information for sequencing. The earliest related detection using this approach was the “spatial transcriptomics” developed by Patrik L Ståhl (48). By positioning histological sections on arrayed reverse transcription primers with unique positional barcodes, Patrik L Ståhl and colleagues successfully obtained high-quality RNA sequencing data with spatial positional information from mouse brain tissue and human breast cancer tissue. Similar techniques based on this principle include: Slide-seq, a method for transferring RNA from tissue sections onto a surface covered in arrays composed of 10 μm-diameter DNA-barcoded beads with known positions, allowing the locations of the RNA to be inferred by sequencing (61); High-definition spatial transcriptomics (HDST), a spatial transcriptomics technique with 2μm spatial resolution. This method captures RNA from histological tissue sections on a dense, spatially barcoded bead array, enabling higher resolution spatial transcriptome sequencing (62).
Combining the previous achievements of single-cell sequencing in fields such as HIV (63), SARS-CoV-2 (64), and Salmonella (65), it is evident that single-cell sequencing plays a crucial role in deciphering the pathogenic mechanisms and exploring therapeutic targets for related diseases. By investigating and refining specific cell populations associated with pathogen infections at the cellular and molecular levels, followed by the identification of genes and phenotypes regulated by these cells, and further uncovering the associations with immune-related genes, we can infer the phenotypes of these genes, elucidate their relevance to the host immune response, and map an immune regulatory atlas. These methods provide new insights into the Mtb-host interaction, as well as the diagnosis and treatment strategies for related diseases.
3 Findings from single-cell sequencing applications in TB research
Previous analysis methods may have neglected some rare cell groups, but these few cells are probably the key factors to determine the tissue state (66). The use of single-cell sequencing can not only avoid this situation, but also identify susceptible cell types, changes in immune status, find biomarkers, and detect intercellular variation of hosts. It helps us better understand the interaction between the host and Mtb, and finally develop drugs and vaccines and develop new treatment strategies. Throughout this section, the main findings using single-cell sequencing in the field of tuberculosis will be explored (Figure 3, Table 1).
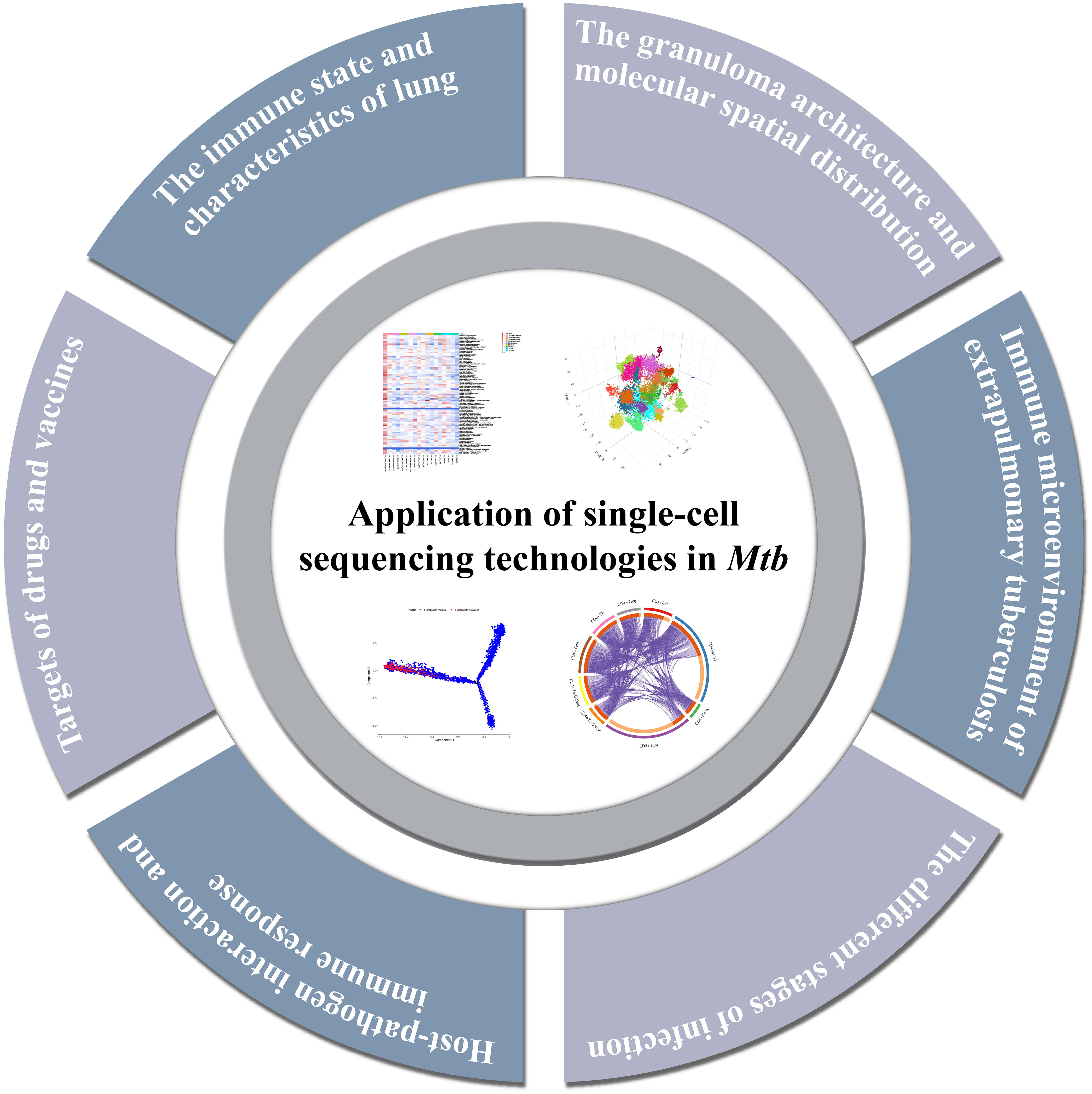
Figure 3 Summary of the major findings resulting from single-cell sequencing application in tuberculosis research.
3.1 The immune state and characteristics of lung
Macrophages are the most important host cells infected by Mtb. Two main macrophage groups have been identified in the lung : tissue-resident alveolar macrophages (AMs) and monocyte-derived interstitial macrophages (IMs). In the airways, Mtb first encounters alveolar macrophages (AMs), which present a permissive niche for infection establishment (84). After the infected AM migrates to the interstitial lung, Mtb will infect more other types of macrophages. During the period of tuberculosis infection, different phenotypes or polarization states of macrophages may affect the growth of bacteria. There are three subtypes of pulmonary macrophages identified from tuberculosis patients, and the expression levels of genes related to growth, metabolism, and hypoxia in these macrophages are different (67). Therefore, it is important to understand the functional heterogeneity of the host macrophage population in controlling or promoting bacterial growth.
The functional identification map of pulmonary macrophage subsets in mice infected with Mtb was constructed by scRNA-seq technology. It found the functional heterogeneity between alveolar macrophages and interstitial macrophages after Mtb infection. It also shows that the main macrophage population in the lung is epigenetically limited in its response to infection. ATAC-seq results showed that the infection of Mtb will result in the induction of similar functional phenotypes in the host macrophage phagocytes (71). Another study revealed the characteristics of alveolar macrophages in bronchoalveolar lavage fluid (BALF) of patients with active tuberculosis. It focuses on the macrophage subgroups related to inflammatory function, and nine macrophage subgroups have been identified, four of which are in the M2 polarization state. At the same time, it was found that the interaction between macrophages and other cells was enhanced after infection (78). In a word, it is feasible to use scRNA-seq to determine the way of bacterial growth control in the macrophage subgroup.
Meanwhile, it is important to explore the immune cell reaction at the infected site, especially the cell proportion, the activation state of cell subsets, and the interaction of immune cells. The lung immunity of rhesus monkeys with latent tuberculosis and active tuberculosis was studied. It was found that NK cells expressing CD27+ in the lung aggregated during the latent period of tuberculosis. During active pulmonary tuberculosis infection, plasma-like dendritic cells (pDCs) and activated T cells will flow into the lungs (74). In the mouse model, the infection of Mtb drives the characteristics of type I IFN in lung lymphocytes and causes the heat shock response of NK cells. Lymphocyte antigen 6 complex A site (Ly6A) was identified as a marker of lymphocyte activation, and it was found that Ly6A was up-regulated in activated lymphocytes after infection (76).
3.2 The granuloma architecture and molecular spatial distribution
The pathological sign of Mtb infection is the formation of granuloma in the lungs. It is a key site for the interaction between host and pathogen, which can limit or promote the survival of bacteria and is composed of a mixture of immune and non-immune cells (85–87). In-situ sequencing was used to analyze pulmonary granulomas in mice at different time points after Mtb infection. It was found that different regions of granulomas could be defined according to the frequency and distribution of transcripts. The different immune landscape of granuloma depends on the time after infection, pathological characteristics, and the proximity to bacteria. The necrosis center may contain transcripts related to immunosuppression (88). Exploring the formation and maintenance mechanism of granuloma can help to eliminate the harmful effects of granuloma on the host and deeply understand the pathogenesis and therapeutic targets of tuberculosis.
To understand the correlation between granuloma and bacterial control, cynomolgus macaques’ granulomas were divided into 13 cell subsets. It is found that bacteria persist in granulomas rich in mast cells, endothelial cells, fibroblasts, and plasma cells. Granuloma, which drives and controls bacteria, is characterized by abundant T cells such as type 1 and type 17, which participate in the pro-inflammatory signal network involving different cell groups. Granuloma in the late stage of infection shows the functional characteristics of restrictive granuloma, which can better control the infection of Mtb (75). On the other hand, macrophages are the main cell type carrying Mtb, which exists in pulmonary tuberculosis granuloma (89). Macrophages and epithelial transformation are necessary for the formation of organized granuloma (90). Single-cell sequencing of the zebrafish-marine mycobacteria model also confirmed the basis of macrophage transformation and the diversity of cells in the granuloma. In granuloma, not only type 1 inflammatory reaction occurs but also type 1 and type 2 reactions are induced. The marker of type 2 reaction is located in the epithelial area of granuloma. Stat6 signaling mediates type 2 immune signaling, which is necessary for the formation of necrotizing granuloma and epithelial macrophages (73). It is the unknown of granuloma that is the basic reason for many difficulties in clinical treatment. However, with the change in treatment methods, most of the current research is carried out in animal models, and the contribution of related molecules and pathways in human tuberculosis remains to be explored.
3.3 Immune microenvironment of extrapulmonary tuberculosis
Tuberculous pleural effusion (TPE) is the second common form of extrapulmonary tuberculosis caused by Mtb infection, which is characterized by the influx of immune cells into the thoracic cavity (91). Its manifestations range from spontaneous absorption of benign pleural effusion to complicated pleural effusion with thickening, empyema, and even fibrosis, which may lead to continuous impairment of lung function (92). Early diagnosis is important to prevent serious complications and treatment. However, the understanding of inflammatory mediators and immune cells in local tissues is relatively backward. Therefore, it is urgent to establish early diagnosis methods and reveal local immune mechanisms. Due to the low sensitivity of other specimens and the invasive and technical difficulty of thoracoscopy, pleural effusion has become the first choice for diagnosis and mechanism research (93).
There are two studies combining single-cell TCR sequencing with scRNA-seq to explore the types, characteristics, and distribution of T cells in tuberculous pleural effusion. One of them mainly discussed the complementarity determination region 3 (CDR 3) length, hydrophobicity, and clonal expansion of T cells. It identified a new subset of polyfunctional CD4 T cells with CD1-restricted, T+H1, and cytotoxic characteristics. This research provides some new thoughts on the protective immunity against Mtb (79). Another research showed that the proportion and differentiation trajectory of T cell clusters in peripheral blood and pleural effusion were different. T cells in pleural effusion have high heterogeneity and unique highly amplified T cell clones. CD8+T cells expressing granzyme K are preferentially enriched in pleural effusion, which may be involved in the occurrence of diseases. These findings reveal the T-cell immune status of patients with tuberculous pleurisy (94). In addition to T cells, tuberculous pleurisy also involves a variety of immune cell types. The study revealed the single-cell landscape of immune cells in tuberculous pleurisy pleural effusion, and found that the immune exhaustion of NK cells and the increase of pro-inflammatory factors driven by macrophages were its important characteristics (83). In addition, it also reveals the unique local immune response in leaking pleural effusion and malignant pleural effusion. These findings will improve the understanding of the infection and immune mechanism of extrapulmonary tuberculosis.
3.4 The different stages of infection
Most patients infected by Mtb are still asymptomatic, which is called latent tuberculosis infection, and some of them will develop into active tuberculosis (95). Major challenges of tuberculosis control are the lack of biomarkers to distinguish the status of different infections, to assess the severity of diseases, and to predict disease outcomes. To make the diagnosis, more and more methods have been established besides traditional cultural techniques. The infection of tuberculosis was diagnosed by immunological methods: Mantoux tubulin skin test (TST) and interferon gamma release assay (IGRA) (96). It was found by flow cytometry that the specific CD4+T cells of dominant TNF-α+ Mtb were different in latent infection and active infection (97). However, even with the development of many molecular detection technologies, there are still problems such as false positive diagnosis among vaccinated people. It is necessary to know the immune factors related to disease progression to promote the immune control of Mtb. The higher resolution and numerous functional parameters brought by single-cell sequencing technology help us to further explore the changes of immune cell subsets and related dynamic regulation ways under different infection conditions.
It has been found that infection can change the frequency of immune cell subsets in TB by comparing the single cell data of healthy people (HC), latent tuberculosis patients (LTBI), and active tuberculosis patients (TB). Specifically, the natural killer cell subsets (CD3-CD7+GZMB+) are gradually depleted from HC to LTBI and TB, and it is proved that the change in the frequency of this subset can distinguish tuberculosis patients from latent patients and healthy people (69). In addition, different stages of the disease can be predicted by developing new algorithms to process the data. Using a dynamic deconvolution algorithm, it was found that compared with LTBI and the control group, NKT cells in TB patients were less activated and monocytes were more activated. LTBI individuals with a higher risk of developing active tuberculosis can be identified by different characteristics of infected monocytes (68). The above research also proves that scRNA-seq can establish significant differences between different stages of the disease according to the discovery of new cell subsets and identification of the characteristics of cell subsets.
3.5 Host-pathogen interaction and immune response
The innate immunity plays a role in resisting the initial infection of Mtb. Host coordinates various signal transduction and initiates various cellular functions, such as phagocytosis, autophagy, apoptosis, and activation of inflammatory bodies, to control or eliminate Mtb (98, 99). Adaptive immunity is the key to controlling infection. The adaptive immune response to Mtb can be detected in 3-8 weeks after infection, which may be beneficial to the colonization of Mtb (100). Host-pathogen interaction will lead to different results in subsequent treatment strategies, such as cell exhaustion and immune escape, which will not be conducive to the prognosis of tuberculosis. Host-Mtb interaction and regulation of immune response are indispensable research in tuberculosis research.
T cell exhaustion is one of the important strategies for Mycobacterium tuberculosis to hinder adaptive immune response during infection. Exploring cell exhaustion can better design prognostic and diagnostic markers and formulate intervention measures (101). Studies have found that patients with severe tuberculosis will experience T cell exhaustion. Yi Wang et al. have successfully constructed a comprehensive immune map of the peripheral blood of active pulmonary tuberculosis patients with different severity by single-cell sequencing (81). It was found that the proportion of inflammatory immune cells (such as monocytes) increased significantly, while the abundance of various lymphocytes (such as natural killer cells and γδT cells) decreased significantly. The apoptosis induced by perforin/granzyme, FAS and XAF1 may be the reasons for the decrease in lymphocyte abundance. In addition, the immune status of severe patients is characterized by extensive immune failure of Th1, CD8+T, and NK cells and high cytotoxicity of CD8+T and NK cells.
Understanding the immune escape mechanism of Mtb is of great significance for the prevention, diagnosis and treatment of tuberculosis. A large number of studies have expounded the molecular mechanism (102). For example, the protein kinase G (PknG) in Mtb can inhibit the innate immunity of the host by binding to the host E2 protein UbcH7 through the ubiquitin-like (Ubl) domain (103). Cytoplasmic sensors cGAS, IFI204, and AIM2 recognize Mtb DNA, while RIG-1, MDA5, and PKR detect RNA. After that, activated cytosolic DNA/RNA sensors further induce the activation of inflammasomes or NF-κB- and IRF3-mediated innate immune pathways to regulate host anti-Mtb responsese (104). However, single cell sequencing technology has not been fully used in the study of immune evasion of tuberculosis. We hope that high-throughput sequencing can be combined with existing research methods in future research, so as to further clarify the immune escape mechanism.
3.6 Targets of drugs and vaccines
Due to the improper use of antibiotics, the evolution of Mtb, and co-infection with HIV, the emergence of extensively drug-resistant tuberculosis and completely drug-resistant tuberculosis is increasing (105, 106). At the same time, the drug treatment of tuberculosis has not changed. At present, it mainly includes four drugs: isoniazid, rifampicin, pyrazinamide, and ethambutol. Studies have shown that there are at least 14 candidate drugs for susceptible, drug-resistant, and latent tuberculosis in the world at the stage of clinical drug development (107). However, it is still far from enough to develop new tuberculosis drugs and prevention strategies. In recent years, only two anti-tuberculosis drugs have been developed (107, 108).
Rifampicin (RIF) was first used in the clinical treatment of tuberculosis in 1968, and it is still a first-line anti-tuberculosis drug (109, 110). Rifampicin is a semi-synthetic rifamycin drug, which can act on the rpoB gene encoding the β subunit of RNA polymerase and interfere with the synthesis of deoxyribonucleic acid and protein (111, 112). Therefore, more than 95% of RIF drug-resistant mutations are related to rpoB gene mutations (112, 113). The pharmacokinetics of RIF and rifapentine were studied by spatial metabonomics technology. The research on spatial resolution clearly and intuitively showed the metabolic pattern of drugs in animal models. The results showed that there were differences between RIF and rifapentine in penetrating into the cavity, which explained the reasons why the two drugs were not effective in treating patients with large lung cavities (114).
At present, the only licensed vaccine to prevent tuberculosis in the world is Bacille Calmette-Guérin (BCG). It has high efficacy in newborns, but with the continuous advancement of research, clinical experiments have shown that the effect of BCG in preventing tuberculosis in adolescents and adults is not satisfactory. In addition, the enhancer of BCG has not been proved to be effective (115). Through single-cell sequencing, it was found that BCG can reduce systemic inflammation and highlight the genes with non-specific protective effect of BCG. It showed the changes related to BCG-induced immune and protective effects of circulating monocytes (115, 116). T cell receptors related to the control and disease progression of Mtb infection were extensively screened by using scTCR-seq. These T cell antigens can be regarded as high priority targets for vaccine development (82). With the continuous development of single-cell sequencing, more therapeutic targets are gradually screened out. This provides a theoretical basis for establishing an evaluation system of tuberculosis immune protection and guiding the research and development of vaccines and drugs.
4 Discussion
Single-cell sequencing is changing traditional molecular biology research by establishing single-cell maps between different cells and tissues. At the same time, the mature commercial sequencing platform and a variety of developed new technologies have greatly reduced the sequencing cost, but each technology still has limitations. When some pathogens are infected, there is no 3’ poly-A tail in its mRNA, which will lead to the inability of scRNA-seq to recognize it (117). Because of the high proportion of binuclear cells in the blood of pulmonary tuberculosis patients, there are two mixed transcription groups, which will have a certain impact on data processing and analysis (118). Moreover, scRNA-seq can identify the cell subsets in the tissue, but it can’t capture their spatial distribution. In a word, there are still many challenges and opportunities in single-cell sequencing technology.
With the development of single-cell research, scientists are no longer satisfied with obtaining single information. By adding time and space information and combining with higher resolution detection technology, further development is carried out. The scRNA-seq approach combined it with other powerful approaches (multiplex of genomic, and proteomic assays, to list a few) will certainly enhance the quest to reveal some of the enigmas related to Mtb infection and disease in humans. Spatial transcriptomics has also played a promoting role in the related research of Mtb infection. Andrew J Sawyer et al. used single-cell multiple fluorescence phenotype analysis and spatial single-cell analysis algorithm to analyze tuberculosis tissue slice samples. The whole landscape map was constructed, and the classification and pathological classification of tuberculosis granuloma were systematically established. In addition to the recognized necrotizing lesions, it is also identified that there are at least four types of obvious non-necrotizing lesions in composition and space. These lesions are formed by adjacent necrotizing granulomas and tissues in the focus of immune cells (119). However, although the development of related spatial transcriptome sequencing technology has provided researchers with higher dimensional choices, spatial transcriptome technology cannot achieve the analysis of deep single-cell transcriptome resolution at present (31).
Rapid identification of bacterial phenotype, virulence and antibiotic sensitivity is important for infectious disease management. As a high-resolution tool, whole genome sequencing has been widely used in clinical diagnosis and risk management. However, at present, single-cell genome sequencing is rarely used in clinical research. The combination of single cell sequencing and whole-genome amplification can reveal the lifestyle of environmental bacteria (120). Combined with metagenomics, it can be used to identify the biosynthetic pathway of apratoxin (a potent cancer cell toxin with medicinal prospects) (121). The antigens and targets related to infection, immunity and vaccine of Mtb can be found by whole genome sequencing (122). Through DNA sequencing, it was found that compared with the original strain, there were gene mutations related to virulence in the strains exposed to the vaccine (123). However, we have not found that single-cell genome sequencing is used in the research related to Mtb. With the maturity of technology, we believe that this method is conducive to the clinical identification of a variety of bacterial infections, bacteria related to genetic susceptibility and new uncultured pathogens (124). It can also expand our understanding of host-Mtb interaction.
With the breakthrough of related technologies and the improvement of resolution, single-cell sequencing also provides support for the research of protein omics (125). By detecting the proteins interacting between Mtb and 11 kinds of STPKs, the STPKs- protein interaction network map was constructed, including 492 binding proteins and 1027 interacting proteins. It is found that PknG maintains the integrity of the cell wall through peptidoglycan (PG) biosynthesis (126). Through the combination of various omics techniques, people can further grasp the changes and characteristics of tuberculosis at multiple levels and tissues, reveal the relationship between molecules, and promote the identification of interrelated biological processes (127, 128). This also provides a new dimension for drug research and development and contributes to the prevention and control of tuberculosis.
The integration of omics technology needs many methods and tools to analyze and interpret the generated multidimensional data. Every omics has developed statistical methods to analyze its high-quality data. However, when data from different sequencing methods and platforms are integrated for analysis, the inconsistency of data characteristics may not be properly handled (129, 130). This is also a difficult point in multivariate analysis. Some studies have tried to use integrated genomics to characterize the resistance mechanism of p-aminosalicylic acid to multidrug-resistant tuberculosis and extensively drug-resistant tuberculosis (131). The study of integrated omics at single cell level is rare, but we think it has potential research value in understanding the gene regulation, metabolism, adaptability and pathogenicity of Mtb.
In a word, the complexity of cell subsets in body fluids increases with the development of advanced technology. Single-cell sequencing provides a new direction for precise treatment by exploring specific cell subsets, mining key molecules, and clarifying the mechanism of pathogen-host interaction. In the future, single-cell sequencing will be more widely used in the study of pathogenic microbial infections and other diseases.
Author contributions
JP: Writing – original draft, Conceptualization, Writing – review & editing. ZC: Writing – original draft, Conceptualization, Writing – review & editing. XZ: Visualization, Writing – review & editing. QD: Investigation, Writing – review & editing. HZ: Investigation, Writing – review & editing. JS: Supervision, Writing – review & editing. GW: Supervision, Writing – review & editing.
Funding
The authors declare financial support was received for the research, authorship, and/or publication of this article. This work was supported by the National Natural Science Foundation of China [82072330, 32271511]; Jilin University China-Japan Union Hospital-Basic Medical College Joint Project [KYXZ2022JC02] and International Joint Research Center for Pathogen and Infection Informatics, Jilin Province [20210504004GH].
Conflict of interest
The authors declare that the research was conducted in the absence of any commercial or financial relationships that could be construed as a potential conflict of interest.
Publisher’s note
All claims expressed in this article are solely those of the authors and do not necessarily represent those of their affiliated organizations, or those of the publisher, the editors and the reviewers. Any product that may be evaluated in this article, or claim that may be made by its manufacturer, is not guaranteed or endorsed by the publisher.
Supplementary material
The Supplementary Material for this article can be found online at: https://www.frontiersin.org/articles/10.3389/fimmu.2023.1276194/full#supplementary-material
References
1. Martinez A, Torello S, Kolter R. Sliding motility in mycobacteria. J Bacteriol (1999) 181(23):7331–8. doi: 10.1128/JB.181.23.7331-7338.1999
2. Natarajan A, Beena PM, Devnikar AV, Mali S. A systemic review on tuberculosis. Indian J Tuberc (2020) 67(3):295–311. doi: 10.1016/j.ijtb.2020.02.005
3. Eurosurveillance editorial t. World tuberculosis day 2021: keeping an eye on the 2030 target. Euro Surveill (2021) 26(11). doi: 10.2807/1560-7917.ES.2021.26.11.2103181
4. Turner RD, Bothamley GH. Cough and the transmission of tuberculosis. J Infect Dis (2015) 211(9):1367–72. doi: 10.1093/infdis/jiu625
5. MaChado D, Couto I, Viveiros M. Advances in the molecular diagnosis of tuberculosis: from probes to genomes. Infect Genet Evol (2019) 72:93–112. doi: 10.1016/j.meegid.2018.11.021
6. Steingart KR, Henry M, Ng V, Hopewell PC, Ramsay A, Cunningham J, et al. Fluorescence versus conventional sputum smear microscopy for tuberculosis: A systematic review. Lancet Infect Dis (2006) 6(9):570–81. doi: 10.1016/S1473-3099(06)70578-3
7. Lebrun L, Espinasse F, Poveda JD, Vincent-Levy-Frebault V. Evaluation of nonradioactive DNA probes for identification of mycobacteria. J Clin Microbiol (1992) 30(9):2476–8. doi: 10.1128/jcm.30.9.2476-2478.1992
8. Ferguson JS, Voelker DR, McCormack FX, Schlesinger LS. Surfactant protein D binds to mycobacterium tuberculosis bacilli and lipoarabinomannan via carbohydrate-lectin interactions resulting in reduced phagocytosis of the bacteria by macrophages. J Immunol (1999) 163(1):312–21. doi: 10.4049/jimmunol.163.1.312
9. Wang L, Wu J, Li J, Yang H, Tang T, Liang H, et al. Host-mediated ubiquitination of a mycobacterial protein suppresses immunity. Nature (2020) 577(7792):682–8. doi: 10.1038/s41586-019-1915-7
10. Wilk AJ, Rustagi A, Zhao NQ, Roque J, Martinez-Colon GJ, McKechnie JL, et al. A single-cell atlas of the peripheral immune response in patients with severe Covid-19. Nat Med (2020) 26(7):1070–6. doi: 10.1038/s41591-020-0944-y
11. Ho DW, Tsui YM, Chan LK, Sze KM, Zhang X, Cheu JW, et al. Single-cell Rna sequencing shows the immunosuppressive landscape and tumor heterogeneity of Hbv-associated hepatocellular carcinoma. Nat Commun (2021) 12(1):3684. doi: 10.1038/s41467-021-24010-1
12. Adil A, Kumar V, Jan AT, Asger M. Single-cell transcriptomics: current methods and challenges in data acquisition and analysis. Front Neurosci (2021) 15:591122. doi: 10.3389/fnins.2021.591122
13. Kumar P, Kiran S, Saha S, Su Z, Paulsen T, Chatrath A, et al. Atac-seq identifies thousands of extrachromosomal circular DNA in cancer and cell lines. Sci Adv (2020) 6(20):eaba2489. doi: 10.1126/sciadv.aba2489
14. Saichi M, Ladjemi MZ, Korniotis S, Rousseau C, Ait Hamou Z, Massenet-Regad L, et al. Single-cell Rna Sequencing of Blood antigen-presenting cells in severe Covid-19 reveals multi-process defects in antiviral immunity. Nat Cell Biol (2021) 23(5):538–51. doi: 10.1038/s41556-021-00681-2
15. Tang F, Barbacioru C, Wang Y, Nordman E, Lee C, Xu N, et al. Mrna-seq whole-transcriptome analysis of a single cell. Nat Methods (2009) 6(5):377–82. doi: 10.1038/nmeth.1315
16. Lei Y, Tang R, Xu J, Wang W, Zhang B, Liu J, et al. Applications of single-cell sequencing in cancer research: progress and perspectives. J Hematol Oncol (2021) 14(1):91. doi: 10.1186/s13045-021-01105-2
17. Kolodziejczyk AA, Kim JK, Svensson V, Marioni JC, Teichmann SA. The technology and biology of single-cell Rna sequencing. Mol Cell (2015) 58(4):610–20. doi: 10.1016/j.molcel.2015.04.005
18. Islam S, Kjallquist U, Moliner A, Zajac P, Fan JB, Lonnerberg P, et al. Characterization of the single-cell transcriptional landscape by highly multiplex Rna-seq. Genome Res (2011) 21(7):1160–7. doi: 10.1101/gr.110882.110
19. Ramskold D, Luo S, Wang YC, Li R, Deng Q, Faridani OR, et al. Full-length mrna-seq from single-cell levels of Rna and individual circulating tumor cells. Nat Biotechnol (2012) 30(8):777–82. doi: 10.1038/nbt.2282
20. Hashimshony T, Wagner F, Sher N, Yanai I. Cel-seq: single-cell Rna-seq by multiplexed linear amplification. Cell Rep (2012) 2(3):666–73. doi: 10.1016/j.celrep.2012.08.003
21. Picelli S, Bjorklund AK, Faridani OR, Sagasser S, Winberg G, Sandberg R. Smart-seq2 for sensitive full-length transcriptome profiling in single cells. Nat Methods (2013) 10(11):1096–8. doi: 10.1038/nmeth.2639
22. Islam S, Zeisel A, Joost S, La Manno G, Zajac P, Kasper M, et al. Quantitative single-cell Rna-seq with unique molecular identifiers. Nat Methods (2014) 11(2):163–6. doi: 10.1038/nmeth.2772
23. Sasagawa Y, Nikaido I, Hayashi T, Danno H, Uno KD, Imai T, et al. Quartz-seq: A highly reproducible and sensitive single-cell Rna sequencing method, reveals non-genetic gene-expression heterogeneity. Genome Biol (2013) 14(4):R31. doi: 10.1186/gb-2013-14-4-r31
24. Jaitin DA, Kenigsberg E, Keren-Shaul H, Elefant N, Paul F, Zaretsky I, et al. Massively parallel single-cell Rna-seq for marker-free decomposition of tissues into cell types. Science (2014) 343(6172):776–9. doi: 10.1126/science.1247651
25. Macosko EZ, Basu A, Satija R, Nemesh J, Shekhar K, Goldman M, et al. Highly parallel genome-wide expression profiling of individual cells using nanoliter droplets. Cell (2015) 161(5):1202–14. doi: 10.1016/j.cell.2015.05.002
26. Keren-Shaul H, Kenigsberg E, Jaitin DA, David E, Paul F, Tanay A, et al. Mars-seq2.0: an experimental and analytical pipeline for indexed sorting combined with single-cell Rna sequencing. Nat Protoc (2019) 14(6):1841–62. doi: 10.1038/s41596-019-0164-4
27. Klein AM, Mazutis L, Akartuna I, Tallapragada N, Veres A, Li V, et al. Droplet barcoding for single-cell transcriptomics applied to embryonic stem cells. Cell (2015) 161(5):1187–201. doi: 10.1016/j.cell.2015.04.044
28. Pederson T. The spatial organization of the genome in mammalian cells. Curr Opin Genet Dev (2004) 14(2):203–9. doi: 10.1016/j.gde.2004.02.008
29. Jackson DA. Nuclear organization: uniting replication foci, chromatin domains and chromosome structure. Bioessays (1995) 17(7):587–91. doi: 10.1002/bies.950170704
30. Thurman RE, Rynes E, Humbert R, Vierstra J, Maurano MT, Haugen E, et al. The accessible chromatin landscape of the human genome. Nature (2012) 489(7414):75–82. doi: 10.1038/nature11232
31. Geraldes I, Fernandes M, Fraga AG, Osorio NS. The impact of single-cell genomics on the field of mycobacterial infection. Front Microbiol (2022) 13:989464. doi: 10.3389/fmicb.2022.989464
32. Kasinathan S, Orsi GA, Zentner GE, Ahmad K, Henikoff S. High-resolution mapping of transcription factor binding sites on native chromatin. Nat Methods (2014) 11(2):203–9. doi: 10.1038/nmeth.2766
33. Schmid M, Durussel T, Laemmli UK. Chic and chec; genomic mapping of chromatin proteins. Mol Cell (2004) 16(1):147–57. doi: 10.1016/j.molcel.2004.09.007
34. Boyle AP, Davis S, Shulha HP, Meltzer P, Margulies EH, Weng Z, et al. High-resolution mapping and characterization of open chromatin across the genome. Cell (2008) 132(2):311–22. doi: 10.1016/j.cell.2007.12.014
35. Lay FD, Liu Y, Kelly TK, Witt H, Farnham PJ, Jones PA, et al. The role of DNA methylation in directing the functional organization of the cancer epigenome. Genome Res (2015) 25(4):467–77. doi: 10.1101/gr.183368.114
36. Buenrostro JD, Giresi PG, Zaba LC, Chang HY, Greenleaf WJ. Transposition of native chromatin for fast and sensitive epigenomic profiling of open chromatin, DNA-binding proteins and nucleosome position. Nat Methods (2013) 10(12):1213–8. doi: 10.1038/nmeth.2688
37. Picelli S, Bjorklund AK, Reinius B, Sagasser S, Winberg G, Sandberg R. Tn5 transposase and tagmentation procedures for massively scaled sequencing projects. Genome Res (2014) 24(12):2033–40. doi: 10.1101/gr.177881.114
38. Gawad C, Koh W, Quake SR. Single-cell genome sequencing: current state of the science. Nat Rev Genet (2016) 17(3):175–88. doi: 10.1038/nrg.2015.16
39. Navin NE. Cancer genomics: one cell at a time. Genome Biol (2014) 15(8):452. doi: 10.1186/s13059-014-0452-9
40. Gole J, Gore A, Richards A, Chiu YJ, Fung HL, Bushman D, et al. Massively parallel polymerase cloning and genome sequencing of single cells using nanoliter microwells. Nat Biotechnol (2013) 31(12):1126–32. doi: 10.1038/nbt.2720
41. White AK, VanInsberghe M, Petriv OI, Hamidi M, Sikorski D, Marra MA, et al. High-throughput microfluidic single-cell rt-qpcr. Proc Natl Acad Sci U.S.A. (2011) 108(34):13999–4004. doi: 10.1073/pnas.1019446108
42. Leung K, Zahn H, Leaver T, Konwar KM, Hanson NW, Page AP, et al. A programmable droplet-based microfluidic device applied to multiparameter analysis of single microbes and microbial communities. Proc Natl Acad Sci U.S.A. (2012) 109(20):7665–70. doi: 10.1073/pnas.1106752109
43. Dean FB, Nelson JR, Giesler TL, Lasken RS. Rapid amplification of plasmid and phage DNA using phi 29 DNA polymerase and multiply-primed rolling circle amplification. Genome Res (2001) 11(6):1095–9. doi: 10.1101/gr.180501
44. Telenius H, Carter NP, Bebb CE, Nordenskjold M, Ponder BA, Tunnacliffe A. Degenerate oligonucleotide-primed pcr: general amplification of target DNA by a single degenerate primer. Genomics (1992) 13(3):718–25. doi: 10.1016/0888-7543(92)90147-k
45. Fu Y, Li C, Lu S, Zhou W, Tang F, Xie XS, et al. Uniform and accurate single-cell sequencing based on emulsion whole-genome amplification. Proc Natl Acad Sci U.S.A. (2015) 112(38):11923–8. doi: 10.1073/pnas.1513988112
46. Zong C, Lu S, Chapman AR, Xie XS. Genome-wide detection of single-nucleotide and copy-number variations of a single human cell. Science (2012) 338(6114):1622–6. doi: 10.1126/science.1229164
47. Hou Y, Wu K, Shi X, Li F, Song L, Wu H, et al. Comparison of variations detection between whole-genome amplification methods used in single-cell resequencing. Gigascience (2015) 4:37. doi: 10.1186/s13742-015-0068-3
48. Stahl PL, Salmen F, Vickovic S, Lundmark A, Navarro JF, Magnusson J, et al. Visualization and analysis of gene expression in tissue sections by spatial transcriptomics. Science (2016) 353(6294):78–82. doi: 10.1126/science.aaf2403
49. Williams CG, Lee HJ, Asatsuma T, Vento-Tormo R, Haque A. An introduction to spatial transcriptomics for biomedical research. Genome Med (2022) 14(1):68. doi: 10.1186/s13073-022-01075-1
50. Moses L, Pachter L. Museum of spatial transcriptomics. Nat Methods (2022) 19(5):534–46. doi: 10.1038/s41592-022-01409-2
51. Femino AM, Fay FS, Fogarty K, Singer RH. Visualization of single Rna transcripts in situ. Science (1998) 280(5363):585–90. doi: 10.1126/science.280.5363.585
52. Asp M, Bergenstrahle J, Lundeberg J. Spatially resolved transcriptomes-next generation tools for tissue exploration. Bioessays (2020) 42(10):e1900221. doi: 10.1002/bies.201900221
53. Lubeck E, Coskun AF, Zhiyentayev T, Ahmad M, Cai L. Single-cell in situ Rna profiling by sequential hybridization. Nat Methods (2014) 11(4):360–1. doi: 10.1038/nmeth.2892
54. Maynard KR, Collado-Torres L, Weber LM, Uytingco C, Barry BK, Williams SR, et al. Transcriptome-scale spatial gene expression in the human dorsolateral prefrontal cortex. Nat Neurosci (2021) 24(3):425–36. doi: 10.1038/s41593-020-00787-0
55. Chen KH, Boettiger AN, Moffitt JR, Wang S, Zhuang X. Rna imaging. Spatially resolved, highly multiplexed Rna profiling in single cells. Science (2015) 348(6233):aaa6090. doi: 10.1126/science.aaa6090
56. Borm LE, Mossi Albiach A, Mannens CCA, Janusauskas J, Ozgun C, Fernandez-Garcia D, et al. Scalable in situ single-cell profiling by electrophoretic capture of mrna using eel fish. Nat Biotechnol (2023) 41(2):222–31. doi: 10.1038/s41587-022-01455-3
57. Ke R, Mignardi M, Pacureanu A, Svedlund J, Botling J, Wahlby C, et al. In situ sequencing for Rna analysis in preserved tissue and cells. Nat Methods (2013) 10(9):857–60. doi: 10.1038/nmeth.2563
58. Chen X, Sun YC, Church GM, Lee JH, Zador AM. Efficient in situ barcode sequencing using padlock probe-based baristaseq. Nucleic Acids Res (2018) 46(4):e22. doi: 10.1093/nar/gkx1206
59. Lee JH, Daugharthy ER, Scheiman J, Kalhor R, Ferrante TC, Terry R, et al. Fluorescent in situ sequencing (Fisseq) of Rna for gene expression profiling in intact cells and tissues. Nat Protoc (2015) 10(3):442–58. doi: 10.1038/nprot.2014.191
60. Wang X, Allen WE, Wright MA, Sylwestrak EL, Samusik N, Vesuna S, et al. Three-dimensional intact-tissue sequencing of single-cell transcriptional states. Science (2018) 361(6400):eaat5691. doi: 10.1126/science.aat5691
61. Rodriques SG, Stickels RR, Goeva A, Martin CA, Murray E, Vanderburg CR, et al. Slide-seq: A scalable technology for measuring genome-wide expression at high spatial resolution. Science (2019) 363(6434):1463–7. doi: 10.1126/science.aaw1219
62. Vickovic S, Eraslan G, Salmen F, Klughammer J, Stenbeck L, Schapiro D, et al. High-definition spatial transcriptomics for in situ tissue profiling. Nat Methods (2019) 16(10):987–90. doi: 10.1038/s41592-019-0548-y
63. Wang XM, Zhang JY, Xing X, Huang HH, Xia P, Dai XP, et al. Global transcriptomic characterization of T cells in individuals with chronic hiv-1 infection. Cell Discovery (2022) 8(1):29. doi: 10.1038/s41421-021-00367-x
64. Ziegler CGK, Allon SJ, Nyquist SK, Mbano IM, Miao VN, Tzouanas CN, et al. Sars-cov-2 receptor ace2 is an interferon-stimulated gene in human airway epithelial cells and is detected in specific cell subsets across tissues. Cell (2020) 181(5):1016–35 e19. doi: 10.1016/j.cell.2020.04.035
65. Yi X, Zhou H, Chao Y, Xiong S, Zhong J, Chai Z, et al. Bacteria-triggered tumor-specific thrombosis to enable potent photothermal immunotherapy of cancer. Sci Adv (2020) 6(33):eaba3546. doi: 10.1126/sciadv.aba3546
66. Ramachandran P, Matchett KP, Dobie R, Wilson-Kanamori JR, Henderson NC. Single-cell technologies in hepatology: new insights into liver biology and disease pathogenesis. Nat Rev Gastroenterol Hepatol (2020) 17(8):457–72. doi: 10.1038/s41575-020-0304-x
67. Gierahn TM, Wadsworth MH 2nd, Hughes TK, Bryson BD, Butler A, Satija R, et al. Seq-well: portable, low-cost Rna sequencing of single cells at high throughput. Nat Methods (2017) 14(4):395–8. doi: 10.1038/nmeth.4179
68. Bossel Ben-Moshe N, Hen-Avivi S, Levitin N, Yehezkel D, Oosting M, Joosten LAB, et al. Predicting bacterial infection outcomes using single cell Rna-sequencing analysis of human immune cells. Nat Commun (2019) 10(1):3266. doi: 10.1038/s41467-019-11257-y
69. Cai Y, Dai Y, Wang Y, Yang Q, Guo J, Wei C, et al. Single-cell transcriptomics of blood reveals a natural killer cell subset depletion in tuberculosis. EBioMedicine (2020) 53:102686. doi: 10.1016/j.ebiom.2020.102686
70. Khan N, Downey J, Sanz J, Kaufmann E, Blankenhaus B, Pacis A, et al. M. Tuberculosis reprograms hematopoietic stem cells to limit myelopoiesis and impair trained immunity. Cell (2020) 183(3):752–70 e22. doi: 10.1016/j.cell.2020.09.062
71. Pisu D, Huang L, Narang V, Theriault M, Le-Bury G, Lee B, et al. Single cell analysis of M. Tuberculosis phenotype and macrophage lineages in the infected lung. J Exp Med (2021) 218(9):e20210615. doi: 10.1084/jem.20210615
72. Nathan A, Beynor JI, Baglaenko Y, Suliman S, Ishigaki K, Asgari S, et al. Multimodally profiling memory T cells from a tuberculosis cohort identifies cell state associations with demographics, environment and disease. Nat Immunol (2021) 22(6):781–93. doi: 10.1038/s41590-021-00933-1
73. Cronan MR, Hughes EJ, Brewer WJ, Viswanathan G, Hunt EG, Singh B, et al. A non-canonical type 2 immune response coordinates tuberculous granuloma formation and epithelialization. Cell (2021) 184(7):1757–74 e14. doi: 10.1016/j.cell.2021.02.046
74. Esaulova E, Das S, Singh DK, Choreno-Parra JA, Swain A, Arthur L, et al. The immune landscape in tuberculosis reveals populations linked to disease and latency. Cell Host Microbe (2021) 29(2):165–78 e8. doi: 10.1016/j.chom.2020.11.013
75. Gideon HP, Hughes TK, Tzouanas CN, Wadsworth MH 2nd, Tu AA, Gierahn TM, et al. Multimodal profiling of lung granulomas in macaques reveals cellular correlates of tuberculosis control. Immunity (2022) 55(5):827–46 e10. doi: 10.1016/j.immuni.2022.04.004
76. Akter S, Chauhan KS, Dunlap MD, Choreno-Parra JA, Lu L, Esaulova E, et al. Mycobacterium tuberculosis infection drives a type I Ifn signature in lung lymphocytes. Cell Rep (2022) 39(12):110983. doi: 10.1016/j.celrep.2022.110983
77. Xu Y, Tan Y, Zhang X, Cheng M, Hu J, Liu J, et al. Comprehensive identification of immuno-related transcriptional signature for active pulmonary tuberculosis by integrated analysis of array and single cell Rna-seq. J Infect (2022) 85(5):534–44. doi: 10.1016/j.jinf.2022.08.017
78. Chen Q, Hu C, Lu W, Hang T, Shao Y, Chen C, et al. Characteristics of alveolar macrophages in bronchioalveolar lavage fluids from active tuberculosis patients identified by single-cell Rna sequencing. J BioMed Res (2022) 36(3):167–80. doi: 10.7555/JBR.36.20220007
79. Shao MM, Yi FS, Huang ZY, Peng P, Wu FY, Shi HZ, et al. T cell receptor repertoire analysis reveals signatures of T cell responses to human mycobacterium tuberculosis. Front Microbiol (2022) 13:829694. doi: 10.3389/fmicb.2022.829694
80. Oelen R, de Vries DH, Brugge H, Gordon MG, Vochteloo M, Ye CJ, et al. Single-Cell Rna-Sequencing of Peripheral blood mononuclear cells reveals widespread, context-specific gene expression regulation upon pathogenic exposure. Nat Commun (2022) 13(1):3267. doi: 10.1038/s41467-022-30893-5. single-cell e Qc.
81. Wang Y, Sun Q, Zhang Y, Li X, Liang Q, Guo R, et al. Systemic immune dysregulation in severe tuberculosis patients revealed by a single-cell transcriptome atlas. J Infect (2023) 86(5):421–38. doi: 10.1016/j.jinf.2023.03.020
82. Musvosvi M, Huang H, Wang C, Xia Q, Rozot V, Krishnan A, et al. T cell receptor repertoires associated with control and disease progression following mycobacterium tuberculosis infection. Nat Med (2023) 29(1):258–69. doi: 10.1038/s41591-022-02110-9
83. Yang X, Yan J, Xue Y, Sun Q, Zhang Y, Guo R, et al. Single-cell profiling reveals distinct immune response landscapes in tuberculous pleural effusion and non-tpe. Front Immunol (2023) 14:1191357. doi: 10.3389/fimmu.2023.1191357
84. Cohen SB, Gern BH, Delahaye JL, Adams KN, Plumlee CR, Winkler JK, et al. Alveolar macrophages provide an early mycobacterium tuberculosis niche and initiate dissemination. Cell Host Microbe (2018) 24(3):439–46 e4. doi: 10.1016/j.chom.2018.08.001
85. Lin PL, Ford CB, Coleman MT, Myers AJ, Gawande R, Ioerger T, et al. Sterilization of granulomas is common in active and latent tuberculosis despite within-host variability in bacterial killing. Nat Med (2014) 20(1):75–9. doi: 10.1038/nm.3412
86. Ehlers S, Schaible UE. The granuloma in tuberculosis: dynamics of a host-pathogen collusion. Front Immunol (2012) 3:411. doi: 10.3389/fimmu.2012.00411
87. Pagan AJ, Ramakrishnan L. Immunity and immunopathology in the tuberculous granuloma. Cold Spring Harb Perspect Med (2014) 5(9):a018499. doi: 10.1101/cshperspect.a018499
88. Carow B, Hauling T, Qian X, Kramnik I, Nilsson M, Rottenberg ME. Spatial and temporal localization of immune transcripts defines hallmarks and diversity in the tuberculosis granuloma. Nat Commun (2019) 10(1):1823. doi: 10.1038/s41467-019-09816-4
89. Mayer-Barber KD, Barber DL. Innate and adaptive cellular immune responses to mycobacterium tuberculosis infection. Cold Spring Harb Perspect Med (2015) 5(12):a018424. doi: 10.1101/cshperspect.a018424
90. Cronan MR, Beerman RW, Rosenberg AF, Saelens JW, Johnson MG, Oehlers SH, et al. Macrophage epithelial reprogramming underlies mycobacterial granuloma formation and promotes infection. Immunity (2016) 45(4):861–76. doi: 10.1016/j.immuni.2016.09.014
91. Mitra DK, Sharma SK, Dinda AK, Bindra MS, Madan B, Ghosh B. Polarized helper T cells in tubercular pleural effusion: phenotypic identity and selective recruitment. Eur J Immunol (2005) 35(8):2367–75. doi: 10.1002/eji.200525977
92. Shaw JA, Diacon AH, Koegelenberg CFN. Tuberculous pleural effusion. Respirology (2019) 24(10):962–71. doi: 10.1111/resp.13673
93. Du J, Shao MM, Yi FS, Huang ZY, Qiao X, Chen QY, et al. Interleukin 32 as a potential marker for diagnosis of tuberculous pleural effusion. Microbiol Spectr (2022) 10(4):e0255321. doi: 10.1128/spectrum.02553-21
94. Cai Y, Wang Y, Shi C, Dai Y, Li F, Xu Y, et al. Single-cell immune profiling reveals functional diversity of T cells in tuberculous pleural effusion. J Exp Med (2022) 219(3):e20211777. doi: 10.1084/jem.20211777
95. Boom WH, Schaible UE, Achkar JM. The knowns and unknowns of latent mycobacterium tuberculosis infection. J Clin Invest (2021) 131(3):e136222. doi: 10.1172/JCI136222
96. Rahlwes KC, Dias BRS, Campos PC, Alvarez-Arguedas S, Shiloh MU. Pathogenicity and virulence of mycobacterium tuberculosis. Virulence (2023) 14(1):2150449. doi: 10.1080/21505594.2022.2150449
97. Harari A, Rozot V, Bellutti Enders F, Perreau M, Stalder JM, Nicod LP, et al. Dominant Tnf-alpha+ Mycobacterium tuberculosis-specific cd4+ T cell responses discriminate between latent infection and active disease. Nat Med (2011) 17(3):372–6. doi: 10.1038/nm.2299
98. Liu CH, Liu H, Ge B. Innate immunity in tuberculosis: host defense vs pathogen evasion. Cell Mol Immunol (2017) 14(12):963–75. doi: 10.1038/cmi.2017.88
99. Lerner TR, Borel S, Gutierrez MG. The innate immune response in human tuberculosis. Cell Microbiol (2015) 17(9):1277–85. doi: 10.1111/cmi.12480
100. Jasenosky LD, Scriba TJ, Hanekom WA, Goldfeld AE. T cells and adaptive immunity to mycobacterium tuberculosis in humans. Immunol Rev (2015) 264(1):74–87. doi: 10.1111/imr.12274
101. Khan N, Vidyarthi A, Amir M, Mushtaq K, Agrewala JN. T-cell exhaustion in tuberculosis: pitfalls and prospects. Crit Rev Microbiol (2017) 43(2):133–41. doi: 10.1080/1040841X.2016.1185603
102. Zhai W, Wu F, Zhang Y, Fu Y, Liu Z. The immune escape mechanisms of mycobacterium tuberculosis. Int J Mol Sci (2019) 20(2):340. doi: 10.3390/ijms20020340
103. Wang J, Ge P, Lei Z, Lu Z, Qiang L, Chai Q, et al. Mycobacterium tuberculosis protein kinase G acts as an unusual ubiquitinating enzyme to impair host immunity. EMBO Rep (2021) 22(6):e52175. doi: 10.15252/embr.202052175
104. Chai Q, Wang L, Liu CH, Ge B. New insights into the evasion of host innate immunity by mycobacterium tuberculosis. Cell Mol Immunol (2020) 17(9):901–13. doi: 10.1038/s41423-020-0502-z
105. Santos-Pereira A, Magalhaes C, Araujo PMM, Osorio. NS. Evolutionary genetics of mycobacterium tuberculosis and hiv-1: "The tortoise and the hare". Microorganisms (2021) 9(1):147. doi: 10.3390/microorganisms9010147
106. Hameed HMA, Islam MM, Chhotaray C, Wang C, Liu Y, Tan Y, et al. Molecular targets related drug resistance mechanisms in Mdr-, Xdr-, and Tdr-mycobacterium tuberculosis strains. Front Cell Infect Microbiol (2018) 8:114. doi: 10.3389/fcimb.2018.00114
107. Tiberi S, du Plessis N, Walzl G, Vjecha MJ, Rao M, Ntoumi F, et al. Tuberculosis: progress and advances in development of new drugs, treatment regimens, and host-directed therapies. Lancet Infect Dis (2018) 18(7):e183–e98. doi: 10.1016/S1473-3099(18)30110-5
108. Zumla A, Nahid P, Cole ST. Advances in the development of new tuberculosis drugs and treatment regimens. Nat Rev Drug Discovery (2013) 12(5):388–404. doi: 10.1038/nrd4001
109. Sensi P. History of the development of rifampin. Rev Infect Dis (1983) 5 Suppl 3:S402–6. doi: 10.1093/clinids/5.supplement_3.s402
110. van Ingen J, Aarnoutse RE, Donald PR, Diacon AH, Dawson R, Plemper van Balen G, et al. Why do we use 600 mg of rifampicin in tuberculosis treatment? Clin Infect Dis (2011) 52(9):e194–9. doi: 10.1093/cid/cir184
111. Rattan A, Kalia A, Ahmad N. Multidrug-resistant mycobacterium tuberculosis: molecular perspectives. Emerg Infect Dis (1998) 4(2):195–209. doi: 10.3201/eid0402.980207
112. Zaw MT, Emran NA, Lin Z. Mutations inside rifampicin-resistance determining region of rpob gene associated with rifampicin-resistance in mycobacterium tuberculosis. J Infect Public Health (2018) 11(5):605–10. doi: 10.1016/j.jiph.2018.04.005
113. Lai C, Xu J, Tozawa Y, Okamoto-Hosoya Y, Yao X, Ochi K. Genetic and physiological characterization of rpob mutations that activate antibiotic production in streptomyces lividans. Microbiol (Reading) (2002) 148(Pt 11):3365–73. doi: 10.1099/00221287-148-11-3365
114. Rifat D, Prideaux B, Savic RM, Urbanowski ME, Parsons TL, Luna B, et al. Pharmacokinetics of rifapentine and rifampin in a rabbit model of tuberculosis and correlation with clinical trial data. Sci Transl Med (2018) 10(435):eaai7786. doi: 10.1126/scitranslmed.aai7786
115. Mangtani P, Abubakar I, Ariti C, Beynon R, Pimpin L, Fine PE, et al. Protection by Bcg vaccine against tuberculosis: A systematic review of randomized controlled trials. Clin Infect Dis (2014) 58(4):470–80. doi: 10.1093/cid/cit790
116. Kong LJ, Moorlag SJCFM, Lefkovith A, Li BH, Matzaraki V, Van Emst L, et al. Single-cell transcriptomic profiles reveal changes associated with Bcg-induced trained immunity and protective effects in circulating monocytes. Cell Rep (2021) 37(7):110028. doi: 10.1016/j.celrep.2021.110028
117. Luo G, Gao Q, Zhang S, Yan B. Probing infectious disease by single-cell Rna sequencing: progresses and perspectives. Comput Struct Biotechnol J (2020) 18:2962–71. doi: 10.1016/j.csbj.2020.10.016
118. Burel JG, Pomaznoy M, Lindestam Arlehamn CS, Weiskopf D, da Silva Antunes R, Jung Y, et al. Circulating T cell-monocyte complexes are markers of immune perturbations. Elife (2019) 8:e46045. doi: 10.7554/eLife.46045
119. Sawyer AJ, Patrick E, Edwards J, Wilmott JS, Fielder T, Yang Q, et al. Spatial mapping reveals granuloma diversity and histopathological superstructure in human tuberculosis. J Exp Med (2023) 220(6):e20221392. doi: 10.1084/jem.20221392
120. Siegl A, Kamke J, Hochmuth T, Piel J, Richter M, Liang C, et al. Single-cell genomics reveals the lifestyle of poribacteria, a candidate phylum symbiotically associated with marine sponges. ISME J (2011) 5(1):61–70. doi: 10.1038/ismej.2010.95
121. Grindberg RV, Ishoey T, Brinza D, Esquenazi E, Coates RC, Liu WT, et al. Single cell genome amplification accelerates identification of the apratoxin biosynthetic pathway from a complex microbial assemblage. PloS One (2011) 6(4):e18565. doi: 10.1371/journal.pone.0018565
122. Coppola M, Ottenhoff TH. Genome wide approaches discover novel mycobacterium tuberculosis antigens as correlates of infection, disease, immunity and targets for vaccination. Semin Immunol (2018) 39:88–101. doi: 10.1016/j.smim.2018.07.001
123. Zatarain-Barron ZL, Ramos-Espinosa O, Marquina-Castillo B, Barrios-Payan J, Cornejo-Granados F, Maya-Lucas O, et al. Evidence for the effect of vaccination on host-pathogen interactions in a murine model of pulmonary tuberculosis by mycobacterium tuberculosis. Front Immunol (2020) 11:930. doi: 10.3389/fimmu.2020.00930
124. Bertelli C, Greub G. Rapid bacterial genome sequencing: methods and applications in clinical microbiology. Clin Microbiol Infect (2013) 19(9):803–13. doi: 10.1111/1469-0691.12217
125. Mahdessian D, Cesnik AJ, Gnann C, Danielsson F, Stenstrom L, Arif M, et al. Spatiotemporal dissection of the cell cycle with single-cell proteogenomics. Nature (2021) 590(7847):649–54. doi: 10.1038/s41586-021-03232-9
126. Wu FL, Liu Y, Jiang HW, Luan YZ, Zhang HN, He X, et al. The ser/thr protein kinase protein-protein interaction map of M. Tuberculosis. Mol Cell Proteomics (2017) 16(8):1491–506. doi: 10.1074/mcp.M116.065771
127. Ahamad N, Gupta S, Parashar D. Using omics to study leprosy, tuberculosis, and other mycobacterial diseases. Front Cell Infect Microbiol (2022) 12:792617. doi: 10.3389/fcimb.2022.792617
128. Pitaloka DAE, Syamsunarno M, Abdulah R, Chaidir L. Omics biomarkers for monitoring tuberculosis treatment: A mini-review of recent insights and future approaches. Infect Drug Resist (2022) 15:2703–11. doi: 10.2147/IDR.S366580
129. Li X, Chen W, Chen Y, Zhang X, Gu J, Zhang MQ. Network embedding-based representation learning for single cell Rna-seq data. Nucleic Acids Res (2017) 45(19):e166. doi: 10.1093/nar/gkx750
130. Butler A, Hoffman P, Smibert P, Papalexi E, Satija R. Integrating single-cell transcriptomic data across different conditions, technologies, and species. Nat Biotechnol (2018) 36(5):411–20. doi: 10.1038/nbt.4096
Keywords: single-cell sequencing, scRNA-seq, tuberculosis, Mycobacterium tuberculosis, host-pathogen interaction
Citation: Pan J, Chang Z, Zhang X, Dong Q, Zhao H, Shi J and Wang G (2023) Research progress of single-cell sequencing in tuberculosis. Front. Immunol. 14:1276194. doi: 10.3389/fimmu.2023.1276194
Received: 14 August 2023; Accepted: 29 September 2023;
Published: 13 October 2023.
Edited by:
Mohlopheni Jackson Marakalala, Africa Health Research Institute (AHRI), South AfricaReviewed by:
Quan Zou, University of Electronic Science and Technology of China, ChinaThabo Mpotje, Africa Health Research Institute (AHRI), South Africa
Copyright © 2023 Pan, Chang, Zhang, Dong, Zhao, Shi and Wang. This is an open-access article distributed under the terms of the Creative Commons Attribution License (CC BY). The use, distribution or reproduction in other forums is permitted, provided the original author(s) and the copyright owner(s) are credited and that the original publication in this journal is cited, in accordance with accepted academic practice. No use, distribution or reproduction is permitted which does not comply with these terms.
*Correspondence: Guoqing Wang, cWluZ0BqbHUuZWR1LmNu; Jingwei Shi, c2hpamluZ3dlaUBqbHUuZWR1LmNu
†These authors have contributed equally to this work