- 1Department of Dermatology, Xiangya Hospital, Central South University, Changsha, Hunan, China
- 2Hunan Key Laboratory of Aging Biology, Xiangya Hospital, Central South University, Changsha, Hunan, China
- 3National Clinical Research Center for Geriatric Disorders, Xiangya Hospital, Central South University, Changsha, Hunan, China
Background: Observational studies have shown that gut microbiota is closely associated with inflammatory dermatoses such as psoriasis, rosacea, and atopic dermatitis (AD). However, the causal relationship between gut microbiota and inflammatory dermatosis remains unclear.
Methods: Based on Maximum Likelihood (ML), MR-Egger regression, Inverse Variance Weighted (IVW), MR Pleiotropy RESidual Sum and Outlier (MR-PRESSO), Weighted Mode, and Weighted Median Estimator (WME) methods, we performed a bidirectional two-sample Mendelian randomization (MR) analysis to explore the causal relationship between gut microbiota and inflammatory dermatosis. The genome-wide association study (GWAS) summary data of gut microbiota came from the MiBioGen consortium, while the GWAS summary data of inflammatory dermatosis (including psoriasis, AD, rosacea, vitiligo, acne, and eczema) came from the FinnGen consortium and IEU Open GWAS project. Cochran’s IVW Q test tested the heterogeneity among instrumental variables (IVs). The horizontal pleiotropy was tested by MR-Egger regression intercept analysis and MR-PRESSO analysis.
Results: Eventually, the results indicated that 5, 16, 17, 11, 15, and 12 gut microbiota had significant causal effects on psoriasis, rosacea, AD, vitiligo, acne, and eczema, respectively, including 42 protective and 34 risk causal relationships. Especially, Lactobacilli and Bifidobacteria at the Family and Genus Level, as common probiotics, were identified as protective factors for the corresponding inflammatory dermatoses. The results of reverse MR analysis suggested a bidirectional causal effect between AD and genus Eubacterium brachy group, vitiligo and genus Ruminococcaceae UCG004. The causal relationship between gut microbiota and psoriasis, rosacea, acne, and eczema is unidirectional. There was no significant heterogeneity among these IVs. In conclusion, this bidirectional two-sample MR study identified 76 causal relationships between the gut microbiome and six inflammatory dermatoses, which may be helpful for the clinical prevention and treatment of inflammatory dermatoses.
Introduction
Inflammatory dermatoses represent a diverse group of diseases with multiple etiologies, including genetic factors, infections, and immune dysregulation (1), involving the activation of various immune cells and inflammatory mediators in both the innate and adaptive immune systems. Current studies have shown that gut microbiota may affect the host’s immune function. Under normal conditions, the interaction between gut microbiota and Toll-like receptors (TLR) on intestinal epithelial cells and immune cells facilitates homeostasis of the immune system (2). Gut microbiota may thus affect host skin immunity directly or indirectly through the gut-skin axis (3).
Various studies have shown significant differences in the gut microbial composition of patients with inflammatory dermatoses (4). For example, Bifidobacterium, considered a probiotic, decreases in eczema (5), acne (6), psoriases (7), and atopic dermatitis (AD) (8), but is enriched in rosacea (9). However, studies have varied in the relationship between gut microbiota and inflammatory dermatoses, such as Akkermansia muciniphila in psoriasis (10, 11). In observational studies, the association between the gut microbiota and inflammatory dermatoses is easily affected by confounding factors such as dietary patterns, environment, age, and lifestyle (12), making it challenging to draw a causal inference between gut microbiota and dermatoses.
The genome-wide association study (GWAS) establishes variant-trait associations by detecting genetic variation in individual genomes (13). Mendelian randomization (MR) integrates summary data from GWAS and explores causal relationships between exposure and outcomes by using exposure-related genetic variation as a substitute for exposure (14). Research design with MR follows the Mendelian inheritance law where “parental alleles are randomly assigned to offspring.” If genotype determines phenotype, the genotype is associated with a particular disease through the phenotype, and thus the association between inflammatory dermatoses and gut microbiota can be inferred using the genotype as an instrumental variable. MR is less prone to confounding factors because germline genetic variation is randomly assigned during meiosis and therefore reflects exposure without being affected by reverse causality. As an extension of the MR approach, bidirectional MR can be used to determine the direction of causality between two related phenotypes. In this study, we performed a bidirectional two-sample MR analysis of gut microbiota and six inflammatory dermatoses to reveal the causal relationships between gut microbiota and inflammatory dermatoses.
Methods
Data sources
This analysis employed the largest-scale gut microbiome genome-wide meta-analysis to date obtained from the international consortium MibioGen (15). The project studied the genome-wide genotypes and 16S ribosomal RNA gene sequencing of 18,340 participants in 24 cohorts from 11 countries, targeting variable regions V4, V3–V4, and V1–V2 of the 16S rRNA gene to delineate the microbial composition and to conduct classification using direct classification. Microbiome trait loci (mbTL) mapping was performed to identify genetic loci that affect the relative abundance in gut microflora. A total of 131 genera, 35 families, 20 orders, 16 classes, and 9 phyla with an average abundance of over 0.1% were included.
The GWAS summary data of Eczema came from the data released by the IEU Open GWAS project (GWAS ID: ieu-a-996, Trait name: Eczema) (16). The GWAS data included 11,059,641 single-nucleotide polymorphisms (SNPs) and 40,835 samples, including 10,788 in the case group and 30,047 in the control group. The GWAS summary data of Psoriasis (Number of SNPs = 16,380,464; ncase = 4,510; ncontrol = 212,242), Rosacea (Number of SNPs = 16,380,452; ncase = 1,195; ncontrol = 211,139), AD (Number of SNPs = 16,380,443; ncase = 7,021; ncontrol = 198,740), Vitiligo (Number of SNPs = 16,380,442; ncase = 131; ncontrol = 207,482), and Acne (Number of SNPs = 16,380,454; ncase = 1,299; ncontrol = 211,139) came from the data released by FinnGen consortium (GWAS ID: finn-b-L12_PSORIASIS, finn-b-L12_ROSACEA, finn-b-L12_ATOPIC, finn-b-L12_VITILIGO, and finn-b-L12_ACNE, Trait name: Psoriasis, Rosacea, Atopic dermatitis, Vitiligo, and Acne) (17). Age, sex, top 10 major components, and genotyping batches were corrected during the original author’s analysis (18). The details of the data sources used in this study are shown in Table S1 (Supplementary 1).
Instrumental variable (IV)
The bacterial taxa were classified into five hierarchical levels (phylum, class, order, family, and genus) to analyze, and each taxon was considered as a feature. SNPs associated with the gut microbiome were identified and used as instrumental variables. To ensure the authenticity and accuracy of the conclusions on the causal link between gut microbiome and inflammatory dermatoses risk, we selected instrumental variables (IVs) based on the following four criteria. First, the SNP-phenotype association level must reach the locus-wide significance threshold (P< 5 * 10-8) to select potential IVs. Unfortunately, only a small number of SNPs were selected as IVs. To explore more relationships between inflammatory dermatoses and gut microbiota to obtain more comprehensive results, the second threshold (P< 1 * 10-5) was used to identify SNPs which were selected as the second IV set. Second, SNPs with minor allele frequency (MAF) ≤ 0.01 were removed. Third, SNPs with R2 values<0.001 (clumping window size=10,000 kb) were filtered, and only the one with the lowest p-value was kept. Fourth, when palindromic SNPs existed, the allele frequency data were used to infer the forward-strand alleles.
Statistical analysis
The principle design of the whole study is shown in Figure 1. The detailed research flow chart and the three assumptions of Mendelian randomization analysis are presented in Figure S1 of Supplementary 2. In this study, the following popular MR methods were used to examine whether there was a causal association between gut microbiota and inflammatory dermatoses: Inverse Variance Weighted (IVW) test, Maximum Likelihood (ML), Weighted Mode, MR-Egger regression, Weighted median estimator (WME), and MR Pleiotropy RESidual Sum and Outlier (MR-PRESSO). IVW test obtains the overall estimation of the influence of the gut microbiota on inflammatory dermatoses, by combining the Wald estimates for each SNP with a meta-analytic approach. It ignores the intercept term in regression and uses the inverse of outcome variance (se2) as the weight for fitting. If there is no horizontal heterogeneity, the IVW results would be unbiased (19). The ML method is analogous to IVW, and its standard error is smaller when the same assumptions are met (20). Based on the assumption of instrument strength independent of direct effect (InSIDE), MR-Egger regression is used to evaluate the existence of horizontal pleiotropy with intercept terms (21). If the intercept term is zero, it indicates no horizontal pleiotropy, and the MR-Egger regression result agrees with IVW (21). The WME method can estimate causality more correctly when more than 50% of the instrumental variables are invalid (22). When the InSIDE assumption is not fulfilled, the Weighted Mode has been shown to have a superior ability to detect a causal effect, with less bias and a lower type I error rate than MR-Egger regression (22). We also identified and corrected pronounced outliers with MR-PRESSO tests and MR-Egger regression (23).
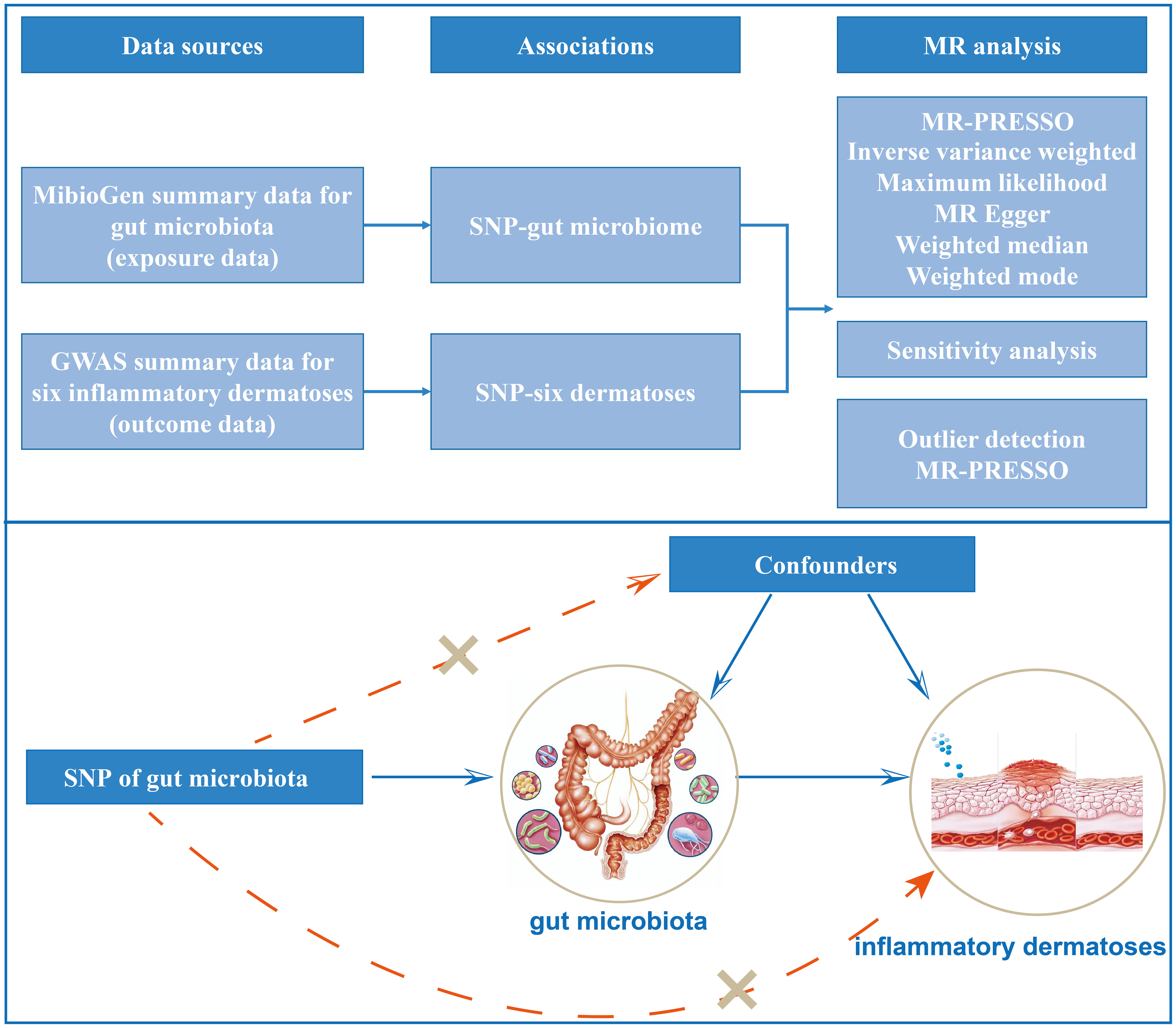
Figure 1 Study design of the two-sample Mendelian randomization for the effect of genetically predicted gut microbiome on inflammatory dermatoses.
Furthermore, we quantified the heterogeneity among the selected SNPs with Cochran’s Q statistic and identified potential heterogeneous SNPs with the “leave-one-out” analysis omitting each instrumental SNP in turn. Lastly, a reverse MR analysis of gut microbiota and inflammatory dermatoses was conducted with the same procedures and parameters as the forward MR.
In addition, we calculated the F statistic performed to assess the strength of the relevance between instrumental variables and exposure by the formula . R2 is the proportion of the variance of the trait accounted for by the SNP, k is the number of IVs, and n is the sample size (24). An F value over 10 indicates no significant weak instrumental bias. We utilized an online calculator tool available on https://shiny.cnsgenomics.com/mRnd/, developed by Marie-Jo A Brion et al., to determine the power of MR estimates (25, 26).
Furthermore, we utilized the PhenoScanner software to extract genes encompassing all identified SNPs and subsequently conducted a pathway enrichment analysis on those genes linked to SNPs contained within the instrumental variables of gut microbes demonstrating a significant causal association with inflammatory skin disorders (27). The enrichment analysis was executed using the clusterProfiler package (28). We omitted KEGG pathways with a P-value exceeding 0.05 and those with fewer than three mRNAs represented in the enrichment path.
False discovery rate (FDR) correction was conducted by applied fdrtools procedure, with a false discovery rate of FDR< 0.1 (29). All analysis was performed on R (version 4.2.2) and MR Analysis was based on MR-PRESSO (version 1.0) (23), TwoSampleMR (version 0.5.6) (30), and meta (version 6.2.1) packages (31).
Results
SNP selection
According to the selection criteria of IVs and removing the number of SNP repeated in different microorganisms, we identified 27 SNPs associated with gut microbiota at a significance level of p< 5 × 10−8. The details of 27 SNPs in the exposure and six outcome variables are shown in Table S2 (Supplementary 1). Such few IVs is not enough for high-performance MR analysis. Therefore, under the screening of another threshold p< 1 × 10−5, we got 2,123 SNPs associated with gut microbiota in psoriasis, AD, rosacea, vitiligo, and acne, and 2,155 SNPs for eczema. The details of 2,155 SNP in the exposure and six outcome variables are shown in Table S3 (Supplementary 1).
Causal effects of gut microbiota on the development of six inflammatory dermatoses
In our study, we employed gut microbiota as an exposure and investigated its association with six inflammatory dermatoses using MR analysis. We filtered the IVs based on a threshold of p< 5 × 10−8. As indicated in Table S4 (Supplementary 1), the limited number of available IVs restricted us to only two MR evaluation methods for analysis. However, the results showed that there was no causal relationship between the other five dermatoses and gut microbiota, except for acne, which had a causal relationship with a few gut microbiota. It is worth noting that MR analysis is not recommended when there are less than 3 IVs, as per the authoritative statement on MR analysis. Therefore, we opted to use IVs filtered by a threshold of p< 1 × 10−5 for subsequent MR analysis.
All identified gut microbiota with no less than 3 IVs were retained. The causal effects of the remaining 192 gut microbiota on psoriasis, rosacea, atopic dermatitis, vitiligo, and acne are shown in Table S5 (Supplementary 1).
Based on the estimate of IVW and ML, the result showed five, 18, 20, 14, 16, and 12 gut microbiotas had significant causal effects on psoriasis (Figure 2A), rosacea (Figure 2B), AD (Figure 2C), vitiligo (Figure 2D), acne (Figure 2E), and eczema (Figure 2F), respectively. It is worth noting that the common probiotics such as family Bifidobacteriaceae (OR = 0.82, 95%CI: 0.68–0.99, Pvalue = 0.0230, FDR = 0.0499) and genus Bifidobacterium (OR = 0.84, 95%CI: 0.73–0.98, Pvalue = 0.0197, FDR = 0.0289) have a protective effect on AD (Figure 2C). Order Lactobacillales (OR = 0.25, 95%CI: 0.06–0.97, Pvalue = 0.0447, FDR = 0.0757) had a protective effect on vitiligo (Figure 2D). In addition, family Bifidobacteriaceae (OR = 0.62, 95%CI: 0.43–0.90, Pvalue = 0.0118, FDR = 0.0319), family Lactobacillaceae (OR = 0.70, 95%CI: 0.51–0.96, Pvalue = 0.0159, FDR = 0.0260), and genus Lactobacillus (OR = 0.68, 95%CI: 0.51–0.91, Pvalue = 0.0068, FDR = 0.0103) have a protective effect on acne (Figure 2E).
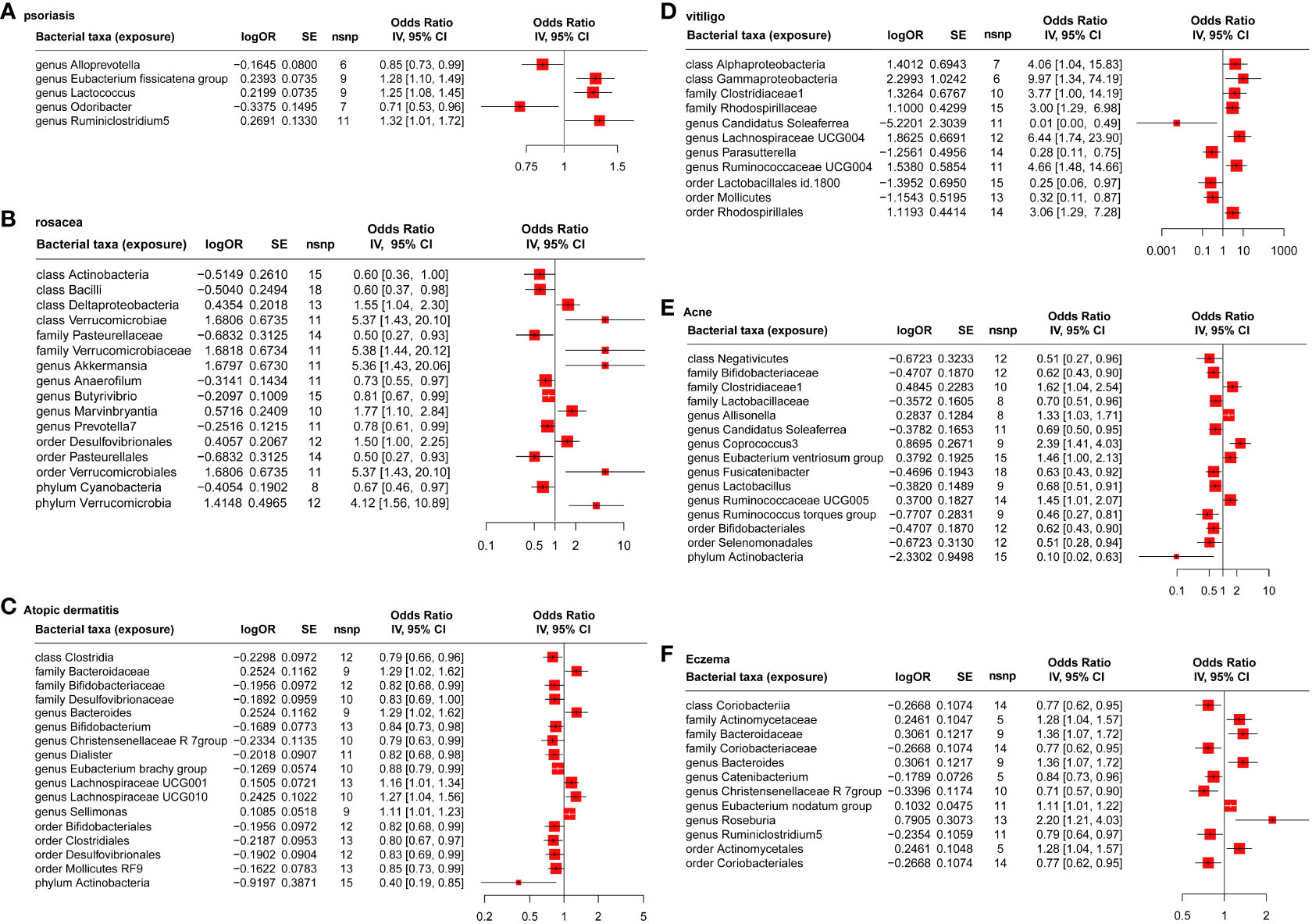
Figure 2 MR analysis of the causal relationship between genetically predicted gut microbiota and psoriasis (A), rosacea (B), atopic dermatitis (C), vitiligo (D), acne (E), and eczema (F).
Figure S2 (Supplementary 2) presents a consolidated network highlighting gut microbiota implicated in several inflammatory skin conditions concurrently.
Sensitivity analyses
As shown in Table S5 (Supplementary 1), the explaining rate of the total variation (R2 values) of the 192 gut microbiota in six inflammatory dermatoses ranged from 0.40% to 10.58%, and the F values ranged from 12.27 to 139.83, excluding the possibility of weak genetic tool variables. Based on Cochran’s IVW Q test, there was no significant heterogeneity among these IVs (Table S6 in Supplementary 1). In addition, according to the results of MR-Egger regression intercept analysis (Table S7 in Supplementary 1), except for phylum Verrucomicrobia (p = 0.029) on rosacea, the other 205 intestinal microorganisms had no significant horizontal pleiotropy. However, further MR-PRESSO analysis (Table S8 in Supplementary 1) did not find the horizontal pleiotropy in phylum Verrucomicrobia (Global test P-value = 0.356) on rosacea.
Bidirectional causal effects between gut microbiota and six inflammatory dermatoses
Based on the selection criteria of IVs, we obtained 38, 14, 58, 7, 12, and 42 SNPs (P< 1 * 10-5, R2< 0.001) significantly associated with psoriasis, rosacea, AD, vitiligo, acne, and eczema, respectively. Summaries and details of each SNP are presented in Table S9 (Supplementary 1). The results of reverse MR analysis (Table S10 in Supplementary 1) showed that AD was causally associated with genus Eubacterium brachy group (OR = 1.41, 95%CI: 1.07–1.87, Pvalue = 0.0185, FDR = 0.0442, MR Egger). Vitiligo was causally associated with genus Ruminococcaceae UCG004 (OR = 0.97, 95%CI: 0.95–0.99, Pvalue = 0.0197, FDR = 0.0279, IVW), which indicated a bidirectional causal effect between them. No other significant causal relationship was found between six inflammatory dermatoses and the gut microbiota (Table S10 in Supplementary 1). No notable heterogeneity was detected by Cochran’s Q statistics (Table S11 in Supplementary 1, P > 0.05). The results of MR-Egger regression intercept analysis indicated a significant horizontal pleiotropy when we evaluated the cause-effect of AD on genus Eubacterium brachy group (p = 0.0217) (Table S12 in Supplementary 1). But, further MR-PRESSO global test (Table S13 in Supplementary 1) suggested no evidence of pleiotropy (Global test P = 0.566).
By investigating the genes aligned with the instrumental variables of gut microbes showing a marked causal association with inflammatory skin disorders (refer to Table S14 in Supplementary 1) and conducting KEGG pathway enrichment analysis, we discerned a notable enrichment in inflammatory signaling pathways, including IL-17 signaling pathway, Chemokine signaling pathway and Cytokine-cytokine receptor interaction (refer to Table S15 in Supplementary 1, Figure S3 in Supplementary 2). This underlines the potential mechanisms by which gut microbes might influence the progression of inflammatory skin diseases via pathways like interleukins and cytokines.
Discussion
This study constituted the first-ever attempt to explore a causal relationship between gut microbiota and inflammatory dermatoses using the summary statistics of the largest genome-wide meta-analysis of gut microbiotas conducted by the MiBioGen consortium. Based on the two-sample MR analysis, we identified 88 causal relationships between the gut microbiome and six inflammatory dermatoses.
Previous studies generally tended to consider increased gut microbiota in inflammatory dermatoses as potential risk factors and vice versa. Despite rich findings in the past (7), our MR analysis showed only five genera associated with psoriasis. Nam JH et al. reported that the genus Desulfovibrio decreased in patients with rosacea (32), while MR analysis showed that the order Desulfovibrionales was a risk factor. MR analysis indicated that among the gut microbiota of patients with AD, the protective factors contained the genus Bifidobacterium, family Desulfovibrionaceae, phylum Actinobacteria, etc. In addition, the findings about the genera Dialister and Bacteroides were consistent with previous studies (33–35). Limited studies of the gut microbiota in vitiligo have unveiled an association between disease duration and Ruminococcus, which is consistent with MR results (36). For acne, related studies found a decrease in the genera Bifidobacterium, Lactobacillus, Ruminococcaceae and phylum Actinobacteria (6, 37), which was consistent with our MR analysis results. For patients with eczema, MR analysis identified the genus Bacteroides and family Bacteroidaceae as risk factors while the genus Christensenellaceae R-7 group was a protective factor, which was consistent with the previous findings (5, 38, 39). In comparison, we can find that many findings are consistent, confirming the reliability of our MR results. However, the correlation between gut microbiota and inflammatory dermatoses can hardly be indicated based on the altered abundance of the gut microbiota.
The microbiota, identified as protective or risk factors, have also been much studied in their impact on inflammatory dermatoses. Bacteria such as Akkermansia, Ruminococcus, Bifidobacterium, Eubacterium, and Coprococcus are producers of short-chain fatty acids (SCFAs) (40, 41), including acetate, propionate, and butyrate. SCFAs are transported from the intestine to the skin via peripheral circulation. They modulate the function of immune cell function to reduce inflammatory factor release (42), improve mitochondrial function (43), and promote keratinocyte metabolism and differentiation (43). These effects are achieved through binding to G protein-coupled receptors (GPCR) and peroxisome proliferator-activated receptor gamma (PPARγ) (42, 44), promoting mitochondrial fatty acid β-oxidation (FAO) (45), and inhibiting histone deacetylase (HDAC) (46). Epidermal keratinocytes can metabolize butyrate into long-chain fatty acids (LCFAs) and very long-chain fatty acids (VLCFAs), which contribute to ceramide synthesis for skin barrier repair (43). In addition, SCFAs benefit the intestinal barrier (47). Topical and oral administration of SCFAs or their derivatives have also been found to be effective in treating inflammatory dermatoses (48). Lactobacillus and Bifidobacterium may inhibit immune inflammation by increasing tryptophan (Trp) and Trp metabolites, maintaining intestinal barrier function on the one hand and reducing acne inflammation on the other (49, 50). Bifidobacterium longum can metabolize Trp to indole-3-carbaldehyde (I3C), activating the aryl hydrocarbon receptor (AHR)-mediated immune signaling pathway, suppressing Th2 cells, and thus relieving AD (51). Sulfate-reducing bacteria (SRB), including Desulfovibrionaceae, convert sulfate in the intestine to hydrogen sulfide (H2S), which impairs epithelial barrier function by interfering oxidation of butyrate in the colon, with subsequent disruption of intestinal permeability (52). Bifidobacterium, Lactobacillus, and Roseburia spp metabolize polyunsaturated fatty acids, including omega-3 and omega-6 fatty acids, to conjugated linoleic acid (CLA) (53, 54), thereby inhibiting COX-2/5-LOX and TLR4/NF-κB signaling pathway and attenuating skin lesions of AD (55). Oral administration of Lactobacillus can reduce insulin-like growth factor 1 (IGF-1) and increase forkhead box protein O1 (FoxO1) expression in the skin, improving the condition of acne (56). However, the microbiota of similar lineages may bring different outcomes for the same or different inflammatory dermatoses. For example, Ruminococcus produces SCFAs which can alleviate inflammatory dermatoses. However, the genera Ruminococcaceae UCG004 and Ruminocaccaceae UCG005 were identified as risk factors for vitiligo and acne, respectively, while the genus Ruminococcus torques group was identified as a protective factor for acne. Future studies should clarify the effects of different microbiota for different dermatoses.
Existing findings have pointed out a potential avenue for treating inflammatory dermatoses by manipulating the gut microbiota. From a dietary standpoint, dietary composition and bioactive substances can influence inflammatory dermatoses by altering the gut microbial structure (57–59). The intake of probiotics, prebiotics, and synbiotics also alleviates inflammatory dermatoses (60). It is important to emphasize that the common probiotics, Lactobacillus and Bifidobacterium were identified as protective factors for the corresponding inflammatory dermatoses. After receiving fecal microbiota transplantation (FMT) from healthy mice, AD mice experienced changes in their gut microbiota, including a significant increase in the family Desulfovibrionaceae and the genus Lactobacillus. The level of SCFA in the feces of AD mice increased after FMT, balancing the abnormal immune responses and alleviating AD skin lesions (61).
The present study has the following strengths. First, our study is the first to assess the bidirectional causal relationship between gut microbiota and inflammatory dermatoses using a two-sample MR analysis. Second, the results of the two-sample MR analysis were less susceptible to confounding factors, reverse causality, and exposure than in the observational study. Third, the strength of instruments in the MR analysis was ensured by using the most extensive available GWAS meta-analysis of the MiBioGen consortium; sensitivity analysis was performed to ensure the consistency of causal estimation and the robustness of the results; the detection and exclusion of horizontal pleiotropy by using MR-PRESSO and MR-Egger regression intercept term tests.
However, there are some limitations to this study. First, the analysis used pooled data of disease types and therefore did not allow for subgroup analysis around disease subtype and severity. Second, the main participants in GWAS are from Europe, so the extrapolation of results to other ethnicities will be limited. Third, the SNPs used in the analysis did not reach the traditional GWAS significance threshold (P< 5 × 10-8). Still, we needed to include more genetic variation as IV for sensitivity analysis and horizontal pleiotropy detection. When the P value is set to 5 × 10-8 or 1 × 10-6, only one or no SNP is available for each microbe. For this, we used FDR correction to restrict the possibility of false positives. There are a lot of high-level studies screened in this way (15, 62). Finally, the bacterial groups were analyzed only at the order or family level. Future studies using more advanced metagenome sequencing analysis will produce more specific and accurate results.
Conclusion
In conclusion, the results of our two-sample MR analysis support a potential causal relationship between gut microbiota and six inflammatory dermatoses. Various probiotics, including Lactobacillus and Bifidobacterium, have been shown to have protective causality against inflammatory dermatoses. However, the gut microbiota of similar lineages or that share the same characteristics in some way may bring different outcomes for the same or different inflammatory dermatoses. The results of this study deepen the understanding of the “gut-skin axis” and help to prevent and treat inflammatory dermatoses by regulating the structure of the gut microbiota.
Data availability statement
The datasets presented in this study can be found in online repositories. The names of the repository/repositories and accession number(s) can be found in the article/Supplementary Material.
Author contributions
RM: Conceptualization, Methodology, Software, Investigation, Visualization, Writing an original draft. QY: Methodology, Writing an original draft. JL: Conceptualization, Writing - original draft, Funding acquisition, Supervision. All authors contributed to the article and approved the submitted version.
Funding
This work was supported by the National Key Research and Development Program of China (No.2021YFF1201200), the National Natural Science Foundation of China (82273557, 82221002, 82173448, and 81874251), the National Natural Science Funds for Distinguished Young Scholars (82225039).
Acknowledgments
We thank all the researchers for providing their GWAS data. We also thank the participants and researchers of the IEU open GWAS project, FinnGen consortium, GWAS catalog, and the MiBioGen consortium.
Conflict of interest
The authors declare that the research was conducted in the absence of any commercial or financial relationships that could be construed as a potential conflict of interest.
Publisher’s note
All claims expressed in this article are solely those of the authors and do not necessarily represent those of their affiliated organizations, or those of the publisher, the editors and the reviewers. Any product that may be evaluated in this article, or claim that may be made by its manufacturer, is not guaranteed or endorsed by the publisher.
Supplementary material
The Supplementary Material for this article can be found online at: https://www.frontiersin.org/articles/10.3389/fimmu.2023.1231848/full#supplementary-material
Abbreviations
MR, Mendelian randomization; ML, maximum likelihood; IVW, Inverse variance weighted; MR-PRESSO, MR Pleiotropy RESidual Sum and Outlier; GWAS, genome-wide association study.
References
1. Liu TJ, Lin LL, McMeniman E, Wu J, Kao YC, Kumari S, et al. Cytokine/chemokine assessment as a complementary diagnostic tool for inflammatory skin diseases. Front Immunol (2022) 13:1028435. doi: 10.3389/fimmu.2022.1028435
2. Yiu JH, Dorweiler B, Woo CW. Interaction between gut microbiota and toll-like receptor: from immunity to metabolism. J Mol Med (Berlin Germany) (2017) 95(1):13–20. doi: 10.1007/s00109-016-1474-4
3. Salem I, Ramser A, Isham N, Ghannoum MA. The gut microbiome as a major regulator of the gut-skin axis. Front Microbiol (2018) 9:1459. doi: 10.3389/fmicb.2018.01459
4. Polkowska-Pruszyńska B, Gerkowicz A, Krasowska D. The gut microbiome alterations in allergic and inflammatory skin diseases - an update. J Eur Acad Dermatol Venereol: JEADV (2020) 34(3):455–64. doi: 10.1111/jdv.15951
5. Zheng H, Liang H, Wang Y, Miao M, Shi T, Yang F, et al. Altered gut microbiota composition associated with eczema in infants. PloS One (2016) 11(11):e0166026. doi: 10.1371/journal.pone.0166026
6. Yan HM, Zhao HJ, Guo DY, Zhu PQ, Zhang CL, Jiang W. Gut microbiota alterations in moderate to severe acne vulgaris patients. J Dermatol (2018) 45(10):1166–71. doi: 10.1111/1346-8138.14586
7. Olejniczak-Staruch I, Ciążyńska M, Sobolewska-Sztychny D, Narbutt J, Skibińska M, Lesiak A. Alterations of the skin and gut microbiome in psoriasis and psoriatic arthritis. Int J Mol Sci (2021) 22(8):3998. doi: 10.3390/ijms22083998
8. Penders J, Stobberingh EE, Thijs C, Adams H, Vink C, van Ree R, et al. Molecular fingerprinting of the intestinal microbiota of infants in whom atopic eczema was or was not developing. Clin Exp Allergy (2006) 36(12):1602–8. doi: 10.1111/j.1365-2222.2006.02599.x
9. Chen YJ, Lee WH, Ho HJ, Tseng CH, Wu CY. An altered fecal microbial profiling in rosacea patients compared to matched controls. J Formosan Med Assoc = Taiwan yi zhi (2021) 120(1 Pt 1):256–64. doi: 10.1016/j.jfma.2020.04.034
10. Tan L, Zhao S, Zhu W, Wu L, Li J, Shen M, et al. The akkermansia muciniphila is a gut microbiota signature in psoriasis. Exp Dermatol (2018) 27(2):144–9. doi: 10.1111/exd.13463
11. Huang L, Gao R, Yu N, Zhu Y, Ding Y, Qin H. Dysbiosis of gut microbiota was closely associated with psoriasis. Sci China Life Sci (2019) 62(6):807–15. doi: 10.1007/s11427-018-9376-6
12. Rinninella E, Raoul P, Cintoni M, Franceschi F, Miggiano GAD, Gasbarrini A, et al. What is the healthy gut microbiota composition? A changing ecosystem across age, environment, diet, and diseases. Microorganisms (2019) 7(1):14. doi: 10.3390/microorganisms7010014
13. Tam V, Patel N, Turcotte M, Bossé Y, Paré G, Meyre D. Benefits and limitations of genome-wide association studies. Nat Rev Genet (2019) 20(8):467–84. doi: 10.1038/s41576-019-0127-1
14. Emdin CA, Khera AV, Kathiresan S. Mendelian randomization. Jama (2017) 318(19):1925–6. doi: 10.1001/jama.2017.17219
15. Kurilshikov A, Medina-Gomez C, Bacigalupe R, Radjabzadeh D, Wang J, Demirkan A, et al. Large-scale association analyses identify host factors influencing human gut microbiome composition. Nat Genet (2021) 53(2):156–65. doi: 10.1038/s41588-020-00763-1
16. Paternoster L, Standl M, Waage J, Baurecht H, Hotze M, Strachan DP, et al. Multi-ancestry genome-wide association study of 21,000 cases and 95,000 controls identifies new risk loci for atopic dermatitis. Nat Genet (2015) 47(12):1449–56. doi: 10.1038/ng.3424
17. Kurki MI, Karjalainen J, Palta P, Sipilä TP, Kristiansson K, Donner KM, et al. Finngen provides genetic insights from a well-phenotyped isolated population. Nature (2023) 613(7944):508–18. doi: 10.1038/s41586-022-05473-8
18. Sakaue S, Kanai M, Tanigawa Y, Karjalainen J, Kurki M, Koshiba S, et al. A cross-population atlas of genetic associations for 220 human phenotypes. Nat Genet (2021) 53(10):1415–24. doi: 10.1038/s41588-021-00931-x
19. Burgess S, Dudbridge F, Thompson SG. Combining information on multiple instrumental variables in mendelian randomization: comparison of allele score and summarized data methods. Stat Med (2016) 35(11):1880–906. doi: 10.1002/sim.6835
20. Pierce BL, Burgess S. Efficient design for mendelian randomization studies: subsample and 2-sample instrumental variable estimators. Am J Epidemiol (2013) 178(7):1177–84. doi: 10.1093/aje/kwt084
21. Bowden J, Davey Smith G, Burgess S. Mendelian randomization with invalid instruments: effect estimation and bias detection through egger regression. Int J Epidemiol (2015) 44(2):512–25. doi: 10.1093/ije/dyv080
22. Hartwig FP, Davey Smith G, Bowden J. Robust inference in summary data mendelian randomization via the zero modal pleiotropy assumption. Int J Epidemiol (2017) 46(6):1985–98. doi: 10.1093/ije/dyx102
23. Verbanck M, Chen CY, Neale B, Do R. Detection of widespread horizontal pleiotropy in causal relationships inferred from mendelian randomization between complex traits and diseases. Nat Genet (2018) 50(5):693–8. doi: 10.1038/s41588-018-0099-7
24. Staiger D, Stock JHJE. Instrumental variables regression with weak instruments. Econometrica (1997) 65 557–86. doi: 10.3386/t0151
25. Burgess S. Sample size and power calculations in mendelian randomization with a single instrumental variable and a binary outcome. Int J Epidemiol (2014) 43(3):922–9. doi: 10.1093/ije/dyu005
26. rstudio. Online Sample Size and Power Calculator for Mendelian Randomization with a Binary Outcome (2013). Available at: https://sb452.shinyapps.io/power/.
27. Kamat MA, Blackshaw JA, Young R, Surendran P, Burgess S, Danesh J, et al. Phenoscanner V2: an expanded tool for searching human genotype-phenotype associations. Bioinf (Oxford England) (2019) 35(22):4851–3. doi: 10.1093/bioinformatics/btz469
28. Wu T, Hu E, Xu S, Chen M, Guo P, Dai Z, et al. Clusterprofiler 4.0: A universal enrichment tool for interpreting omics data. Innovation (Cambridge (Mass)) (2021) 2(3):100141. doi: 10.1016/j.xinn.2021.100141
29. Storey JD, Tibshirani R. Statistical significance for genomewide studies. Proc Natl Acad Sci United States America (2003) 100(16):9440–5. doi: 10.1073/pnas.1530509100
30. Hemani G, Tilling K, Davey Smith G. Orienting the causal relationship between imprecisely measured traits using gwas summary data. PloS Genet (2017) 13(11):e1007081. doi: 10.1371/journal.pgen.1007081
31. Balduzzi S, Rücker G, Schwarzer G. How to perform a meta-analysis with R: A practical tutorial. Evidence-Based Ment Health (2019) 22(4):153–60. doi: 10.1136/ebmental-2019-300117
32. Nam JH, Yun Y, Kim HS, Kim HN, Jung HJ, Chang Y, et al. Rosacea and its association with enteral microbiota in Korean females. Exp Dermatol (2018) 27(1):37–42. doi: 10.1111/exd.13398
33. Galazzo G, van Best N, Bervoets L, Dapaah IO, Savelkoul PH, Hornef MW, et al. Development of the microbiota and associations with birth mode, diet, and atopic disorders in a longitudinal analysis of stool samples, collected from infancy through early childhood. Gastroenterology (2020) 158(6):1584–96. doi: 10.1053/j.gastro.2020.01.024
34. Vael C, Desager K. The importance of the development of the intestinal microbiota in infancy. Curr Opin Pediatr (2009) 21(6):794–800. doi: 10.1097/MOP.0b013e328332351b
35. Ye S, Yan F, Wang H, Mo X, Liu J, Zhang Y, et al. Diversity analysis of gut microbiota between healthy controls and those with atopic dermatitis in a Chinese population. J Dermatol (2021) 48(2):158–67. doi: 10.1111/1346-8138.15530
36. Badal VD, Vaccariello ED, Murray ER, Yu KE, Knight R, Jeste DV, et al. The gut microbiome, aging, and longevity: A systematic review. Nutrients (2020) 12(12):3759. doi: 10.3390/nu12123759
37. Deng Y, Wang H, Zhou J, Mou Y, Wang G, Xiong X. Patients with acne vulgaris have a distinct gut microbiota in comparison with healthy controls. Acta dermato-venereologica (2018) 98(8):783–90. doi: 10.2340/00015555-2968
38. Zimmermann P, Messina N, Mohn WW, Finlay BB, Curtis N. Association between the intestinal microbiota and allergic sensitization, eczema, and asthma: A systematic review. J Allergy Clin Immunol (2019) 143(2):467–85. doi: 10.1016/j.jaci.2018.09.025
39. Hu C, van Meel ER, Medina-Gomez C, Kraaij R, Barroso M, Kiefte-de Jong J, et al. A population-based study on associations of stool microbiota with atopic diseases in school-age children. J Allergy Clin Immunol (2021) 148(2):612–20. doi: 10.1016/j.jaci.2021.04.001
40. Bian X, Wu W, Yang L, Lv L, Wang Q, Li Y, et al. Administration of akkermansia muciniphila ameliorates dextran sulfate sodium-induced ulcerative colitis in mice. Front Microbiol (2019) 10:2259. doi: 10.3389/fmicb.2019.02259
41. Reddel S, Del Chierico F, Quagliariello A, Giancristoforo S, Vernocchi P, Russo A, et al. Gut microbiota profile in children affected by atopic dermatitis and evaluation of intestinal persistence of a probiotic mixture. Sci Rep (2019) 9(1):4996. doi: 10.1038/s41598-019-41149-6
42. Yao Y, Cai X, Fei W, Ye Y, Zhao M, Zheng C. The role of short-chain fatty acids in immunity, inflammation and metabolism. Crit Rev Food Sci Nutr (2022) 62(1):1–12. doi: 10.1080/10408398.2020.1854675
43. Trompette A, Pernot J, Perdijk O, Alqahtani RAA, Domingo JS, Camacho-Muñoz D, et al. Gut-derived short-chain fatty acids modulate skin barrier integrity by promoting keratinocyte metabolism and differentiation. Mucosal Immunol (2022) 15(5):908–26. doi: 10.1038/s41385-022-00524-9
44. Krejner A, Bruhs A, Mrowietz U, Wehkamp U, Schwarz T, Schwarz A. Decreased expression of G-protein-coupled receptors gpr43 and gpr109a in psoriatic skin can be restored by topical application of sodium butyrate. Arch Dermatol Res (2018) 310(9):751–8. doi: 10.1007/s00403-018-1865-1
45. Bachem A, Makhlouf C, Binger KJ, de Souza DP, Tull D, Hochheiser K, et al. Microbiota-derived short-chain fatty acids promote the memory potential of antigen-activated cd8(+) T cells. Immunity (2019) 51(2):285–97.e5. doi: 10.1016/j.immuni.2019.06.002
46. Thiruvengadam M, Subramanian U, Venkidasamy B, Thirupathi P, Samynathan R, Shariati MA, et al. Emerging role of nutritional short-chain fatty acids (Scfas) against cancer via modulation of hematopoiesis. Crit Rev Food Sci Nutr (2023) 63(6):827–44. doi: 10.1080/10408398.2021.1954874
47. De Benedetto A, Rafaels NM, McGirt LY, Ivanov AI, Georas SN, Cheadle C, et al. Tight junction defects in patients with atopic dermatitis. J Allergy Clin Immunol (2011) 127(3):773–86.e1-7. doi: 10.1016/j.jaci.2010.10.018
48. Xiao X, Hu X, Yao J, Cao W, Zou Z, Wang L, et al. The role of short-chain fatty acids in inflammatory skin diseases. Front Microbiol (2022) 13:1083432. doi: 10.3389/fmicb.2022.1083432
49. Jung GW, Tse JE, Guiha I, Rao J. Prospective, randomized, open-label trial comparing the safety, efficacy, and tolerability of an acne treatment regimen with and without a probiotic supplement and minocycline in subjects with mild to moderate acne. J cutaneous Med Surg (2013) 17(2):114–22. doi: 10.2310/7750.2012.12026
50. Więdłocha M, Marcinowicz P, Janoska-Jaździk M, Szulc A. Gut microbiota, kynurenine pathway and mental disorders - review. Prog Neuropsychopharmacol Biol Psychiatry (2021) 106:110145. doi: 10.1016/j.pnpbp.2020.110145
51. Fang Z, Pan T, Li L, Wang H, Zhu J, Zhang H, et al. Bifidobacterium longum mediated tryptophan metabolism to improve atopic dermatitis via the gut-skin axis. Gut Microbes (2022) 14(1):2044723. doi: 10.1080/19490976.2022.2044723
52. Blachier F, Beaumont M, Kim E. Cysteine-derived hydrogen sulfide and gut health: A matter of endogenous or bacterial origin. Curr Opin Clin Nutr Metab Care (2019) 22(1):68–75. doi: 10.1097/mco.0000000000000526
53. Fang Z, Li L, Zhang H, Zhao J, Lu W, Chen W. Gut microbiota, probiotics, and their interactions in prevention and treatment of atopic dermatitis: A review. Front Immunol (2021) 12:720393. doi: 10.3389/fimmu.2021.720393
54. Kishino S, Takeuchi M, Park SB, Hirata A, Kitamura N, Kunisawa J, et al. Polyunsaturated fatty acid saturation by gut lactic acid bacteria affecting host lipid composition. Proc Natl Acad Sci United States America (2013) 110(44):17808–13. doi: 10.1073/pnas.1312937110
55. Tang L, Li XL, Deng ZX, Xiao Y, Cheng YH, Li J, et al. Conjugated linoleic acid attenuates 2,4-dinitrofluorobenzene-induced atopic dermatitis in mice through dual inhibition of cox-2/5-lox and tlr4/nf-Kb signaling. J Nutr Biochem (2020) 81:108379. doi: 10.1016/j.jnutbio.2020.108379
56. Fabbrocini G, Bertona M, Picazo Ó, Pareja-Galeano H, Monfrecola G, Emanuele E. Supplementation with lactobacillus rhamnosus sp1 normalises skin expression of genes implicated in insulin signalling and improves adult acne. Beneficial Microbes (2016) 7(5):625–30. doi: 10.3920/bm2016.0089
57. Nayak RR. Western diet and psoriatic-like skin and joint diseases: A potential role for the gut microbiota. J Invest Dermatol (2021) 141(7):1630–2. doi: 10.1016/j.jid.2021.01.003
58. Gürtler A, Laurenz S. The impact of clinical nutrition on inflammatory skin diseases. J der Deutschen Dermatologischen Gesellschaft = J German Soc Dermatol: JDDG (2022) 20(2):185–202. doi: 10.1111/ddg.14683
59. Lee SY, Hong SH, Kim HI, Ku JM, Choi YJ, Kim MJ, et al. Paeonia lactiflora pallas extract alleviates antibiotics and dncb-induced atopic dermatitis symptoms by suppressing inflammation and changing the gut microbiota composition in mice. Biomed pharmacother = Biomed pharmacother (2022) 154:113574. doi: 10.1016/j.biopha.2022.113574
60. Buhaş MC, Gavrilaş LI, Candrea R, Cătinean A, Mocan A, Miere D, et al. Gut microbiota in psoriasis. Nutrients (2022) 14(14):2970. doi: 10.3390/nu14142970
61. Kim JH, Kim K, Kim W. Gut microbiota restoration through fecal microbiota transplantation: A new atopic dermatitis therapy. Exp Mol Med (2021) 53(5):907–16. doi: 10.1038/s12276-021-00627-6
Keywords: gut microbiota, two-sample mendelian randomization, psoriasis, rosacea, atopic dermatitis, vitiligo, eczema, acne
Citation: Mao R, Yu Q and Li J (2023) The causal relationship between gut microbiota and inflammatory dermatoses: a Mendelian randomization study. Front. Immunol. 14:1231848. doi: 10.3389/fimmu.2023.1231848
Received: 31 May 2023; Accepted: 12 September 2023;
Published: 27 September 2023.
Edited by:
Floriana Cappiello, Sapienza University of Rome, ItalyReviewed by:
Ignacio Montero, MicroViable Therapeutics SL, SpainMichael S. Granitsiotis, Stilla Technologies Inc., United States
Copyright © 2023 Mao, Yu and Li. This is an open-access article distributed under the terms of the Creative Commons Attribution License (CC BY). The use, distribution or reproduction in other forums is permitted, provided the original author(s) and the copyright owner(s) are credited and that the original publication in this journal is cited, in accordance with accepted academic practice. No use, distribution or reproduction is permitted which does not comply with these terms.
*Correspondence: Ji Li, liji_xy@csu.edu.cn
†These authors have contributed equally to this work