- 1Rheumatology Division, Bone Metabolism Laboratory, Faculdade de Medicina, Universidade de São Paulo, São Paulo, Brazil
- 2Department of Internal Medicine 3-Rheumatology and Immunology, Friedrich Alexander Universität Erlangen-Nürnberg and Universitätsklinikum Erlangen, Erlangen, Germany
Introduction: Evidence-based data suggest that under inflammatory conditions, classical monocytes are the main source of osteoclasts and might be involved in bone erosion pathophysiology. Here, we analyze the transcriptomic profile of classical monocytes in erosive and non-erosive rheumatoid arthritis patients in order to better understand their contribution to bone erosion.
Methods: Thirty-nine premenopausal RA patients were consecutively enrolled and divided into two groups based on the presence of bone erosions on hand joints. Classical monocytes were isolated from peripheral blood through negative selection, and RNA-seq was performed using a poly-A enrichment kit and Illumina® platform. Classical monocytes transcriptome from healthy age-matched women were also included to identify differentially expressed genes (DEGs). Therefore, gene sets analysis was performed to identify the enriched biological pathways.
Results: RNA-seq analysis resulted in the identification of 1,140 DEGs of which 89 were up-regulated and 1,051 down-regulated in RA patients with bone erosion compared to those without bone erosions. Among up-regulated genes, there was a highlighted expression of IL18RAP and KLF14 related to the production of pro-inflammatory cytokines, innate and adaptive immune response. Genes related to collagen metabolism (LARP6) and bone formation process (PAPPA) were down-regulated in RA patients with erosions. Enriched pathways in patients with erosions were associated with greater activation of immune activation, and inflammation. Interestingly, pathways associated with osteoblast differentiation and regulation of Wnt signaling were less activated in RA patients with erosions.
Conclusion: These findings suggest that alterations in expression of monocyte genes related to the inflammatory process and impairment of bone formation might have an important role in the pathophysiology of bone erosions in RA patients.
1 Introduction
Rheumatoid arthritis (RA) is an autoimmune disease characterized by articular and extra-articular bone loss (1). Bone erosions, the hallmark lesions of RA, are induced by inflammation and are important predictor of disability, disease severity, and mortality in RA. Bone erosion pathophysiology involves the activation of immune cell clusters such as T cells, B cells, and monocytes/macrophages (2).
It has been demonstrated that classical monocytes subset represents about 80-90% of the total monocytes and secretes high levels of pro-inflammatory cytokines such as tumor necrosis factor alpha (TNF-α), interleukin-1 beta (IL-1β), and interleukin-6 (IL-6) in response to immune complex activation, and lipopolysaccharide stimulation (3). This subset also express the chemokine receptor CCR2 (4), which is related to transmigration of classical monocytes to inflammatory sites (5).
Considering that under inflammatory conditions, classical monocytes are an important source of pro-inflammatory cytokine production and are the main source of precursor of osteoclasts (6), these cells could represent an important link between inflammation and bone erosion in RA. Even in healthy individuals, classical monocytes express genes involved in immune responses, inflammatory process and tissue remodeling, such as S100A12, S100A9, CSF3R, and MMP25 (7). Therefore, changes in gene expression of classical monocytes may substantially influence inflammation and bone loss in patients with RA.
Although evidence suggest that classical monocytes play a key role in supporting inflammation in RA patients, no data regarding the influence of these cells on bone destruction exists. Thus, the aim of the present study was to investigate the changes of the classical monocyte transcriptomic profile in erosive RA patients as compared to non-erosive patients.
2 Materials and methods
2.1 Study design and participants
Thirty-nine premenopausal RA women regularly followed in the RA Outpatient Clinic at the Hospital das Clinicas da Universidade de São Paulo, were recruited for this study. All patients fulfilled the American College of Rheumatology/European Alliance of Associations for Rheumatology 2010 classification criteria for RA. Patients were excluded if they presented metabolic bone disease (e.g., rickets, primary hypoparathyroidism, osteomalacia, and Paget’s disease); ii) bone-associated chronic disease (e.g., renal or liver failure, hyperthyroidism, hypothyroidism, and malabsorption); iii) use of any medication interfering with bone metabolism (e.g., prednisone doses higher than 7.5 mg/day, bisphosphonates, and bone-targeted monoclonal antibodies iv) an autoimmune disease other than RA, or v) being pregnant or lactating.
Premenopausal status was assessed through a self-reported information. Participants were asked to complete a questionnaire that included questions about their menstrual history, such as the age of menarche, regularity of menstrual cycles, and the presence or absence of menstrual periods in the past 12 months.
Based on data on bone erosion from high-resolution peripheral quantitative computed tomography (HR-pQCT), RA patients were classified into erosive and non-erosive patients according to the presence or absence of bone erosions on metacarpophalangeal (MCP) and proximal interphalangeal (PIP) joints of the dominant hand.
In addition, 20 age- and body mass index-matched healthy subjects were enrolled to the control group. The study was approved by the local Ethics Committee on Human Research from Sao Paulo University-CAPPesq (#51178115.1.0000.0068). All participants gave written informed consent, in accordance with the principles of the Declaration of Helsinki.
2.2 Clinical and laboratory assessments
Demographic and clinical data were obtained through interviews and medical records of the participants. Erythrocyte sedimentation rate (ESR), C-reactive protein (CRP), rheumatoid factor (RF), and anti–cyclic citrullinated peptide (anti-CCP) antibodies were measured using standard automated methods. Disease activity of RA patients was determined according to simplified disease activity index (SDAI), an activity disease score depicted as a simple linear sum of the follow parameters: tender and swollen joints count based on a 28-joint assessment, patient global assessment of disease activity (0–10), physician global assessment of disease activity (0–10) and CRP in mg/dL (8, 9).
2.3 Evaluation of bone erosions using HR-pQCT
Bone erosions were analyzed in RA patients using HR-pQCT as previously described (10). Briefly, HR-pQCT scans were performed in the second and third MCP and PIP joints of the dominant hand. Images were analyzed using OsiriX Lite (version 11.0.2; 32-bit for MacOS). Erosions were defined as a cortical break detected in two consecutive slices, visible in at least two planes, non-linear in shape, with underlying trabecular bone loss (11).
2.4 Classical monocytes isolation from human peripheral blood
Blood (70 ml) from RA patients and control group were collected, and peripheral blood mononuclear cells (PBMCs) were isolated by Ficoll density and dextran sedimentation. In both studied groups, monocytes were isolated from PBMCs by depletion of non-monocytes cells using an indirect magnetic labeling system. Classical monocytes were indirectly isolated using the CD16 MicroBeads human kit (Miltenyi Biotec®, Germany).
2.5 RNA‐sequencing
Classical monocytes RNA was isolated using the RNeasy Plus Mini (Qiagen®), and RNA integrity number (RIN ≥ 7) was evaluated by the ScreenTape method using Bioanalyser 2100 (Agilent®). RNA library was constructed using the Quant-seq® 30 mRNA-Seq Library Prep Kit (Lexogen®) and sequenced using an Illumina Seq 2500 platform (Illumina®).
2.6 RNA-sequencing data processing
Only high-quality raw sequencing data were included in the present study after trimming to remove low quality sequence and poly-A using BBDuk tool. Therefore, reads were mapped to human reference genome (GRCh38/Ensembl) using STAR aligner (12). Read count matrices were imported in R version 4.1 for downstream analyses.
2.7 Differential expression analysis
Raw read counts were pre-filtered and Differential Expression (DE) analysis based on negative binomial distribution was performed using DESeq2 and compared the transcriptome of RA patients with controls (13). In this first sub-analysis RA patient’s, data were merged in a unique disease group without considering their erosion status. RA patients were then classified in two subgroups based on the presence of erosion. Therefore, DE was performed using a likelihood ratio test (LRT) to compare differences between groups. Finally, a pairwise comparison was performed between the subsets of patients to identify differentially expressed genes (DEGs) in erosion patients group using Wald test (13). In both analyses, DEGs were identified based on fold change of 1.5 and a Benjamini-Hochberg false discovery rate (FDR) using a cut-off set at < 0.05.
2.8 Gene sets enrichment analysis and network reconstruction
Main biological processes associated with the top ranked DEGs were manually curated. Furthermore, more rigorous gene sets enrichment analyses were performed with GSEA and the single-sample GSEA (ssGSEA) tools (14). Threshold of p-value < 0.05 was considered significant. Network constituted by the significant GSEA pathways, and their inter-connected shared genes was reconstructed in Cystoscape (15). Network connectivity was analysed to identify hub genes, based on their centrality (highest degree).
List of relevant genes associated with RA was mined from Open target platform which integrates public data relevant to the association between targets and diseases including data from genetics, somatic mutations, expression analysis, drugs and animal models. The median Open Target association score and the centrality of the up-regulated genes were used to prioritize relevant targets in RA.
2.9 Correlation analysis
Pearson correlation analysis was performed to decipher the relationship between clinical and laboratorial characteristics, and pathway activity obtained from the GSEA score at single sample level (ssGSEA). p-value of < 0.05 were considered significant.
2.10 Volcano plot and heatmap
Volcano plots and Heatmaps were designed using ggplot2 package and the ComplexHeatmap package (16). Heatmap data were scaled by z-score and clustered using hierarchical clustering.
3 Results
3.1 Sample characteristics
The demographic and clinical characteristics of RA patients and control group are demonstrated in Table 1. All patients and controls were premenopausal women. Age, body mass index and disease activity were similar between erosive and non-erosive RA patients. Based on the SDAI scores, both the erosive and non-erosive patients demonstrated a moderate disease severity. The scores remained at 19.1 for erosive and 13.2 for non-erosive patients, showing no marked difference between them (p = 0.140). This moderate severity likely accounts for the consistent CRP levels seen across all groups. Notably, there was no significant variance in CRP levels when comparing either the erosive with non-erosive patients (p = 0.231) or the non-erosive patients with the controls (p = 0.090). In this way, CRP levels remained consistently comparable.
The ESR was statistically significant between different groups as determined by one-way ANOVA. A Bonferroni post hoc test revealed that ESR was higher in erosive RA patients in comparison with non-erosive patients (p = 0.018), as well as with controls (p = 0.022) groups. The positivity for autoantibodies (RF and anti-CCP) was significantly higher in erosive RA patients in comparison to non-erosive patients.
3.2 Circulating classical monocytes exhibit an immuno-inflammatory phenotype in RA patients
Gene expression profile of circulating classical monocytes of RA patients (including erosive and non-erosive RA patients) were compared with controls and showed 1,050 DEGs (Figure 1A) of which 693 were up-regulated and 357 down-regulated. Unsupervised clustering of samples using the expression pattern of the top-50 ranked genes (25 up- and 25 down-regulated) revealed a clear stratification of patients and control different clusters (Figure 1B).
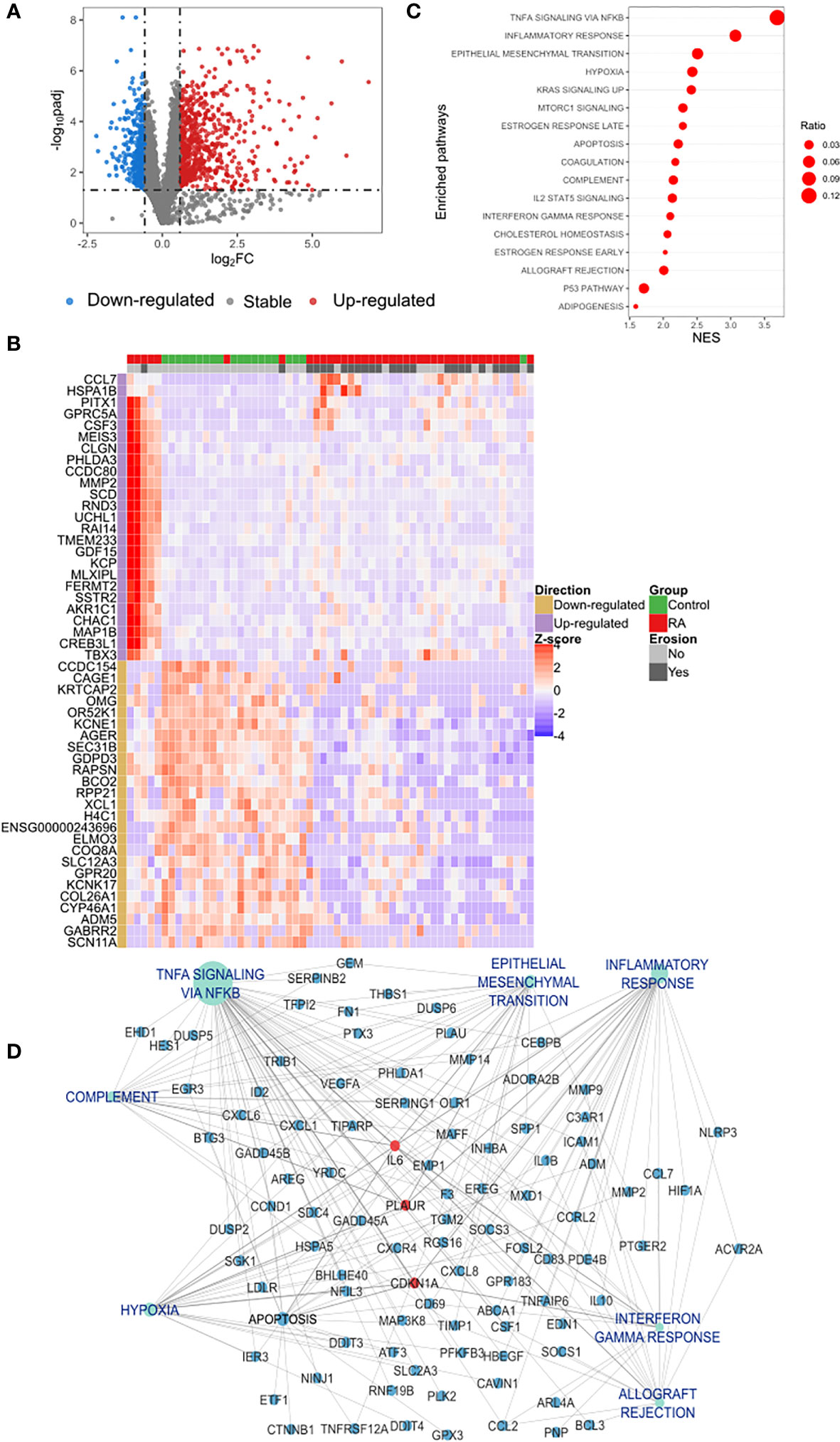
Figure 1 Differential expression analysis of classical monocytes’ genes in RA and functional gene set enrichment analysis (A) Protein coding transcriptome comparison of RA vs. control group showed a differential expression of 1,050 genes of which 693 were up-regulated (red dots) and 357 down-regulated (blue dots). Differentially expressed genes (DEGs) were identified based on a fold change of 1.5 and a Benjamini-Hochberg false discovery rate (FDR) using a cut-off set at < 0.05. (B) Unsupervised clustering of the expression pattern of the top-50 DEGs showed a clear stratification of RA and controls in two different clusters. Hierarchical clustering of samples was performed based on the Euclidean distance calculated from the normalized expression. (C) Dot plot of functional analysis performed using the hallmark gene sets showed the enrichment of biological processes associated with inflammation and immune activation. Dot size represents the ratio of DEGs that are involved each process and the X axis indicates the normalized GSEA enrichment score. Pathways with p-value of < 0.05 was considered significant. (D) Subnetwork of the GSEA showing the hub gene IL-6 connected with the most relevant pathways identified in the functional analysis. DEGs, Differential Expressed Genes; GSEA, Gene Set Enrichment Analysis; NES, Normalized Enrichment Score.
Analyzing the list of DEGs, an enrichment of different families of cytokines and their receptors was observed in the up-regulated list of genes (Supplementary Figure S1). A manual curation of the main biological processes associated with the top 10 up-regulated genes also identified: the predominance of genes involved in inflammation and immune activation (GDF15, CSF3, CCL7, MMP2) as demonstrated in Table 2. In contrast, the main down-regulated genes were found in collagen formation (COL26A1) and bone mineralization (CCDC154) (Table 2).
3.3 Inflammatory response is driven by IL6, TNF and Interferon gamma in RA
To achieve further insights into the pathways that are increased in classical monocytes of RA as compared to controls, a functional gene set enrichment analysis, using all the DEGs identified in RA, was performed. This analysis further confirmed an increase in distinct biological processes associated with inflammation in RA (Figure 1C; Supplementary Figure S2). Furthermore, a network-based pathway was constructed (Supplementary Figure S3A) using the pathways shown in Figure 1C and their associated DEGs. Based on network centrality analysis (Degree), IL6 was identified as the main hub gene, confirming its prominent role in the regulation of the inflammatory response observed in RA (Supplementary Figure S3A). The subnetwork containing only IL6 connections (Figure 1D) also contains two other top-ranked hub genes CDKN1A, and PLAUR, as well as central pathways such as TNF-a signaling via factor nuclear kappa B (NF-κB) (betweenness centrality 0.46 and 0.15 respectively). This indicates a coordinated regulation of these processes and suggests a proinflammatory network in classical monocytes in RA.
Therefore, we investigated whether these pathways were also related to disease manifestation. Pearson’s correlation analysis revealed a correlation between the intensity of Interferon gamma response (ssGSEA score) and SDAI score (R = 0.39, p = 0.01) (Figure 2A). Unexpectedly, levels of vitamin D were positively correlated with hypoxia, early estrogen response, coagulation, late estrogen response and apoptosis pathways (Figure 2A). Furthermore, genes associated with interferon gamma response were sufficient to stratify RA patients and controls in two different clusters (Figure 2B), confirming its importance in RA pathogenesis.
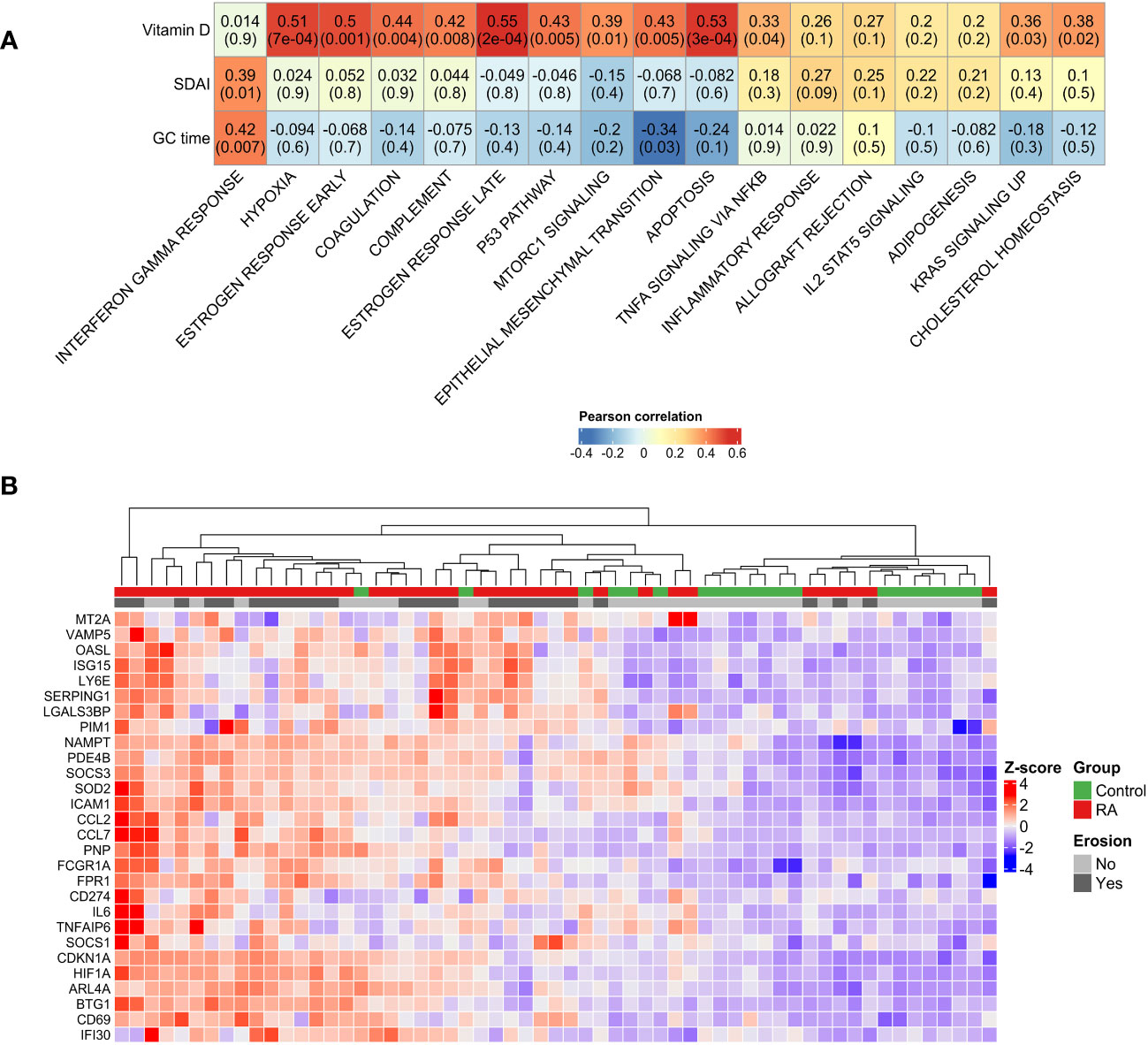
Figure 2 Disease manifestation and activated pathways. (A) Correlation plot showing the Pearson’s correlation between the GSEA pathways activated in RA and clinical parameters. High positive correlations have been observed between disease activity and interferon gamma pathway. Unexpectedly, positive correlations have been observed between vitamin D serum levels and TNF signaling, apoptosis and hypoxia. (B) Unsupervised clustering of interferon gamma response genes showed the stratification of RA patients and control group in two different groups. GC duration, Glucocorticoid duration; SDAI, Simplified Disease Activity Index; RA, Rheumatoid Arthritis.
3.4 Differential genes signature observed in bone erosion
In addition, we investigated the difference between the transcriptomic features of classical monocytes of RA patients considering their bone erosion status. A pairwise comparison (erosion vs. non- erosion) revealed 1,140 DEGs (Figure 3A) in RA patients with erosions, of which 89 were up-regulated and 1,051 down-regulated. To show the pattern of expression of the top ranked genes, a respective heatmap with the top-50 DEGs is presented in Figure 3B. Twenty-three genes that were up-regulated in RA regardless of erosion status (RA vs. controls) were also differentially expressed in patients with erosions in comparison with non-erosive patients (Figure 3C).
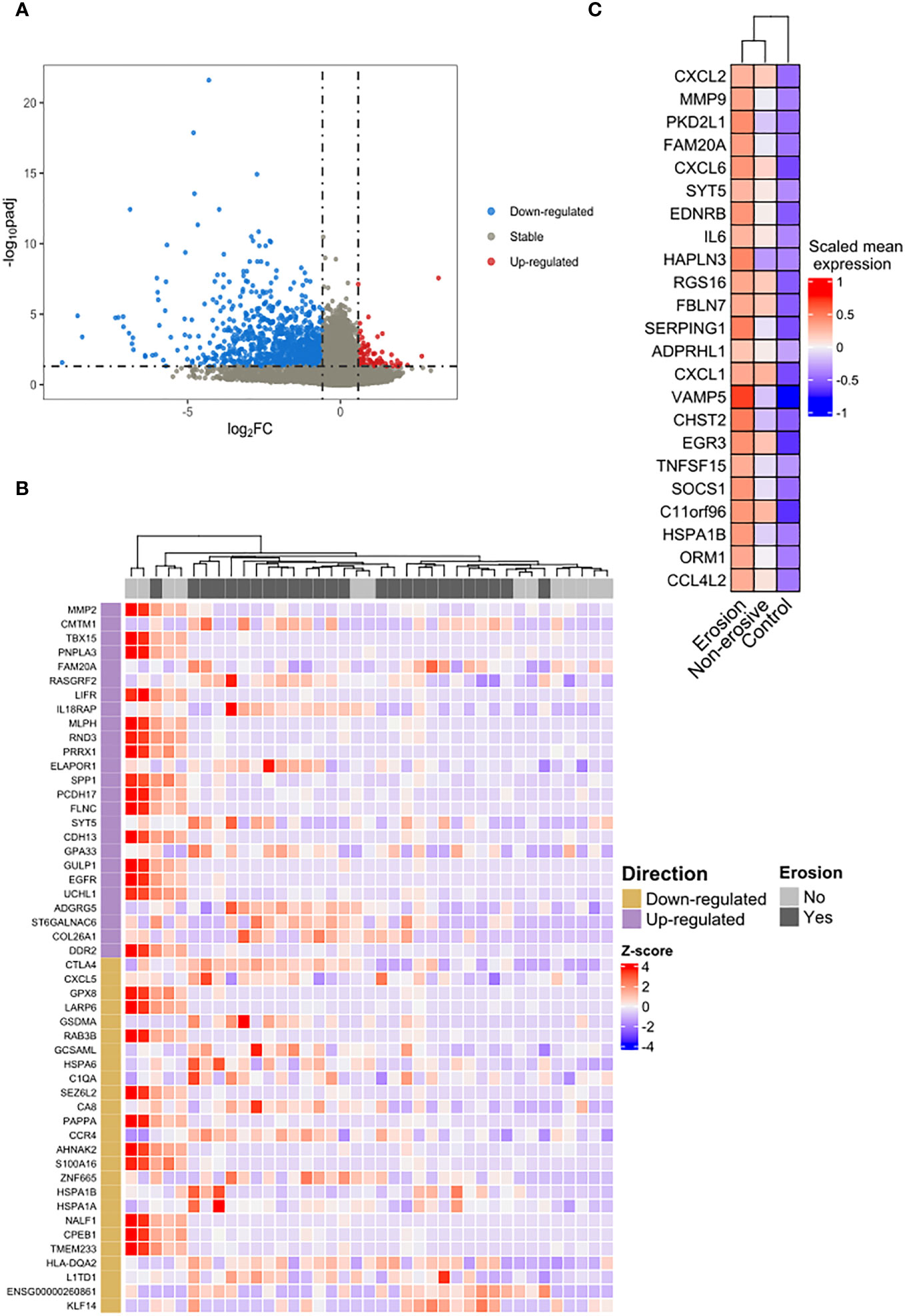
Figure 3 Differential expression and overrepresentation of immuno-inflammatory genes in bone erosion. (A) The comparison between RA patients with erosion and those without erosion identified 89 genes up-regulated (red dots) and 1,051 down-regulated (blue dots). (B) Heatmap of the top-50 highlighted a slight heterogeneity in the group of patients without erosion. (C) An overrepresentation of genes involved in cytokines response was identified in the list of shared up-regulated genes between the list of DEGs observed in erosion (erosion vs. without erosion) and those observed in RA regardless of the erosion status (RA vs. control).
3.5 Bone erosion is driven by Immuno-inflammatory pathways
Figure 3C illustrates an enrichment of genes associated with immuno-inflammatory response such as IL6, CXCL1, CXCL2, CXCL6 and the metalloproteinase MMP9 in erosive disease. To verify whether this was a general characteristic of classical monocytes in erosive disease, we analyzed the biological processes that were enriched in the DEGs in erosive disease. A manual curation of the top ranked genes (Table 3) revealed immune response genes such as HLA-DQA2, KLF14, IL18RAP, CMTM1 being upregulated in erosive disease. In the list of down-regulated genes, those associated with general biological and metabolic processes (Table 3) were identified. Interestingly, the down-regulated genes PAPPA and AHNAK2 were associated with bone formation and wound healing (Table 3).
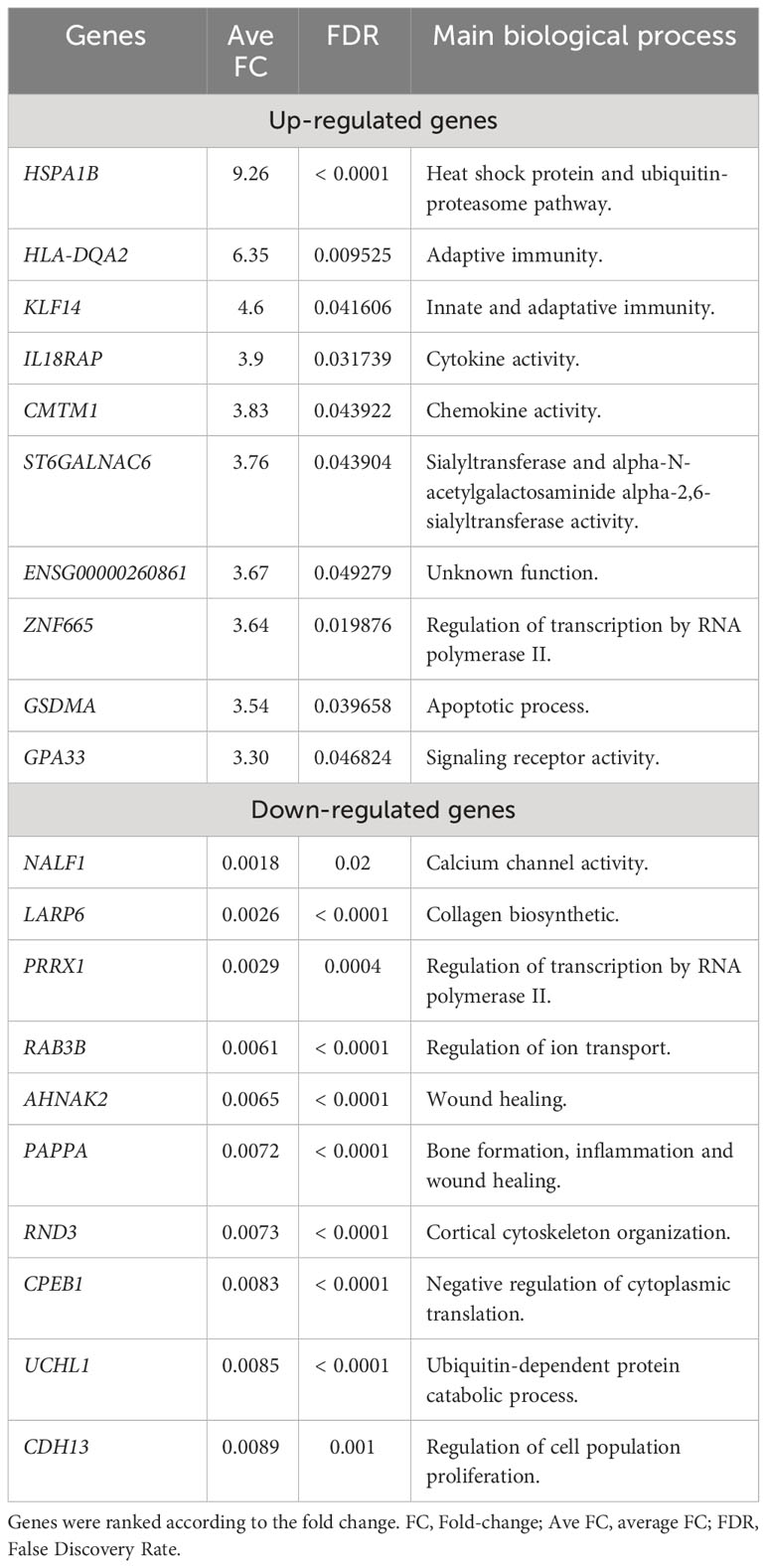
Table 3 Top differentially expressed genes identified in erosive rheumatoid arthritis patients (n=26).
3.6 Increased activation of immuno-inflammation is correlated with disease activity and number of erosions
The increase of immuno-inflammatory activation described above might potentially be a driver of bone erosion in RA. To test this hypothesis, we performed a gene set analysis using the DEGs identified in bone erosion and proceeded with a correlation analysis to identify any relationship between the biological pathways’ activation and disease activity. This gene sets enrichment analysis confirmed an increased activation of cytokine response genes, including response to interferon gamma and TNF, and the activation of immune response by leukocytes (Figure 4A). The network analysis identified IL6, CD40, XCL1, SOCS1, CORO1A, HSPA1B, CTLA4, HLA-DQA2, HSPA1A, SERPING1 as the most connected genes with the highest degree of connectivity (ranging from 4 to 8). To further prioritize potentially relevant targets, we filtered the list of up-regulated genes identified in erosion with a list of relevant genes associated with RA downloaded from the Open target platform. This strategy identified SOCS1, CORO1A, IL18RAP, CMKLR1 and HSPA1A as genes associated with erosive RA (Figure 4B). These genes include most of the connected genes identified in the network analysis.
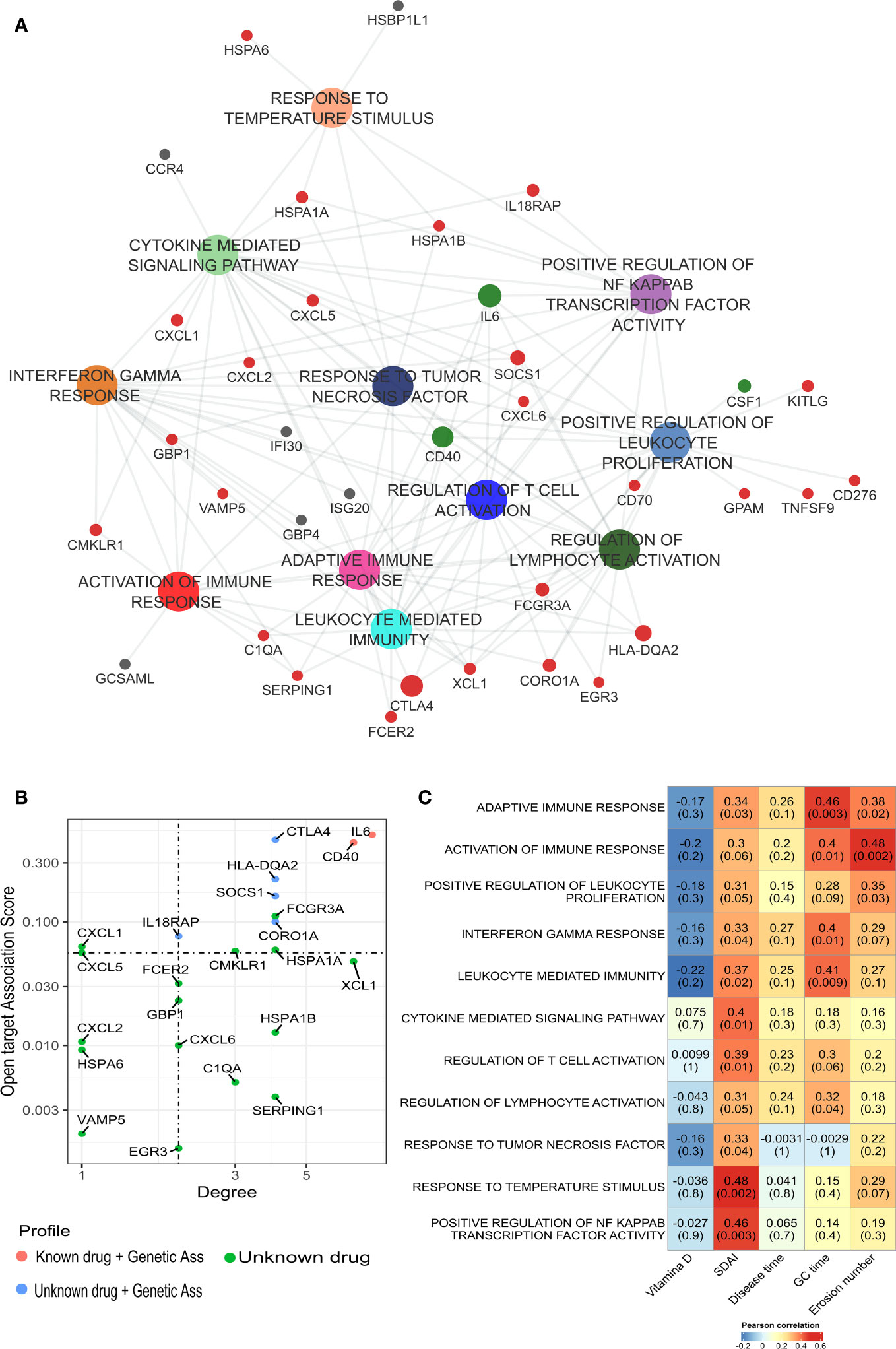
Figure 4 Gene set enrichment analysis of DEGs identified in classical monocytes from bone erosion. (A) ssGSEA analysis was performed to identified pathways that were enriched in erosion using the list of up-regulated genes. Enriched pathways are involved in immune-inflammatory processes with an overrepresentation of cytokine-mediated responses. (B) The network is enriched by genes associated with RA. Red dot: genes previously targeted by known drugs; blue: genes without drug but with genetic association. This information has been retrieved from Open Target database. (C) Pearson’s correlation analysis showed a positive correlation between bone erosion number and, cytokine and immune mediated pathway. Most of the pathways enriched in bone erosion are positively correlated with SDAI score. GC duration, Glucocorticoid duration; SDAI, Simplified Disease Activity Index; ssGSEA, Single Sample Gene Set Enrichment analysis.
To identify the more activated pathways in bone erosion, a comparison of the activation rates on enriched pathways (ssGSEA score) between patients’ groups was performed. The most differentially activated pathways in classical monocytes in RA patients with bone erosions were related to immune response (Supplementary Figures S4A–C). In addition, a Receiver Operating Characteristic (ROC) curve analysis was performed, in order to evaluate the performance of these pathways to discriminate erosive RA from non-erosive RA based on their activation level (ssGSEA score). This analysis showed that these pathways might be applicable to classify erosion status, achieving an accuracy of at least 0.8 (Supplementary Figures S5A–C).
Moreover, it was checked whether the differential activation of these pathways was related to disease activity and erosions numbers by performing a Pearson’s correlation analysis. Interestingly, bone erosions number was positively correlated with the activity of immune pathways (Figure 4C), including activation of immune response (R = 0.48, p = 0.002), adaptative immune response (R = 0.38, p = 0.02), and positive regulation of leukocyte proliferation (R = 0.35, p = 0.03). It is worth mentioning that these pathways were those identified as suitable for distinguishing erosive from non-erosive disease (Supplementary Figures S5A–C). Furthermore, most of the enriched pathways related to bone erosions were positively correlated with SDAI score (Figure 4C).
3.7 CD14+CD16- monocytes and down-regulation of osteoblast differentiation in bone erosion
We also investigated which biological pathways were down-regulated in erosive RA patients: Analyses found a predominance of pathways involved in osteoblast differentiation, and the regulation of canonical Wnt signaling pathway (Figure 5A). Hence correlation between the activation rate of osteoblast differentiation and the regulation of canonical Wnt signaling pathway (Figure 5B). Additionally, the most connected genes (GREM1, NOG, DDIT3, COL1A1, PRKD1, LGR4, FERMT2, WNT4, RSPO3, WWTR1, CAV1) were implicated in bone formation. When investigating the degree of down-regulation of these pathways and RA disease activity we found good correlation (Figure 5C). A negative correlation was observed between the number of erosions and the following pathways: Osteoblast differentiation (R = -0.35, p = 0.03), and Regulation of cell matrix adhesion (R = -0.45, p = 0.004).
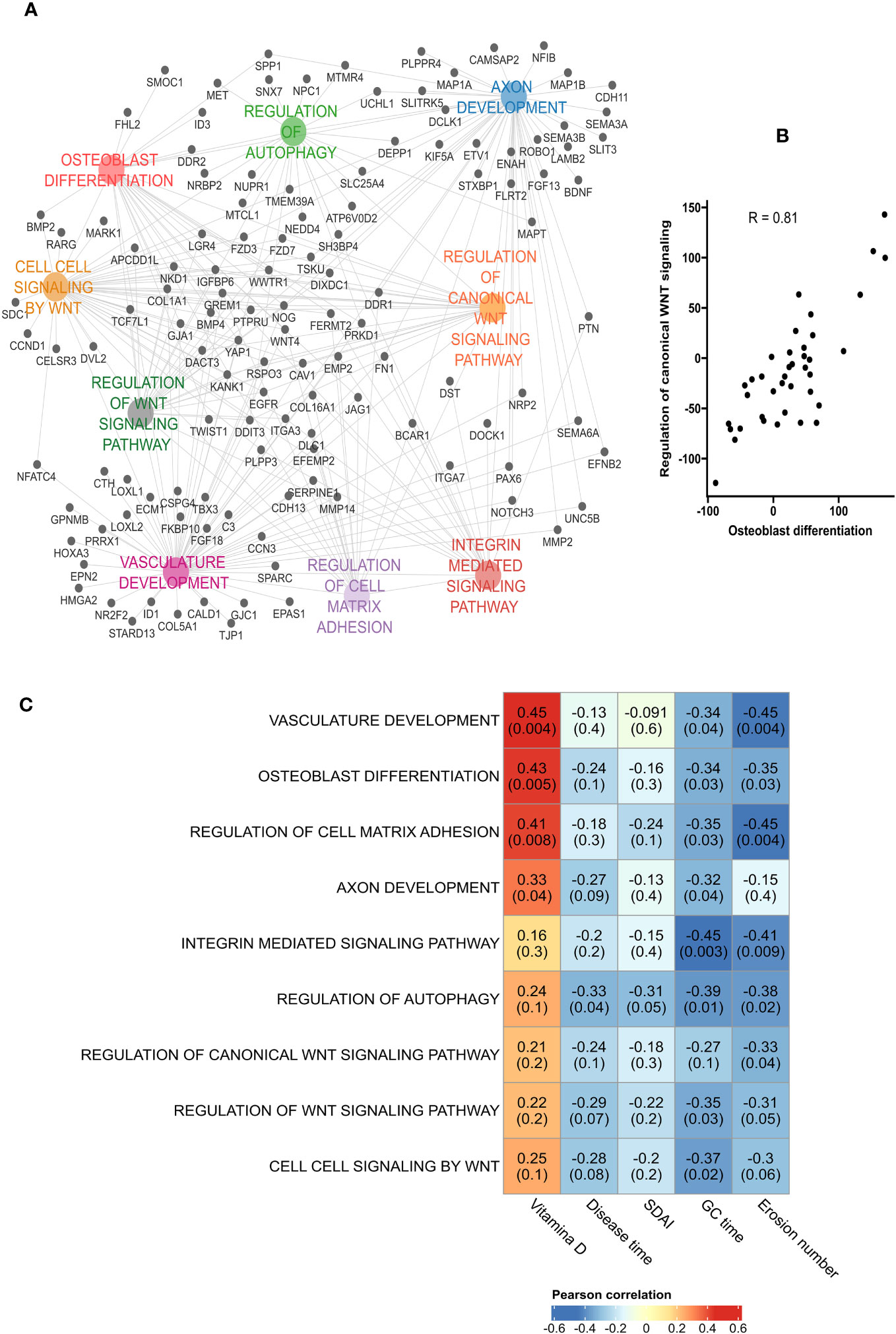
Figure 5 Down-regulation of osteoblast differentiation in bone erosion. (A) Pathways analysis of the down-regulated genes in erosion showed an enrichment of biological processes associated with osteoblast differentiation and bone formation. (B) High positive correlation was obtained between osteoblast differentiation and the regulation of canonical Wnt signaling. (C) Pearson’s correlation between the down-regulated pathways and patients’ characteristics showed a negative correlation between the number of erosion and pathways such as Vasculature development, Regulation of cell matrix adhesion, Regulation of autophagy and osteoblast differentiation. GC duration, Glucocorticoid duration; SDAI, Simplified Disease Activity Index.
4 Discussion
Herein we show the activation of classically activated monocytes in RA, which is even more pronounced in erosive than non-erosive disease. These data suggest that erosiveness in RA is linked to a pronounced activation of immune response genes in classical monocytes as well as a downregulation of genes that stimulate bone formation. Thus, alterations in the gene expression pattern in classically activated monocytes may orchestrate the altered bone homeostasis in RA.
Monocytes, i.e. classical monocytes have been associated with RA pathogenesis and disease progression (17). However, the association of specific monocytes subsets to bone erosion remained unclear. To the best of our knowledge, the implication of classical monocytes in bone erosions has not been studied at transcriptomic level in RA patients. When profiling the transcriptomic features of classical monocytes in RA patients, expression of immuno-inflammatory genes such as IL6, which are also known to stimulate osteoclast formation was higher in erosive than in non-erosive disease. Furthermore, a cluster of genes including WNT4, which are involved in bone formation, were down-regulated in monocytes from erosive RA patients.
Through the transcriptomic analysis this study also identified genes that were upregulated in classical monocytes of RA patients as compared to controls indicative for an upregulation of inflammatory and immune response genes in RA (18). In this way, among the top-ranked genes, we highlighted the upregulation of GDF15, CSF3, CCL7 and MMP2.
The GDF15 gene encodes a secreted ligand of the TGF-beta (transforming growth factor-beta) superfamily of proteins (19). It is up-regulated in many pathological conditions, including inflammation (20). GDF-15 plays distinct roles in regulating biological processes such as bone formation and hematopoietic development (21). Moreover, it has garnered attention as a potential biomarker for several diseases and is considered an indicator of overall mortality risk (22).
CSF3 is a Protein Coding gene that encodes the granulocyte colony-stimulating factor 3. The encoded cytokine controls the production, differentiation, and function of granulocytes (19). In periodontal inflammation, suppressing CSF3 has been shown to potentially reduce the mRNA expression of CXCL1, CXCL2 and CXCL3, IL-1β, IL-6 and matrix metalloproteinases 9 (23). Additionally, evidence suggests that a decrease in the receptor activator of nuclear factor κB ligand/osteoprotegerin (RANKL/OPG) ratio and the number of osteoclasts can contribute to the mitigation of damage to periodontal tissues (23). CCL7 encodes the monocyte chemotactic protein 3 (MCP-3), a chemokine which attracts monocytes during inflammatory processes (24). Recently, it has been shown that CCL7 levels are higher in serum and synovial fluid of RA patients compared to patients with osteoarthritis (25). Moreover, CCL7 levels have been positively correlated with anti-CCP antibodies in synovial fluid (25), and have also been involved in promoting local bone resorption through osteoclast migrating and differentiation (26).
In the same way, MMP2, a member of the matrix metalloproteinase family, acts on the activation and cleavage of several chemokines, including CCL7, therefore contributing to the creation of a chemotactic gradient and subsequent immune cell infiltration (27). It has been demonstrated that MMP-2 and MMP-9 are expressed in synovial tissue by synoviocytes, vascular endothelial and monocytes derived macrophages, suggesting their contribution to angiogenesis and pannus formation in RA (28). These observations might indicate the capability of classical monocytes to modulate immune cell influx into synovial tissue and the remodeling of synovial tissue during inflammation.
When comparing gene expression of classical monocytes in erosive vs. non-erosive RA immune response genes and those related to cytokine activation were among the most differentially expressed ones. This finding is not surprising since several proinflammatory cytokines have osteoclastogenic properties and thereby contribute to bone erosion (29). In part, this cytokine upregulation can be explained by the observed up-regulation of SOCS1 that is a negative-feedback inhibitor of JAK/STAT pathway (30). Of note, expression of cytokine-mediated pathways was also correlated with the extent of erosions.
Apart from cytokine pathways, some unexpected differences were observed among the expression patterns of monocytes from erosive and non-erosive RA patients. For instance, we found KLF14, a member of the Krüppel-like factors (KLF) family, as differentially expressed gene, which is involved in regulating T-regulatory cell differentiation (31). Furthermore, a recent study demonstrated that KLF14 is down-regulated during osteogenic differentiation of bone marrow mesenchymal stem cells, and its overexpression suppressed cell viability and osteogenic differentiation (32). Interesting, expression of KLF14 is negatively correlated with the WNT3A expression, a key factor for bone formation (32). The Wnt signaling is known to be implicated in the pathogenesis of many autoimmune diseases and vascular disorders and is one of the key biological processes associated with RA pathogenesis (33). Furthermore, Wnt pathway directly affects osteoblast and osteoclast, inducing an overall increase on bone formation and a decrease on bone resorption pathways (34).
Among the genes down-regulated in erosive RA are those associated with bone formation (AHNAK2 and PAPPA). The family of protein encoded by AHNAK2 plays a role in calcium signaling by interacting with calcium channel proteins, and an important role in bone metabolism (35). In fact, femur and tibia from AHNAK knock-out mice are shortened, and bone strength is significantly decreased (36). PAPPA gene, encodes a secreted metalloproteinase that cleaves insulin-like growth factor (IGF) binding proteins, and increases activation and availability of IGF (19). Inhibition of PAPPA in mice leads to reduced cortical area and trabecular thickness in long and vertebral bones (37). Furthermore, animals treated with anti-PAPP-A show impairment on bone formation rate and a tendency to reduce the osteoblast number, mineralizing surface, and osteoblast surface (37). Accordingly, pathways involved in osteoblast differentiation, and the regulation of canonical Wnt signaling pathway are down-regulated in erosive RA patients indicating that the myeloid compartment exerts negative effects on bone formation and repair.
In conclusion, the present study highlights the contribution of excessive activation of immuno-inflammatory pathways in classical monocytes as a contributing factor to bone erosion in patients with RA. Moreover, a downregulation of genes of bone formation processes was observed that suggest that classical monocytes orchestrate a milieu of suppression of bone formation and repair in erosive RA.
Data availability statement
The original contributions presented in the study are publicly available. This data can be found here: https://www.ebi.ac.uk/biostudies/arrayexpress/studies/E-MTAB-13361.
Ethics statement
The studies involving humans were approved by local Ethics Committee on Human Research from Sao Paulo University-CAPPesq (#51178115.1.0000.0068). The studies were conducted in accordance with the local legislation and institutional requirements. The participants provided their written informed consent to participate in this study.
Author contributions
LS, VC, CF, and RP designed the research. LS, BH, MP, VC, DD, EN, CF, and RP performed the research. BH performed bioinformatics analysis. LS, BH, GS, CF, and RP wrote the manuscript, and MP, VC, DD, GS, and EN revised the manuscript. All authors contributed to the article and approved the submitted version.
Funding
This study was supported by FAPESP (#2016/00006-7 RP and EB; #2020/14223-5 BH; #2018/01315-9 CF; 2018/05596-2 MP) and CNPQ (#303378/2022-0 to EFB and #305556/2017-7 RP).
Acknowledgments
We thank the Multi-user equipment in large-scale sequencing lab (SELA) supported by FAPESP (#2014/50137-5).
Conflict of interest
The authors declare that the research was conducted in the absence of any commercial or financial relationships that could be construed as a potential conflict of interest.
Publisher’s note
All claims expressed in this article are solely those of the authors and do not necessarily represent those of their affiliated organizations, or those of the publisher, the editors and the reviewers. Any product that may be evaluated in this article, or claim that may be made by its manufacturer, is not guaranteed or endorsed by the publisher.
Supplementary material
The Supplementary Material for this article can be found online at: https://www.frontiersin.org/articles/10.3389/fimmu.2023.1251034/full#supplementary-material
Glossary
References
1. Perez MO, Figueiredo CP, Sales LP, Medeiros-Ribeiro AC, Takayama L, Domiciano DS, et al. Association of bone erosions and osteophytes with systemic bone involvement on high-resolution peripheral quantitative computed tomography in premenopausal women with longstanding rheumatoid arthritis. Arthritis Rheumatol (2022) 74:407–17. doi: 10.1002/art.41961
2. Schett G, Gravallese E. Bone erosion in rheumatoid arthritis: mechanisms, diagnosis and treatment. Nat Rev Rheumatol (2012) 8:656–64. doi: 10.1038/nrrheum.2012.153
3. Rana AK, Li Y, Dang Q, Yang F. Monocytes in rheumatoid arthritis: Circulating precursors of macrophages and osteoclasts and, their heterogeneity and plasticity role in RA pathogenesis. Int Immunopharmacol (2018) 65:348–59. doi: 10.1016/j.intimp.2018.10.016
4. Auffray C, Sieweke MH, Geissmann F. Blood monocytes: development, heterogeneity, and relationship with dendritic cells. Annu Rev Immunol (2009) 27:669–92. doi: 10.1146/annurev.immunol.021908.132557
5. Boring L, Gosling J, Chensue SW, Kunkel SL, Farese RV, Broxmeyer HE, et al. Impaired monocyte migration and reduced type 1 (Th1) cytokine responses in C-C chemokine receptor 2 knockout mice. J Clin Invest (1997) 100:2552–61. doi: 10.1172/JCI119798
6. Charles JF, Hsu L-Y, Niemi EC, Weiss A, Aliprantis AO, Nakamura MC. Inflammatory arthritis increases mouse osteoclast precursors with myeloid suppressor function. J Clin Invest (2012) 122:4592–605. doi: 10.1172/JCI60920
7. Wong KL, Tai JJ-Y, Wong W-C, Han H, Sem X, Yeap W-H, et al. Gene expression profiling reveals the defining features of the classical, intermediate, and nonclassical human monocyte subsets. Blood J Am Soc Hematol (2011) 118:e16–31. doi: 10.1182/blood-2010-12-326355
8. Aletaha D, Smolen JS. The Simplified Disease Activity Index (SDAI) and Clinical Disease Activity Index (CDAI) to monitor patients in standard clinical care. Best Pract Res Clin Rheumatol (2007) 21:663–75. doi: 10.1016/j.berh.2007.02.004
9. Smolen JS, Breedveld FC, Schiff MH, Kalden JR, Emery P, Eberl G, et al. A simplified disease activity index for rheumatoid arthritis for use in clinical practice. Rheumatology (2003) 42:244–57. doi: 10.1093/rheumatology/keg072
10. Figueiredo CP, Perez MO, Sales LP, Caparbo V de F, Pereira RMR. Evaluation of bone erosion in rheumatoid arthritis patients by high-resolution peripheral quantitative computed tomography scans: comparison between two semi-automated programs in a three-dimensional setting. Int J Rheum Dis (2021) 24:948–53. doi: 10.1111/1756-185X.14157
11. Barnabe C, Toepfer D, Marotte H, Hauge E-M, Scharmga A, Kocijan R, et al. Definition for rheumatoid arthritis erosions imaged with high resolution peripheral quantitative computed tomography and interreader reliability for detection and measurement. J Rheumatol (2016) 43:1935–40. doi: 10.3899/jrheum.160648
12. Dobin A, Davis CA, Schlesinger F, Drenkow J, Zaleski C, Jha S, et al. STAR: ultrafast universal RNA-seq aligner. Bioinformatics (2013) 29:15–21. doi: 10.1093/bioinformatics/bts635
13. Love MI, Huber W, Anders S. Moderated estimation of fold change and dispersion for RNA-seq data with DESeq2. Genome Biol (2014) 15:1–21. doi: 10.1186/s13059-014-0550-8
14. Chapman SJ, Khor CC, Vannberg FO, Maskell NA, Davies CW, Hedley EL, et al. GenePattern 2.0. Nat Genet (2006) 38:500–1. doi: 10.1038/ng0506-500
15. Shannon P, Markiel A, Ozier O, Baliga NS, Wang JT, Ramage D, et al. Cytoscape: a software environment for integrated models of biomolecular interaction networks. Genome Res (2003) 13:2498–504. doi: 10.1101/gr.1239303
16. Gu Z, Eils R, Schlesner M. Complex heatmaps reveal patterns and correlations in multidimensional genomic data. Bioinformatics (2016) 32:2847–9. doi: 10.1093/bioinformatics/btw313
17. Culemann S, Grüneboom A, Krönke G. Origin and function of synovial macrophage subsets during inflammatory joint disease. Adv Immunol (2019) 143:75–98. doi: 10.1016/bs.ai.2019.08.006
18. Smolen JS, Aletaha D, Barton A, Burmester GR, Emery P, Firestein GS, et al. Rheumatoid arthritis. Nat Rev Dis Primers (2018) 4:1–23. doi: 10.1038/nrdp.2018.1
19. GeneCards®: the human gene database (2023). Available at: https://www.genecards.org/.
20. Baek SJ, Eling T. Growth differentiation factor 15 (GDF15): A survival protein with therapeutic potential in metabolic diseases. Pharmacol Ther (2019) 198:46–58. doi: 10.1016/j.pharmthera.2019.02.008
21. Modi A, Dwivedi S, Roy D, Khokhar M, Purohit P, Vishnoi J, et al. Growth differentiation factor 15 and its role in carcinogenesis: an update. Growth Factors (2019) 37:190–207. doi: 10.1080/08977194.2019.1685988
22. Wiklund FE, Bennet AM, Magnusson PKE, Eriksson UK, Lindmark F, Wu L, et al. Macrophage inhibitory cytokine-1 (MIC-1/GDF15): a new marker of all-cause mortality. Aging Cell (2010) 9:1057–64. doi: 10.1111/j.1474-9726.2010.00629.x
23. Zhang Z, Yuan W, Deng J, Wang D, Zhang T, Peng L, et al. Granulocyte colony stimulating factor (G-CSF) regulates neutrophils infiltration and periodontal tissue destruction in an experimental periodontitis. Mol Immunol (2020) 117:110–21. doi: 10.1016/j.molimm.2019.11.003
24. Chang T-T, Chen C, Chen J-W. CCL7 as a novel inflammatory mediator in cardiovascular disease, diabetes mellitus, and kidney disease. Cardiovasc Diabetol (2022) 21:185. doi: 10.1186/s12933-022-01626-1
25. Rump L, Mattey DL, Kehoe O, Middleton J. An initial investigation into endothelial CC chemokine expression in the human rheumatoid synovium. Cytokine (2017) 97:133–40. doi: 10.1016/j.cyto.2017.05.023
26. Yuan S, Hu H, Wang X, Yang J, Zhou R, Bai X, et al. Bindarit reduces bone loss in ovariectomized mice by inhibiting CCL2 and CCL7 expression via the NF-κB signaling pathway. Orthop Surg (2022) 14:1203–16. doi: 10.1111/os.13252
27. de Jager SCA, Hoefer IE. Beyond the matrix: MMP2 as critical regulator of inflammation-mediated vascular dysfunction. Cardiovasc Res (2017) 113:1705–7. doi: 10.1093/cvr/cvx202
28. Zhou M, Qin S, Chu Y, Wang F, Chen L, Lu Y. Immunolocalization of MMP-2 and MMP-9 in human rheumatoid synovium. Int J Clin Exp Pathol (2014) 7:3048–56.
29. Jung SM, Kim KW, Yang C-W, Park S-H, Ju JH. Cytokine-mediated bone destruction in rheumatoid arthritis. J Immunol Res (2014) 2014:1–15. doi: 10.1155/2014/263625
30. Liau NPD, Laktyushin A, Lucet IS, Murphy JM, Yao S, Whitlock E, et al. The molecular basis of JAK/STAT inhibition by SOCS1. Nat Commun (2018) 9:1558. doi: 10.1038/s41467-018-04013-1
31. Wei X, Yang R, Wang C, Jian X, Li L, Liu H, et al. A novel role for the Krüppel-like factor 14 on macrophage inflammatory response and atherosclerosis development. Cardiovasc Pathol (2017) 27:1–8. doi: 10.1016/j.carpath.2016.11.003
32. Weng J, Wu J, Chen W, Fan H, Liu H. KLF14 inhibits osteogenic differentiation of human bone marrow mesenchymal stem cells by downregulating WNT3A. Am J Transl Res (2020) 12:4445–55.
33. Cici D, Corrado A, Rotondo C, Cantatore FP. Wnt signaling and biological therapy in rheumatoid arthritis and spondyloarthritis. Int J Mol Sci (2019) 20:5552. doi: 10.3390/ijms20225552
34. Baron R, Kneissel M. WNT signaling in bone homeostasis and disease: from human mutations to treatments. Nat Med (2013) 19:179–92. doi: 10.1038/nm.3074
35. Zardab M, Stasinos K, Grose RP, Kocher HM. The obscure potential of AHNAK2. Cancers (Basel) (2022) 14:528. doi: 10.3390/cancers14030528
36. Kim IY, Yi SS, Shin JH, Kim YN, Ko C-Y, Kim HS, et al. Intensive morphometric analysis of enormous alterations in skeletal bone system with micro-CT for AHNAK–/– mice. Anat Sci Int (2020) 95:323–33. doi: 10.1007/s12565-020-00525-3
Keywords: rheumatoid arthritis, classical monocytes, bone erosions, inflammation, bone formation
Citation: Sales LP, Hounkpe BW, Perez MO, Caparbo VF, Domiciano DS, Borba EF, Schett G, Figueiredo CP and Pereira RMR (2023) Transcriptomic characterization of classical monocytes highlights the involvement of immuno-inflammation in bone erosion in Rheumatoid Arthritis. Front. Immunol. 14:1251034. doi: 10.3389/fimmu.2023.1251034
Received: 30 June 2023; Accepted: 21 September 2023;
Published: 05 October 2023.
Edited by:
Steven O’Reilly, STipe Therapeutics, DenmarkReviewed by:
Amy J. Naylor, University of Birmingham, United KingdomMercedes Lopez-Santalla, Centro de Investigaciones Energeticas, Medioambientales y Tecnologicas (CIEMAT), Spain
Copyright © 2023 Sales, Hounkpe, Perez, Caparbo, Domiciano, Borba, Schett, Figueiredo and Pereira. This is an open-access article distributed under the terms of the Creative Commons Attribution License (CC BY). The use, distribution or reproduction in other forums is permitted, provided the original author(s) and the copyright owner(s) are credited and that the original publication in this journal is cited, in accordance with accepted academic practice. No use, distribution or reproduction is permitted which does not comply with these terms.
*Correspondence: Camille Pinto Figueiredo, figueiredocamille@gmail.com
†These authors have contributed equally to this work
‡Deceased