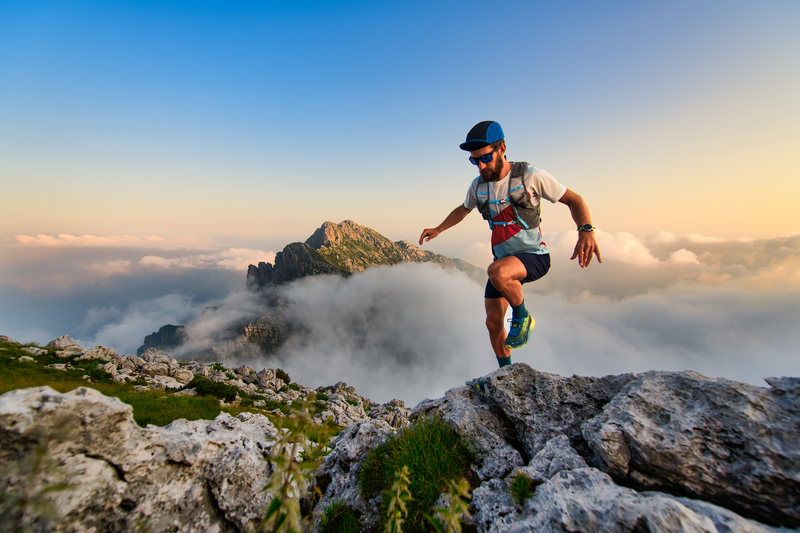
95% of researchers rate our articles as excellent or good
Learn more about the work of our research integrity team to safeguard the quality of each article we publish.
Find out more
PERSPECTIVE article
Front. Immunol. , 10 August 2023
Sec. Cancer Immunity and Immunotherapy
Volume 14 - 2023 | https://doi.org/10.3389/fimmu.2023.1237565
This article is part of the Research Topic New insights into Innate Immune cell-based immunotherapies in cancer View all 19 articles
Immunotherapies are a key therapeutic strategy to fight cancer. Diverse approaches are used to activate tumor-directed immunity and to overcome tumor immune escape. The dynamic interplay between tumor cells and their tumor(immune)microenvironment (T(I)ME) poses a major challenge to create appropriate model systems. However, those model systems are needed to gain novel insights into tumor (immune) biology and a prerequisite to accurately develop and test immunotherapeutic approaches which can be successfully translated into clinical application. Several model systems have been established and advanced into so-called patient avatars to mimic the patient´s tumor biology. All models have their advantages but also disadvantages underscoring the necessity to pay attention in defining the rationale and requirements for which the patient avatar will be used. Here, we briefly outline the current state of tumor model systems used for tumor (immune)biological analysis as well as evaluation of immunotherapeutic agents. Finally, we provide a recommendation for further development to make patient avatars a complementary tool for testing and predicting immunotherapeutic strategies for personalization of tumor therapies.
Immunotherapy has emerged as an important pillar in cancer therapy comprising multiple strategies, e.g. cell-based approaches as chimeric antigen receptor T cells (CAR T cells) (1–4) or tumor infiltrating lymphocytes (TIL) (5), immune checkpoint inhibitors (ICI) (6–13), oncolytic viruses (14) and tumor vaccines (15). However, despite promising preclinical data, only a very low percentage of oncological treatments reach phase III trials or even clinical application (16, 17) and even those strategies that have entered clinical routine often exert less pronounced anti-tumor effects than observed in model systems. In addition, clinicians are faced with great heterogeneity in terms of patient responses to therapy even if levels of predictive biomarkers (e.g. specific mutations or immunohistochemical staining of protein biomarkers) are comparable. This highlights the limitation of personalizing treatment strategies solely based on genomics and single biomarkers as well as the need for valid co-clinical testing systems. Functional drug testing in those co-clinical models representing the individual tumor biology of a patient as accurately as possible (also termed “patient avatars”) to predict the individual susceptibility to drugs appears as a desirable approach to truly personalize patient treatment (18). Increasing efforts are therefore made to improve preclinical tumor models in order to optimally represent the complex and dynamic interplay between tumor cells and the immune system, especially in the tumor microenvironment (TME) of solid and hematologic malignancies. Irrespective of whether the model system is used for tumor immunological studies or individualized therapy prediction, an optimal patient avatar needs to reflect intra- and intertumoral heterogeneity (19) and comprise the entire tumor (immune) microenvironment (T(I)ME) (20–22). Particularly, to test immunotherapeutic strategies, the whole spectrum of innate and adaptive immune cells should be present in the patient avatar to mimic the direct and indirect cellular interactions of tumor cells and all stromal (cell) components of the tumor.
Two-dimensional (2D) tumor cell models comprise established and often immortalized cell lines or primary cell cultures directly established from fresh tumor material. Established cancer cell lines derived from solid tumors, leukemias and lymphomas have been extensively used for basic cell biology experiments and drug discovery since the early 1950s (23). As these cells grow in monolayers, culture maintenance is comparatively simple, inexpensive and analyses (including imaging) are easy to perform due to limited complexity.
To improve the representation of the complex TME, monolayer cell cultures have been advanced into co-cultures enriched by coating with defined extracellular matrix (ECM) proteins or addition of distinct stem, stroma or (allogeneic) immune cell populations to allow the study of direct cell-cell interactions of different cell types or paracrine interactions in indirect cultures mostly using transwell inserts. The presence of immune cell populations (e.g. peripheral blood mononuclear cells (PBMC) or purified effector cells) is a prerequisite to study the preclinical effect of immunotherapies that aim to activate present immune cell populations. Alternatively, the cellular therapy itself (e.g. CAR T cells) constitutes the immune cell component within the co-culture model. Immunotherapeutic strategies including ICI (12), CAR T cells (1), CD3-targeted bispecific antibodies (24, 25) or oncolytic viruses (14) have been tested within 2D co-cultures. Of note, the cellular composition, activation and fitness status of circulating and tumor infiltrating immune cells often differs between healthy donors and cancer patients as they often display signs of reduced effector function and increased levels of exhaustion (26–30). Therefore, the integration of immune cells isolated directly from tumor tissue or PBMC of cancer patients into 2D cell cultures (as well as 3D co-culture models) is of great interest to approximate the functional capacity of the patient´s immune system. However, the use of allogeneic co-cultures to test respective immunotherapeutics is limited to a short experimental period up to a few days to avoid MHC-mediated alloreactions. Another critical point of this model system is that intratumor heterogeneity is not well reflected, as established tumor cell lines undergo clonal selection and genetic drift (31–33). Moreover, the complex tumor architecture with respect to spatial and cellular composition, ECM, gradients of oxygen, nutrients and other soluble factors including cytokines is obviously lacking (34, 35). Subsequently, these models have shown to have limited predictive value (36–38) as they do not optimally represent the complex tumor biology.
To improve the representation of patient’s tumors, primary tumor cells may be used instead. For example, Kodack et al. established mono cell cultures with primary cells isolated from tumor tissues of different tumor entities and advanced them into co-cultures with fibroblasts for drug testing of tyrosine kinase inhibitors (39). However, their success rate was limited to 26% with differing rates between tumor entities (39). Kornauth et al. demonstrated the potential of leukemia and lymphoma cell suspensions as a predictive tool for individualized treatment in aggressive hematologic malignancies (40). Within a clinical trial, single cell suspensions of tumor material (biopsies, blood or bone marrow aspirates) were generated and directly subjected to treatment with 139 drugs circumventing the time-consuming and failure-prone establishment of cancer cell lines. In this approach, the drug response of tumor cells within the cell bulk was determined by immunofluorescent microscopy and quantification of the surviving proportion of tumor cells in comparison to controls (40). Of note, 56 heavily pretreated patients were treated based on the results of this testing resulting in a clinical benefit in 54% (30 patients) including a relevant number of exceptional responses. Although these results are promising in terms of a co-clinical model, evaluation of immunotherapeutic strategies was not included in this trial and requires further advancement of this model by adding effector cell types or cellular therapies.
A further improvement of the above mentioned 2D cultures are spheroids which are three dimensional aggregates of one or multiple cell types. Spheroids can be comprised of tumor cells (primary cells or cell lines) only or of mixtures of tumor and stroma/immune cells (41). Furthermore, ECM can be supplemented. The 3D structure results in formation of a hypoxic zone in the spheroid core as it is commonly observed in tumors where the tumor center is often hypoxic (42). Different culture techniques are used to generate spheroids, e.g. using low-adherent surface plates or the hanging drop method, but all of them are based on preventing attachment of tumor cells to the culture plate and promoting 3D cell-cell aggregation (43, 44). The fast and easy way to generate spheroids from established tumor cell lines along with established readout assays (45) allows high throughput drug screens which can be particularly beneficial for testing novel therapeutic approaches. Recently, 3D spheroids have been used to evaluate different immunotherapeutics, e.g. CAR NK cells against triple negative breast cancer (46), ICI targeting of PD-L1 in pancreatic ductal adenocarcinoma (PDAC) (41) or a strategy to activate tumor associated macrophages via CSF1R inhibition and CD40 activation in Her2-positive breast cancer (47).
However, besides most of the limitations mentioned for 2D cultures, the uncontrollable arrangement of the cells in the spheroids and the reduced complexity of the spheroids with regard to an incomplete cellular and acellular composition (42) limit the usage of 3D spheroids as co-clinical model particularly for testing immunotherapeutic strategies.
Organoid technology has rapidly developed as a transformative 3D model since Clevers et al. established an intestinal 3D culture system from intestinal stem cells in 2009 (48). Organoid technology is now vastly used for modeling of physiological tissue but also of different cancers in patient-derived organoids (PDO). To generate organoids, small tissue fragments from surgical specimen or biopsies are dissociated into single cells and subsequently cultured, most often embedded in 3D matrices providing ECM support and in complex culture media enriched with multiple growth factors (49, 50). Today, PDO are available for multiple tumor entities, including prostate cancer (51), colorectal cancer (48, 52), or PDAC (53). Compared to 2D and spheroid cell cultures, PDO offer an improved insight into tumor biology as the heterogeneity of driver mutations and phenotypes of the primary tumor are better retained (53) and thus, tumor cell complexity, differentiation, and functionality are better represented (54, 55). Furthermore, PDO allow genetic engineering and genomic analyses that cannot be accurately modeled in animals (22, 56). However, major limitations of PDO remain the lack of vascularization and the complex TME (57) which sometimes constitutes the major compartment of a tumor, e.g. in cancers like PDAC (58, 59). Furthermore, time of establishment (currently weeks to months) is still time-consuming and the success rates are highly variable (16% to > 90%) differing between patients and tumor entities (60–64). Despite these limitations, PDO have been constantly advanced and increasingly used for preclinical testing of immunotherapies including ICI (7–9), bispecific antibodies (65), CAR T cells (66) or TIL generation (67).
For co-clinical evaluation, co-culture models of PDO with autologous immune cells and additional components of the TME appear to be ideal (68–70). To this end, Forsythe et al. established co-culture PDO models of appendiceal cancer with autologous immune cell populations to evaluate the efficacy of ICI nivolumab and pembrolizumab and identified 10-20% of PDO to be susceptible to ICI therapy (71). PDO may also offer a cost-effective opportunity to select for and expand TIL or generate patient specific cellular therapies. Dijkstra et al. successfully enriched autologous tumor reactive T cells from peripheral blood of colorectal and lung cancer patients (67). Similarly, Parikh et al. used organoids derived from metastases of multiple solid cancers to identify and generate TIL directed against individual tumor neoantigens with highly effective anti-tumor activity (72). These TIL co-cultured PDO could be established within two months for 75% of resected samples (72). To test CAR T cell treatment strategies in solid cancers, Schnalzger et al. used colorectal cancer PDO for evaluation of tumor cell killing and established a protocol to test the tumor cell specificity in competition assays using spiked-in organoids derived from healthy intestinal tissue (73). Beyond preclinical testing of immunotherapeutics, PDO can be employed to produce tumor cell specific T cells from induced pluripotent stem cells. This strategy may enable the production of allogeneic “off-the-shelf” CAR T cells circumventing the laborious and expensive generation of autologous CAR T cells (74). Moreover, large drug screens were successfully conducted implementing automated organoid seeding using automated microscopy or destructive viability assays as read-outs for drug efficacy paving the way for applications within the highly regulated clinical setting (75, 76). Recent studies indicate that PDO can be also used as co-clinical models for the prediction of treatment responses (60, 77, 78) and clinical trials are underway using functional testing in PDO to guide treatment decisions (79). Hence, several smaller collectives have been already established indicating a moderate to good correlation of drug responses in organoid-based patient avatar models with clinical responses (60, 77, 80). Guillen et al. combined mouse PDX and matched PDO of treatment resistant metastatic breast cancer to improve accuracy of modeling and combination of in vitro and in vivo drug testing (81).
However, to incorporate PDO-based treatment prediction into clinical workflows, PDO need to be improved in terms of reducing establishment time and optimizing generation success rates (61–64), highly varying among cancer patients and entities (60) not ensuring PDO generation from every patient. Finally, to accelerate meaningful implementation of PDO-based patient avatars into clinical application, prospective and systematic evaluation of their accurate representation of biological properties of the disease of origin and their predictive properties need to be considered in translational programs accompanying prospective clinical trials. Additionally, the implementation of the TME requires further developments, PDO generation needs to be methodologically standardized following standard operating procedures and predefined cut-offs for treatment response need to be defined to guide clinical decisions (82). Here, synthetic ECM substitutes have been already used to significantly reduce batch variability of ECM components ensuring a higher degree of standardization (83, 84). Hopes are high to use living PDO biobanks (52, 81, 85) for testing immunotherapies to build the translational bridge between basic research and patient care.
To evaluate novel immunotherapies and identify biomarkers, humanized patient-derived xenografts (hPDX) are an important platform (86–92). Meanwhile, more than 45 PDX models are available including NSG, NOD-scid, NRG, BRGS, SRG and next-generation humanized mice (86, 89). Besides therapeutic responses, possible side effects as well as tumor progression and metastasis can be studied in these whole organism models. In hPDX, almost all histological, genetic, molecular, and immunological characteristics are at least represented at low passages (93, 94), fulfilling several key requirements of patient avatars (95–98). Particularly, testing of immunotherapies demands the patient’s immune system which can be activated towards the patient’s tumor. For this purpose, hPDX models require humanization of mice and full engraftment with the patient’s immune system. However, it has not been possible to reconstitute mice with the complete operational immune system of cancer patients, yet (86, 90, 91, 99). For testing immunotherapies based on T cells, the engraftment with patient’s PBMCs is of great interest. However, this is only feasible for short-term experiments due to the rapid onset of graft-versus-host disease (GvHD). This issue has been diminished by eliminating MHC-I and -II expression (100) or using mice lacking murine CD47 (101). Of note, PBMC-engrafted mice can undergo a switch in immune cellular composition within 7 days. As a result, T cells might dominate and concomitantly myeloid as well as B and NK cells are underrepresented (89) thereby not fully representing the immune system of patients. Alternatively, engraftment can be achieved by CD34+ human hematopoietic stem cells to study immunotherapies in hPDX (91, 102, 103).
An important limiting factor of hPDX as patient avatar is the generation duration of months up to a year (91) depending on tumor entity, technology and mouse strain (89). In most cases, this time frame is not feasible to establish a patient avatar as co-clinical model as patient´s treatment must start within a short period of time (i.e. most often within a few weeks, in cases with high tumor burden even faster). Additionally, it is necessary to take into account potential effects of patient’s pre-treatment in terms of acquired resistance mechanisms (104) or cumulative toxicity, which remains difficult to model in hPDX (105). Finally, even if tumors are transplanted with their respective human stroma, the TME in hPDX will be remodeled, e.g. by conversion from human to murine ECM (94).
Weighting the above-mentioned improvements and remaining limitations, good correlations between tumor responses in hPDX models and clinical responses of the corresponding patients were observed suggesting that this model is principally suitable as co-clinical model for therapy prediction (106–108). Moreover, hPDX have been used as major models to study CAR T cell therapies (2–4), ICI (5, 6, 11) and TIL (5).
As a strategy to avoid animal experiments, organ-on-a-chip (OOC) models have been designed to mimic physiological functions of different organs or tissues (109, 110). OOC can be based on established cell lines or organoids co-cultured with immune cells, fibroblasts or endothelial cells (111, 112). Additionally, epithelial and endothelial linings as well as ECM proteins may be included. In contrast to a conventional direct co-culture, in OOC cells are assembled on a chip containing a chamber and channels allowing for medium influx and efflux. Adding microfluidics via constant pumping of media allows to maintain gradients (e.g. of growth factors) and micromechanics (e.g. shear stress) while ensuring culture conditions for multiple cell types simultaneously. Geyer et al. modeled the physical barrier formed by pancreatic stellate cells (PSC) that prevent PBMCs, especially T cells, to migrate towards PDAC cells in a PDAC OOC. This barrier was overcome by treatment with Halofuginon inducing PSC death thereby increasing PBMC migration (113). These findings again illustrate the importance to consider the TME in the model system to properly test immunotherapeutic strategies. An additional layer of functional complexity can be added by including cell types that mediate drug metabolism, i.e. hepatocytes allowing the study of prodrugs (114). Cui et al. used a patient specific OOC to analyze the efficacy of anti-PD1 immunotherapy in different glioblastoma subtypes (10), Nyen et al. examined the response to trastuzumab and the impact of the tumor stroma in a breast cancer OOC (115) and Paterson et al. evaluated a CAR T construct in another breast cancer OOC (116). These studies clearly indicate the potential of OOC as patient avatar for individual therapy response prediction. Although OOC is a promising model for this purpose as it allows the combination of different cell types in one system and microscopic analysis is enabled by transparent polymers (109, 110), the most critical point is again the time needed for model establishment. Tumor cell isolation, organoid formation, OOC generation and treatment are all time-consuming steps, limiting its potential application as a patient avatar particularly for fast progressing and advanced cancers.
Finally, patient derived organotypic slice cultures (OTSC) have emerged as a sophisticated patient avatar with a great potential to reduce the number of animal experiments (117, 118). OTSC are derived from tumor tissues obtained during surgical resection or via core needle biopsy (118–120). Afterward, tissues are cut mostly using a vibratom into tissue slices (117, 119, 120) The slice thickness varies from 150-500 µm and depending on tissue origin and cultivation method, OTSC remain intact for distinct time periods. Thus, it has been shown that OTSC remain viable for 5-9 days for PDAC (119–122), up to 10 days for non-small cell lung cancer (123), up to 6 days for breast cancer (124) and up to 16 days in glioblastoma (125). However, changes in the T(I)ME over time were not always characterized in detail (117, 119, 120, 122). Cultivation often takes place on inserts at the air-liquid-interface to ensure sufficient oxygenation and to prevent cell death due to hypoxia (120, 126). Here, the composition of the medium is a critical factor, as certain media can support growth of certain cell types and thus influence the original tissue composition by driving selection of certain cell clones and phenotypes (119, 127).
In contrast to organoids and other cell culture models, which often represent only a reconstruction of the original tumor cell compartment, OTSC preserve the tumor and stroma heterogeneity thereby representing the tumor in its native environment, comprising epithelial/tumor cells, entire ECM as well as stroma and immune cells (117, 121, 128). In this way, all cells retain their function (hormone secretion, vascular contractility, cytokine secretion) along with their proteome and secretome (e.g. for immunological functions), and neurons also remain viable due to the presence of nerve growth factor (117, 129). This high similarity to the original tumor tissue creates unique conditions for analyzing the interplay of tumor cells with their TME thereby providing improved insights into tumor biology. Embedding of glioblastoma spheroids in brain tissue slices, Decotret et al. showed that the brain TME has a decisive influence on glioblastoma cell invasion (130). Besides, OTSC also appear to be well suited for the development and testing of novel therapeutic approaches (117, 118, 131). Thus, a combination treatment targeting carcinoma associated fibroblasts (CAF) by CXCR4 blockade and immune cells by ICI, increased T cell migration and activation towards tumor cells was observed resulting in tumor cell apoptosis (118). In line with these results, ECM reduction in OTSC improved T cell invasion towards tumor cells and increases the efficacy of blockade of the immune checkpoint molecule PD-1 (13). As OTSC are the only model system preserving the entire patient’s tumor contexture over a distinct time period, it can be considered the best patient avatar to date. Importantly first studies indicate that OTSC are suitable for testing immunotherapies (123), although data on the correlation between treatment responses in patients and corresponding OTSC is still scarce. Therefore, the predictive power of OTSC has to be proven yet.
Besides these important advantages of OTSC, some critical points still deserve optimization. As mentioned above, the medium composition impacts survival and proliferation of cells thereby selecting certain cell populations (127). Furthermore, despite cultivation at the air-liquid-interface, longer cultivation might lead to hypoxia resulting in culture-induced cell death in certain areas of the section (120, 126). The limited culture duration in turn also impedes long-term studies including studies analyzing long-term effects of applied treatments. Furthermore, long-term storage of viable OTSC for future analyses is not possible yet, and the limited number of OTSC which can be obtained from one patient limits high throughput drug screening (120). Finally, to properly assess treatment responses, appropriate and reliable readout parameters have to be identified and quantified. Here, (live cell) imaging might be difficult due to the thickness of the OTSC (117).
However, since the response to (immuno)therapies often varies among cancer patients, OTSC have a high potential to play a role in the development of patient tailored therapy. The rapid availability of OTSC after surgery or core needle biopsy allows for rapid drug testing and, at the same time, characterization of the entire tumor including its T(I)ME even in patients with advanced tumor diseases. This offers the great opportunity to allow a prompt therapy prediction for the patient.
Significant progress has been made advancing existing in vitro, ex vivo and in vivo tumor models into patient avatars containing the patient´s T(I)ME thereby trying to mimic the patient´s tumor characteristics in the best possible manner. These efforts have led to invaluable insights into tumor (immune) biology and the efficacy of immunotherapeutic strategies. However, as outlined above and summarized in Table 1, every model system bears its advantages and disadvantages which need to be carefully weighed in order to make the right choice of the patient avatar for research or co-clinical therapy testing and prediction. Accordingly, further efforts are needed to focus on the following two aspects: First, addressing remaining limitations in the representation of the T(I)ME in existing models and second, advancing existing models towards co-clinical patient avatars that support clinical decision making based on functional assays. Results from these assays may then complement existing strategies to personalize tumor therapies based on genomics, transcriptomics and immunohistochemical tumor analysis.
Table 1 Overview of key features of currently used patient avatars and their suitability as co-clinical models for testing of immunotherapies.
Finally, to advance patient avatars towards clinical application, a critical and important point is the standardization, e.g. by using harmonized protocols for generation and maintenance, reducing batch variability in reagents, increasing throughput while reducing costs for generation and characterization and defining experimental endpoints that are clinically meaningful (82, 132). Organoid or OTSC-based models may then even serve to develop patient specific therapies such as TIL and CAR T cells.
The current dynamics of the field are reflected by a multitude of ongoing clinical trials set up to evaluate the power of organoid or PDX based-models to predict clinical outcomes in cancer patients (133). Results from these mostly observational clinical trials will provide novel insights into feasible strategies to advance and implement personalized functional assays based on patient avatars for evaluation of (immuno) therapeutics.
The original contributions presented in the study are included in the article/Supplementary Material. Further inquiries can be directed to the corresponding author.
Conceptualization, CK, AB, AW, SS. Supervision, SS. Visualization, CK, AB. Writing – original draft, AB, CK, AW, SS. Writing – review and editing, AB, CK, AW, SS. All authors contributed to the article and approved the submitted version.
This project and its publication were supported by Deutsche Forschungsgemeinschaft (DFG, German Research Foundation) – Projektnummer 413490537) and the Stiftung für Krebsentstehung und Immunologie. We acknowledge financial support by Land Schleswig-Holstein within the funding programme Open Access Publikationsfonds.
The authors declare that the research was conducted in the absence of any commercial or financial relationships that could be construed as a potential conflict of interest.
All claims expressed in this article are solely those of the authors and do not necessarily represent those of their affiliated organizations, or those of the publisher, the editors and the reviewers. Any product that may be evaluated in this article, or claim that may be made by its manufacturer, is not guaranteed or endorsed by the publisher.
The Supplementary Material for this article can be found online at: https://www.frontiersin.org/articles/10.3389/fimmu.2023.1237565/full#supplementary-material
BRGS, BALB/cRag2-/-Il2rg-/-SirpaNOD mice; CAF, Carcinoma associated fibroblasts; CAR-NK cells, Chimeric antigen receptor-natural killer cells; CAR-T cells, Chimeric antigen receptor-T cells; ECM, Extracellular matrix; GvHD, Graft-versus-host disease; hPDX, Humanized patient-derived xenografts; ICI, Immune checkpoint inhibitors; NOD-scid, Nonobese diabetic/severe combined immunodeficiency; NRG, NOD-Rag1nullIL2rgnull; NSG, NOD scid gamma mice; PBMC, Peripheral blood mononuclear cells; PDAC, Pancreatic ductal adenocarcinoma; PD-1, Programmed cell death protein-1; PD-L1, Programmed-death ligand 1; PDO, Patient derived organoids; PSC, Pancreatic stellate cells; OOC, Organ-on-a-chip; OTSC, Organotypic slice cultures; SRG, Sprague Dawley-Rag2em2heraIl2rgem1hera/HblCrl; TIL, Tumor infiltrating lymphocytes; T(I)ME, Tumor (immune) microenvironment; TME, Tumor microenvironment.
1. Bhatia V, Kamat NV, Pariva TE, Wu LT, Tsao A, Sasaki K, et al. Targeting advanced prostate cancer with STEAP1 chimeric antigen receptor T cell and tumor-localized IL-12 immunotherapy. Nat Commun (2023) 14. doi: 10.1038/s41467-023-37874-2
2. Barrett DM, Zhao Y, Liu X, Jiang S, Carpenito C, Kalos M, et al. Treatment of advanced leukemia in mice with mRNA engineered T cells. Hum Gene Ther (2011) 22:1575–86. doi: 10.1089/hum.2011.070
3. Myburgh R, Kiefer JD, Russkamp NF, Magnani CF, Nuñez N, Simonis A, et al. Anti-human CD117 CAR T-cells efficiently eliminate healthy and Malignant CD117-expressing hematopoietic cells. Leukemia (2020) 34:2688–703. doi: 10.1038/s41375-020-0818-9
4. Mhaidly R, Verhoeyen E. Humanized mice are precious tools for preclinical evaluation of car t and car nk cell therapies. Cancers (Basel) (2020) 12:1–22. doi: 10.3390/cancers12071915
5. Gitto SB, Kim H, Rafail S, Omran DK, Medvedev S, Kinose Y, et al. An autologous humanized patient-derived-xenograft platform to evaluate immunotherapy in ovarian cancer. Gynecol Oncol (2020) 156:222–32. doi: 10.1016/j.ygyno.2019.10.011
6. Wang M, Yao LC, Cheng M, Cai D, Martinek J, Pan CX, et al. Humanized mice in studying efficacy and mechanisms of PD-1-targeted cancer immunotherapy. FASEB J (2018) 32:1537–49. doi: 10.1096/fj.201700740R
7. Holokai L, Chakrabarti J, Lundy J, Croagh D, Adhikary P, Richards SS, et al. Murine-and human-derived autologous organoid/immune cell co-cultures as pre-clinical models of pancreatic ductal adenocarcinoma. Cancers (Basel) (2020) 12:1–25. doi: 10.3390/cancers12123816
8. Neal JT, Li X, Zhu J, Giangarra V, Grzeskowiak CL, Ju J, et al. Organoid modeling of the tumor immune microenvironment. Cell (2018) 175:1972–1988.e16. doi: 10.1016/j.cell.2018.11.021
9. Scognamiglio G, De Chiara A, Parafioriti A, Armiraglio E, Fazioli F, Gallo M, et al. Patient-derived organoids as a potential model to predict response to PD-1/PD-L1 checkpoint inhibitors. Br J Cancer (2019) 121:979–82. doi: 10.1038/s41416-019-0616-1
10. Cui X, Ma C, Vasudevaraja V, Serrano J, Tong J, Peng Y, et al. Dissecting the immunosuppressive tumor microenvironments in glioblastoma-on-a-chip for optimized Pd-1 immunotherapy. Elife (2020) 9:1–21. doi: 10.7554/eLife.52253
11. Capasso A, Lang J, Pitts TM, Jordan KR, Lieu CH, Davis SL, et al. Characterization of immune responses to anti-PD-1 mono and combination immunotherapy in hematopoietic humanized mice implanted with tumor xenografts. J Immunother Cancer (2019) 7. doi: 10.1186/s40425-019-0518-z
12. Wang C, Thudium KB, Han M, Wang XT, Huang H, Feingersh D, et al. In vitro characterization of the anti-PD-1 antibody nivolumab, BMS-936558, and in vivo toxicology in non-human primates. Cancer Immunol Res (2014) 2:846–56. doi: 10.1158/2326-6066.CIR-14-0040
13. Nicolas-Boluda A, Vaquero J, Vimeux L, Guilbert T, Barrin S, Kantari-Mimoun C, et al. Tumor stiffening reversion through collagen crosslinking inhibition improves t cell migration and anti-pd-1 treatment. Elife (2021) 10. doi: 10.7554/eLife.58688.sa2
14. Crupi MJF, Taha Z, Janssen TJA, Petryk J, Boulton S, Alluqmani N, et al. Oncolytic virus driven T-cell-based combination immunotherapy platform for colorectal cancer. Front Immunol (2022) 13. doi: 10.3389/fimmu.2022.1029269
15. Yin Q, Zhao N, Chang Y, Dong M, Xu M, Xu W, et al. Melanoma stem cell vaccine induces effective tumor immunity against melanoma. Hum Vaccin Immunother (2023) 19. doi: 10.1080/21645515.2022.2158670
16. Dimasi JA, Reichert JM, Feldman L, Malins A. Clinical approval success rates for investigational cancer drugs. Clin Pharmacol Ther (2013) 94:329–35. doi: 10.1038/clpt.2013.117
17. Wong CH, Siah KW, Lo AW. Estimation of clinical trial success rates and related parameters. Biostatistics (2019) 20:273–86. doi: 10.1093/biostatistics/kxx069
18. Letai A, Bhola P, Welm AL. Functional precision oncology: Testing tumors with drugs to identify vulnerabilities and novel combinations. Cancer Cell (2022) 40:26–35. doi: 10.1016/j.ccell.2021.12.004
19. Aldag L, Beckinger S, Daunke T, Philipp LM, Surrow A, Yesilyurt UU. The heterogeneity of the tumor microenvironment as essential determinant of development, progression and therapy response of pancreatic cancer. Cancers (Basel) (2021) 13. doi: 10.3390/cancers13194932
20. Genta S, Coburn B, Cescon DW, Spreafico A. Patient-derived cancer models: Valuable platforms for anticancer drug testing. Front Oncol (2022) 12. doi: 10.3389/fonc.2022.976065
21. Elinav E, Garrett WS, Trinchieri G, Wargo J. The cancer microbiome. Nat Rev Cancer (2019) 19:371–6. doi: 10.1038/s41568-019-0155-3
22. Li Q, Hu W, Liu W-X, Zhao L-Y, Huang D, Liu X-D, et al. Streptococcus thermophilus inhibits colorectal tumorigenesis through secreting β-galactosidase. Gastroenterology (2021) 160:1179–1193.e14. doi: 10.1053/j.gastro.2020.09.003
23. Scherer WF, Syverton JT, Gey GO. Studies on the propagation in vitro of poliomyelitis viruses. IV. Viral multiplication in a stable strain of human Malignant epithelial cells (strain HeLa) derived from an epidermoid carcinoma of the cervix. J Exp Med (1953) 97:695–710. doi: 10.1084/jem.97.5.695
24. Warwas KM, Meyer M, Gonçalves M, Moldenhauer G, Bulbuc N, Knabe S, et al. Co-stimulatory bispecific antibodies induce enhanced T cell activation and tumor cell killing in breast cancer models. Front Immunol (2021) 12. doi: 10.3389/fimmu.2021.719116
25. Park JA, NK V. Cheung. Overcoming tumor heterogeneity by ex vivo arming of T cells using multiple bispecific antibodies. J Immunother Cancer (2022) 10. doi: 10.1136/jitc-2021-003771
26. Krijgsman D, de Vries NL, Skovbo A, Andersen MN, Swets M, Bastiaannet E, et al. Characterization of circulating T-, NK-, and NKT cell subsets in patients with colorectal cancer: the peripheral blood immune cell profile. Cancer Immunol Immunother (2019) 68:1011–24. doi: 10.1007/s00262-019-02343-7
27. Steele NG, Carpenter ES, Kemp SB, Sirihorachai VR, The S, Delrosario L, et al. Multimodal mapping of the tumor and peripheral blood immune landscape in human pancreatic cancer. Nat Cancer (2020) 1:1097–112. doi: 10.1038/s43018-020-00121-4
28. Moradpoor R, Gharebaghian A, Shahi F, Mousavi A, Salari S, Akbari ME, et al. Identification and validation of stage-associated PBMC biomarkers in breast cancer using MS-based proteomics. Front Oncol (2020) 10. doi: 10.3389/fonc.2020.01101
29. Xu J, Sai H, Li Y, Jordan AC, McGettigan SE, Chen JH, et al. Peripheral blood T-cell fitness is diminished in patients with pancreatic carcinoma but can be improved with homeostatic cytokines. CMGH (2019) 8:656–658.e6. doi: 10.1016/j.jcmgh.2019.07.008
30. Oberg H-H, Grage-Griebenow E, Adam-Klages S, Jerg E, Peipp M, Kellner C, et al. Monitoring and functional characterization of the lymphocytic compartment in pancreatic ductal adenocarcinoma patients. Pancreatology (2016) 16:1069–79. doi: 10.1016/j.pan.2016.07.008
31. Gerlinger M, Rowan AJ, Horswell S, Larkin J, Endesfelder D, Gronroos E, et al. Intratumor heterogeneity and branched evolution revealed by multiregion sequencing. New Engl J Med (2012) 366:883–92. doi: 10.1056/NEJMoa1113205
32. Yates LR, Gerstung M, Knappskog S, Desmedt C, Gundem G, Van Loo P, et al. Subclonal diversification of primary breast cancer revealed by multiregion sequencing. Nat Med (2015) 21:751–9. doi: 10.1038/nm.3886
33. Quevedo R, Smirnov P, Tkachuk D, Ho C, El-Hachem N, Safikhani Z, et al. Assessment of genetic drift in large pharmacogenomic studies. Cell Syst (2020) 11:393–401.e2. doi: 10.1016/j.cels.2020.08.012
34. Pampaloni F, Reynaud EG, Stelzer EHK. The third dimension bridges the gap between cell culture and live tissue. Nat Rev Mol Cell Biol (2007) 8:839–45. doi: 10.1038/nrm2236
35. Kleinman HK, Philp D, Hoffman MP. Role of the extracellular matrix in morphogenesis. Curr Opin Biotechnol (2003) 14:526–32. doi: 10.1016/j.copbio.2003.08.002
36. Kim HS, Sung YJ, Paik S. Cancer cell line panels empower genomics-based discovery of precision cancer medicine. Yonsei Med J (2015) 56:1186–98. doi: 10.3349/ymj.2015.56.5.1186
37. Haibe-Kains B, El-Hachem N, Birkbak NJ, Jin AC, Beck AH, Aerts HJWL, et al. Inconsistency in large pharmacogenomic studies. Nature (2013) 504:389–93. doi: 10.1038/nature12831
38. Gillet JP, Varma S, Gottesman MM. The clinical relevance of cancer cell lines. J Natl Cancer Inst (2013) 105:452–8. doi: 10.1093/jnci/djt007
39. Kodack DP, Farago AF, Dastur A, Held MA, Dardaei L, Friboulet L, et al. Primary patient-derived cancer cells and their potential for personalized cancer patient care. Cell Rep (2017) 21:3298–309. doi: 10.1016/j.celrep.2017.11.051
40. Kornauth C, Pemovska T, Vladimer GI, Bayer G, Bergmann M, Eder S, et al. Functional precision medicine provides clinical benefit in advanced aggressive hematologic cancers and identifies exceptional responders. Cancer Discovery (2022) 12:372–87. doi: 10.1158/2159-8290.CD-21-0538
41. Beckinger S, Daunke T, Aldag L, Krüger S, Heckl S, Wesch D, et al. Hepatic myofibroblasts exert immunosuppressive effects independent of the immune checkpoint regulator PD-L1 in liver metastasis of pancreatic ductal adenocarcinoma. Front Oncol (2023) 13. doi: 10.3389/fonc.2023.1160824
42. Nunes AS, Barros AS, Costa EC, Moreira AF, Correia IJ. 3D tumor spheroids as in vitro models to mimic in vivo human solid tumors resistance to therapeutic drugs. Biotechnol Bioeng (2019) 116:206–26. doi: 10.1002/bit.26845
43. Gündel B, Liu X, Löhr M, Heuchel R. Pancreatic Ductal Adenocarcinoma: Preclinical in vitro and ex vivo Models. Front Cell Dev Biol (2021) 9. doi: 10.3389/fcell.2021.741162
44. Lin R-Z, Chou L-F, Chien C-CM, Chang H-Y. Dynamic analysis of hepatoma spheroid formation: roles of E-cadherin and β1-integrin. Cell Tissue Res (2006) 324:411–22. doi: 10.1007/s00441-005-0148-2
45. Hedemann N, Herz A, Schiepanski JH, Dittrich J, Sebens S, Dempfle A, et al. Adam17 inhibition increases the impact of cisplatin treatment in ovarian cancer spheroids. Cancers (Basel) (2021) 13. doi: 10.3390/cancers13092039
46. Raftery MJ, Franzén AS, Radecke C, Boulifa A, Schönrich G, Stintzing S. Next generation CD44v6-specific CAR-NK cells effective against triple negative breast cancer. Int J Mol Sci (2023) 24. doi: 10.3390/ijms24109038
47. Rodriguez-Perdigon M, Haeni L, Rothen-Rutishauser B, Rüegg C. Dual CSF1R inhibition and CD40 activation demonstrates anti-tumor activity in a 3D macrophage- HER2+ breast cancer spheroid model. Front Bioeng Biotechnol (2023) 11. doi: 10.3389/fbioe.2023.1159819
48. Sato T, Vries RG, Snippert HJ, Van De Wetering M, Barker N, Stange DE, et al. Single Lgr5 stem cells build crypt-villus structures in vitro without a mesenchymal niche. Nature (2009) 459:262–5. doi: 10.1038/nature07935
49. Driehuis E, van Hoeck A, Moore K, Kolders S, Francies HE, Gulersonmez MC, et al. Pancreatic cancer organoids recapitulate disease and allow personalized drug screening. Proc Natl Acad Sci (2019) 116:26580–90. doi: 10.1073/pnas.1911273116
50. Kretzschmar K. Cancer research using organoid technology. J Mol Med (2021) 99:501–15. doi: 10.1007/s00109-020-01990-z
51. Gao D, Vela I, Sboner A, Iaquinta PJ, Karthaus WR, Gopalan A, et al. Organoid cultures derived from patients with advanced prostate cancer. Cell (2014) 159:176–87. doi: 10.1016/j.cell.2014.08.016
52. Van De Wetering M, Francies HE, Francis JM, Bounova G, Iorio F, Pronk A, et al. Prospective derivation of a living organoid biobank of colorectal cancer patients. Cell (2015) 161:933–45. doi: 10.1016/j.cell.2015.03.053
53. Boj SF, Il Hwang C, Baker LA, Chio IIC, Engle DD, Corbo V, et al. Organoid models of human and mouse ductal pancreatic cancer. Cell (2015) 160:324–38. doi: 10.1016/j.cell.2014.12.021
54. Krieger TG, Le Blanc S, Jabs J, Ten FW, Ishaque N, Jechow K, et al. Single-cell analysis of patient-derived PDAC organoids reveals cell state heterogeneity and a conserved developmental hierarchy. Nat Commun (2021) 12:5826. doi: 10.1038/s41467-021-26059-4
55. Atanasova VS, de Jesus Cardona C, Hejret V, Tiefenbacher A, Mair T, Tran L, et al. Mimicking tumor cell heterogeneity of colorectal cancer in a patient-derived organoid-fibroblast model. Cell Mol Gastroenterol Hepatol (2023) 15:1391–419. doi: 10.1016/j.jcmgh.2023.02.014
56. Kim J, Koo BK, Knoblich JA. Human organoids: model systems for human biology and medicine. Nat Rev Mol Cell Biol (2020) 21:571–84. doi: 10.1038/s41580-020-0259-3
57. Sun CP, Lan HR, Fang XL, Yang XY, Jin KT. Organoid models for precision cancer immunotherapy. Front Immunol (2022) 13. doi: 10.3389/fimmu.2022.770465
58. Feig C, Gopinathan A, Neesse A, Chan DS, Cook N, Tuveson DA. The pancreas cancer microenvironment. Clin Cancer Res (2012) 18:4266–76. doi: 10.1158/1078-0432.CCR-11-3114
59. Huang L, Holtzinger A, Jagan I, Begora M, Lohse I, Ngai N, et al. Ductal pancreatic cancer modeling and drug screening using human pluripotent stem cell- and patient-derived tumor organoids. Nat Med (2015) 21:1364–71. doi: 10.1038/nm.3973
60. Verduin M, Hoeben A, Ruysscher D, Vooijs M. Patient-derived cancer organoids as predictors of treatment response. Front Oncol (2021) 11. doi: 10.3389/fonc.2021.641980
61. Magré L, Verstegen MMA, Buschow S, Laan LJWvd, Peppelenbosch M, Desai J. Emerging organoid-immune co-culture models for cancer research: From oncoimmunology to personalized immunotherapies. J Immunother Cancer (2023) 11. doi: 10.1136/jitc-2022-006290
62. Mo S, Tang P, Luo W, Zhang L, Li Y, Hu X, et al. Patient-derived organoids from colorectal cancer with paired liver metastasis reveal tumor heterogeneity and predict response to chemotherapy. Adv Sci (2022) 9. doi: 10.1002/advs.202204097
63. He X, Jiang Y, Zhang L, Li Y, Hu X, Hua G, et al. Patient-derived organoids as a platform for drug screening in metastatic colorectal cancer. Front Bioeng Biotechnol (2023) 11. doi: 10.3389/fbioe.2023.1190637
64. Ooft SN, Weeber F, Dijkstra KK, McLean CM, Kaing S, Werkhoven Ev. Patient-derived organoids can predict response to chemotherapy in metastatic colorectal cancer patients. Sci Transl Med (2019) 11. doi: 10.1126/scitranslmed.aay2574
65. Herpers B, Eppink B, James MI, Cortina C, Cañellas-Socias A, Boj SF, et al. Functional patient-derived organoid screenings identify MCLA-158 as a therapeutic EGFR × LGR5 bispecific antibody with efficacy in epithelial tumors. Nat Cancer (2022) 3:418–36. doi: 10.1038/s43018-022-00359-0
66. Dekkers JF, Alieva M, Cleven A, Keramati F, Wezenaar AKL, van Vliet EJ, et al. Uncovering the mode of action of engineered T cells in patient cancer organoids. Nat Biotechnol (2023) 41:60–9. doi: 10.1038/s41587-022-01397-w
67. Dijkstra KK, Cattaneo CM, Weeber F, Chalabi M, van de Haar J, Fanchi LF, et al. Generation of tumor-reactive T cells by co-culture of peripheral blood lymphocytes and tumor organoids. Cell (2018) 174:1586–1598.e12. doi: 10.1016/j.cell.2018.07.009
68. Cattaneo CM, Dijkstra KK, Fanchi LF, Kelderman S, Kaing S, van Rooij N, et al. Tumor organoid–T-cell coculture systems. Nat Protoc (2020) 15:15–39. doi: 10.1038/s41596-019-0232-9
69. Schuth S, Blanc S, Krieger TG, Jabs J, Schenk M, Giese NA, et al. Patient-specific modeling of stroma-mediated chemoresistance of pancreatic cancer using a three-dimensional organoid-fibroblast co-culture system. J Exp Clin Cancer Res (2022) 41. doi: 10.1186/s13046-022-02519-7
70. Go YH, Choi WH, Bae WJ, Jung SI, Cho CH, Lee SA, et al. Modeling pancreatic cancer with patient-derived organoids integrating cancer-associated fibroblasts. Cancers (Basel) (2022) 14. doi: 10.3390/cancers14092077
71. Forsythe SD, Erali RA, Sasikumar S, Laney P, Shelkey E, D’Agostino R, et al. Organoid platform in preclinical investigation of personalized immunotherapy efficacy in appendiceal cancer: Feasibility study. Clin Cancer Res (2021) 27:5141–51. doi: 10.1158/1078-0432.CCR-21-0982
72. Parikh AY, Masi R, Gasmi B, Hanada K, Parkhurst M, Gartner J, et al. Using patient-derived tumor organoids from common epithelial cancers to analyze personalized T-cell responses to neoantigens. Cancer Immunol Immunother (2023). doi: 10.1007/s00262-023-03476-6
73. Schnalzger TE, de Groot MH, Zhang C, Mosa MH, Michels BE, Röder J, et al. 3D model for CAR -mediated cytotoxicity using patient-derived colorectal cancer organoids. EMBO J (2019) 38. doi: 10.15252/embj.2018100928
74. Wang Z, McWilliams-Koeppen HP, Reza H, Ostberg JR, Chen W, Wang X, et al. 3D-organoid culture supports differentiation of human CAR+ iPSCs into highly functional CAR T cells. Cell Stem Cell (2022) 29:515–527.e8. doi: 10.1016/j.stem.2022.02.009
75. Tebon PJ, Wang B, Markowitz AL, Davarifar A, Tsai BL, Krawczuk P, et al. Drug screening at single-organoid resolution via bioprinting and interferometry. Nat Commun (2023) 14. doi: 10.1038/s41467-023-38832-8
76. Feodoroff M, Mikkonen P, Arjama M, Murumägi A, Kallioniemi O, Potdar S, et al. Protocol for 3D drug sensitivity and resistance testing of patient-derived cancer cells in 384-well plates. SLAS Discovery (2023) 28:36–41. doi: 10.1016/j.slasd.2022.11.003
77. Wensink GE, Elias SG, Mullenders J, Koopman M, Boj SF, Kranenburg OW, et al. Patient-derived organoids as a predictive biomarker for treatment response in cancer patients. NPJ Precis Oncol (2021) 5. doi: 10.1038/s41698-021-00168-1
78. Veninga V, Voest EE. Tumor organoids: Opportunities and challenges to guide precision medicine. Cancer Cell (2021) 39:1190–201. doi: 10.1016/j.ccell.2021.07.020
79. Loewa A, Feng JJ, Hedtrich S. Human disease models in drug development. Nat Rev Bioengineering (2023). doi: 10.1038/s44222-023-00063-3
80. Seppälä TT, Zimmerman JW, Suri R, Zlomke H, Ivey GD, Szabolcs A, et al. Precision medicine in pancreatic cancer: Patient derived organoid pharmacotyping is a predictive biomarker of clinical treatment response. Clin Cancer Res (2022) 28(15): 3296–307. doi: 10.1158/1078-0432.CCR-21-4165
81. Guillen KP, Fujita M, Butterfield AJ, Scherer SD, Bailey MH, Chu Z, et al. A human breast cancer-derived xenograft and organoid platform for drug discovery and precision oncology. Nat Cancer (2022) 3:232–50. doi: 10.1038/s43018-022-00337-6
82. van Renterghem AWJ, van de Haar J, Voest EE. Functional precision oncology using patient-derived assays: bridging genotype and phenotype. Nat Rev Clin Oncol (2023) 20:305–17. doi: 10.1038/s41571-023-00745-2
83. Osuna de la Peña D, Trabulo SMD, Collin E, Liu Y, Sharma S, Tatari M, et al. Bioengineered 3D models of human pancreatic cancer recapitulate in vivo tumour biology. Nat Commun (2021) 12(5623). doi: 10.1038/s41467-021-25921-9
84. Below CR, Kelly J, Brown A, Humphries JD, Hutton C, Xu J, et al. A microenvironment-inspired synthetic three-dimensional model for pancreatic ductal adenocarcinoma organoids. Nat Mater (2022) 21:110–9. doi: 10.1038/s41563-021-01085-1
85. Sachs N, Papaspyropoulos A, Ommen DDZ-v, Heo I, Böttinger L, Klay D, et al. Long-term expanding human airway organoids for disease modeling. EMBO J (2019) 38. doi: 10.15252/embj.2018100300
86. Chuprin J, Buettner H, Seedhom MO, Greiner DL, Keck JG, Ishikawa F, et al. Humanized mouse models for immuno-oncology research. Nat Rev Clin Oncol (2023) 20:192–206. doi: 10.1038/s41571-022-00721-2
87. Guil-Luna S, Sedlik C, Piaggio E. Humanized mouse models to evaluate cancer immunotherapeutics. Annu Rev Cancer Biol (2021) 5:119–36. doi: 10.1146/annurev-cancerbio-050520-100526
88. Teng R, Zhao J, Zhao Y, Gao J, Li H, Zhou S, et al. Chimeric antigen receptor-modified T cells repressed solid tumors and their relapse in an established patient-derived colon carcinoma xenograft model. J Immunother (2018) 42(2):33–42. doi: 10.1097/CJI.0000000000000251
89. Chen A, Neuwirth I, Herndler-Brandstetter D. Modeling the tumor microenvironment and cancer immunotherapy in next-generation humanized mice. Cancers (Basel) (2023) 15. doi: 10.3390/cancers15112989
90. Zhao Y, Shuen TWH, Toh TB, Chan XY, Liu M, Tan SY, et al. Development of a new patient-derived xenograft humanised mouse model to study human-specific tumour microenvironment and immunotherapy. Gut (2018) 67:1845–54. doi: 10.1136/gutjnl-2017-315201
91. Choi Y, Lee S, Kim K, Kim S-H, Chung Y-J, Lee C. Studying cancer immunotherapy using patient-derived xenografts (PDXs) in humanized mice. Exp Mol Med (2018) 50:1–9. doi: 10.1038/s12276-018-0167-1
92. Rosato RR, Dávila-González D, Choi DS, Qian W, Chen W, Kozielski AJ, et al. Evaluation of anti-PD-1-based therapy against triple-negative breast cancer patient-derived xenograft tumors engrafted in humanized mouse models. Breast Cancer Res (2018) 20:108. doi: 10.1186/s13058-018-1037-4
93. Woo XY, Giordano J, Srivastava A, Zhao ZM, Lloyd MW, de Bruijn R, et al. Conservation of copy number profiles during engraftment and passaging of patient-derived cancer xenografts. Nat Genet (2021) 53:86–99. doi: 10.1038/s41588-020-00750-6
94. Hoge ACH, Getz M, Zimmer A, Ko M, Raz L, Beroukhim R, et al. DNA-based copy number analysis confirms genomic evolution of PDX models. NPJ Precis Oncol (2022) 6. doi: 10.1038/s41698-022-00268-6
95. Gould SE, Junttila MR, De Sauvage FJ. Translational value of mouse models in oncology drug development. Nat Med (2015) 21:431–9. doi: 10.1038/nm.3853
96. Liu Y, Wu W, Cai C, Zhang H, Shen H, Han Y. Patient-derived xenograft models in cancer therapy: technologies and applications. Signal Transduct Target Ther (2023) 8:160. doi: 10.1038/s41392-023-01419-2
97. Zanella ER, Grassi E, Trusolino L. Towards precision oncology with patient-derived xenografts. Nat Rev Clin Oncol (2022) 19:719–32. doi: 10.1038/s41571-022-00682-6
98. De La Rochere P, Guil-Luna S, Decaudin D, Azar G, Sidhu SS, Piaggio E. Humanized mice for the study of immuno-oncology. Trends Immunol (2018) 39:748–63. doi: 10.1016/j.it.2018.07.001
99. Jin KT, Du WL, Lan HR, Liu YY, Sen Mao C, Du JL, et al. Development of humanized mouse with patient-derived xenografts for cancer immunotherapy studies: A comprehensive review. Cancer Sci (2021) 112:2592–606. doi: 10.1111/cas.14934
100. Brehm MA, Kenney LL, Wiles MV, Low BE, Tisch RM, Burzenski L, et al. Lack of acute xenogeneic graft-versus-host disease, but retention of T-cell function following engraftment of human peripheral blood mononuclear cells in NSG mice deficient in MHC class I and II expression. FASEB J (2019) 33:3137–51. doi: 10.1096/fj.201800636R
101. Holguin L, Echavarria L, Burnett JC. Novel Humanized Peripheral Blood Mononuclear Cell Mouse Model with Delayed Onset of Graft-versus-Host Disease for Preclinical HIV Research. J Virol (2022) 96(3):e01394-21. doi: 10.1128/JVI.01394-21
102. Meraz IM, Majidi M, Meng F, Shao RP, Ha MJ, Neri S, et al. An improved patient-derived xenograft humanized mouse model for evaluation of lung cancer immune responses. Cancer Immunol Res (2019) 7:1267–79. doi: 10.1158/2326-6066.CIR-18-0874
103. Jung J, Seol HS, Chang S. The generation and application of patient-derived xenograft model for cancer research. Cancer Res Treat (2018) 50:1–10. doi: 10.4143/crt.2017.307
104. Olson B, Li Y, Lin Y, Liu ET, Patnaik A. Mouse models for cancer immunotherapy research. Cancer Discovery (2018) 8:1358–65. doi: 10.1158/2159-8290.CD-18-0044
105. Hidalgo M, Amant F, Biankin AV, Budinská E, Byrne AT, Caldas C, et al. Patient-derived Xenograft models: An emerging platform for translational cancer research. Cancer Discovery (2014) 4:998–1013. doi: 10.1158/2159-8290.CD-14-0001
106. Izumchenko E, Paz K, Ciznadija D, Sloma I, Katz A, Vasquez-Dunddel D, et al. Patient-derived xenografts effectively capture responses to oncology therapy in a heterogeneous cohort of patients with solid tumors. Ann Oncol (2017) 28:2595–605. doi: 10.1093/annonc/mdx416
107. Gu Z, Jiang J, Yan Y, Tan T, Yu T, Liu Z, et al. Evaluation of the correlations between patient-derived xenograft (PDX) model-based mouse trials and cancer patient-based clinical trials. J Clin Oncol (2017) 35:e23140–0. doi: 10.1200/JCO.2017.35.15_suppl.e23140
108. Juric D, Castel P, Griffith M, Griffith OL, Won HH, Ellis H, et al. Convergent loss of PTEN leads to clinical resistance to a PI(3)Kα inhibitor. Nature (2015) 518:240–4. doi: 10.1038/nature13948
109. Bhatia SN, Ingber DE. Microfluidic organs-on-chips. Nat Biotechnol (2014) 32:760–72. doi: 10.1038/nbt.2989
110. Baptista LS, Porrini C, Kronemberger GS, Kelly DJ, Perrault CM. 3D organ-on-a-chip: The convergence of microphysiological systems and organoids. Front Cell Dev Biol (2022) 10. doi: 10.3389/fcell.2022.1043117
111. Lai BFL, Lu RXZ, Hu Y, Huyer LD, Dou W, Wang EY, et al. Recapitulating pancreatic tumor microenvironment through synergistic use of patient organoids and organ-on-a-chip vasculature. Adv Funct Mater (2020) 30:2000545. doi: 10.1002/adfm.202000545
112. Haque MR, Wessel CR, Leary DD, Wang C, Bhushan A, Bishehsari F. Patient-derived pancreatic cancer-on-a-chip recapitulates the tumor microenvironment. Microsyst Nanoeng (2022) 8. doi: 10.1038/s41378-022-00370-6
113. Geyer M, Gaul LM, Agosto SLD, Corbo V, Queiroz K. The tumor stroma influences immune cell distribution and recruitment in a PDAC-on-a-chip model. Front Immunol (2023) 14:1155085. doi: 10.3389/fimmu.2023.1155085
114. Gökçe F, Kaestli A, Lohasz C, de Geus M, Kaltenbach H, Renggli K, et al. Microphysiological drug-testing platform for identifying responses to prodrug treatment in primary leukemia. Adv Healthc Mater (2023) 12:2202506. doi: 10.1002/adhm.202202506
115. Nguyen M, De Ninno A, Mencattini A, Mermet-Meillon F, Fornabaio G, Evans SS, et al. Dissecting effects of anti-cancer drugs and cancer-associated fibroblasts by on-chip reconstitution of immunocompetent tumor microenvironments. Cell Rep (2018) 25:3884–3893.e3. doi: 10.1016/j.celrep.2018.12.015
116. Paterson K, Paterson S, Mulholland T, Coffelt SB, Zagnoni M. Assessment of CAR-T cell-mediated cytotoxicity in 3D microfluidic cancer co-culture models for combination therapy. IEEE Open J Eng Med Biol (2022) 3:86–95. doi: 10.1109/OJEMB.2022.3178302
117. Jiang X, Seo YD, Chang JH, Coveler A, Nigjeh EN, Pan S, et al. Long-lived pancreatic ductal adenocarcinoma slice cultures enable precise study of the immune microenvironment. Oncoimmunology (2017) 6:e1333210. doi: 10.1080/2162402X.2017.1333210
118. Seo YD, Jiang X, Sullivan KM, Jalikis FG, Smythe KS, Abbasi A, et al. Mobilization of CD8+ T cells via CXCR4 blockade facilitates PD-1 checkpoint therapy in human pancreatic cancer. Clin Cancer Res (2019) 25:3934–45. doi: 10.1158/1078-0432.CCR-19-0081
119. Braun R, Lapshyna O, Eckelmann S, Honselmann K, Bolm L, Winkel MT, et al. Organotypic slice cultures as preclinical models of tumor microenvironment in primary pancreatic cancer and metastasis. J Visualized Experiments (2021) 2021:e62541. doi: 10.3791/62541
120. Misra S, Moro CF, Chiaro MD, Pouso S, Sebestyén A, Löhr M, et al. Ex vivo organotypic culture system of precision-cut slices of human pancreatic ductal adenocarcinoma. Sci Rep (2019) 9:2133. doi: 10.1038/s41598-019-38603-w
121. Jiang X, Seo YD, Sullivan KM, Pillarisetty VG. Establishment of slice cultures as a tool to study the cancer immune microenvironment. In: Cancer Immunosurveillance (Humana New York, NY :Springer Science+Business Media, LLC, part of Springer Nature). (2019). doi: 10.1007/978-1-4939-8885-3
122. Lim CY, Chang JH, Lee WS, Lee KM, Yoon YC, Kim J, et al. Organotypic slice cultures of pancreatic ductal adenocarcinoma preserve the tumor microenvironment and provide a platform for drug response. Pancreatology (2018) 18:913–27. doi: 10.1016/j.pan.2018.09.009
123. Junk D, Krämer S, Broschewitz J, Laura H, Massa C, Moulla Y, et al. Human tissue cultures of lung cancer predict patient susceptibility to immune-checkpoint inhibition. Cell Death Discovery (2021) 7:264. doi: 10.1038/s41420-021-00651-5
124. Naipal KAT, Verkaik NS, Sánchez H, Deurzen CHMv, Bakker MAd, Hoeijmakers JHJ. Tumor slice culture system to assess drug response of primary breast cancer. BMC Cancer (2016) 16:78. doi: 10.1186/s12885-016-2119-2
125. Merz F, Gaunitz F, Dehghani F, Renner C, Meixensberger J, Gutenberg A, et al. Organotypic slice cultures of human glioblastoma reveal different susceptibilities to treatments. Neuro Oncol (2013) 15:670–81. doi: 10.1093/neuonc/not003
126. Qadir MMF, Álvarez-Cubela S, Weitz J, Panzer JK, Klein D, Moreno-Hernández Y. Long-term culture of human pancreatic slices as a model to study real-time islet regeneration. Nat Commun (2020) 11:3265. doi: 10.1038/s41467-020-17040-8
127. Davies EJ, Dong M, Gutekunst M, Närhi K, Zoggel HJAAV, Blom S, et al. Capturing complex tumour biology in vitro: Histological and molecular characterisation of precision cut slices. Sci Rep (2015) 5:17187. doi: 10.1038/srep17187
128. Zhao W, Dovas A, Spinazzi EF, Levitin HM, Banu MA, Upadhyayula P, et al. Deconvolution of cell type-specific drug responses in human tumor tissue with single-cell RNA-seq. Genome Med (2021) 13:82. doi: 10.1186/s13073-021-00894-y
129. Gahwiler BH, Rietschin L, Knöpfel T, Enz A. Continuous Presence of Nerve Growth Factor is required for Maintenance of cholinergic septal Neurons in organotypic Slice Cultures. Neuroscience (1990) 36:27–31. doi: 10.1016/0306-4522(90)90348-8
130. Decotret LR, Shi R, Thomas KN, Hsu M, Pallen CJ, Bennewith KL. Development and validation of an advanced ex vivo brain slice invasion assay to model glioblastoma cell invasion into the complex brain microenvironment. Front Oncol (2023) 13. doi: 10.3389/fonc.2023.976945
131. Zhu L, Retana D, García-Gómez P, Álvaro-Espinosa L, Priego N, Masmudi-Martín M, et al. A clinically compatible drug-screening platform based on organotypic cultures identifies vulnerabilities to prevent and treat brain metastasis. EMBO Mol Med (2022) 14:e14552. doi: 10.15252/emmm.202114552
132. Mackenzie NJ, Nicholls C, Templeton AR, Perera MPJ, Jeffery PL, Zimmermann K. Modelling the tumor immune microenvironment for precision immunotherapy. Clin Transl Immunol (2022) 11:e1400. doi: 10.1002/cti2.1400
133. Clinical Trials.gov. Search Term: Organoid. Available at: https://www.clinicaltrials.gov/search?cond=cancer&term=ORGANOID.
Keywords: organoids, organotypic tissue slice culture, organ-on-a-chip, patient-derived xenografts, tumor (immune) microenvironment, precision oncology, translational oncology
Citation: Kayser C, Brauer A, Susanne S and Wandmacher AM (2023) The challenge of making the right choice: patient avatars in the era of cancer immunotherapies. Front. Immunol. 14:1237565. doi: 10.3389/fimmu.2023.1237565
Received: 09 June 2023; Accepted: 24 July 2023;
Published: 10 August 2023.
Edited by:
Virginie Lafont, Institut National de la Santé et de la Recherche Médicale (INSERM), FranceReviewed by:
Narendra Sankpal, Saint Joseph Hospital Medical Center Phoenix, United StatesCopyright © 2023 Kayser, Brauer, Susanne and Wandmacher. This is an open-access article distributed under the terms of the Creative Commons Attribution License (CC BY). The use, distribution or reproduction in other forums is permitted, provided the original author(s) and the copyright owner(s) are credited and that the original publication in this journal is cited, in accordance with accepted academic practice. No use, distribution or reproduction is permitted which does not comply with these terms.
*Correspondence: Sebens Susanne, c3VzYW5uZS5zZWJlbnNAZW1haWwudW5pLWtpZWwuZGU=
†These authors have contributed equally to this work and share first authorship
‡These authors have contributed equally to this work and share last authorship
Disclaimer: All claims expressed in this article are solely those of the authors and do not necessarily represent those of their affiliated organizations, or those of the publisher, the editors and the reviewers. Any product that may be evaluated in this article or claim that may be made by its manufacturer is not guaranteed or endorsed by the publisher.
Research integrity at Frontiers
Learn more about the work of our research integrity team to safeguard the quality of each article we publish.