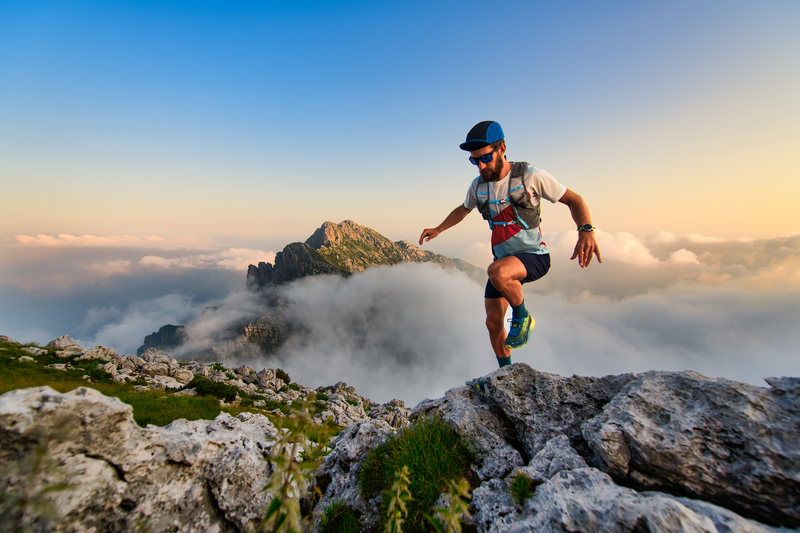
95% of researchers rate our articles as excellent or good
Learn more about the work of our research integrity team to safeguard the quality of each article we publish.
Find out more
ORIGINAL RESEARCH article
Front. Immunol. , 15 June 2023
Sec. Autoimmune and Autoinflammatory Disorders : Autoimmune Disorders
Volume 14 - 2023 | https://doi.org/10.3389/fimmu.2023.1200732
Objectives: Lupus nephritis (LN) remains one of the most severe manifestations in patients with systemic lupus erythematosus (SLE). Onset and overall LN risk among SLE patients remains considerably difficult to predict. Utilizing a territory-wide longitudinal cohort of over 10 years serial follow-up data, we developed and validated a risk stratification strategy to predict LN risk among Chinese SLE patients – Risk and Factors associated with disease manifestations in systemic Lupus Erythematosus – Lupus Nephritis (RIFLE-LN).
Methods: Demographic and longitudinal data including autoantibody profiles, clinical manifestations, major organ involvement, LN biopsy results and outcomes were documented. Association analysis was performed to identify factors associated with LN. Regression modelling was used to develop a prediction model for 10-year risk of LN and thereafter validated.
Results: A total of 1652 patients were recruited: 1382 patients were assigned for training and validation of the RIFLE-LN model; while 270 were assigned for testing. The median follow-up duration was 21 years. In the training and validation cohort, 845 (61%) of SLE patients developed LN. Cox regression and log rank test showed significant positive association between male sex, age of SLE onset and anti-dsDNA positivity. These factors were thereafter used to develop RIFLE-LN. The algorithm was tested in 270 independent patients and showed good performance (AUC = 0·70).
Conclusion: By using male sex, anti-dsDNA positivity, age of SLE onset and SLE duration; RIFLE-LN can predict LN among Chinese SLE patients with good performance. We advocate its potential utility in guiding clinical management and disease monitoring. Further validation studies in independent cohorts are required.
Systemic lupus erythematosus (SLE) is a complex autoimmune disease with a wide range of heterogenous manifestations. The clinical diagnosis of SLE is usually made in reference to the various versions of classification criteria (1–3). Nonetheless a fundamental disadvantage of using such systems is that individuals who fulfil such criteria for the umbrella diagnosis of “SLE” can vary hugely and there remains much patient heterogeneity. Individuals can vary widely in terms of different organ involvement, disease severity and/or laboratory abnormalities. This diverse heterogeneity also leads to huge diagnostic and therapeutic challenges, imposing limitations on disease diagnosis, prognostication, and treatment (4–6).
A prime example of such heterogeneity is lupus nephritis (LN), with its incidence and prevalence varying greatly across different populations and ethnicities. LN remains one of the most severe organ manifestations, and affects up to 50-60% of SLE patients (5, 7, 8). Despite advancement in treatment strategies, the risk of progression to end-stage renal disease (ESRD) remained at 10-30% (9). The prevalence and incidence of LN varies greatly depending on a myriad of factors, including age of disease onset, sex, ethnicity, and autoantibody profile (4, 10–12). However, studies investigating the incidence of LN remain scarce, and longitudinal studies investigating risk factors associated with LN development are lacking. Identification of patients at risk of LN development will facilitate early diagnosis and treatment to minimize risk of organ damage.
Prediction models provide the foundation for personalized medicine and risk prediction models have been increasingly utilized to assist clinical decision making (13, 14). Given the diverse heterogeneity in SLE patients, prediction models may potentially be useful to inform physicians and patients on possible disease manifestations and outcomes (15). For example, patients deemed at higher risk of developing LN may benefit from more intensive or frequent monitoring, have lower threshold for decision towards invasive renal biopsies or costly immunosuppressive therapies.
To tackle the unpredictability of SLE manifestations and enhanced personalized medicine for SLE patients, we recruited a territory-wide longitudinal cohort to develop prediction strategies for disease manifestations and flares – Risk and Factors associated with disease manifestations in systemic Lupus Erythematosus (RIFLE). Only patients with at least 10 years of serial follow-up data were recruited. In this study involving a multidisciplinary team of bioinformaticians, immunologists, paediatricians, nephrologists and rheumatologists, we developed and validated a risk stratification strategy to predict LN risk among Chinese SLE patients - RIFLE-LN.
RIFLE-LN was established with patient recruitment from eight major tertiary referral centres across Hong Kong. Only physician diagnosed SLE patients with at least 10 years of serial follow-up data or all-cause deaths were included and longitudinally analysed. Patients were regularly followed-up according to their clinical need (with average frequency of around every 4 months). Patients of Chinese descent were included (reported by patients). Clinical, blood and urine results were documented during each visit. Data from all clinical notes and visits were extracted for analysis; this included age of disease onset, gender, presence of SLE-related autoantibodies (ever-positive), urine results, clinical manifestations, renal biopsy results. All patients with a physician diagnosis of LN based on clinical and laboratory evidence suggestive of disease activity and renal involvement were identified. This included the presence of persistent proteinuria (urine protein concentration >0·5 gram per day on ≥ 2 occasions), urinary cellular casts, or histological evidence of LN (2).
In patients with biopsy-proven LN, histological classes according to the International Society of Nephrology/Renal Pathology Society classification were recorded (16). Estimated glomerular filtration rate (eGFR) was calculated using modification of diet in real disease (MDRD) formula. Chronic kidney disease (CKD) was defined according to the Kidney Disease: Improving Global Outcome classification as eGFR<60ml/min/1·73m2 for three months or more (17). End-stage renal disease (ESRD) was defined as stage 5 chronic kidney disease (eGFR <15ml/min/1·73m2) or the initiation of long-term renal replacement therapy (RRT).
Patients were randomly assigned to either the training and validation cohort and the testing cohort in 5:1 ratio. Based on clinical and serological features in the training and validation cohort, a prediction model was developed based on factors that showed statistically significant association with LN in regression analysis and SLE duration. Generalized linear model (GLM) was used to predict the probability of LN based on age of SLE onset, male sex, and anti-dsDNA positivity. The results from GLM were used to generate the predicted probability of LN at various timepoints after SLE onset. Data from 90% of patients were randomly selected for training and the remaining 10% were used for validation. The process was repeated 10 times to overcome selection bias. The values with greatest sensitivity and specificity after ten cycles of training and validation were selected.
Continuous variables were expressed as median with interquartile range (IQR) and compared using Mann-Whitney U test. Categorical variables were expressed as percentage and compared using chi-square test. Cox regression analysis was performed to identify variables associated with LN. Variables with p-value <0·1 in the univariate analysis were included in the multivariate model. Variables with p-value <0·05 in multivariate analyses were considered as significant. Hazard ratios were reported with 95% confidence interval. The variables were tested in using log rank test.
Prediction performance of RIFLE-LN was evaluated in the testing cohort using area under the receiver operating curve (AUC-ROC). The performance was defined as excellent (AUC 0·9-1), very good (AUC 0·8-0·9), good (AUC 0·7-0·8), satisfactory (AUC 0·6-0·7) or unsatisfactory (0·5-0·6). R version 4·0·3 was used for the development of the prediction model, and SPSS Statistics version 28 was used for other statistical analyses.
A total of 1652 Chinese patients with SLE were recruited with a median age of disease onset at 29 years. The median duration of follow-up was 21 years. 1382 patients were assigned for training and validation of the RIFLE-LN model, while 270 were assigned for testing. All patients were tested positive for anti-nuclear antibody. Details of patient demographics, disease manifestations, autoantibody profiles, major organ involvement, mortality and breakdown of various renal profiles for LN patients in the training and validation cohort and the testing cohort are shown in Tables 1, 2, respectively. Patient characteristics of the two cohorts were comparable, except a higher frequency of anti-Sm and anti-La in the testing cohort (Supplementary Table 1). Use of immunosuppressive agents is summarised in Table 3.
The training and validation cohort included 1382 patients with SLE, and lupus nephritis developed in 845 (61·1%) patients primarily manifested early in the disease course (Figure 1). Among patients who ever developed LN, 45·4%, 71·1% and 85·2% presented within their first year, 5 years and 10 years of SLE onset; respectively. A total of 707 (83·7%) patients had biopsy-proven LN. Proliferative (or mixed proliferative and membranous) LN were the most common histological subtypes and occurred in 537 (63·6%) patients. Pure membranous LN occurred in 111 (13·1%) patients. ESRD developed in 67 (7.9%) of LN patients after a median of 16·5 years after LN onset. A total of 139 deaths were observed with a median age of death of 57 years. Infection, cardiovascular events, and malignancy were the most common causes of death. Other causes included active SLE, pancreatitis, suicide, and surgical complications. There was no significance difference in mortality and age of death in patients who developed LN.
Variables including age of SLE onset, male sex, autoantibodies and clinical features were evaluated for their association with LN in Cox regression analysis (Table 4). In univariate analysis, factors associated with LN included young age of onset, male sex, anti-dsDNA, and haematological involvement; whereas pulmonary involvement was a negative predictor. Multivariate analysis showed that young age of onset (HR 1.50, 95% CI 1.09-2.06, p=0.013), male sex (HR 1·40, 95% CI 1·12-1·75, p=0·003), and anti-dsDNA autoantibody positivity (HR 1·57, 95% CI 1·30-1·90, p<0·001) were significantly associated with LN; pulmonary involvement remained a negative predictor (HR 0.78, 95% CI 0.62-0.98, p=0.035). Considering the limited number of patients with pulmonary involvement in the training and validation cohort (accounting for 10.9%), only young age of onset, male sex, anti-dsDNA autoantibody and disease duration were further used for model development. Figure 2 represents the Kaplan-Meier analysis showing the effects of anti-dsDNA positivity, male sex and age of SLE onset on the occurrence of LN. These factors were therefore used for incorporation of the RIFLE-LN risk prediction model.
Figure 2 Log rank test of factors associated with LN development: (A) – antids-DNA positivity; (B) – male sex; (C) – age of SLE onset.
Risk factors identified in regression analysis (age of onset, male sex, and anti-dsDNA autoantibody) and SLE duration were used to develop RIFLE-LN, a prediction model for the 10-year risk of LN development. Model training and validation was repeated ten times, and model parameters with the greatest sensitivity and specificity after ten cycles were used. Probability graphs of RIFLE-LN were generated for visualisation based on age of SLE onset (< 18 years old, 18-50 years old, > 50 years old), sex, anti-dsDNA positivity and SLE duration (Figure 3).
The performance of RIFLE-LN was evaluated in a testing cohort of 270 independent patients with SLE. The model performance was assessed using AUC-ROC, and it demonstrated good performance (AUC = 0·70) (Figure 4). The sensitivity and specificity were 0·73 and 0·57, respectively.
Using the comprehensive data from a territory-wide longitudinal cohort, we developed a prediction model for the 10-year risk of LN in Chinese patients with SLE. The model demonstrated good performance in LN prediction and a potential role in clinical application.
LN remains a major risk factor for morbidity and mortality in patients with SLE (18). A clinical challenge exists in predicting and identifying patients at risk of LN. The unmet needs are particularly important among Asian SLE patients, who have a higher frequency and more severe LN (19). In our study, the burden of LN was significant; over 60% of patients were diagnosed with LN and 63.6%% had diffuse proliferative LN (or mixed proliferative and membranous). Intriguingly, while some SLE patients experience recurrent LN flares, a substantial proportion of patients do not develop LN during their entire disease course; which suggests marked heterogeneity and the possibility of sub-phenotypes even among LN patients. LN represents a distinct subtype which occurs in certain SLE patients. LN risk prediction among SLE patients may facilitate a more personalised approach in disease monitoring and management; where patients deemed at higher risk of developing LN may benefit from more intensive monitoring, have lower threshold for decision towards invasive renal biopsies or costly immunosuppressive therapies.
The risk of LN development is attributed by a combination of clinical and genetic factors (6). RIFLE-LN is a prediction model developed based on four readily-available features derived from regression analysis. These factors are in line with previous studies as key to LN development: age of SLE onset, male sex, anti-dsDNA autoantibody and SLE duration. Young age of SLE onset has been consistently reported as an important risk factor of LN, likely due to a stronger genetic contribution in disease pathogenesis among these patients (20). The association between male sex and renal involvement highlights the complex interaction of multiple sex hormones involved in SLE (21). On the autoantibody level, loss of immune tolerance and the production of autoantibodies are central to SLE pathogenesis. Anti-dsDNA autoantibody has been strongly linked to LN, and different mechanisms underpinning its nephritogenic potential have been proposed (22). Utilizing age of SLE onset, male sex and anti-dsDNA autoantibody positivity, RIFLE-LN incorporated the different aspects of disease pathogenesis in LN prediction.
Our longitudinal data allowed us to capture a key factor in LN prediction, namely the SLE disease duration. We confirmed that LN often developed early, and 71% of LN in our study occurred within five years of SLE diagnosis. The immunopathogenic mechanisms responsible for SLE initiation, may have directly contributed to LN development. However, LN can develop late in the disease course. Previous studies showed no difference in disease profile and treatment outcome in patients with late-onset LN (23). Maintaining vigilance in identifying LN, especially in patients at ongoing risk is therefore important. RIFLE-LN is a prediction model of 10-year LN risk in SLE. Our algorithm captures the LN probability from each of the first 10 years after SLE diagnosis, addressing the unmet needs of personalised risk assessment and monitoring for LN in SLE.
RIFLE-LN was a multidisciplinary collaboration and developed jointly by a group of rheumatologists, nephrologists, paediatricians, immunologist and bioinformaticians across multiple tertiary centres. Our unique cohort captured a wide spectrum of SLE patients from different specialty clinics. We included over 1000 SLE patients for algorithm training and validation, and comprising different patient subgroups (8·4% patients were male, 18·2% patients had young-onset SLE defined as age of SLE onset younger than 18 years, and 61·1% had LN). Derived from a cohort with diverse disease heterogeneity, RIFLE-LN can be applied across different clinical settings.
Artificial intelligence prediction models have been increasingly applied in medicine and healthcare (24). Regression analysis is one of the commonest prediction models, with the advantages of simple implementation and interpretation. Despite using only four factors, RIFLE-LN demonstrated good performance in our testing cohort. The rapid development in artificial intelligence has changed the landscape of medical research and healthcare in areas including basic research, translational medicine and clinical practice (25). RIFLE-LN aims to improve risk stratification, guide disease monitoring, facilitate early recognition of LN, and complement physician’s judgement in clinical decision and diagnosis.
The exact aetiology of SLE remains elusive, and a combination of polygenic and environmental risk factors are believed to orchestrate disease development. Our centre was among the first to conduct genome-wide association studies (GWAS) of SLE in Asia. Over a hundred of genetic loci have been identified through GWAS analysis (26, 27), and polygenic risk score has been explored as an approach to evaluate an individual’s genetic predisposition from genome-wide risk measurement and the aggregated risk from different disease alleles. Furthermore, the variety of subphenotypes is most likely due to different underlying disease mechanisms contributed by distinct genetic predispositions (28). RIFLE-LN sets a good foundation for LN risk prediction, and we propose that future prediction model should incorporate genetic risk measurement together with clinical and serological features in SLE.
There were several limitations in this study. Despite our large patient cohort, only patients of Chinese ethnicity were included. External validation of the algorithm in patients with other ethnicities is warranted. All patients with LN, with or without renal biopsy, were included. Among patients without renal biopsy, LN was diagnosed by treating physicians based on clinical and laboratory evidence of disease activity and renal involvement. Patient’s refusal, unstable medical conditions, and bleeding risk were the three major reasons why biopsy was not performed. Histological information and classes were not available in patients who did not undergo renal biopsy. However, this approach allowed a more accurate reflection of the true prevalence of LN among patients with SLE. Furthermore, our study the presence of SLE-related autoantibodies was defined as ever-positive, and changes in anti-dsDNA autoantibody titre were not evaluated. RIFLE-LN aims to improve risk stratification of LN but does not replace physician’s judgement in disease diagnosis.
By using sex, anti-dsDNA positivity, age of onset and SLE duration; RIFLE-LN can predict LN development among Chinese SLE patients with good performance. We advocate its potential utility in guiding patient treatment and disease monitoring. Further validation studies using independent cohorts, especially with different ethnicities and populations, are required.
The original contributions presented in the study are included in the article/Supplementary Material; further inquiries can be directed to the corresponding authors.
The studies involving human participants were reviewed and approved by Hospital Authority Central Institutional Review Board. Written informed consent for participation was not required for this study in accordance with the national legislation and the institutional requirements.
TC, YL, WL, PHL, and CL contributed to study planning and design. PPL, WL, SY, NT, AL, CM, KL, TL, HHL, WWY, and CH contributed to the acquisition, analysis or interpretation of data. SC, and PHL contributed to drafting of the manuscript. TC, YL, WL, RW, and CL contributed to the critical revision of the manuscript. The first and corresponding authors had full access of all the data in the study and had final responsibility for the decision to submit the manuscript for publication. All authors contributed to the article and approved the submitted version.
This work was partially funding by the Department of Medicine, School of Clinical Medicine, The University of Hong Kong.
The authors declare that the research was conducted in the absence of any commercial or financial relationships that could be construed as a potential conflict of interest.
All claims expressed in this article are solely those of the authors and do not necessarily represent those of their affiliated organizations, or those of the publisher, the editors and the reviewers. Any product that may be evaluated in this article, or claim that may be made by its manufacturer, is not guaranteed or endorsed by the publisher.
The Supplementary Material for this article can be found online at: https://www.frontiersin.org/articles/10.3389/fimmu.2023.1200732/full#supplementary-material
1. Aringer M, Costenbader K, Daikh D, Brinks R, Mosca M, Ramsey-Goldman R, et al. European League against Rheumatism/American college of rheumatology classification criteria for systemic lupus erythematosus. Arthritis Rheumatol (2019) 71(9):1400–12. doi: 10.1002/art.40930
2. Hochberg MC. Updating the American college of rheumatology revised criteria for the classification of systemic lupus erythematosus. Arthritis Rheum (1997) 40(9):1725. doi: 10.1002/art.1780400928
3. Petri M, Orbai AM, Alarcon GS, Gordon C, Merrill JT, Fortin PR, et al. Derivation and validation of the systemic lupus international collaborating clinics classification criteria for systemic lupus erythematosus. Arthritis Rheum (2012) 64(8):2677–86. doi: 10.1002/art.34473
4. Li PH, Wong WH, Lee TL, Lau CS, Chan TM, Leung AMH, et al. Relationship between autoantibody clustering and clinical subsets in SLE: cluster and association analyses in Hong Kong Chinese. Rheumatol (Oxford) (2013) 52(2):337–45. doi: 10.1093/rheumatology/kes261
5. To CH, Mok CC, Tang SS, Ying SK, Wong RW, Lau CS. Prognostically distinct clinical patterns of systemic lupus erythematosus identified by cluster analysis. Lupus (2009) 18(14):1267–75. doi: 10.1177/0961203309345767
6. Kaul A, Gordon C, Crow MK, Touma Z, Urowitz MB, van Vollenhoven R, et al. Systemic lupus erythematosus. Nat Rev Dis Primers (2016) 2:16039. doi: 10.1038/nrdp.2016.39
7. Almaani S, Meara A, Rovin BH. Update on lupus nephritis. Clin J Am Soc Nephrol (2017) 12(5):825–35. doi: 10.2215/CJN.05780616
8. Cervera R, Khamashta MA, Font J, Sebastiani GD, Gil A, Lavilla P, et al. Systemic lupus erythematosus: clinical and immunologic patterns of disease expression in a cohort of 1,000 patients. the European working party on systemic lupus erythematosus. Med (Baltimore) (1993) 72(2):113–24. doi: 10.1097/00005792-199303000-00005
9. Tektonidou MG, Dasgupta A, Ward MM. Risk of end-stage renal disease in patients with lupus nephritis, 1971-2015: a systematic review and Bayesian meta-analysis. Arthritis Rheumatol (2016) 68(6):1432–41. doi: 10.1002/art.39594
10. Wenderfer SE, Ruth NM, Brunner HI. Advances in the care of children with lupus nephritis. Pediatr Res (2017) 81(3):406–14. doi: 10.1038/pr.2016.247
11. Ramirez Sepulveda JI, Bolin K, Mofors J, Leonard D, Svenungsson E, Jonsen A, et al. Sex differences in clinical presentation of systemic lupus erythematosus. Biol Sex Differ (2019) 10(1):60.
12. Seligman VA, Lum RF, Olson JL, Li H, Criswell LA. Demographic differences in the development of lupus nephritis: a retrospective analysis. Am J Med (2002) 112(9):726–9. doi: 10.1016/S0002-9343(02)01118-X
13. D'Agostino RB Sr, Vasan RS, Pencina MJ, Wolf PA, Cobain M, Massaro JM, et al. General cardiovascular risk profile for use in primary care: the framingham heart study. Circulation (2008) 117(6):743–53. doi: 10.1161/CIRCULATIONAHA.107.699579
14. Candido Dos Reis FJ, Wishart GC, Dicks EM, Greenberg D, Rashbass J, Schmidt M, et al. An updated PREDICT breast cancer prognostication and treatment benefit prediction model with independent validation. Breast Cancer Res (2017) 19(1):58.
15. Adamichou C, Genitsaridi I, Nikolopoulos D, Nikoloudaki M, Repa A, Bortoluzzi A, et al. Lupus or not? SLE risk probability index (SLERPI): a simple, clinician-friendly machine learning-based model to assist the diagnosis of systemic lupus erythematosus. Ann Rheum Dis (2021) 80(6):758–66. doi: 10.1136/annrheumdis-2020-219069
16. Bajema IM, Wilhelmus S, Alpers CE, Bruijn JA, Colvin RB, Cook HT, et al. Revision of the international society of Nephrology/Renal pathology society classification for lupus nephritis: clarification of definitions, and modified national institutes of health activity and chronicity indices. Kidney Int (2018) 93(4):789–96. doi: 10.1016/j.kint.2017.11.023
17. Levey AS, Eckardt KU, Tsukamoto Y, Levin A, Coresh J, Rossert J, et al. Definition and classification of chronic kidney disease: a position statement from kidney disease: improving global outcomes (KDIGO). Kidney Int (2005) 67(6):2089–100. doi: 10.1111/j.1523-1755.2005.00365.x
18. Mok CC, Kwok RC, Yip PS. Effect of renal disease on the standardized mortality ratio and life expectancy of patients with systemic lupus erythematosus. Arthritis Rheum (2013) 65(8):2154–60. doi: 10.1002/art.38006
19. Yap DY, Yung S, Chan TM. Lupus nephritis: an update on treatments and pathogenesis. Nephrol (Carlton) (2018) 23(Suppl 4):80–3. doi: 10.1111/nep.13469
20. Ambrose N, Morgan TA, Galloway J, Ionnoau Y, Beresford MW, Isenberg DA, et al. Differences in disease phenotype and severity in SLE across age groups. Lupus (2016) 25(14):1542–50. doi: 10.1177/0961203316644333
21. Petri M. Sex hormones and systemic lupus erythematosus. Lupus (2008) 17(5):412–5. doi: 10.1177/0961203308090026
22. Rekvig OP. The dsDNA, anti-dsDNA antibody, and lupus nephritis: what we agree on, what must be done, and what the best strategy forward could be. Front Immunol (2019) 10:1104. doi: 10.3389/fimmu.2019.01104
23. Delfino J, Dos Santos T, Skare TL. Comparison of lupus patients with early and late onset nephritis: a study in 71 patients from a single referral center. Adv Rheumatol (2020) 60(1):5. doi: 10.1186/s42358-019-0105-5
24. Davenport T, Kalakota R. The potential for artificial intelligence in healthcare. Future Healthc J (2019) 6(2):94–8. doi: 10.7861/futurehosp.6-2-94
25. Yu KH, Beam AL, Kohane IS. Artificial intelligence in healthcare. Nat BioMed Eng (2018) 2(10):719–31. doi: 10.1038/s41551-018-0305-z
26. Wang YF, Zhang Y, Lin Z, Zhang H, Wang TY, Cao Y, et al. Identification of 38 novel loci for systemic lupus erythematosus and genetic heterogeneity between ancestral groups. Nat Commun (2021) 12(1):772. doi: 10.1038/s41467-021-21049-y
27. Yin X, Kim K, Suetsugu H, Bang SY, Wen L, Koido M, et al. Meta-analysis of 208370 East asians identifies 113 susceptibility loci for systemic lupus erythematosus. Ann Rheum Dis (2021) 80(5):632–40. doi: 10.1136/annrheumdis-2020-219209
Keywords: systemic lupus erythematosus, lupus nephritis, risk assessment, prediction, model
Citation: Chan SCW, Wang Y-F, Yap DYH, Chan TM, Lau YL, Lee PPW, Lai WM, Ying SKY, Tse NKC, Leung AMH, Mok CC, Lee KL, Li TWL, Tsang HHL, Yeung WWY, Ho CTK, Wong RWS, Yang W, Lau CS and Li PH (2023) Risk and Factors associated with disease manifestations in systemic lupus erythematosus – lupus nephritis (RIFLE-LN): a ten-year risk prediction strategy derived from a cohort of 1652 patients. Front. Immunol. 14:1200732. doi: 10.3389/fimmu.2023.1200732
Received: 05 April 2023; Accepted: 22 May 2023;
Published: 15 June 2023.
Edited by:
Ryusuke Yoshimi, Yokohama City University, JapanReviewed by:
Massimo Radin, University of Turin, ItalyCopyright © 2023 Chan, Wang, Yap, Chan, Lau, Lee, Lai, Ying, Tse, Leung, Mok, Lee, Li, Tsang, Yeung, Ho, Wong, Yang, Lau and Li. This is an open-access article distributed under the terms of the Creative Commons Attribution License (CC BY). The use, distribution or reproduction in other forums is permitted, provided the original author(s) and the copyright owner(s) are credited and that the original publication in this journal is cited, in accordance with accepted academic practice. No use, distribution or reproduction is permitted which does not comply with these terms.
*Correspondence: Philip H. Li, bGlwaGlsaXBAaGt1Lmhr; Chak Sing Lau, Y3NsYXVAaGt1Lmhr
†These authors have contributed equally to this work
‡These authors have contributed equally to this work
Disclaimer: All claims expressed in this article are solely those of the authors and do not necessarily represent those of their affiliated organizations, or those of the publisher, the editors and the reviewers. Any product that may be evaluated in this article or claim that may be made by its manufacturer is not guaranteed or endorsed by the publisher.
Research integrity at Frontiers
Learn more about the work of our research integrity team to safeguard the quality of each article we publish.