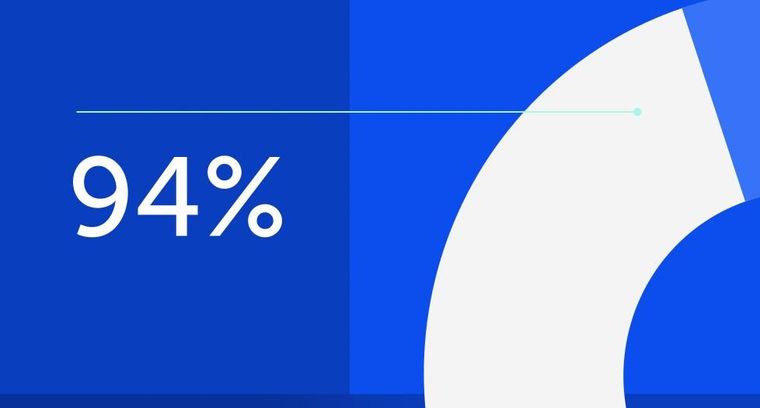
94% of researchers rate our articles as excellent or good
Learn more about the work of our research integrity team to safeguard the quality of each article we publish.
Find out more
ORIGINAL RESEARCH article
Front. Immunol., 14 July 2023
Sec. Autoimmune and Autoinflammatory Disorders : Autoimmune Disorders
Volume 14 - 2023 | https://doi.org/10.3389/fimmu.2023.1205616
Introduction: Assessing labial salivary gland exocrinopathy is a cornerstone in primary Sjögren’s syndrome. Currently this relies on the histopathologic diagnosis of focal lymphocytic sialadenitis and computing a focus score by counting lym=phocyte foci. However, those lesions represent advanced stages of primary Sjögren’s syndrome, although earlier recognition of primary Sjögren’s syndrome and its effective treatment could prevent irreversible damage to labial salivary gland. This study aimed at finding early biomarkers of primary Sjögren’s syndrome in labial salivary gland combining metabolomics and machine-learning approaches.
Methods: We used a standardized targeted metabolomic approach involving high performance liquid chromatography coupled with mass spectrometry among newly diagnosed primary Sjögren’s syndrome (n=40) and non- primary Sjögren’s syndrome sicca (n=40) participants in a prospective cohort. A metabolic signature predictive of primary Sjögren’s syndrome status was explored using linear (logistic regression with elastic-net regularization) and non-linear (random forests) machine learning architectures, after splitting the data set into training, validation, and test sets.
Results: Among 126 metabolites accurately measured, we identified a discriminant signature composed of six metabolites with robust performances (ROC-AUC = 0.86) for predicting primary Sjögren’s syndrome status. This signature included the well-known immune-metabolite kynurenine and five phospholipids (LysoPC C28:0; PCaa C26:0; PCaaC30:2; PCae C30:1, and PCaeC30:2). It was split into two main components: the first including the phospholipids was related to the intensity of lymphocytic infiltrates in salivary glands, while the second represented by kynurenine was independently associated with the presence of anti-SSA antibodies in participant serum.
Conclusion: Our results reveal an immuno-lipidomic signature in labial salivary gland that accurately distinguishes early primary Sjögren’s syndrome from other causes of sicca symptoms.
Primary Sjögren’s syndrome (pSS) is an autoimmune disease characterized by the lymphocytic infiltration of exocrine glands (1). The demonstration of this exocrinopathy and its consequences on tear and saliva productions constitute most of the current items of the 2016 ACR/EULAR (American College of Rheumatology/European League against Rheumatisms) classification criteria (2). The evidence of exocrine glands involvement is based on a labial salivary glands (LSG) biopsy and the presence of focal lymphocytic sialadenitis (FLS) with calculation of the focus score (FS; number of clusters or foci containing ≥ 50 lymphocytes per 4 mm² of parenchyma) (3). LSG have the advantage of easy access compared to other exocrine glands and have been shown to be representative of major salivary glands (4). This identification of histological lesions is of major interest in patients without anti-SSA antibodies in order to certify the autoimmune character of the exocrinopathy. The LSG biopsy has good performances for pSS classification with sensitivity ranging from 79% to 91.4% and a specificity ranging from 81.8% to 100% (5–7). It also has a prognostic value with the presence of germinal centers and some lymphoid subpopulations being associated with systemic manifestations and a higher occurrence of lymphoma (8).
However, the diagnosis of FLS (with calculation of FS) does not take into account other lesions such as acinar atrophy, epithelial cells proliferation, or fibrosis (9), and is not appropriate for small focal infiltrates (10). Indeed, the LSG biopsy highlights the late histological consequences of exocrinopathy as the unstimulated salivary flow rate does for salivary function with a threshold of 0.1 mL/min (7). This contributes to the long delay in diagnosis often experienced by pSS patients (11). Consequently, there is a crucial need for better characterization of early stages of exocrine gland involvement to enable earlier diagnosis (12). In addition to various immune cells infiltrates (13), many chemokines in LSG have been associated with pSS status [chemokine C-C ligand 19 (CCL19) (14), CCL21 (14), CCL25 (15), chemokine ligand receptor (CCR7) (14), chemokine C-X-C ligand 1 (CXCL1) (16), CXCL9 (17), CXCL10 (15, 17), CXCL12 (14), CXCL13 (14), chemokine C-X-C ligand receptor 4 (CXCR4) (14), CXCR5 (14), CXCR1 (16), CXCR3 (17), lymphotoxins (LT) alpha and beta (14), Thymic Stromal Lymphopoietin (18)] and notably those associated with B lymphocytes development (19). However, few results have been reproduced except for BAFF (14, 20), CCL21 (14), and CXCL13 (14). In their study of salivary gland secretomes, Blokland et al. were able to correctly (95.8%) distinguish pSS from non-pSS sicca patients based on four chemokines, including CXCL13 (21). However, such parameters mainly focus on immune cells while the role of epithelial cells, which are of great importance in pSS pathogenesis (22), is poorly reflected. Omics approaches are unbiased and have opened new horizons in the study of SS pathogenesis. Studies based on RNA-Sequencing on LSG in pSS are scarce. However, two such studies have already highlighted the main role of the Inducible T cell costimulatory (ICOS) pathway (23). Proteomic studies on LSG essentially confirmed the implication of some already known proteins in pSS such as beta-2-microglobulin, lactoferrin, immunoglobulin kappa light chain, polymeric Ig receptor, lysozyme C and cystatin C, amylase and carbonic anhydrase VI (24), alpha-defensine-1 and calmoduline (25). However, some of these proteomic studies were unable to highlight any differences between samples from pSS and from non-pSS sicca patients (26).
Clinical metabolomics consist in measuring the concentration of a large number of metabolites in biological fluids or tissues and then modeling their predictive power for a pathological condition compared to a control group. Using this strategy, we have recently identified a metabolomic signature predictive of pSS in tears (27). To our knowledge, no study to date reported a metabolomic approach performed in LSG from pSS patients.
The present study aimed at i) identifying a metabolomic signature within LSG from participants with newly diagnosed pSS, compared to those presenting another non-immune cause of sicca symptoms; and ii) evaluating the variation in this metabolic signature with respect to age, sex, immunological status, and dryness severity.
The METABOGREN study was approved by the Ethical Committees of Angers University Hospital (CPP DC2014-2224, AC2017-2993, bioethics n°2018/42) and was conducted in compliance with the declaration of Helsinki. All participants signed a written informed consent.
We included all incident patients with an objective measure of eye and/or mouth dryness between May 2017 and May 2018 in this study cohort, as previously described (27). Objective eye dryness was defined with a Schirmer I test ≤ 5 mm/5 min on at least one eye and/or an Ocular Staining Score (OSS) ≥ 3 and/or a break-up time (BUT) test < 10 seconds on at least one eye. Objective mouth dryness was defined as an unstimulated saliva flow (UWS) ≤ 1.5 mL/15 minutes. We assess all patients prospectively with respect to whether or not they met classification criteria for SS using the 2016 ACR-EULAR criteria. Cases were defined as those who met the 2016 ACR/EULAR criteria (2). Controls did not meet the 2016 ACR/EULAR criteria, had no anti-SSA or anti-SSB antibody, and had a LSG biopsy negative for FLS. Participants were enrolled using a consecutive sampling strategy until we had two groups with 40 participants each.
We excluded patients with a previous diagnosis of pSS and as follows: history of labial surgery; history of ocular surgery; wearers of contact lenses and scleral contact lenses; other systemic autoimmune disease (systemic lupus erythematosus, antiphospholipid syndrome, rheumatoid arthritis, immune-mediated myopathy, systemic scleroderma, mixed connective tissue disease, eosinophilic fasciitis, sarcoidosis, IgG4-related disease, ANCA-associated vasculitis, periarteritis nodosa, IgA vasculitis, Cogan’s syndrome, Behçet disease, Still disease); inflammatory eye disease (uveitis, ocular pemphigoid); graft versus host disease; solid cancer and/or lymphoma/leukemia without remission at least for 2 years except for MALT-associated B lymphoma of parotid; severe or non-controlled arterial hypertension; chronic heart failure, defined as left ventricular ejection fraction ≤ 30%; non-controlled diabetes defined as glycated hemoglobin ≥ 8% or diabetes with microangiopathy; chronic respiratory disease necessitating O2 support; chronic kidney failure defined as creatinine clearance with MDRD (Modified Diet for Renal Disease) formula ≤ 30 ml/min/1.73 m²; known mitochondria disease or metabolic genetic disorder; psychotic disorder or unstable psychiatric disease; use of systemic immunosuppressor or immunomodulatory drugs; use of systemic corticosteroids; and use of eye drops, with the exception of artificial tears. Pregnant women and patients unable to give informed consent were also excluded.
Participants were instructed to discontinue using any artificial saliva or sialogogues 48 h before the biospecimen collection. All the following tests were performed in a standardized manner by the same operator: Schirmer I test (GECIS, Neung sur Beuvron, France), OSS for the lacrimal function, and UWS flow rate for the saliva production, were performed as previously described (7, 27). In addition, we collected the following data: age, sex, tobacco use, use of anticholinergic drugs (28), presence of autoantibodies (antinuclear antibodies, anti-SSA and anti-SSB antibodies, rheumatoid factors), presence of hypergammoglobulinemia (defined as gammoglobulin level over 1600 mg/dL), presence of cryoglobulinemia, and presence of extraglandular involvement of pSS (29).
Salivary glands were removed from the inferior labial mucosa in front of the canine teeth after local anesthesia with xylocaine. At least two glands were immediately placed in Eppendorf® tubes (Eppendorf, Hamburg, Germany) into liquid nitrogen for transfer, and then stored at −80°C. Other glands were fixed in 4% paraformaldehyde, later transferred to 70% ethanol, then embedded in paraffin, and finally cut into 3 μm sections for histological staining. To determine the degree of lymphocytic infiltration, we used the focus score (FS < 1, FS = 1, FS > 1) and the staging according to Chisholm and Mason classification, as follows: no infiltrate (0), slight infiltrate (1), moderate infiltrate and FS < 1 (2), one focus (3) and more than one focus (4) (3, 30). The presence of granuloma and amyloid deposits was also systematically evaluated.
The cryopreserved LSG were firstly weighted for solvents normalization and then transferred in precooled 2.0 ml homogenization Precellys tubes (Bertin Technologies, Montigny-le-Bretonneux, France) prefilled with 1.4 mm diameter ceramic beads and microfiltered water. The glands were homogenized by two grinding cycles, each at 6500 rpm for 30 sec, spaced 20 sec apart, using a Precellys® homogenizer kept at +4°C. The homogenate was centrifuged at 12,000 g for 5 min and 5 µL were separately collected for later protein quantification. Cold methanol (3 µL/mg) was added into the homogenate, followed by a new grinding cycle at 6500 rpm for 30 sec. Then, the supernatant was recovered after centrifuging the homogenate at 20,000 g for 10 min and kept at -80°C until metabolomic analysis (27).
We applied a targeted, quantitative metabolomic approach to the tear extracts using the Biocrates® AbsoluteIDQ p180 kit (Biocrates Life Sciences AG, Innsbruck, Austria) and an AB Sciex QTRAP 5500 (Life Sciences Holdings, Villebon-sur-Yvette, France) mass spectrometer (MS) as previously described (27) (Figure 1A). More details about the metabolomic kit, the MS and liquid chromatography (LC) parameters are available in Supplementary Material. All analyses were performed with the same kit to avoid batch effect.
Figure 1 Analytical (A) and Statistical (B) workflow of the study. FIA, flow injection analysis; LC, liquid chromatography; MS, mass spectrometry; MS/MS, tandem mass spectrometry.
A cleaning step was performed to filter out unusable data: metabolites with more than 50% of values lying outside the quantification detection limits were removed from the datasets. For all potentially withdrawn features, a Chi-squared test for independence was performed between the number of values within bounds and out-of-bounds in pSS and non-pSS Sicca patients, to prevent removal of discriminating features, i.e. features with a significant difference between the 2 groups (31). Metabolite concentrations were normalized with protein concentration.
Continuous variables were summarized using medians, and 25th and 75th percentiles, and categorical variables with absolute values and percentages. For univariate analyses, we used Student t-test or Mann-Whitney test as appropriate for continuous variables, and Chi-squared test or Fisher’s test as appropriate for categorical variables. The type I error was 5%. Image manipulation and formatting were performed using GNU Image Manipulation Program (GIMP) software (version 2.10). The analyses were carried out using R software (version 4.0.1, R-project-org, Vienna, Austria) and SPSS (v23.0, IBM Corp, NY, USA). R packages are listed hereafter: FactoMineR for principal component analysis (PCA) (32); Rpart for stratified partitioning (33); glmnet for logistic regression with or without regularization (34) based on λ with 1 standard error (SE); randomForest (35); and pROC to evaluate the area under curves for receiver operating characteristic curves - area under the curve (ROC-AUC) (36).
Full statistical workflow has been previously detailed (27) and is available in Supplementary Material. In brief, we randomly partitioned the study population into three data subsets with stratification on case-control status: training set (n = 52), validation set (n = 10) and test set (n = 18) (Figure 1B). To identify the best predictive model, we carried out a feature selection using elastic-net regularized logistic regression and we compared ROC-AUC from linear (elastic-net regularized logistic regression) and non-linear (random forests) machine learning architectures in the training and validation sets. The model with the highest performance was finally evaluated on the test set to determine the metabolomic signature. The main components of this signature were extracted by means of PCA and the influences of main confounders [age, sex, presence of anti-SSA antibody, tobacco use, use of anticholinergic drugs, rate of UWS test, and presence of lymphocytic sialadenitis and its grade (3)] were tested on them using linear regression.
Among 100 patients explored for sicca symptoms, 90 were included in the cohort. The study population was composed of 40 patients who were found to meet classification criteria for pSS, and among whom 20 had anti-SSA antibodies, and 40 non-pSS Sicca patients randomly selected from the 50 remaining patients who met inclusion criteria as controls. Median age for the whole studied population was 60.5 [46.8-67] years with sex ratio (W/M) of 7.9. General characteristics are summarized in Table 1. The LSG weight and protein concentration did not differ between the two groups.
Table 1 General and immunological characteristics of the two groups, composed of pSS patients and non-pSS sicca patients.
Among the 188 measured metabolites, 62 were excluded as they were beyond the limits of detection. Unsupervised analysis did not reveal any spontaneous clustering nor outliers on principal component analysis. For the feature selection among the 126 metabolites, the logistic regression with elastic-net regularization isolated eight models with the best AUC (0.80) and, among them, seven highly consistent models (alpha coefficients: 0.10; 0.11; 0.15; 0.17; 0.21; 0.22; 0.37) with the same six metabolites of interest and one model (alpha = 0.09) with solely five metabolites but all included in the list of metabolites present in the seven other models. We selected the models including six metabolites, one biogenic amine and five phospholipids: kynurenine; lysophosphatidylcholines (LysoPC) C28:0; phoshatidylcholine diacyl (PCaa) C26:0; PCaaC30:2; phoshatidylcholine acyl alkyl (PCae) C30:1; and PCaeC30:2.
Subsequently, we sought the best learning architectures between linear and non-linear ones on the validation set. With the six previously selected metabolites, logistic regression yielded 23 models with AUCs of 0.84, homogenous with the prediction obtained on the training set (AUC of 0.80). The random forest disclosed AUCs of 0.72 whether over the six selected metabolites or the 126 metabolites, with a very large gap in comparison to the AUC of 1.00 obtained with random forest on the training set. Thus, we selected as the final model, the logistic regression with the lowest alpha value (alpha 0.30) to retain the maximum information among the metabolites of interest. The final prediction on the test set provided an AUC of 0.86. In the final signature, kynurenine was the sole metabolite with increased concentration in the pSS group compared to the non-pSS sicca group while all five phospholipids had decreased concentration.
Dimensional reduction of the metabolomic signature revealed that the two first principal components (PC) explained 91.1% of total variance (PC1 74.3%, PC2 15.8%). The distribution of metabolites over these two PC was clearly cut: the five phospholipids were mainly distributed in PC1 whereas the variance of kynurenine expressed itself in PC2 (Figure 2). Either gland weight or protein concentration were not significantly associated with the two main PCs: gland weight (PC1: p = 0.50; PC2: p = 0.55) and protein concentration (PC1: p = 0.70; PC2: p = 0.72) using linear regression models.
Figure 2 Distribution of the metabolites from the metabolomic signature in the two main components. the two main components were extracted after dimensional reduction with principal component analysis. LysoPC, lysophoshatidylcholine; PC, principal component; PCaa, phoshatidylcholine diacyl; PCae, phoshatidylcholine acyl alkyl.
The association between the metabolomic signature and the pSS status was not altered by age, sex, tobacco use, use of anticholinergic drugs, and UWS flow rate in linear regression models analyzing the two first principal components (Table 2). The inclusion in these models of anti-SSA antibodies affected the significant association between the pSS status and the metabolomics signature for PC2 but not for PC1 (model 2). However, this association was disrupted for both PC1 and PC2 when including the lymphocytic sialadenitis grade (model 4). When excluding the pSS status and including both anti-SSA antibodies and lymphocytic sialadenitis grade in a new linear regression model, PC2 was strongly associated with the presence of anti-SSA antibodies (p = 0.002) but not with lymphocytic sialadenitis (p = 0.10). In comparison, PC1 was solely associated with the lymphocytic sialadenitis grade (p = 0.003).
Table 2 Influence of presence of anti-SSA antibodies and lymphocytic sialadenitis on the metabolomic signature.
The metabolic signature we highlighted here is to our knowledge the first to be reported in the LSG in Sjögren’s syndrome. Its dichotomous composition, involving on one hand the increase of kynurenine known as one of the main immune-metabolites (37), and on the other hand the decrease of five phospholipids, leads us to consider it as an immuno-lipidomic signature. In addition, this dichotomy also reflects two distinct pathological phenomena since kynurenine was predominantly associated with the presence of anti-SSA antibodies and the phospholipid component with the intensity of lymphocytic infiltrates.
Concerning the five phospholipids (LysoPCaC28:0, PCaaC26:0, PCaaC30:2, PCaeC30:1, PCaeC30:2) with decreased concentration in pSS gland tissue, there is a reverse correlation between the phospholipid concentrations and the severity of lymphocytic sialadenitis (i.e., more intense was the grade more decreased were the concentrations). At first glance, this might suggest an inadequate production of phospholipids which could be consistent with previous works showing that a decreased concentration of phosphatidylcholines within dental plaque was associated with the risk of dental caries, including in pSS patients (38). Indeed, glycerophospholipids help to protect from caries by limiting diffusion of lactic acid (39). In addition, this is in accordance with findings from Tomita Y. et al. highlighting that saliva concentration of lipids correlates with salivary flow (40). Thus, this could link the intensity of sialadenitis, the reduced salivary flow, and the low saliva concentration of phospholipids to explain the severity of dental disorders in pSS. In addition, previous works noticed a significant increase of choline in saliva from pSS patients (41, 42), that could be related to this alteration of phosphatidylcholines concentration. The correlation between phosphatidylcholines impairment with the intensity of lymphocytic sialadenitis could be interesting for early diagnosis since these changes probably precede the occurrence of lymphocytes infiltrates, currently used in classification criteria, over the disease course.
The kynurenine is a biogenic amine synthesized from tryptophan under the action of indole-amine 2,3-dioxygenase (IDO), that can be transformed either into kynurenic acid via the kynurenine aminotransferase with properties of immunomodulation and neuroprotection, or into quinolinic acid via kynurenine 3-monooxygenase with proinflammatory and proapoptotic properties (43). The balance between those enzymes is regulated by the influence of different cytokines: type I and II interferons but also interleukine 1 (IL-1), IL-6, and TNF-alpha: IDO is finely regulated, its gene Indo containing a promoter stimulated by interferons (44), although IDO also induces biofeedback on interferon alpha through dendritic cells and CD80 (45). Imbalance of tryptophan/kynurenine has largely been documented in immune diseases (37) including pSS (46), in which increased activity of IDO (i.e., increased ratio kynurenine/tryptophan) was associated with an immunologically-active disease (46) and with intense interferon signature (44). Some authors emphasized the potential role of kynurenine in neurological involvement, chronic pain, and fatigue in pSS (47). To our knowledge, this study is the first to highlight the importance of kynurenine in salivary glands for pSS. In addition, Sardenberg W.M. et al. demonstrated that the elevation of plasma kynurenine was associated with greater adipose infiltration in LSG in pSS patients, suggesting that the pathway interferon-IDO-kynurenine could be implicated in salivary gland destruction (48). Our study brings a new milestone to those findings because we have unraveled a close relationship between the increase of kynurenine in salivary glands and the presence of anti-SSA antibodies in blood, even after adjustment for intensity of lymphocytic sialadenitis. Kynurenine might not only be a marker of gland destruction but the witness of an early specific immunological process within salivary glands in pSS, under the influence of type I interferons (23), that may precede the intense lymphocyte infiltrates. This would make kynurenine a candidate biomarker of high interest for early diagnosis of pSS.
The limitations of this study include the targeted metabolomic approach. Untargeted metabolomic studies would cover a wider part of metabolome. However, the untargeted approaches are also limited by their low level of standardization, with large variation of metabolite concentrations, which make them difficult to use in the discovery of clinical biomarkers. This study is also limited by its bulk nature, which did not allow us to precisely determine the source of metabolite changes within salivary glands, whether they originate from infiltrating lymphocytes or from epithelial cells. However, we were able to demonstrate that the phospholipidic component of our signature was correlated with the intensity of lymphocyte infiltration, unlike kynurenine. We hypothesized that alterations in kynurenine levels were more likely to be associated with qualitative changes related to the presence of systemic autoimmunity. In order to confirm this hypothesis and gain a deeper understanding of the underlying mechanisms, targeted analyses using techniques such as single-cell analysis, sorting, or microdissection will be necessary. However, the identification of kynurenine among the metabolic signature, a well-known metabolite for its role in immunometabolism balance, confirms the robustness of our findings and the potential for a clinical use.
To conclude, this study is the first to report a metabolomic signature able to distinguish pSS from non-pSS sicca syndromes at an early stage of the disease. The immuno-lipidomic signature has two main components, one with kynurenine is related to anti-SSA antibodies auto-immunity, while the phospholipid component is related to the intensity of lymphocytic infiltrates. These two components of the signature could therefore constitute excellent biomarker candidates for early diagnosis of pSS from the salivary glands, with the main objective of reducing the diagnostic errancy in this disease.
The raw data supporting the conclusions of this article will be made available by the authors, without undue reservation.
The studies involving human participants were reviewed and approved by Ethical Committees of Angers University Hospital (CPP DC2014-2224, AC2017-2993, bioethics n°2018/42). The patients/participants provided their written informed consent to participate in this study.
GU, FC, CL and PR contributed to conception and design of the study. GU, ED, CaL, BD, JC, and GS collected and managed the samples and organized the database. OB managed the sample biocollection. GU and FC performed the statistical analysis. GU, FC, CL and PR wrote the first draft of the manuscript. All authors contributed to manuscript revision, read, and approved the submitted version.
This work was supported by grants from the Association Française du Gougerot-Sjögren (AFGS) and the Société Nationale Française de Médecine Interne (SNFMI).
We warmly thank all the individuals who participated to this study. We would also like to thank Lydie Tessier, and Stéphanie Chupin for their technical assistance, and Caroline Shiboski for her insightful comments and editorial suggestions.
The authors declare that the research was conducted in the absence of any commercial or financial relationships that could be construed as a potential conflict of interest.
All claims expressed in this article are solely those of the authors and do not necessarily represent those of their affiliated organizations, or those of the publisher, the editors and the reviewers. Any product that may be evaluated in this article, or claim that may be made by its manufacturer, is not guaranteed or endorsed by the publisher.
The Supplementary Material for this article can be found online at: https://www.frontiersin.org/articles/10.3389/fimmu.2023.1205616/full#supplementary-material
1. Mariette X, Criswell LA. Primary Sjögren’s syndrome. N Engl J Med (2018) 378:931–9. doi: 10.1056/NEJMcp1702514
2. Shiboski CH, Shiboski SC, Seror R, Criswell LA, Labetoulle M, Lietman TM, et al. 2016 American College of Rheumatology/European league against rheumatism classification criteria for primary Sjögren’s syndrome: a consensus and data-driven methodology involving three international patient cohorts. Arthritis Rheumatol (Hoboken NJ) (2017) 69:35–45. doi: 10.1002/art.39859
3. Chisholm DM, Mason DK. Labial salivary gland biopsy in Sjögren’s disease. J Clin Pathol (1968) 21:656–60. doi: 10.1136/jcp.21.5.656
4. Pijpe J, Kalk WWI, van der Wal JE, Vissink A, Kluin PM, Roodenburg JLN, et al. Parotid gland biopsy compared with labial biopsy in the diagnosis of patients with primary sjogren’s syndrome. Rheumatol (Oxford) (2007) 46:335–41. doi: 10.1093/rheumatology/kel266
5. Vitali C, Moutsopoulos HM, Bombardieri S. The European community study group on diagnostic criteria for Sjögren’s syndrome. sensitivity and specificity of tests for ocular and oral involvement in Sjögren’s syndrome. Ann Rheum Dis (1994) 53:637–47. doi: 10.1136/ard.53.10.637
6. van Stein-Callenfels D, Tan J, Bloemena E, van Vugt RM, Voskuyl AE, Santana NTY, et al. The role of a labial salivary gland biopsy in the diagnostic procedure for Sjögren’s syndrome; a study of 94 cases. Med Oral Patol Oral Cir Bucal (2014) 19:e372–376. doi: 10.4317/medoral.20010
7. Lacombe V, Lacout C, Lozac’h P, Ghali A, Gury A, Lavigne C, et al. Unstimulated whole saliva flow for diagnosis of primary Sjögren’s syndrome: time to revisit the threshold? Arthritis Res Ther (2020) 22:38. doi: 10.1186/s13075-020-2132-3
8. Sène D, Ismael S, Forien M, Charlotte F, Kaci R, Cacoub P, et al. Ectopic germinal center-like structures in minor salivary gland biopsy tissue predict lymphoma occurrence in patients with primary Sjögren’s syndrome. Arthritis Rheumatol (2018) 70:1481–8. doi: 10.1002/art.40528
9. Barone F, Campos J, Bowman S, Fisher BA. The value of histopathological examination of salivary gland biopsies in diagnosis, prognosis and treatment of Sjögren’s syndrome. Swiss Med Weekly (2015) 145:w14168. doi: 10.4414/smw.2015.14168
10. Vivino FB, Gala I, Hermann GA. Change in final diagnosis on second evaluation of labial minor salivary gland biopsies. J Rheumatol (2002) 29:938–44.
11. Huang Y-T, Lu T-H, Chou P-L, Weng M-Y. Diagnostic delay in patients with primary Sjögren’s syndrome: a population-based cohort study in Taiwan. Healthcare (Basel) (2021) 9:363. doi: 10.3390/healthcare9030363
12. Baldini C, Ferro F, Elefante E, Bombardieri S. Biomarkers for Sjögren’s syndrome. biomark Med (2018) 12:275–86. doi: 10.2217/bmm-2017-0297
13. Witas R, Gupta S, Nguyen CQ. Contributions of major cell populations to Sjögren’s syndrome. J Clin Med (2020) 9:E3057. doi: 10.3390/jcm9093057
14. Carubbi F, Alunno A, Cipriani P, Di Benedetto P, Ruscitti P, Berardicurti O, et al. Is minor salivary gland biopsy more than a diagnostic tool in primary Sjögren’s syndrome? association between clinical, histopathological, and molecular features: a retrospective study. Semin Arthritis Rheum (2014) 44:314–24. doi: 10.1016/j.semarthrit.2014.05.015
15. Blokland SLM, Kislat A, Homey B, Smithson GM, Kruize AA, Radstake TRDJ, et al. Decreased circulating CXCR3 + CCR9+T helper cells are associated with elevated levels of their ligands CXCL10 and CCL25 in the salivary gland of patients with Sjögren’s syndrome to facilitate their concerted migration. Scand J Immunol (2020) 91:e12852. doi: 10.1111/sji.12852
16. Lee JH, Kwok S-K, Jung SM, Lee J, Lee J-S, Baek SY, et al. Role of fractalkine in the pathogenesis of primary sjögren syndrome: increased serum levels of fractalkine, its expression in labial salivary glands, and the association with clinical manifestations. J Rheumatol (2014) 41:2425–38. doi: 10.3899/jrheum.130892
17. Aota K, Yamanoi T, Kani K, Nakashiro K-I, Ishimaru N, Azuma M. Inverse correlation between the number of CXCR3+ macrophages and the severity of inflammatory lesions in Sjögren’s syndrome salivary glands: a pilot study. J Oral Pathol Med (2018) 47:710–8. doi: 10.1111/jop.12756
18. Hillen MR, Kruize AA, Bikker A, Wenting-van Wijk M, Radstake TRDJ, Hack CE, et al. Decreased expression of thymic stromal lymphopoietin in salivary glands of patients with primary Sjögren’s syndrome is associated with increased disease activity. Mod Rheumatol (2016) 26:105–9. doi: 10.3109/14397595.2015.1054089
19. Navarro-Mendoza EP, Aguirre-Valencia D, Posso-Osorio I, Correa-Forero SV, Torres-Cutiva D-F, Loaiza D, et al. Cytokine markers of b lymphocytes in minor salivary gland infiltrates in Sjögren’s syndrome. Autoimmun Rev (2018) 17:709–14. doi: 10.1016/j.autrev.2018.02.003
20. Carrillo-Ballesteros FJ, Palafox-Sánchez CA, Franco-Topete RA, Muñoz-Valle JF, Orozco-Barocio G, Martínez-Bonilla GE, et al. Expression of BAFF and BAFF receptors in primary Sjögren’s syndrome patients with ectopic germinal center-like structures. Clin Exp Med (2020) 20:615–26. doi: 10.1007/s10238-020-00637-0
21. Blokland SLM, Hillen MR, van Vliet-Moret FM, Bikker A, de Jager W, Pandit A, et al. Salivary gland secretome: a novel tool towards molecular stratification of patients with primary Sjögren’s syndrome and non-autoimmune sicca. RMD Open (2019) 5:e000772. doi: 10.1136/rmdopen-2018-000772
22. Verstappen GM, Pringle S, Bootsma H, Kroese FGM. Epithelial-immune cell interplay in primary sjögren syndrome salivary gland pathogenesis. Nat Rev Rheumatol (2021) 17:333–48. doi: 10.1038/s41584-021-00605-2
23. Pontarini E, Murray-Brown WJ, Croia C, Lucchesi D, Conway J, Rivellese F, et al. Unique expansion of IL-21+ tfh and tph cells under control of ICOS identifies Sjögren’s syndrome with ectopic germinal centres and MALT lymphoma. Ann Rheum Dis (2020) 79:1588–99. doi: 10.1136/annrheumdis-2020-217646
24. Ryu OH, Atkinson JC, Hoehn GT, Illei GG, Hart TC. Identification of parotid salivary biomarkers in sjogren’s syndrome by surface-enhanced laser desorption/ionization time-of-flight mass spectrometry and two-dimensional difference gel electrophoresis. Rheumatol (Oxford) (2006) 45:1077–86. doi: 10.1093/rheumatology/kei212
25. Hjelmervik TOR, Jonsson R, Bolstad AI. The minor salivary gland proteome in Sjögren’s syndrome. Oral Dis (2009) 15:342–53. doi: 10.1111/j.1601-0825.2009.01531.x
26. Sembler-Møller ML, Belstrøm D, Locht H, Pedersen AML. Proteomics of saliva, plasma, and salivary gland tissue in Sjögren’s syndrome and non-sjögren patients identify novel biomarker candidates. J Proteomics (2020) 225:103877. doi: 10.1016/j.jprot.2020.103877
27. Urbanski G, Assad S, Chabrun F, Chao de la Barca JM, Blanchet O, Simard G, et al. Tear metabolomics highlights new potential biomarkers for differentiating between Sjögren’s syndrome and other causes of dry eye. Ocul Surf (2021) 22:110–6. doi: 10.1016/j.jtos.2021.07.006
28. Durán CE, Azermai M, Vander Stichele RH. Systematic review of anticholinergic risk scales in older adults. Eur J Clin Pharmacol (2013) 69:1485–96. doi: 10.1007/s00228-013-1499-3
29. Seror R, Gottenberg JE, Devauchelle-Pensec V, Dubost JJ, Le Guern V, Hayem G, et al. European League against rheumatism Sjögren’s syndrome disease activity index and European league against rheumatism Sjögren’s syndrome patient-reported index: a complete picture of primary Sjögren’s syndrome patients. Arthritis Care Res (Hoboken) (2013) 65:1358–64. doi: 10.1002/acr.21991
30. Fisher BA, Jonsson R, Daniels T, Bombardieri M, Brown RM, Morgan P, et al. Standardisation of labial salivary gland histopathology in clinical trials in primary Sjögren’s syndrome. Ann Rheum Dis (2017) 76:1161–8. doi: 10.1136/annrheumdis-2016-210448
31. Chabrun F, Dieu X, Rousseau G, Chupin S, Letournel F, Procaccio V, et al. Metabolomics reveals highly regional specificity of cerebral sexual dimorphism in mice. Prog Neurobiol (2020) 184:101698. doi: 10.1016/j.pneurobio.2019.101698
32. Lê S, Josse J, Husson F. FactoMineR: an r package for multivariate analysis. J Stat Software (2008) 25:1–18. doi: 10.18637/jss.v025.i01
33. Therneau T, Atkinson B. Port BR (producer of the initial r, maintainer 1999-2017), in: Rpart: recursive partitioning and regression trees (2019). Available at: https://CRAN.R-project.org/package=rpart.
34. Friedman JH, Hastie T, Tibshirani R. Regularization paths for generalized linear models via coordinate descent. J Stat Software (2010) 33:1–22. doi: 10.18637/jss.v033.i01
35. Liaw, Wiener. Classification and regression by randomForest Andy liaw and Matthew wiener. R News (2002) 2:18–22.
36. Robin X, Turck N, Hainard A, Tiberti N, Lisacek F, Sanchez J-C, et al. pROC: an open-source package for r and s+ to analyze and compare ROC curves. BMC Bioinf (2011) 12:77. doi: 10.1186/1471-2105-12-77
37. Filippini P, Del Papa N, Sambataro D, Del Bufalo A, Locatelli F, Rutella S. Emerging concepts on inhibitors of indoleamine 2,3-dioxygenase in rheumatic diseases. Curr Med Chem (2012) 19:5381–93. doi: 10.2174/092986712803833353
38. Drotleff B, Roth SR, Henkel K, Calderón C, Schlotterbeck J, Neukamm MA, et al. Lipidomic profiling of non-mineralized dental plaque and biofilm by untargeted UHPLC-QTOF-MS/MS and SWATH acquisition. Anal Bioanal Chem (2020) 412:2303–14. doi: 10.1007/s00216-019-02364-2
39. Slomiany BL, Murty VL, Mandel ID, Sengupta S, Slomiany A. Effect of lipids on the lactic acid retardation capacity of tooth enamel and cementum pellicles formed in vitro from saliva of caries-resistant and caries-susceptible human adults. Arch Oral Biol (1990) 35:175–80. doi: 10.1016/0003-9969(90)90052-c
40. Tomita Y, Miyake N, Yamanaka S. Lipids in human parotid saliva with regard to caries experience. J Oleo Sci (2008) 57:115–21. doi: 10.5650/jos.57.115
41. Mikkonen JJ. Metabolic profiling of saliva in patients with primary Sjogren's syndrome. Metabolomics (2012) 3:3. doi: 10.4172/2153-0769.1000128
42. Herrala M, Mikkonen JJW, Pesonen P, Lappalainen R, Tjäderhane L, Niemelä RK, et al. Variability of salivary metabolite levels in patients with Sjögren’s syndrome. J Oral Sci (2020) 63:22–6. doi: 10.2334/josnusd.19-0504
43. Fallarino F, Grohmann U, You S, McGrath BC, Cavener DR, Vacca C, et al. The combined effects of tryptophan starvation and tryptophan catabolites down-regulate T cell receptor zeta-chain and induce a regulatory phenotype in naive T cells. J Immunol (2006) 176:6752–61. doi: 10.4049/jimmunol.176.11.6752
44. Puccetti P. On watching the watchers: IDO and type I/II IFN. Eur J Immunol (2007) 37:876–9. doi: 10.1002/eji.200737184
45. Manlapat AK, Kahler DJ, Chandler PR, Munn DH, Mellor AL. Cell-autonomous control of interferon type I expression by indoleamine 2,3-dioxygenase in regulatory CD19+ dendritic cells. Eur J Immunol (2007) 37:1064–71. doi: 10.1002/eji.200636690
46. Pertovaara M, Raitala A, Uusitalo H, Pukander J, Helin H, Oja SS, et al. Mechanisms dependent on tryptophan catabolism regulate immune responses in primary Sjögren’s syndrome. Clin Exp Immunol (2005) 142:155–61. doi: 10.1111/j.1365-2249.2005.02889.x
47. de Oliveira FR, Fantucci MZ, Adriano L, Valim V, Cunha TM, Louzada-Junior P, et al. Neurological and inflammatory manifestations in Sjögren’s syndrome: the role of the kynurenine metabolic pathway. Int J Mol Sci (2018) 19:E3953. doi: 10.3390/ijms19123953
Keywords: kynurenine, biomarker, metabolomics, Sjögren’s syndrome, sicca, salivary glands, omics
Citation: Urbanski G, Chabrun F, Delattre E, Lacout C, Davidson B, Blanchet O, Chao de la Barca JM, Simard G, Lavigne C and Reynier P (2023) An immuno-lipidomic signature revealed by metabolomic and machine-learning approaches in labial salivary gland to diagnose primary Sjögren’s syndrome. Front. Immunol. 14:1205616. doi: 10.3389/fimmu.2023.1205616
Received: 14 April 2023; Accepted: 28 June 2023;
Published: 14 July 2023.
Edited by:
Efstathia K. Kapsogeorgou, National and Kapodistrian University of Athens, GreeceReviewed by:
Paola Perez, National Institute of Dental and Craniofacial Research (NIH), United StatesCopyright © 2023 Urbanski, Chabrun, Delattre, Lacout, Davidson, Blanchet, Chao de la Barca, Simard, Lavigne and Reynier. This is an open-access article distributed under the terms of the Creative Commons Attribution License (CC BY). The use, distribution or reproduction in other forums is permitted, provided the original author(s) and the copyright owner(s) are credited and that the original publication in this journal is cited, in accordance with accepted academic practice. No use, distribution or reproduction is permitted which does not comply with these terms.
*Correspondence: Geoffrey Urbanski, dXJiYW5za2lnZW9mZnJleUBnbWFpbC5jb20=
†These authors have contributed equally to this work and share last authorship
Disclaimer: All claims expressed in this article are solely those of the authors and do not necessarily represent those of their affiliated organizations, or those of the publisher, the editors and the reviewers. Any product that may be evaluated in this article or claim that may be made by its manufacturer is not guaranteed or endorsed by the publisher.
Research integrity at Frontiers
Learn more about the work of our research integrity team to safeguard the quality of each article we publish.