- Department of Hepatology, The First Hospital of Jilin University, Changchun, China
Introduction: Non-alcoholic fatty liver disease (NAFLD), the emerging cause of end-stage liver disease, is the most common liver disease. Determining the independent risk factors of NAFLD and patients who need more monitoring is important.
Methods: Two-Sample Mendelian randomization (MR) was performed in the analysis to investigate the causal association of different autoimmune diseases with NAFLD using summary level data. Genome-wide association study (GWAS) of 5 autoimmune diseases including celiac disease (CeD), Crohn’s disease (CD), multiple sclerosis (MS), rheumatoid arthritis (RA), and type 1 diabetes (T1D) were selected for Instrument variables (IVs). NAFLD was included as outcome.
Result: After adjusting for confounding factors, genetic predisposition of CeD (OR= 0.973, [0.949,0.997], IVW p-value=0.026), MS (OR= 1.048, [1.012,1.085], IVW p-value= 0.008), RA (OR= 1.036, [1.006,1.066], IVW p-value=0.019), T1D (OR= 1.039, [1.002,1.079], IVW p-value= 0.041) is causally associated with NAFLD. No causal effect was found between CD and NAFLD.
Conclusion: CeD itself may be a protective factor for NAFLD, the results of previous observational studies have been influenced by confounding factors, and the morbidity of NAFLD may be higher in patients with MS, RA, and T1D than in common populations, and monitoring the prevalence of NAFLD in these populations is considerable.
1 Introduction
Non-alcoholic fatty liver disease (NAFLD) is the most common chronic liver disease in the world (1), with a prevalence of close to 25% in the general population, causally associated with obesity and type 2 diabetes (T2D) (2). NAFLD includes a spectrum of liver pathologies including simple steatosis, steatohepatitis, fibrosis, and cirrhosis. Due to its high prevalence, NAFLD is currently the fastest-growing cause of liver-related death worldwide and is emerging as a significant cause of end-stage liver disease, primary liver cancer, liver transplantation, and a significant health economic burden (3). Therefore, it is important to determine the independent risk factors for NAFLD and which patients should receive more stringent health monitoring.
Autoimmune diseases occur when the immune response is misdirected to the tissues of the self, ultimately leading to structural and functional disorders of the body and organs. Genetic factors, environmental influence, and immune abnormalities (including infections) often combine to trigger autoimmune diseases (4). There are nearly 100 identified autoimmune diseases, the most common of which include systemic lupus erythematosus (SLE), rheumatoid arthritis (RA), multiple sclerosis (MS), type 1 diabetes (T1D), Graves’ disease (GD), inflammatory bowel disease (IBD), etc. (5). Considerable epidemiological evidence links these disorders to NAFLD, and most are tend to increasing risk of increased NAFLD (6–10).
The gold standard for exploring causality is randomized controlled trials. In theory, randomized controlled trials require strict randomization of patients with different autoimmune diseases, intervention, and follow-up to document the incidence of NAFLD. Such trials are difficult to conduct because of the time and high cost required. Mendelian randomization (MR) is a statistical research method similar to randomized controlled trials, where MR uses a genetic variation to determine whether the observed association between exposure and outcome is consistent with a causal effect (11). MR relies on the natural random combination of genetic variants during meiosis, resulting in a random distribution of genetic variants in the population (11), and is not prone to confounding factors and reverse causal associations.
In this study, MR was used to investigate the causal relationship between several autoimmune diseases and NAFLD.
2 Methods
2.1 Two-sample MR study design
Based on summary-level data, two-sample MR was performed to investigate the causal association between different autoimmune diseases and NAFLD. A total of 5 autoimmune diseases including celiac disease (CeD), Crohn’s disease (CD), multiple sclerosis (MS), rheumatoid arthritis (RA), and type 1 diabetes (T1D) were selected for our MR study (12–18), Table 1 provides detailed information on all the summary data used in the study.
MR studies should be conducted under three main assumptions (19): Genetic variables should be closely related to exposure (12–16); Genetic variables should not be associated with any confounders that may affect exposure and outcome; Genetic variables can only influence outcomes through exposure. First of all, Qualified IVs were selected based on strict selection criteria. Genetic variants were selected as IVs based on their genome-wide significance (GWAS p-value < 5×10−8) and linkage disequilibrium. (LD, r2 = 0.001, clump window 5000 kilobases). For the selection of qualified IVs, only summary-level GWAS data from European ancestry were utilized. Secondly, SNPs (Single Nucleotide Polymorphism) associated with obesity and T2D were excluded from exposure by Phenoscanner (PhenoScanner (cam.ac.uk)). It is known that obesity and type 2 diabetes are risk factors for NAFLD (3), Inclusion of these SNPs would violate the second and third assumptions (20) (Figure 1). Additionally, exposure and outcome data were harmonized to ensure that each IV had the same effect allele. Finally, MR analysis was performed on the eligible SNPs.
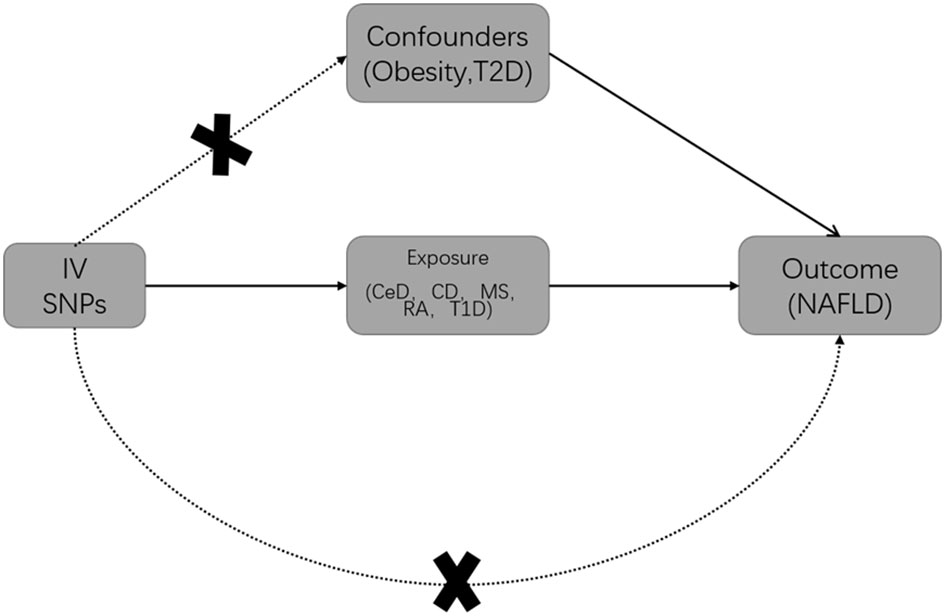
Figure 1 Basic Mendelian randomization (MR) framework for investigating the causal relationship between autoimmune diseases and NAFLD. T2D is Type 2 diabetes; IV is instrumental variable; CeD is celiac disease; CD is Crohn’s disease; MS is multiple sclerosis; RA is rheumatoid arthritis; T1D is Type 1 diabetes; NAFLD is non-alcoholic fatty liver disease.
2.2 Data source for autoimmune diseases and NAFLD
2.2.1 Celiac disease
The IEU Open GWAS project (mrcieu.ac.uk) provided summary-level data for CeD (12), which included 12,041 cases and 12,228 controls, identifying 38 SNPs associated with CeD. A total of 35 exposure-related SNPs were found in the outcome, 3 SNPs associated with potential confounders (rs3184504, rs6457617, rs13195040), and no SNPs were excluded through harmonization.
2.2.2 Crohn’s disease
The IEU Open GWAS project (mrcieu.ac.uk) provided summary-level data for CD (13), which included 17,897 cases and 33,977 controls, identifying 130 SNPs associated with CD. A total of 122 exposure-related SNPs were found in the outcome, and 12 SNPs associated with potential confounders (rs13109404, rs13407913, rs17391694, rs2641348, rs2641348, rs26528, rs3184504, rs3197999, rs56163845, rs6908425, rs77981966, rs780094, rs9264942) and 7 SNPs palindromic with intermediate allele frequencies (rs10878302, rs10956252, rs10995271, rs1927681, rs2266961, rs2847293, rs35730213) were excluded.
2.2.3 Multiple sclerosis
The IEU Open GWAS project (mrcieu.ac.uk) provided summary-level data for MS (14), which included 14,498 cases and 24,091 controls, identifying 47 SNPs associated with MS. A total of 45 exposure-related SNPs were found in the outcome, including two potential confounders (rs11554159, rs3131283), and no SNPs were excluded through harmonization.
2.2.4 Rheumatoid arthritis
The IEU Open GWAS project (mrcieu.ac.uk) provided summary-level data for RA (mrcieu.ac.uk) (15). The GWAS included 5,539 cases and 20,169 controls, identifying 10 SNPs associated with RA. All of the exposure-related SNPs were found in the outcome, one SNP associated with potential confounders (rs497239), and no SNPs were excluded through harmonization.
2.2.5 Type 1 diabetes
The IEU Open GWAS project (mrcieu.ac.uk) provided summary-level data for T1D (16). The GWAS included 9,266 cases and 15,574 controls, identifying 47 SNPs associated with T1D. A total of 33 exposure-related SNPs were found in the outcome, one SNP associated with potential confounders (rs8056814) and 6 SNPs palindromic with intermediate allele frequencies (rs10865468, rs17125653, rs34296259, rs34536443, rs55996894, rs689) were excluded.
2.2.6 NAFLD
Our study mainly focused on the association between CeD, CD, MS, RA, and T1D. For the outcome data, we chose recent GWAS summary data to date. The sources of datasets are listed in Table 1.
Summary-level data for NAFLD were acquired from the GWAS Catalog (https://www.ebi.ac.uk/gwas/home), they performed 2 new GWASs in the UK Biobank and Estonian Biobank and performed a meta-analysis of 4 cohorts (UK Biobank, Estonian Biobank, eMERGE, and FinnGen), totaling 8,434 NAFLD cases, all identified through EHRs (electronic health records), with 770,180 controls (17). All the GWAS have been approved by corresponding Ethics Committees.
2.3 Data reliability analysis
As mentioned above, obesity and T2D are important risk factors for NAFLD (3), and epidemiological and animal experimental evidence for these conclusions has been validated in previous MR analyses (21), and both factors were excluded as confounders in our IVs, so we selected these two factors to validate the reliability of the NAFLD database.
Summary data for BMI, including 461,460 European ancestry individuals, were obtained from the consortium of the MRC Integrative Epidemiology Unit (MRC-IEU) (GWAS ID: ukb-b-19953), and summary data of T2D were retrieved from the Meta-analysis of three GWAS datasets of European ancestry in a very large sample of T2D (62,892 cases and 596,424 controls) (22).
Multiple testing was controlled by the Benjamini-Hochberg method. Results with an adjusted FDR less than 0.05 were considered to be significantly causally related. In addition, results at a threshold of FDR less than 0.1 were considered to imply an significant association.
2.4 Statistic analysis and data visualization
To estimate the combined effect of all SNPs, the inverse-variance-weighted (IVW) method was performed (23). Additionally, as complementary MR methods, weighted median (24), MR-Egger regression (25), simple mode, and weighted mode (26), were performed.
As a result of differences in analysis platforms, experimental conditions, inclusion populations, and SNPs, two-sample MR analysis may have heterogeneity. Therefore, the Cochrane’s Q test was performed to appraise heterogeneity, if the P-value > 0.05, it is considered that there is no heterogeneity in the IVs, and the influence of heterogeneity on the estimation of causal effects can be ignored (27). Forest plots visualized the heterogeneity (28)., MR-Egger regression analysis was used to calculate the intercept and P value of the sensitive SNPs of horizontal pleiotropy.
Statistic analysis and data visualization were performed using TwoSampleMR package (version 0.5.6) (23) and MR-PRESSO package (29) in R (version 4.2.2).
3 Result
Initially, neither CeD (OR=0.987, [0.971, 1.003], IVW p-value=0.118, FDR=0.131) nor CD (OR=1.027, [0.997,1.058], IVW p-value=0.082, FDR=0.103) was causally associated with NAFLD in the preliminary analysis. After adjustment for BMI and T2D, there was a significant negative association between CeD (OR= 0.973,[0.949,0.997], IVW p-value=0.026, FDR=0.065) and NAFLD, although there was still no causal association between CD (OR=1.020, [0.990, 1.051], IVW p-value= 0.185, FDR=0.185) and NAFLD (Figure 2). The results of other methods, such as IVW fixed effect model, weighted median method,and MR-Egger method, show consistent influence direction and significant association between CeD and NAFLD. (Table 2, Supplementary Figures) Cochran’s Q statistic (Q=24.508, p=0.789) suggested low heterogeneity, MR-Egger regression showed non-horizontal pleiotropy (intercept=0.011, p=0.061) and MR-PRESSO method did not find any outlier SNPs. (Table 2) Crohn’s disease was also less probable to be influenced by heterogeneity and horizontal pleiotropy (Q=113.068, p= 0.213; intercept=0.006, p=0.279) (Table 2, Supplementary Figures).
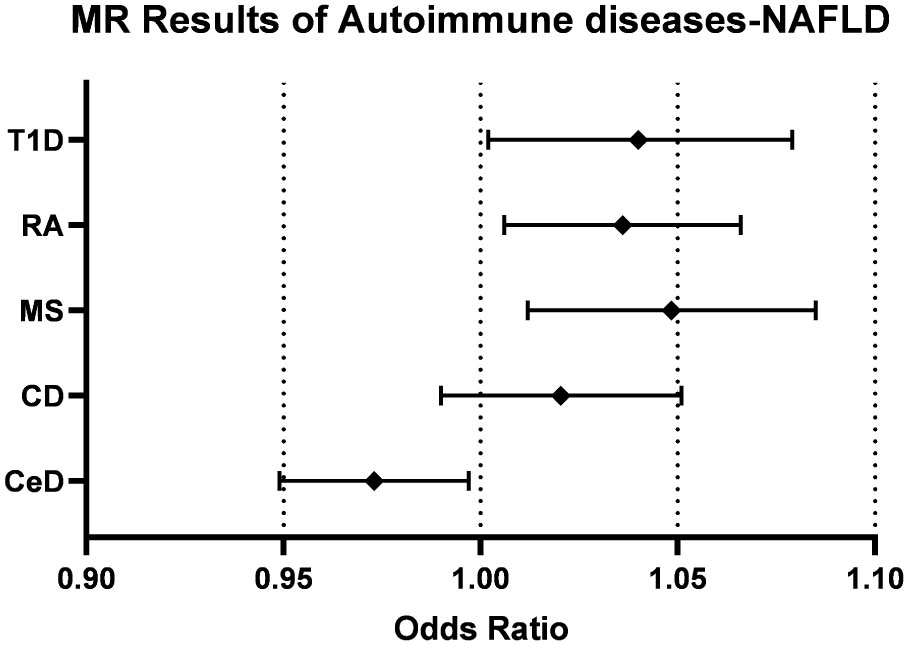
Figure 2 Forest plots of the causal effect of autoimmune diseases on NAFLD. CeD, celiac disease; CD, Crohn’s disease; MS, multiple sclerosis; RA, rheumatoid arthritis; T1D, Type 1 diabetes; NAFLD, non-alcoholic fatty liver disease.
The impact of genetic predisposition of MS (OR=1.047, [1.012,1.083], IVW p-value=0.008, FDR=0.040), RA (OR=1.031, [1.002,1.061], IVW p-value=0.034, FDR=0.066) and T1D (OR=1.038, [1.001,1.076], IVW p-value=0.046, FDR=0.066) all elevated the risk of NAFLD in IVW model. (Table 2) These conclusions were still valid after adjusting confounders, including MS (OR= 1.048, [1.012,1.085], IVW p-value= 0.008, FDR=0.040), RA (OR=1.036, [1.006,1.066], IVW p-value=0.019, FDR=0.063), T1D (OR= 1.039, [1.002,1.079], IVW p-value= 0.041, 0.066). (Table 2, Figure 2).
Sensitivity analysis indicated that the results were not biased by heterogeneity or horizontal pleiotropy: MS (Q=31.997, p=0.868; intercept=0.001, p=0.874), RA (Q=7.620, p=0.471; intercept=0.016, p=0.235), T1D (Q=28.322, p=0.293; intercept=0.002, p=0.837), MR-PRESSO method did not find any outlier SNPs (Table 2, Supplementary Figures).
IVW method indicated causal effect between genetically predicted BMI and NAFLD (OR= 1.715, [1.520,1.934], p-value= 1.701e-18). Genetically predicted T2D-NAFLD also revealed significant association in IVW method (OR= 1.104, [1.009,1.209], p-value= 0.032).
4 Discussion
Genetic susceptibility to all four diseases suggested a significant association with NAFLD, except for CD, which was not associated with NAFLD. CeD was negatively correlated with NAFLD. On the contrary, MS, RA, and T1D all increased the incidence of NAFLD slightly.
Celiac disease, an autoimmune disorder, predominantly impacts the small intestine and arises from the consumption of gluten in genetically predisposed individuals. CeD is widely believed to elevate the risk of NAFLD (7, 30). A cohort study indicated a 13.3 (95% CI 3.5-50.3) elevated risk of NAFLD in CeD patients compared to the general population in the first year after diagnosis of CeD, and continued to be significantly higher 15-year after diagnosis (HR = 2.5; 95% CI 1.0-5.9) (7). Another prospective cohort study showed that CeD patients with gluten-free diet(GFD) were at significantly increased risk of developing NAFLD (31), and that proton pump inhibitors and initial HOMA-IR may be risk factors for developing hepatic steatosis in this group of patients (32).
Previous studies found that CeD patients had a lower BMI than the general population at the time of diagnosis (33), and that this population was at a lower risk of metabolic syndromes such as T2D and hyperlipidemia before GFD treatment was administered (34–36). This may be related to the disruption of the intestinal barrier and relevant malabsorption induced by gluten consumption in CeD patients. NAFLD, on the other hand, is regarded as a fundamental hepatic manifestation of the metabolic syndrome (6), and The disease progression is associated with various risk factors, including obesity, hyperlipidemia, IR, and T2D (37).
The pathogenesis of CeD (38) and NAFLD (39, 40) are both correlated with gut microbiota. The spectrum of intestinal bacteria presented by the two diseases is seldom the same (41, 42), so we guess that the effects of intestinal flora disorders on organism metabolism are different tendencies in these two diseases. Most of the coexisting NAFLD in patients with CeD appear after GFD treatment (7), so the observed coexistence of CeD and NAFLD outcomes may be attributed to GFD treatment after CeD (7, 43). In recent years probiotics and other medications have also started to be utilized in most patients with intestinal diseases, and intestinal probiotic medications have also shown benefit in hepatic steatosis, the overall changes brought about by this new treatment still need further observed. In conclusion, the mechanisms of interaction between the two diseases still need to be further explored.
Our results did not show a causal association in CD-NAFLD, which is different from what we expected, but a previous MR study had similar findings (20). The risk of metabolic disease is lower in patients with CeD, which is the risk factor of NAFLD. The risk of metabolic disease is also low in IBD patients (44), but similar findings were not obtained in the causal association analysis of CD-NAFLD, either with or without adjustment for obesity and T2D. In clinical data, the prevalence of NAFLD in IBD patients varies 14.2%- 48% (8, 9, 45–47), and most studies had significantly higher prevalence compared to healthy controls, and we hypothesize that the treatment of IBD somehow influences the occurrence of subsequent NAFLD.
Most studies have shown that in IBD population, obesity, and diabetes remain risk factors for the development of NAFLD (9, 44, 46, 48), and a history of bowel resection, treatment of IBD with glucocorticoids, methotrexate, and azathioprine are associated with an increased incidence of NAFLD (8, 9, 44, 46), while the results of the anti-tnf-α therapy in patients with NAFLD are inconsistent (44, 49). In addition to known metabolic risk factors, treatment of IBD with glucocorticoids, azathioprine, methotrexate, and intestinal surgery seems to contribute to NAFLD. Notably, glucocorticoids and intestinal surgery may lead to hepatic steatosis or cholestasis (50), although without IBD. Therefore, we still cannot rule out a potential causal association of CD-NAFLD, and we still need to discuss the causal association of CD-NAFLD in larger sample-size GWAS studies and explore whether there are multiple factors whose pleiotropic effects counteract each other.
In our MR study, all three non-intestinal autoimmune diseases were associated with the presence of NAFLD, and the underlying mechanism of their interaction with NAFLD is presumed to remain closely related to the gut microbiota (51).
MS, idiopathic inflammatory demyelinating disease of the central nervous system (CNS), where damage to the blood-brain barrier is the initiating part of MS and is closely associated with subsequent demyelination, axonal injury, neuronal degeneration, and disease progression independent of relapse. Previous studies have indicated that gut microbiota can be involved in regulating the permeability of the blood-brain barrier, activating microglia expressing myelin-forming genes (52). The proportion of elevated intestinal permeability is significantly higher in MS patients than in healthy population (53), while previous studies have also suggested in animal models of MS that gut microbiota may regulate inflammatory cells migrate to the CNS by regulating blood-brain barrier integrity (54), thus we hypothesize that the MS-NAFLD association arises through the gut-brain axis and that molecular mimicry of gut microbiota may be a potential pathway of initiating autoimmune responses and the development of MS (55).
The mucosal origin hypothesis (56) suggested that the mucosa is the earliest site of RA-associated autoantibodies and that elevated levels of various types of RA-associated IgA in RA patients precede the onset of clinical arthritic symptoms (57, 58), while the homologous immune cells were found in the gut and joints of RA patients (59, 60), suggesting that gut-derived immunity in the preclinical phase is relevant to the pathogenesis of RA. The recent hypothesis of the gut-joint axis as a pathogenesis was then formulated, which mainly emphasizes the interaction between the mucosal immune system and the abnormal microbiota (61). Interestingly, gut dysbiosis has been shown to precede the onset of arthritis symptoms in both preclinical models and clinical samples and persist throughout the course of the disease (62), which seems to support a persistent role of gut microbiota in the disease.
T1D is characterized by pancreatic β-cell destruction leading to hyperglycemia and lifelong insulin reliance. The current view is that the presence of T1D exacerbates intrahepatic fat accumulation mainly through acquired insulin resistance (IR) (63). IR is a hit in the current multiple-hit theory of NAFLD (64), besides traditional factors such as sedentary, high-calorie diet, insulin dynamics in the portal circulation, genetic and epigenetic factors, and gut microbiota may contribute to hepatic and peripheral IR in T1D patients (65), leading to NAFLD.
Additionally, other factors may also contribute to NAFLD, such as oxidative stress, decreased physical activity, and drugs used such as corticosteroids. In NAFLD, intrahepatic lipid overload can activate multiple ROS-generating pathways leading to excessive production of oxidants, and high levels of ROS further affect oxidative modifications of cellular macromolecules (DNA, lipids, proteins, etc.), resulting in the accumulation of macromolecular damage and causing liver injury. Oxidative stress and lipid peroxidation are characteristic signs of NAFLD (66), while a common feature of autoimmune diseases such as MS, RA, and T1D is the increased production of ROS and RNS associated with the inflammatory response (67, 68). Oxidative stress can generate neoepitopes through oxidative modifications, and subsequently the organism produces autoantibodies corresponding to the neoepitopes (67), which could subsequently disrupt autoimmune tolerance. Furthermore, Motor system disorders resulting from different diseases such as MS and RA, which led to decreased physical activity, long-term use of corticosteroids in RA, and insulin use in T1D patients, can cause lipid metabolism disorders and weight gain in patient. Reduced physical activity itself is an important risk factor for NAFLD, and as mentioned above corticosteroids themselves may cause hepatic steatosis. Weight gain due to long-term insulin medication and IR itself are also involved in the development of NAFLD. Thus, the role of these factors either cannot be ignored.
The main strengths of our study include the elimination of potential confounders and reverse causal associations by MR analysis and control for heterogeneity and horizontal pleiotropy in the analysis. The study fulfilled the three major assumptions of MR, and the GWAS data related to outcomes were validated through known causal associations. However, there are several limitations: Firstly, all patients included in the GWAS data were Europeans, so it is possible that the results are not available for other ancestors, which means that our results need to be interpreted cautiously. Second, the data of outcomes are aggregated from several different sources and cases all identified through EHRs (electronic health records) (17), so the possibility of misclassification of NAFLD cannot be excluded, even if we validated the summary data. Furthermore, various statistical methods cannot exclude horizontal pleiotropy induced by biological mechanisms, so we should still be concerned about the impact of horizontal pleiotropy on study results, especially null causal association results. Finally, only several autoimmune diseases were analysed in this study due to the lack of support from epidemiological studies of diseases such as SLE and GD with NAFLD.
5 Conclusion
In conclusion, there is a considerable association between gene-driven autoimmune diseases and NAFLD, with CeD negatively associated with NAFLD and MS, RA, T1D positively associated with NAFLD. This result suggests that CeD itself may be a beneficial for NAFLD, suggesting that previous observational studies may be influenced by confounding factors, and patients with MS, RA, and T1D may have slightly higher morbidity of NAFLD than the healthy population, monitoring the prevalence of NAFLD in these populations is considerable. In addition, causal association between CD and NAFLD was ruled out.
Data availability statement
Publicly available datasets were analyzed in this study. This data can be found here: ebi-a-GCST005523, ieu-a-12, ebi-a-GCST005531, ieu-a-834, ebi-a-GCST010681, GCST90091033.
Ethics statement
The current study was based on summary-level GWAS data and each GWAS was approved by its corresponding ethical committee. All datasets can be downloaded freely without restriction.
Author contributions
MX, and TW contributed equally to this manuscript. All authors contributed to the article and approved the submitted version.
Funding
The authors declare financial support was received for the research, authorship, and/or publication of this article. This study was supported by the Health and Health Innovation Program of Jilin Province under Grant no. 2018J043.
Conflict of interest
The authors declare that the research was conducted in the absence of any commercial or financial relationships that could be construed as a potential conflict of interest.
Publisher’s note
All claims expressed in this article are solely those of the authors and do not necessarily represent those of their affiliated organizations, or those of the publisher, the editors and the reviewers. Any product that may be evaluated in this article, or claim that may be made by its manufacturer, is not guaranteed or endorsed by the publisher.
Supplementary material
The Supplementary Material for this article can be found online at: https://www.frontiersin.org/articles/10.3389/fimmu.2023.1229570/full#supplementary-material
Supplementary Figures | Scatterplots of the causal effect of autoimmune diseases (A: CeD, B: MS, C: RA, D: T1D as exposure) on NAFLD in adjusted MR. Analyses were conducted by IVW, IVW-fixed effects, weighted median, MR-Egger, Weighted mode and Simple mode methods. IVW, inverse variance weighted; MR, Mendelian randomization; MR-Egger, MR-Egger regression; NAFLD, non-alcoholic fatty liver disease.
Abbreviations
NAFLD, Non-alcoholic fatty liver disease; MR, Mendelian randomization; GWAS, Genome-wide association study; CeD, Celiac disease; CD, Crohn’s disease; MS, multiple sclerosis; RA, rheumatoid arthritis; T1D, type 1 diabetes; IVs, Instrument variables; T2D, type 2 diabetes; SLE, systemic lupus erythematosus; GD, Graves’ disease; IBD,inflammatory bowel disease; SNP, Single Nucleotide Polymorphism; BMI, body mass index; GFD, gluten-free diet; IR, insulin resistance; ROS, reactive oxygen species; RNS, reactive nitrogen species.
References
1. Cotter TG, Rinella M. Nonalcoholic fatty liver disease 2020: the state of the disease. Gastroenterology (2020) 158(7):1851–64. doi: 10.1053/j.gastro.2020.01.052
2. Roden M. Mechanisms of Disease: hepatic steatosis in type 2 diabetes–pathogenesis and clinical relevance. Nat Clin Pract Endocrinol Metab (2006) 2(6):335–48. doi: 10.1038/ncpendmet0190
3. Powell EE, Wong VW, Rinella M. Non-alcoholic fatty liver disease. Lancet (2021) 397(10290):2212–24. doi: 10.1016/S0140-6736(20)32511-3
4. Xiao ZX, Miller JS, Zheng SG. An updated advance of autoantibodies in autoimmune diseases. Autoimmun Rev (2021) 20(2):102743. doi: 10.1016/j.autrev.2020.102743
5. Mané-Damas M, Hoffmann C, Zong S, Tan A, Molenaar PC, Losen M, et al. Autoimmunity in psychotic disorders. Where we stand, challenges and opportunities. Autoimmun Rev (2019) 18(9):102348. doi: 10.1016/j.autrev.2019.102348
6. Valvano M, Longo S, Stefanelli G, Frieri G, Viscido A, Latella G. Celiac disease, gluten-free diet, and metabolic and liver disorders. Nutrients (2020) 12(4):940. doi: 10.3390/nu12040940
7. Reilly NR, Lebwohl B, Hultcrantz R, Green PH, Ludvigsson JF. Increased risk of non-alcoholic fatty liver disease after diagnosis of celiac disease. J Hepatol (2015) 62(6):1405–11. doi: 10.1016/j.jhep.2015.01.013
8. Hoffmann P, Jung V, Behnisch R, Gauss A. Prevalence and risk factors of nonalcoholic fatty liver disease in patients with inflammatory bowel diseases: A cross-sectional and longitudinal analysis. World J Gastroenterol (2020) 26(46):7367–81. doi: 10.3748/wjg.v26.i46.7367
9. Zou ZY, Shen B, Fan JG. Systematic review with meta-analysis: epidemiology of nonalcoholic fatty liver disease in patients with inflammatory bowel disease. Inflammation Bowel Dis (2019) 25(11):1764–72. doi: 10.1093/ibd/izz043
10. de Vries M, Westerink J, Kaasjager K, de Valk HW. Prevalence of nonalcoholic fatty liver disease (NAFLD) in patients with type 1 diabetes mellitus: A systematic review and meta-analysis. J Clin Endocrinol Metab (2020) 105(12):3842–53. doi: 10.1210/clinem/dgaa575
11. Emdin CA, Khera AV, Kathiresan S. Mendelian randomization. Jama (2017) 318(19):1925–6. doi: 10.1001/jama.2017.17219
12. Trynka G, Hunt KA, Bockett NA, ROmanos J, Mistry V, Szperl A, et al. Dense genotyping identifies and localizes multiple common and rare variant association signals in celiac disease. Nat Genet (2011) 43(12):1193–201. doi: 10.1038/ng.998
13. Liu JZ, van Sommeren S, Huang H, Ng SC, Alberts R, Takahashi A, et al. Association analyses identify 38 susceptibility loci for inflammatory bowel disease and highlight shared genetic risk across populations. Nat Genet (2015) 47(9):979–86. doi: 10.1038/ng.3359
14. Beecham AH, Patsopoulos NA, Xifara DK, Davis MF, Kemppinen A, Cotsapas C, et al. Analysis of immune-related loci identifies 48 new susceptibility variants for multiple sclerosis. Nat Genet (2013) 45(11):1353–60. doi: 10.1038/ng.2770
15. Stahl EA, Raychaudhuri S, Remmers EF, Xie G, Eyre S, Thomson BP, et al. Genome-wide association study meta-analysis identifies seven new rheumatoid arthritis risk loci. Nat Genet (2010) 42(6):508–14. doi: 10.1038/ng.582
16. Forgetta V, Manousaki D, Istomine R, Ross S, Tessier MC, Marchand L, et al. Rare genetic variants of large effect influence risk of type 1 diabetes. Diabetes (2020) 69(4):784–95. doi: 10.2337/db19-0831
17. Ghodsian N, Abner E, Emdin CA, Gobeil É, Taba N, Haas ME, et al. Electronic health record-based genome-wide meta-analysis provides insights on the genetic architecture of non-alcoholic fatty liver disease. Cell Rep Med (2021) 2(11):100437. doi: 10.1016/j.xcrm.2021.100437
18. Liu Y, Basty N, Whitcher B, Bell JD, Sorokin EP, van Bruggen N, et al. Genetic architecture of 11 organ traits derived from abdominal MRI using deep learning. Elife (2021) 10:e65554. doi: 10.7554/eLife.65554.sa2
19. Bowden J, Holmes MV. Meta-analysis and Mendelian randomization: A review. Res Synth Methods (2019) 10(4):486–96. doi: 10.1002/jrsm.1346
20. Chen L, Fan Z, Sun X, Qiu W, Chen Y, Zhou J, et al. Mendelian randomization rules out causation between inflammatory bowel disease and non-alcoholic fatty liver disease. Front Pharmacol (2022) 13:891410. doi: 10.3389/fphar.2022.891410
21. Liu Z, Zhang Y, Graham S, Wang X, Cai D, Huang M, et al. Causal relationships between NAFLD, T2D and obesity have implications for disease subphenotyping. J Hepatol (2020) 73(2):263–76. doi: 10.1016/j.jhep.2020.03.006
22. Xue A, Wu Y, Zhu Z, Zhang F, Kemper KE, Zheng Z, et al. Genome-wide association analyses identify 143 risk variants and putative regulatory mechanisms for type 2 diabetes. Nat Commun (2018) 9(1):2941. doi: 10.1038/s41467-018-04951-w
23. Hemani G, Zheng J, Elsworth B, Wade KH, Haberland V, Baird D, et al. The MR-Base platform supports systematic causal inference across the human phenome. Elife (2018) 7:e34408. doi: 10.7554/eLife.34408
24. Bowden J, Davey Smith G, Haycock PC, Burgess S. Consistent estimation in mendelian randomization with some invalid instruments using a weighted median estimator. Genet Epidemiol. (2016) 40(4):304–14. doi: 10.1002/gepi.21965
25. Bowden J, Davey Smith G, Burgess S. Mendelian randomization with invalid instruments: effect estimation and bias detection through Egger regression. Int J Epidemiol. (2015) 44(2):512–25. doi: 10.1093/ije/dyv080
26. Hartwig FP, Davey Smith G, Bowden J. Robust inference in summary data Mendelian randomization via the zero modal pleiotropy assumption. Int J Epidemiol. (2017) 46(6):1985–98. doi: 10.1093/ije/dyx102
27. Bowden J, Spiller W, Del Greco MF, Sheehan N, Thompson J, Minelli C, et al. Improving the visualization, interpretation and analysis of two-sample summary data Mendelian randomization via the Radial plot and Radial regression. Int J Epidemiol. (2018) 47(6):2100. doi: 10.1093/ije/dyy265
28. Burgess S, Thompson SG. Interpreting findings from Mendelian randomization using the MR-Egger method. Eur J Epidemiol. (2017) 32(5):377–89. doi: 10.1007/s10654-017-0255-x
29. Verbanck M, Chen CY, Neale B, Do R. Detection of widespread horizontal pleiotropy in causal relationships inferred from Mendelian randomization between complex traits and diseases. Nat Genet (2018) 50(5):693–8. doi: 10.1038/s41588-018-0099-7
30. Hoffmanova I, Sanchez D, Tuckova L, Tlaskalova-Hogenova H. Celiac disease and liver disorders: from putative pathogenesis to clinical implications. Nutrients (2018) 10(7):892. doi: 10.3390/nu10070892
31. Tovoli F, Negrini G, Farì R, Guidetti E, Faggiano C, Napoli L, et al. Increased risk of nonalcoholic fatty liver disease in patients with coeliac disease on a gluten-free diet: beyond traditional metabolic factors. Aliment Pharmacol Ther (2018) 48(5):538–46. doi: 10.1111/apt.14910
32. Imperatore N, Tortora R, Testa A, Gerbino N, Caporaso N, Rispo A. Proton pump inhibitors as risk factor for metabolic syndrome and hepatic steatosis in coeliac disease patients on gluten-free diet. J Gastroenterol (2018) 53(4):507–16. doi: 10.1007/s00535-017-1381-7
33. Newnham ED. Coeliac disease in the 21st century: paradigm shifts in the modern age. J Gastroenterol Hepatol (2017) 32(Suppl 1):82–5. doi: 10.1111/jgh.13704
34. Bakhshipour A, Kaykhaei MA, Moulaei N, Mashhadi MA. Prevalence of coeliac disease in patients with non-alcoholic fatty liver disease. Arab J Gastroenterol (2013) 14(3):113–5. doi: 10.1016/j.ajg.2013.08.001
35. Kabbani TA, Kelly CP, Betensky RA, Hansen J, Pallav K, Villafuerte-Gálvez JA, et al. Patients with celiac disease have a lower prevalence of non-insulin-dependent diabetes mellitus and metabolic syndrome. Gastroenterology (2013) 144(5):912–7.e1. doi: 10.1053/j.gastro.2013.01.033
36. West J, Logan RF, Card TR, Smith C, Hubbard R. Risk of vascular disease in adults with diagnosed coeliac disease: a population-based study. Aliment Pharmacol Ther (2004) 20(1):73–9. doi: 10.1111/j.1365-2036.2004.02008.x
37. Eslam M, Sanyal AJ, George J, International Consensus P. MAFLD: A consensus-driven proposed nomenclature for metabolic associated fatty liver disease. Gastroenterology (2020) 158(7):1999–2014.e1. doi: 10.1053/j.gastro.2019.11.312
38. Verdu EF, Galipeau HJ, Jabri B. Novel players in coeliac disease pathogenesis: role of the gut microbiota. Nat Rev Gastroenterol Hepatol (2015) 12(9):497–506. doi: 10.1038/nrgastro.2015.90
39. Chen J, Vitetta L. Bile acids and butyrate in the effects of probiotics/synbiotics on nonalcoholic fatty liver disease. Eur J Gastroenterol Hepatol (2019) 31(11):1475–6. doi: 10.1097/MEG.0000000000001506
40. Chen J, Vitetta L. Gut microbiota metabolites in NAFLD pathogenesis and therapeutic implications. Int J Mol Sci (2020) 21(15):5214. doi: 10.3390/ijms21155214
41. Chibbar R, Dieleman LA. The gut microbiota in celiac disease and probiotics. Nutrients (2019) 11(10):2375. doi: 10.3390/nu11102375
42. Aron-Wisnewsky J, Vigliotti C, Witjes J, Le P, Holleboom AG, Verheij J, et al. Gut microbiota and human NAFLD: disentangling microbial signatures from metabolic disorders. Nat Rev Gastroenterol Hepatol (2020) 17(5):279–97. doi: 10.1038/s41575-020-0269-9
43. Abenavoli L, Milic N, De Lorenzo A, Luzza F. A pathogenetic link between non-alcoholic fatty liver disease and celiac disease. Endocrine (2013) 43(1):65–7. doi: 10.1007/s12020-012-9731-y
44. Sourianarayanane A, Garg G, Smith TH, Butt MI, McCullough AJ, Shen B. Risk factors of non-alcoholic fatty liver disease in patients with inflammatory bowel disease. J Crohns Colitis. (2013) 7(8):e279–85. doi: 10.1016/j.crohns.2012.10.015
45. Fousekis FS, Katsanos KH, Theopistos VI, Baltayiannis G, Kosmidou M, Glantzounis G, et al. Hepatobiliary and pancreatic manifestations in inflammatory bowel diseases: a referral center study. BMC Gastroenterol (2019) 19(1):48. doi: 10.1186/s12876-019-0967-3
46. Lin A, Roth H, Anyane-Yeboa A, Rubin DT, Paul S. Prevalence of nonalcoholic fatty liver disease in patients with inflammatory bowel disease: A systematic review and meta-analysis. Inflammation Bowel Dis (2021) 27(6):947–55. doi: 10.1093/ibd/izaa189
47. Zamani M, Alizadeh-Tabari S, Singh S, Loomba R. Meta-analysis: prevalence of, and risk factors for, non-alcoholic fatty liver disease in patients with inflammatory bowel disease. Aliment Pharmacol Ther (2022) 55(8):894–907. doi: 10.1111/apt.16879
48. Principi M, Iannone A, Losurdo G, Mangia M, Shahini E, Albano F, et al. Nonalcoholic fatty liver disease in inflammatory bowel disease: prevalence and risk factors. Inflammation Bowel Dis (2018) 24(7):1589–96. doi: 10.1093/ibd/izy051
49. Likhitsup A, Dundulis J, Ansari S, Patibandla S, Hutton C, Kennedy K, et al. High prevalence of non-alcoholic fatty liver disease in patients with inflammatory bowel disease receiving anti-tumor necrosis factor therapy. Ann Gastroenterol (2019) 32(5):463–8. doi: 10.20524/aog.2019.0405
50. Sasdelli AS, Agostini F, Pazzeschi C, Guidetti M, Lal S, Pironi L. Assessment of Intestinal Failure Associated Liver Disease according to different diagnostic criteria. Clin Nutr (2019) 38(3):1198–205. doi: 10.1016/j.clnu.2018.04.019
51. Uchiyama K, Naito Y, Takagi T. Intestinal microbiome as a novel therapeutic target for local and systemic inflammation. Pharmacol Ther (2019) 199:164–72. doi: 10.1016/j.pharmthera.2019.03.006
52. Mirza A, Mao-Draayer Y. The gut microbiome and microbial translocation in multiple sclerosis. Clin Immunol (2017) 183:213–24. doi: 10.1016/j.clim.2017.03.001
53. Buscarinu MC, Cerasoli B, Annibali V, Policano C, Lionetto L, Capi M, et al. Altered intestinal permeability in patients with relapsing-remitting multiple sclerosis: A pilot study. Mult Scler. (2017) 23(3):442–6. doi: 10.1177/1352458516652498
54. Mangalam A, Shahi SK, Luckey D, Karau M, Marietta E, Luo N, et al. Human gut-derived commensal bacteria suppress CNS inflammatory and demyelinating disease. Cell Rep (2017) 20(6):1269–77. doi: 10.1016/j.celrep.2017.07.031
55. Wang X, Liang Z, Wang S, Ma D, Zhu M, Feng J. Role of gut microbiota in multiple sclerosis and potential therapeutic implications. Curr Neuropharmacol. (2022) 20(7):1413–26. doi: 10.2174/1570159X19666210629145351
56. Holers VM, Demoruelle MK, Kuhn KA, Buckner JH, Robinson WH, Okamoto Y, et al. Rheumatoid arthritis and the mucosal origins hypothesis: protection turns to destruction. Nat Rev Rheumatol (2018) 14(9):542–57. doi: 10.1038/s41584-018-0070-0
57. Rantapää-Dahlqvist S, de Jong BA, Berglin E, Hallmans G, Wadell G, Stenlund H, et al. Antibodies against cyclic citrullinated peptide and IgA rheumatoid factor predict the development of rheumatoid arthritis. Arthritis Rheumatol (2003) 48(10):2741–9. doi: 10.1002/art.11223
58. Shi J, van de Stadt LA, Levarht EW, Huizinga TW, Hamann D, van Schaardenburg D, et al. Anti-carbamylated protein (anti-CarP) antibodies precede the onset of rheumatoid arthritis. Ann Rheum Dis (2014) 73(4):780–3. doi: 10.1136/annrheumdis-2013-204154
59. May E, Märker-Hermann E, Wittig BM, Zeitz M, Meyer zum Büschenfelde KH, Duchmann R. Identical T-cell expansions in the colon mucosa and the synovium of a patient with enterogenic spondyloarthropathy. Gastroenterology (2000) 119(6):1745–55. doi: 10.1053/gast.2000.20173
60. Trollmo C, Verdrengh M, Tarkowski A. Fasting enhances mucosal antigen specific B cell responses in rheumatoid arthritis. Ann Rheum Dis (1997) 56(2):130–4. doi: 10.1136/ard.56.2.130
61. Zaiss MM, Joyce Wu HJ, Mauro D, Schett G, Ciccia F. The gut-joint axis in rheumatoid arthritis. Nat Rev Rheumatol (2021) 17(4):224–37. doi: 10.1038/s41584-021-00585-3
62. Jubair WK, Hendrickson JD, Severs EL, Schulz HM, Adhikari S, Ir D, et al. Modulation of inflammatory arthritis in mice by gut microbiota through mucosal inflammation and autoantibody generation. Arthritis Rheumatol (2018) 70(8):1220–33. doi: 10.1002/art.40490
63. Memaj P, Jornayvaz FR. Non-alcoholic fatty liver disease in type 1 diabetes: Prevalence and pathophysiology. Front Endocrinol (Lausanne). (2022) 13:1031633. doi: 10.3389/fendo.2022.1031633
64. Buzzetti E, Pinzani M, Tsochatzis EA. The multiple-hit pathogenesis of non-alcoholic fatty liver disease (NAFLD). Metabolism (2016) 65(8):1038–48. doi: 10.1016/j.metabol.2015.12.012
65. Mertens J, Van Gaal LF, Francque SM, De Block C. NAFLD in type 1 diabetes: overrated or underappreciated? Ther Adv Endocrinol Metab (2021) 12:20420188211055557. doi: 10.1177/20420188211055557
66. Bellanti F, Villani R, Facciorusso A, Vendemiale G, Serviddio G. Lipid oxidation products in the pathogenesis of non-alcoholic steatohepatitis. Free Radic Biol Med (2017) 111:173–85. doi: 10.1016/j.freeradbiomed.2017.01.023
67. Smallwood MJ, Nissim A, Knight AR, Whiteman M, Haigh R, Winyard PG. Oxidative stress in autoimmune rheumatic diseases. Free Radic Biol Med (2018) 125:3–14. doi: 10.1016/j.freeradbiomed.2018.05.086
Keywords: Mendelian randomization (MR), autoimmune disease, non-alcoholic fatty liver disease (NAFLD), causality, gut microbiota, risk factor
Citation: Xu M, Wu T, Li Z and Xin G (2023) Influence of genetically predicted autoimmune diseases on NAFLD. Front. Immunol. 14:1229570. doi: 10.3389/fimmu.2023.1229570
Received: 26 May 2023; Accepted: 23 August 2023;
Published: 11 September 2023.
Edited by:
Marcos Lopez-Hoyos, Marqués de Valdecilla Health Research Institute (IDIVAL), SpainReviewed by:
Daniele Sola, University of Eastern Piedmont, ItalyNikolaos Gatselis, Autoimmune Liver Diseases General University Hospital of Larissa, Greece
Copyright © 2023 Xu, Wu, Li and Xin. This is an open-access article distributed under the terms of the Creative Commons Attribution License (CC BY). The use, distribution or reproduction in other forums is permitted, provided the original author(s) and the copyright owner(s) are credited and that the original publication in this journal is cited, in accordance with accepted academic practice. No use, distribution or reproduction is permitted which does not comply with these terms.
*Correspondence: Guijie Xin, xingj@jlu.edu.cn
†These authors have contributed equally to this work
‡ORCID: Min Xu, orcid.org/0009-0009-8247-2418
Tong Wu, orcid.org/0000-0003-1203-0365
Zhaoxia Li, orcid.org/0000-0001-6561-1302
Guijie Xin, orcid.org/0000-0002-0868-0317