- 1Department of Bioinformatics, Institute of Biomedical Chemistry, Moscow, Russia
- 2Department of Bioinformatics, Pirogov Russian National Research Medical University, Moscow, Russia
Introduction: There are difficulties in creating direct antiviral drugs for all viruses, including new, suddenly arising infections, such as COVID-19. Therefore, pathogenesis-directed therapy is often necessary to treat severe viral infections and comorbidities associated with them. Despite significant differences in the etiopathogenesis of viral diseases, in general, they are associated with significant dysfunction of the immune system. Study of common mechanisms of immune dysfunction caused by different viral infections can help develop novel therapeutic strategies to combat infections and associated comorbidities.
Methods: To identify common mechanisms of immune functions disruption during infection by nine different viruses (cytomegalovirus, Ebstein-Barr virus, human T-cell leukemia virus type 1, Hepatitis B and C viruses, human immunodeficiency virus, Dengue virus, SARS-CoV, and SARS-CoV-2), we analyzed the corresponding transcription profiles from peripheral blood mononuclear cells (PBMC) using the originally developed pipeline that include transcriptome data collection, processing, normalization, analysis and search for master regulators of several viral infections. The ten datasets containing transcription data from patients infected by nine viruses and healthy people were obtained from Gene Expression Omnibus. The analysis of the data was performed by Genome Enhancer pipeline.
Results: We revealed common pathways, cellular processes, and master regulators for studied viral infections. We found that all nine viral infections cause immune activation, exhaustion, cell proliferation disruption, and increased susceptibility to apoptosis. Using network analysis, we identified PBMC receptors, representing proteins at the top of signaling pathways that may be responsible for the observed transcriptional changes and maintain the current functional state of cells.
Discussion: The identified relationships between some of them and virus-induced alteration of immune functions are new and have not been found earlier, e.g., receptors for autocrine motility factor, insulin, prolactin, angiotensin II, and immunoglobulin epsilon. Modulation of the identified receptors can be investigated as one of therapeutic strategies for the treatment of severe viral infections.
1 Introduction
Viruses cannot replicate without host cells; therefore, their interaction with hosts is crucial for viral replication, and transmission. On the other hand, the immune system defends the host organism from the virus. Thus, the development of viral infection and the severity of the disease is mediated by a complex interplay between the virus and host immune system (1–4). The intensity and efficacy of the immune response depends on the immune status of a person and the ability of viruses to disrupt human immunity (5). Therefore, the peculiarities of interactions between a particular virus and the human body and corresponding individual host response to infection can be substantial parts of a whole pathogenesis process that determine the severity of viral diseases.
Several viruses, e.g., cytomegalovirus (CMV), Epstein-Barr virus (EBV), human T-cell leukemia virus type 1 (HTLV-1), hepatitis B and C viruses (HBV and HCV), human immunodeficiency virus 1 (HIV-1), can cause chronic or latent infections. Herpesviruses, including CMV and EBV, cause latent infection and can persist for a long time in healthy people without clinical symptoms, but they can be reactivated by inflammatory stimuli or immunosuppression and cause various pathologies (6, 7). Retroviruses such as HTLV-1 and HIV-1 cause chronic infections by integrating viral genome into human DNA. HTLV-1 usually persists in a human organism without symptoms; however, it may cause adult T cell leukemia/lymphoma or HTLV-1-associated myelopathy/tropical spastic paraparesis in a small part of infected people (8, 9). HIV-1 may also persist in the human organism without symptoms for many years but eventually causes acquired immunodeficiency syndrome in almost all people living with HIV and do not receiving antiviral treatment (10).
Other viruses from various families, such as coronaviruses, influenza, and Dengue virus (DENV), cause acute infection diseases with different degrees of severity. In this case, the disease may lead to death or recovery with the complete elimination of the virus (11, 12).
Different viruses, including new, suddenly arising ones, such as SARS-CoV-2, have different transmission routes and target cells, and causes infectious diseases of different duration and severity; therefore, it is challenging to create direct antiviral therapeutics due to differences in the structures and functions of viral targets and the long duration of the drug development process. Although drugs with specific direct antiviral activity (13) including inhibitors of viral nucleic acid polymerases, proteases, integrases, etc., are available for hepatitis C virus (14, 15) and HIV (16), their use may be limited by drug resistance, side effects and toxicity, especially in pediatric and geriatric patients (17), pregnant women (18, 19) and people after surgery. Accessibility of medicines (15, 20) and patient adherence (21) are important determinants of treatment efficacy. Antiretroviral drugs, even if they lead to an undetectable viral load, do not provide the elimination of the virus. Latent HIV infection is another problem that can reduce the effectiveness of drug treatment (10). Thus, pathogenesis-directed therapy can be beneficial for treatment of severe viral infections and associated comorbidities. Despite significant distinctions in the pathogenesis of viral infections, many of them induce the dysfunction of the immune system (22–32). Long-lasting activated immune response with release of cytokines in the case of chronic infections or cytokine storm in the case of some acute infections, e.g., dengue fever (DF) and Coronavirus disease 2019 (COVID-19), lead to cytokine-dependent immune activation, which, in turn, causes immune dysfunction, the so-called “exhaustion”, and significant loss of T lymphocytes. As a result, the immune system cannot eliminate viruses effectively, which leads to high severity of disease, duration, and development of secondary infections and other comorbidities. For instance, chronic cytokine-dependent activation of T cells by HIV-infection causes their apoptosis followed by dysfunction of immune response to infection (33). CMV increases disease progression and mortality in infants with HIV-infection since it raises activation and apoptosis of both CD4(+) and CD8(+) T cells (16). COVID-19 and dengue hemorrhagic fever (DHF) are associated with cytokine storm, which causes the induction of T lymphocytes apoptosis, increasing the disease severity (31, 33, 34). Thus, it is crucial to identify common pathogenetic mechanisms for different viral infections because it will allow developing of new anti-viral drugs applicable for the treatment of severe viral infections.
One of the efficient ways to identify common mechanisms of human immune functions alteration in response to a viral infection is an analysis of genome-wide data obtained by high-throughput experimental methods (10). Most of the studies that analyzed virus-host interactions use the transcriptomics data obtained by microarray and RNA sequencing approaches (35–48). Some of these studies focused on comparing transcriptional changes in blood cells caused by the same or similar viral infections, e.g., respiratory viral infections. For instance, Vavougios G.D. analyzed transcriptomics data and found overlapping host gene signatures between SARS-CoV-2 and other viral and bacterial potential copathogens (40). Dunmire S.K. with colleagues compared the EBV transcription response profile to multiple other acute viral infections, including influenza A, respiratory syncytial virus, human rhinovirus, attenuated yellow fever virus, and DENV. They revealed similarity only to DENV profile as well as that of presented in patients with hemophagocytic syndromes, suggesting that EBV and DENV cause uncontrolled inflammatory responses (35). McClain M.T. with colleagues measured transcription profiles from COVID-19 patients and directly compared them to subjects with seasonal coronavirus, influenza, bacterial pneumonia, and healthy controls. They identified a 23-gene signature, which can be utilized to diagnose and differentiate COVID-19 from other viral diseases (43). Tsalik E.L. and colleagues analyzed gene expression in blood cells and identified transcriptional changes that are either virus-specific or common to respiratory infections such as influenza, enterovirus/rinovirus and human metapneumovirus infections, and nonrespiratory DENV infection (46). Avraham Unterman and co-authors used gene expression analysis of peripheral blood mononuclear cells (PBMC) and searched for protein biomarkers of COVID-19 progression. As a result, the significant increase of interferon-I response and amphiregulin expression levels was found in patients with COVID-19 comparing to the healthy controls. Moreover, some regulatory and tissue repair-associated genes (CD163, IL1R2, AREG, HAVCR2 (encoding TIM-3), and its ligand LGALS9) and pathways (TIM-3/Gal-9 pathway) were found up-regulated in progressive COVID-19 patients (47). Zhidong Tang and co-authors developed the portal of viral infection containing data on dynamic changes and differential expression profiles of genes in viral-infected patients (48).
The current study aimed at the analysis of transcriptomics data for viral diseases focusing on causes for alteration of immune functions. We used the following two criteria for selecting viruses for the analysis. First, we chose the viruses based on their significance to human health, particularly the harm they can cause due to immune dysfunction. Second, we chose the viruses for which there are publicly available transcription level data of blood cells obtained from both viral-infected and uninfected people who did not take any antivirals. Consequently, we obtained transcriptomic data sets of nine viruses. We compared the transcription profiles in blood cells between pathologies caused by nine viruses (49–55). They all cause immune dysfunction, and transcription changes in blood cells may reflect alterations in their functions. Since human peripheral blood cells are typically involved in the host response to a particular viral infection, we suppose that the observed changes in the level of transcription of these cells can represent the most significant peculiarities of immune response to the infection. The corresponding transcriptomics data, measured under the same conditions, including cell types, are available in public databases. We used originally developed pipeline for analysis of transcriptome data collected from patients with several viral infections to reach deep understanding of molecular mechanisms leading to immune dysfunction that can be common for the analyzed infections. The pipeline includes the following steps: (1) collection of transcriptome data; (2) data processing and normalization; (3) data analysis and search for master regulators that can be responsible for altered immune response. In contrast to earlier published studies, we identified not only common differentially expressed genes (DEGs), variations in the interplay of pathways, but also master regulators (MRs), which are the proteins at the top of signaling pathways responsible for the observed transcription changes. Particularly, we found receptors for cytokines, growth factors, hormones, and mediators, which are potentially important regulators for altered immune functions in most of viral diseases caused by nine viruses.
2 Materials and methods
2.1 Collection of transcription datasets
We performed a rigorous search across two transcriptomics databases: Gene Expression Omnibus1 (GEO), and ArrayExpress2 using the following query: “(virus OR viral) AND (lymphocytes OR “B cells” OR “T cells” OR monocytes OR “NK cells” OR PBMC OR “peripheral blood mononuclear” OR neutrophils OR “whole blood”).” We selected datasets containing data on gene transcription in blood cells measured by microarrays or RNA sequencing and obtained from both viral-infected and uninfected people. We selected only datasets obtained from people who did not take antiviral therapeutics. In total, we identified 112 data sets, which were different by the virus, cell type, and experimental method. The most frequently used cell type was PBMC. The datasets related to these cells contain transcription profiles from patients infected by SARS-CoV-1, SARS-CoV-2, DENV, HIV-1, HTLV-1, HBV, HCV, CMV, and EBV.
2.2 Genome enhancer pipeline
2.2.1 Main steps of the analysis
To identify DEGs and MRs, we used the Genome Enhancer tool3 developed by geneXplain GmbH (56). Briefly, Genome Enhancer implemented a pipeline including four main steps:
1. Pre-processing of gene transcription data and identification of DEGs.
2. Identification of the regulatory regions (promoters and enhancers) of the DEGs;
3. Analysis of the regulatory regions of DEGs to predict transcription factor binding sites (TFBSs), identify those enriched in promoters and enhancers of DEGs compared to genes with unchanged transcription, and predict the combinations of TFBSs called “composite regulatory modules” (CRM), which may reflect complexes of co-acting TFs;
4. Reconstruction of the signaling network that activates these TFs and identify MRs at the top of the network.
2.2.2 Pre-processing of gene transcription data and identification of differentially expressed genes
The initial files downloaded from GEO were transformed to tables using functions from Affy and limma R packages, including ReadAffy, read.idat and read.maimages for Affymetrix, Illumina and Agilent platforms, correspondingly. All RNA sequencing datasets were presented as read counts tables and did not require transformation. All microarray datasets were normalized using rma, neqc, and normalizeBetweenArrays functions, for Affymetrix, Illumina and Agilent platforms, correspondingly. Obtained tables were loaded into Genome Enhancer pipeline.
The first stages of Genome Enhancer pipeline are related to additional data processing. Since subsequent stages of the pipeline and comparison of viral-induced transcription profiles between different microarray platforms require data at the level of genes instead probes, several probe intensity values were merged into single value. If the gene was associated with two or more probe intensity values, the maximal value was chosen. As a result, microarray probe ids were converted to ensemble gene ids. All table were additionally normalized using quantile normalization method.
The DEGs were identified between viral disease samples and healthy controls for each of the nine viruses separately. Genome Enhancer allowed identifying DEGs using limma R package for microarray data and edgeR package for RNA sequencing data. For each dataset, the top 300 up-regulated and top 300 down-regulated genes were used for subsequent steps of Genome Enhancer pipeline (see Sections 2.2.3, 2.2.4, 2.2.5).
To perform pathway enrichment analysis and compare transcription profiles between viral infections at the level of particular genes, we defined up- and down-regulated genes with absolute values of log fold changes (log2 values) exceeded 0.5 and p-values less than 0.05. We did not use Benjamini–Hochberg or Bonferroni correction for multiple hypothesis testing because no DEGs for DENV, HTLV-1 and HIV-1 infections were found with any thresholds on adjusted p-values. Moreover, different microarray platforms allow measuring transcription of different numbers of genes, and corresponding correction will be different for each transcriptional dataset that may introduce additional bias into results of analysis.
2.2.3 Identification of the regulatory regions of DEGs
The regulatory regions of DEGs were considered as promoter sequences of length of 1100 base pairs (bp): from 1000 bp before transcription start site (TSS) to 100 bp after TSS
2.2.4 Identification of transcription factors and composite regulatory modules
The identified regulatory regions were then scanned for TFBS and their combinations – CRM, which were enriched in promoters of top 300 up- and top 300 down-regulated genes compared to genes whose transcription was not changed. The TFBS were predicted using positional weight matrices from the TRANSFAC database (57), the CRM were identified using Composite Module Analyst algorithm (58). The corresponding analysis was performed for up- and down-regulated genes separately.
2.2.5 Identification of master regulators
To identify MRs in signaling network, upstream of the identified transcription factors, the comprehensive network from TRANSPATH database (59) and “Upstream Analysis” algorithm (60, 61) were used. TRANSPATH database contains directed interactions between proteins, e.g., the directed interaction between two kinases where the first kinase phosphorylates the second kinase. “Upstream Analysis” algorithm scores potential MRs according to their closeness in network to transcription factors. As a result, we identified two lists of potential MRs: for up- and down-regulated genes, correspondingly.
To identify receptors among revealed MRs, we retrieved a list of receptors from the OmniPath database (62) using the OmnipathR R package.
2.3 Pathway enrichment analysis
To identify KEGG pathways4 that were differentially regulated in PBMC from a particular viral infection-related group of patients compared to healthy control, we performed pathway enrichment analysis (63) using the “enrichr” function from the “enrichR” R package (64). Function “enrichr” allows identifying pathways “enriched” with DEGs compared to the random gene sets. It means that the percent of genes from studied gene list participating in particular pathway is statistically significantly higher than the percent of genes from a random gene set. We performed the corresponding analysis for up- and down-regulated genes separately. For further analysis we selected pathways associated with at least three DEGs and with an adjusted p-value, calculated by Benjamini–Hochberg method, less than 0.05.
2.4 Comparison of KEGG pathways, functionally related groups of genes, and master regulators between viral infection diseases
To compare lists of KEGG pathways, manually selected groups of genes, and MRs obtained for infectious diseases caused by nine viruses, we created heatmaps using the “pheatmap” R package5.
3 Results
3.1 Data on viral-related gene transcription in peripheral blood mononuclear cells
We performed a comprehensive search across transcriptomics databases and selected 10 transcription datasets from the Gene Expression Omnibus (GEO) database containing data on gene transcription in PBMC from healthy people and patients infected by one of the nine viruses (see Table 1). The details are provided in the Materials and Methods section. Some datasets contain samples from patients with a different form of viral infection disease, e.g., different severity (DF or DHF, severe or moderate COVID-19), or duration of disease (acute or latent EBV and CMV infections). Further analysis was performed separately for each of these viral infection-related groups. We defined “viral infection-related group” as a group of samples obtained from a specific transcription dataset and related to the viral infection in a particular form (e.g., severe or moderate, acute or chronic, symptomatic or asymptomatic).
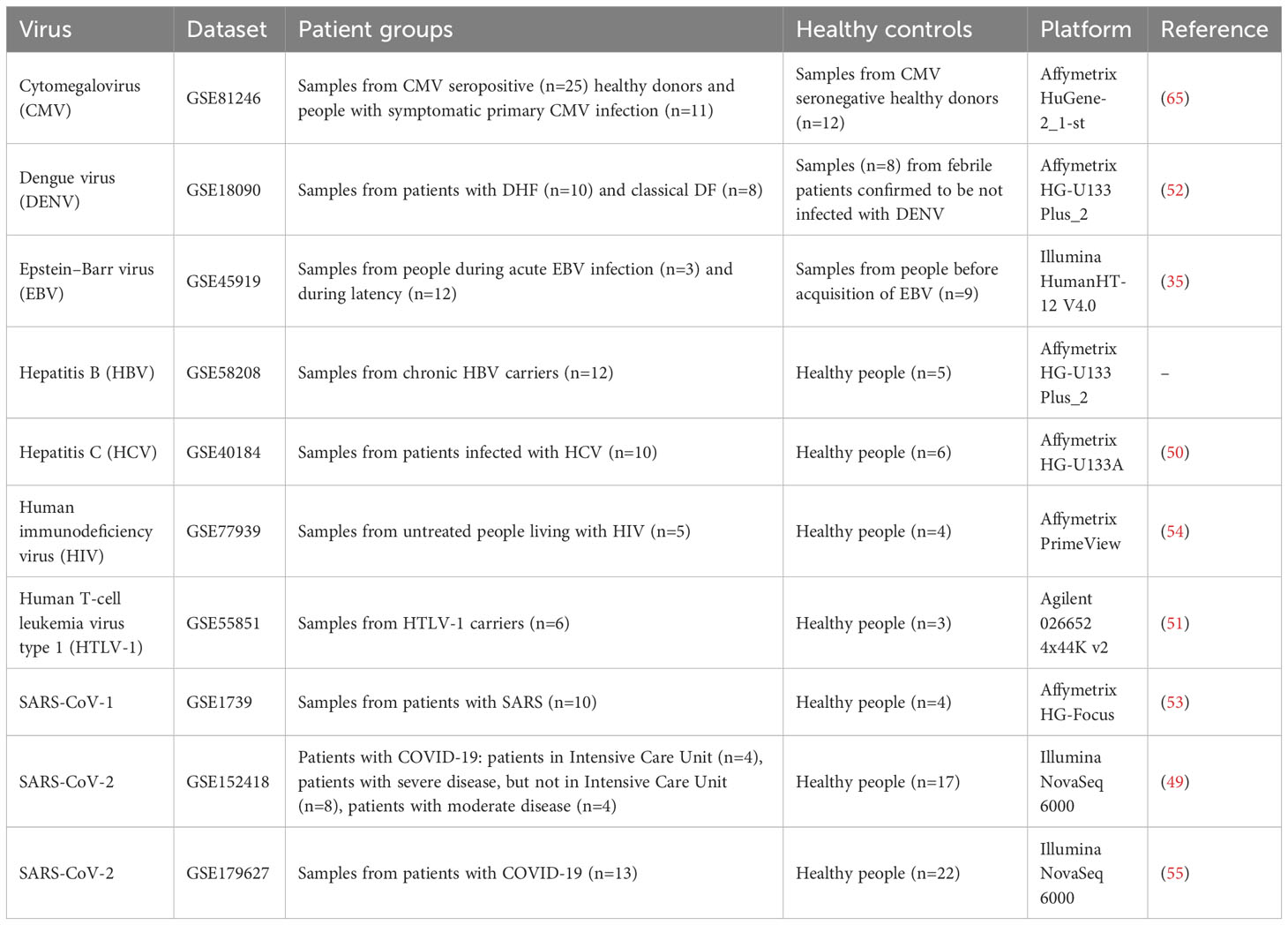
Table 1 Transcription datasets with information on gene transcription in PBMC derived from healthy people and patients infected by one of 9 viruses.
Up-regulated genes for each viral infection-related group (Table 2) were defined as having log fold changes more than 0.5 and p-values less than 0.05. Down-regulated genes for each viral infection-related group were defined as having log fold changes less than -0.5 and p-values less than 0.05. These thresholds were selected empirically to balance the numbers of DEGs and their statistical significance (see section 2.2.2). In total, 16129 DEGs were identified. The full lists of DEGs presented in Tables S1, S2.
Since most of viruses trigger the type I interferon system, we compared the obtained lists of up-regulated genes with the list of 50 interferon-stimulated genes (ISGs) from review of John Schoggins and Charles Rice (66) as a control (see Table S3). We calculated Tanimoto coefficients to estimate the gene lists intersections and performed permutation tests to estimate their statistical significance. The intersections of up-regulated genes with known ISGs were significantly high for the most of viral infection-related groups except CMV_pos, HTLV_1, SARS and COVID19_ICU (Table 2) (see Discussion for details). Figure 1 shows the ISGs that were up- or down-regulated in at least four viral infections.
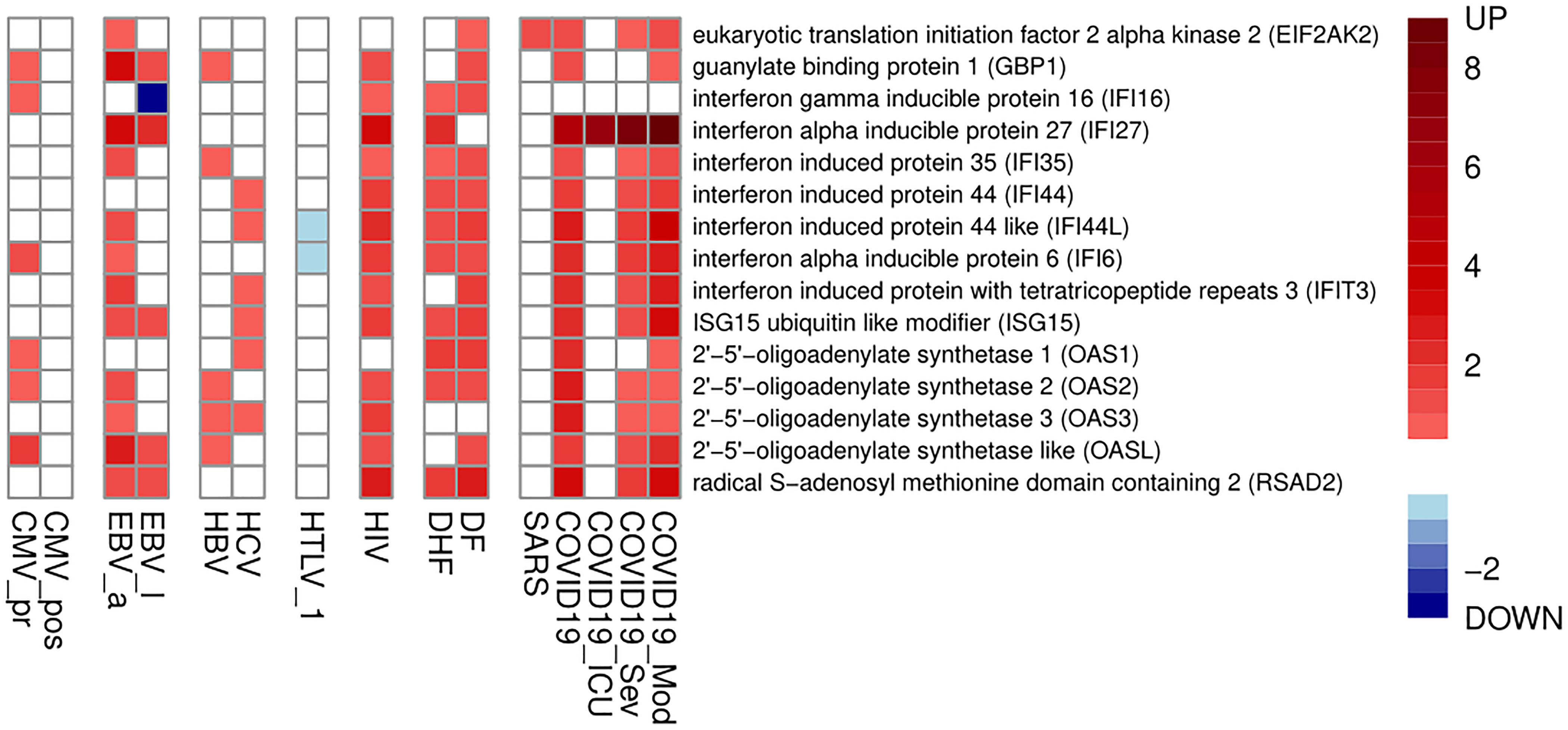
Figure 1 Interferon-stimulated genes, which are up- or down-regulated in at least four viral infections. The color legend shows logarithm of fold change of gene expression.
We considered a gene up- or down-regulated by a particular viral infection if it was up- or down-regulated in at least one of viral infection-related groups, e.g., DF or DHF. We found no genes that were up- or down-regulated by all nine or eight viral infections, and there were only four genes (B4GALT5, CEBPD, H2AC8, PLAUR) differentially regulated in seven viral infections, whereas direction of changes of their expression (up- or down-regulation) depended from particular infection (see Table S2). The observed small intersections between DEGs may be explained by differences in the pathogenesis of viral infections and differences in experimental methods, microarray platforms, and batch effects. We suggested analysing the similarity of viral infections at the level of both individual genes and biological pathways.
3.2 Identification for individual genes and biological pathways that are common for different viral infections
We performed a pathway enrichment analysis (see Materials and Methods) to identify KEGG pathways, which are up- or down-regulated in PBMC during studied viral infections. We considered a pathway to be “enriched” with up- or down-regulated genes, if at least three genes from studied gene list were in the pathway and corresponding proportion of genes was statistically significantly higher than the proportion from a random gene set (adjusted p-value less than 0.05). A pathway was considered as differentially regulated by a particular viral infection if it was differentially regulated in at least one viral infection-related group regardless of the direction of regulation (up- or down-regulation). We created a heatmap (Figure 2) demonstrating pathways, which were differentially regulated by at least three of nine viral infections. The pathways in the Figure 2 were grouped by categories from KEGG PATHWAY database. The full list of pathways is presented in Table S4.
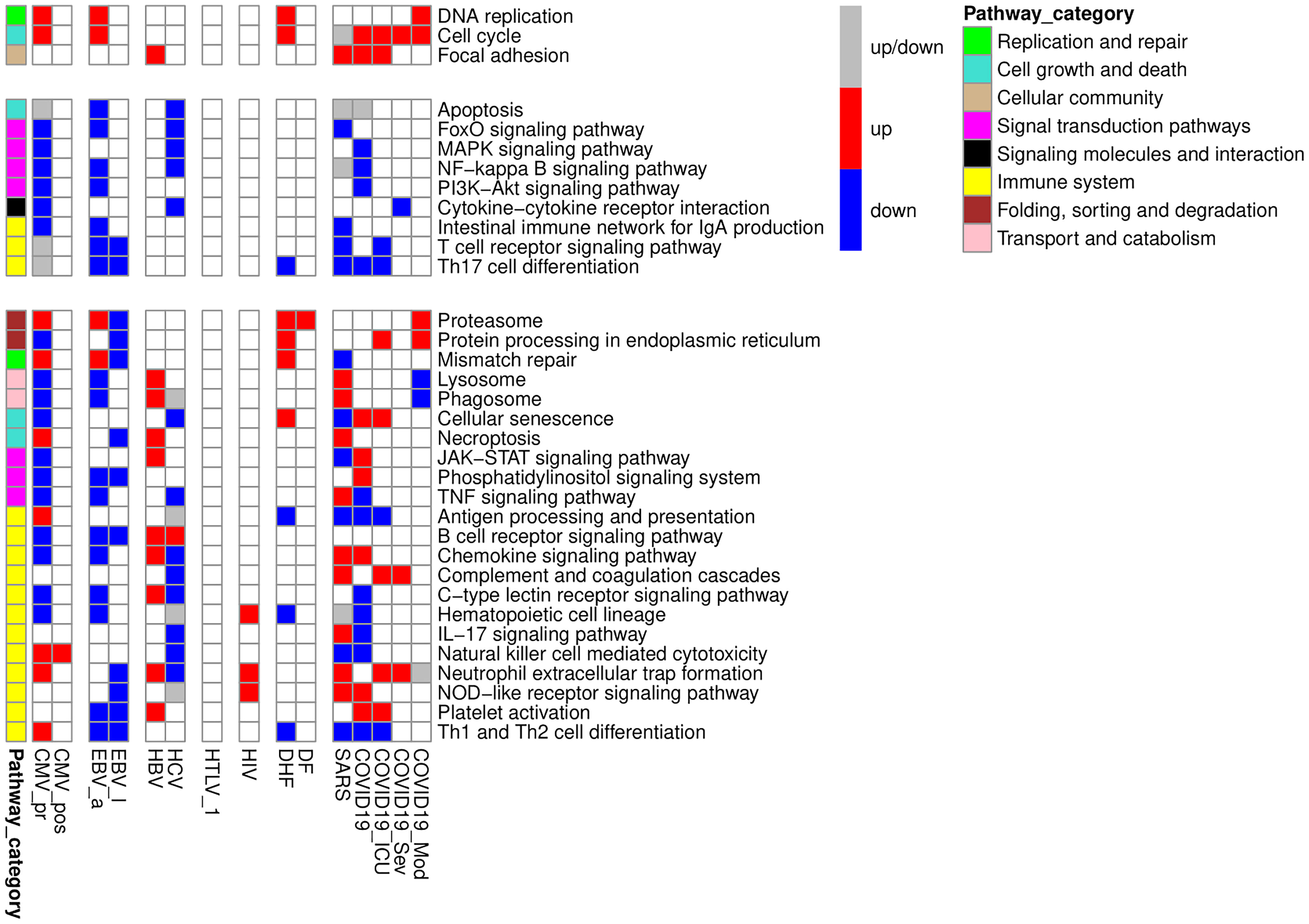
Figure 2 KEGG pathways, up- or down-regulated in PBMC by at least three viral infections. The figure is divided into three parts containing genes which are only up- or down-regulated by viral infections, and genes with mixed pattern of expression changes. The red color of the cell means that the pathway is enriched by up-regulated genes, the blue color of the cell means that the pathway is enriched by down-regulated genes, grey color of the cell means that the pathway is enriched by both up- and down-regulated genes. Pathway category is a related group of pathways according to pathway classification from KEGG PATHWAY database.
We manually investigated the positions of DEGs in the pathway maps and selected genes, which characterize the functional states of PBMC in studied viral infections. Since not all DEGs were presented in KEGG pathways, we also manually investigated functions of genes, which were up- or down-regulated in five or more viral infections, using information from Uniprot database6. We found that most of up-regulated genes belong to two large groups: genes related to cell division and immune-specific functions. Genes related to cell division include key regulators of cell cycle (e.g., cyclins and cyclin-dependent kinases), checkpoint kinases, centrosome, cytoskeletal and motor proteins, enzyme required for DNA replication and repair. The down-regulated genes include immune-related genes and genes related to the processing of genetic information: transcription factors, regulators of splicing, RNA modification and translation.
We manually divided the DEGs into smaller functional groups reflecting functional state of immune cells (Figure 3). Along with key regulators of cell cycle and apoptosis, Figure 3 contains key genes, which are well known positive and negative regulators of B, T, and NK cells activation. Table 3 contains data on additional immune-related genes, whose relationships to viral infections are not as well described, and which are up- or down-regulated by at least five viral infections.
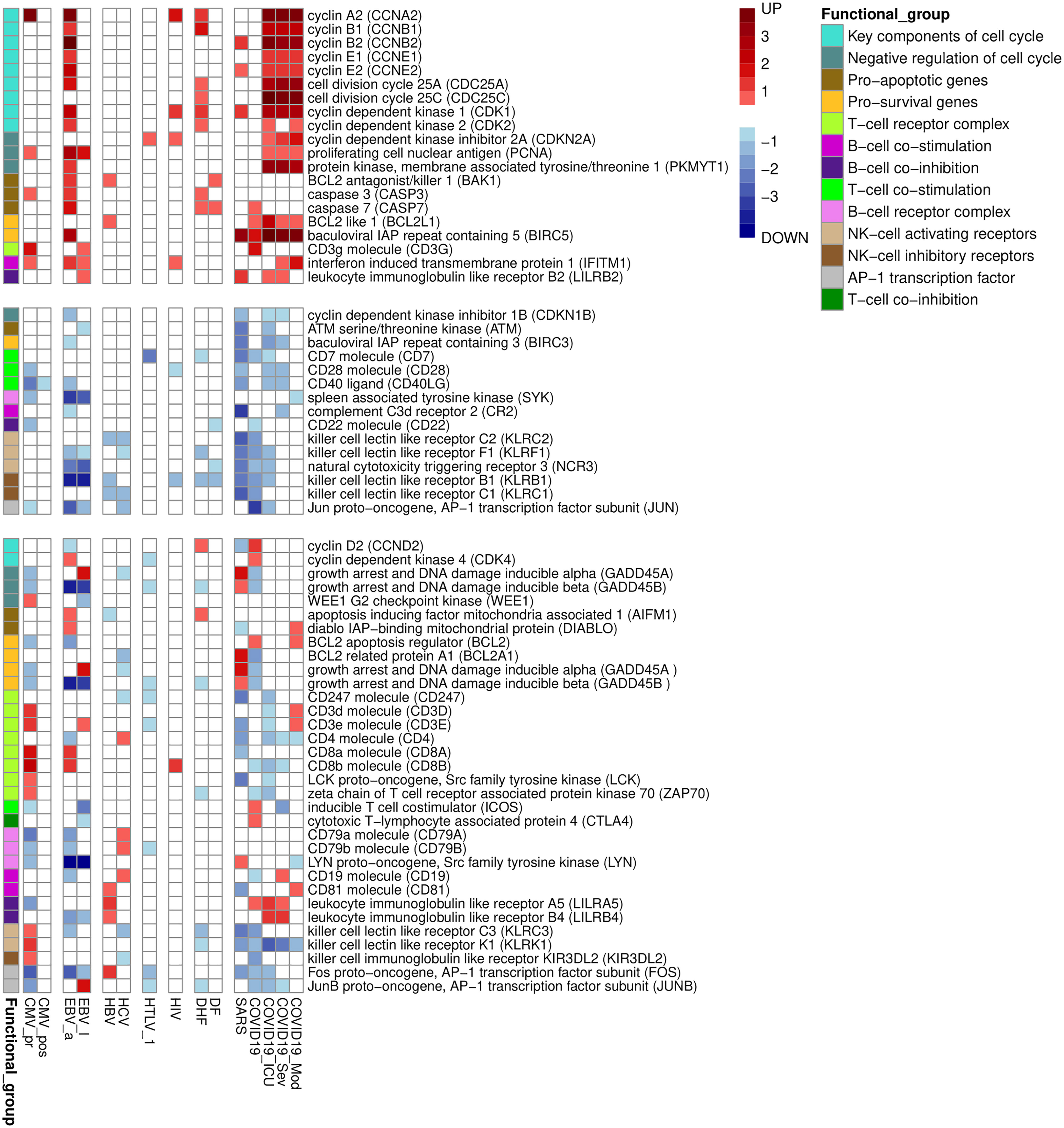
Figure 3 Functionally related groups of genes, up- or down-regulated in PBMC by at least three viral infections. The figure is divided into three parts containing genes which are only up- or down-regulated by viral infections, and genes with mixed pattern of expression changes. The color legend shows logarithm of fold change of gene expression. The functional group is a group of genes related to the particular cell process in PBMC.
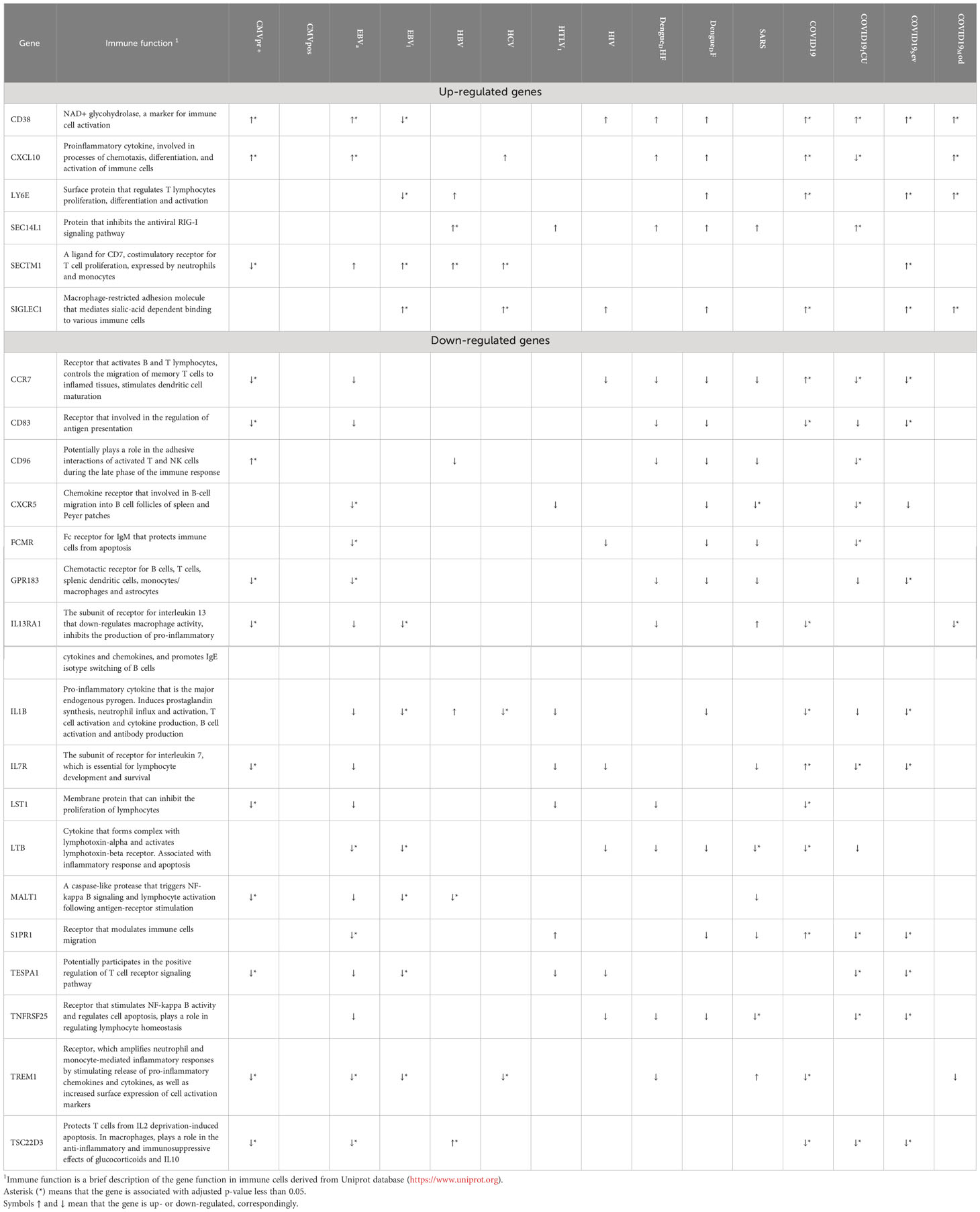
Table 3 Immune-related genes, which are up- or down-regulated in PBMC by at least five viral infections.
Overall, Figures 1-3 and Table 2 demonstrate that PBMC associated with most of viral infection-related groups are characterized by increased proliferation, apoptosis, and altered immune functions (see the Discussion section for details).
3.3 Identification for receptors - master regulators that are common for different viral infections
We identified MRs using the Genome Enhancer pipeline (see Materials and Methods). MRs are the proteins at the top of the signalling network regulating the activity of transcription factors and their complexes and, in turn, are responsible for induction or maintenance of expression changes observed in PBMC. Most obtained MRs are intracellular “hubs,” such as kinases, phosphatases, ubiquitin ligases, GTPases, and transcription factors. We focused on receptors because their interaction with the corresponding ligands is the first of the consequent events leading to gene transcription changes. We selected 56 receptors identified as MRs for at least six viral infections. The receptors were grouped according to their belonging to families and functional similarities (Figure 4). Figure 4 also demonstrates that MRs are up- or down-regulated themselves in part of viral infection-related groups. The full lists of transcription factors and MRs obtained by Genome Enhancer pipeline are presented in Tables S5, S6.
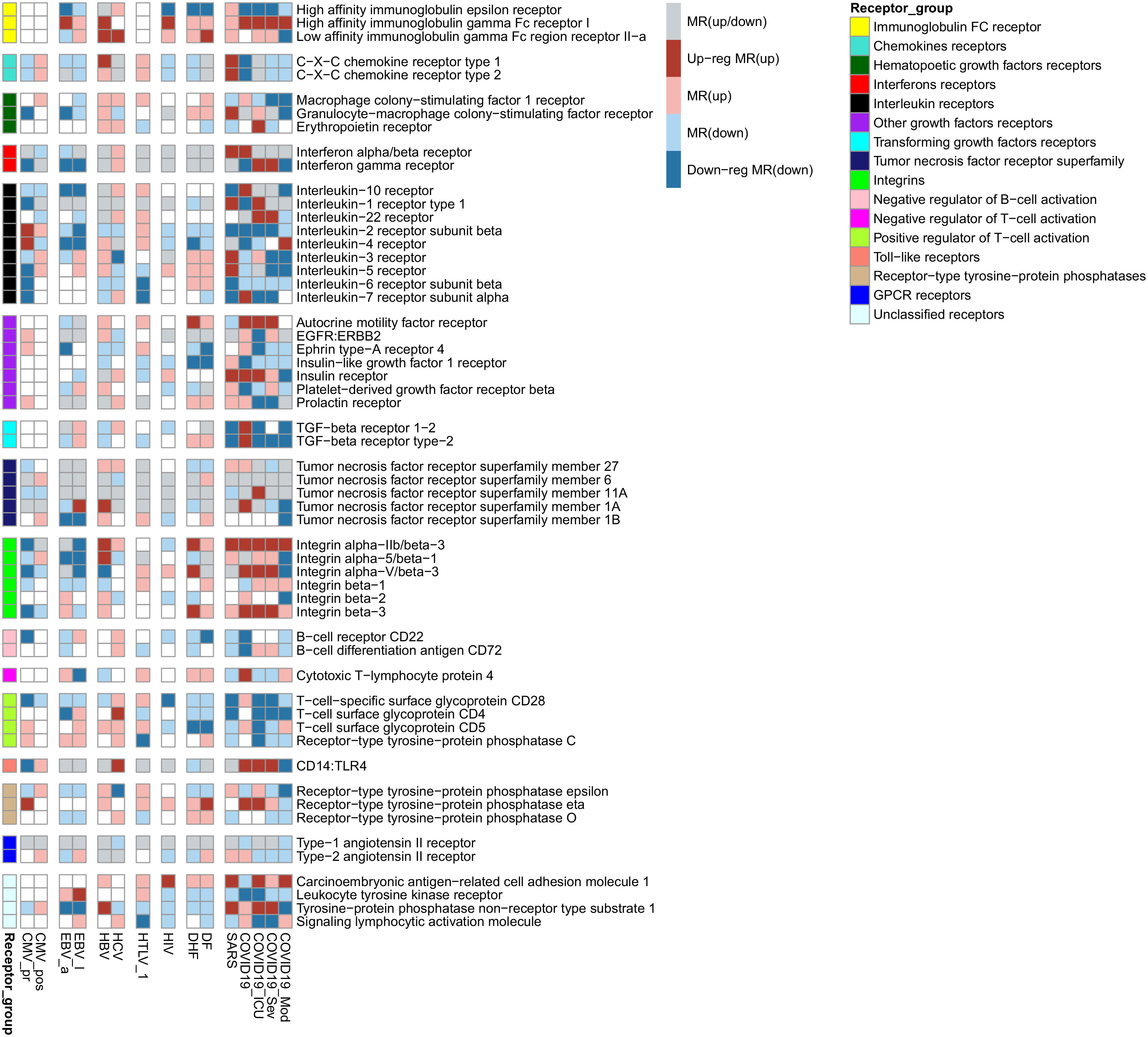
Figure 4 Receptors were identified as MRs for observed transcription changes in PBMC for at least six viral infections. The red color of cells means that the MR is responsible for the observed up-regulation of genes, dark red color means that the MR is responsible for the observed up-regulation of genes and is up-regulated itself, blue color means that the MR controls the observed down-regulation of genes, dark blue color means that the MR is responsible for the observed down-regulation of genes and down-regulated itself, grey color means that the MR controls both up- and down-regulation of genes.
4 Discussion
To identify common molecular mechanisms associated with alteration of immune functions caused by both similar and different viral infections, we compared the transcription profiles in PBMC between pathologies caused by nine viruses: SARS-CoV-1, SARS-CoV-2, DENV, HIV-1, HTLV-1, HBV, HCV, CMV, and EBV. We estimated corresponding mechanisms at the levels of (i) smaller groups of functionally related genes (Figures 1, 3), (ii) individual immune-related genes (Table 3), (iii) biological pathways (Figure 2), and (iv) receptors, the MRs that are the proteins at the top of signalling pathways responsible for the observed transcription changes (Figure 4).
The identified DEGs for each viral infection-related group reflect disease severity (DF or DHF, severe or moderate COVID-19) or duration (acute or latent EBV and CMV infections). There were hundreds DEGs for all groups except latent CMV infection that may indicate significant changes in functional states of immune cells in the corresponding pathologies. Interferon response has been shown to be important for immune-mediated defense process in viral infections that correspond to the earlier findings (47). Therefore, we checked the presence of ISGs among DEGs (Figure 1 and Table 2). We found that most of viral infection-related groups of genes were associated with up-regulation of ISGs. The acute infections such as DF, EBV acute infection, untreated HIV infection and COVID-19, are associated with more up-regulated ISGs comparing to EBV, CMV and HTLV-1 latent infections. On the other hand, PBMC from patients with SARS and COVID-19 treated in Intensive Care Unit (ICU) are not associated with ISGs up-regulation (Figure 1). It was previously shown that type I interferon deficiency is associated with more severe COVID-19 (67). Such observations may indicate that the role of ISGs is significant in acute immune response but the interferon-induced immune response may decrease in time that leads to the development of severe pathology that requires ICU.
Pathway enrichment analysis allowed us to identify KEGG pathways associated with each viral infection-related group (Figure 2). Through the manual analysis of DEGs positions in the corresponding pathway maps, we identified the small groups of functionally related genes responsible for the regulation of the cell cycle, apoptosis, and immune cell activation and exhaustion (Figure 3). Figures 2, 3 demonstrate that all viral infections are associated with changes in these processes. The results obtained are in agreement with the known data on the mechanisms of the immune response to the studied viral infections. Antiviral response includes both non-specific innate immune response and adaptive immune response components (68). The innate immune response is typically mediated by the interferon activity (68), Toll-like receptors (TLRs), RIG-1 receptors (RLRs) signalling pathways and TANK-binding kinase 1 (TBK1) pathway (69). The TLR and TBK-1 pathways are involved in the molecular mechanisms of a viral infection during the early phase. Higher expression levels of interferons 1, 3, and 7 are shown in later stages and are responsible for the inflammation caused by a viral infection (68). The role of TLRs was also shown in activation of pro-apoptotic pathways in infected cells (70). Along with innate immune response, some specific molecular mechanisms are associated with each viral infection. In our study, we suggested that the changes in the transcription levels may reflect the pathways and molecular mechanisms involved in each specific viral infection and compared our results with earlier observations.
Primary CMV infection is known to cause lymphocyte activation and apoptosis, lymphocytosis, and immune exhaustion (25, 29, 65) and therefore it is associated with transcriptional changes that reflect the functional states of immune cells. It was demonstrated that in addition to the activation of pro-apoptotic mechanisms mediated by TLRs, CMV-infection also leads to the changed expression of the cellular anti-apoptotic protein Mcl-1, a member of bcl-2 family (71). This process, in turn, results in an apoptosis of monocytes. HIV infection is associated with the up-regulation of cyclins A, B, and E. Since PBMC are non-synchronized in cell cycle phases, this observation may indicate cell cycle arrest in the G2 phase (72, 73). Down-regulation of the co-stimulation receptor CD28 and increased transcription of the immune checkpoint CTLA4 indicates that HIV infection causes activation and exhaustion of T-lymphocytes. The “exhausted” state of T-cells is characterized by increased expression of immune checkpoints, disrupted proliferation, decreased functions, and increased apoptosis (28, 72). Chronic HCV infection is associated with the disruption of the NK cells functions and the exhaustion of immune cells (74, 75). The number of DEGs related to above mentioned processes increase with disease severity. The DHF is associated with more DEGs related to apoptosis and decreased NK cell functions than DF. It was demonstrated that HTLV-1 can interact with components of NF-kappa B pathway, leading to deregulation of apoptosis (76). Another signalling pathway affected by HTLV-1 is AP-1 signalling pathway, whose components control cell activation and proliferation (76). SARS and severe COVID-19 associated with more transcription changes related to T, B and NK cells functions alterations than moderate coronavirus infection. The T cells are dysregulated through CD147 and receptor tyrosine kinase AXL (77). In particular, the expression of these two molecules was shown to be increased in CD4+/CD8+ T-cells in peripheral blood (77).
Further we provide some examples of genes (Table 3), which are up- or down-regulated by at least five viral infections, and involved in the host immune response to several different viral infections. The relationships of these genes to viral infections are less studied, and they represent new findings. The most of them are down-regulated by viral infections, but some of them are up-regulated. In particular, up-regulated lymphocyte antigen 6 family member E (LY6E) is a surface protein that regulates host immune response including enhancement of T-lymphocytes activation and proliferation (78). LY6E inhibits entry of SARS-CoV, SARS-CoV-2 and MERS-CoV into cells by interfering with spike protein-mediated membrane fusion (79), but promotes entry of HIV-1, and DENV. C-X-C motif chemokine ligand 10 (CXCL10) is involved in proinflammatory signal pathways, including chemotaxis, differentiation, and activation of immune cells, and immune response to viral infections (80, 81). The corresponding gene is up-regulated in five viral infections (Table 3). It is known that the increased level of CXCL10 is associated with COVID-19 severity (82). Earlier studies also confirm the involvement in immune response for several molecules that correspond to DEGs revealed in our study, including SEC14 like lipid binding 1 (SEC14L1). C-C motif chemokine receptor 7 (CCR7), and CD83 (83–85). CD96 downregulates inflammatory and cytokine response causing by NK cells (86). It is interesting, the expression of CD96 is decreased in some samples obtained from patients infected with DENV, coronaviruses SARS and SARS-CoV-2 and HBV. Therefore, one may suggest that for these infections cytokine inflammatory response can be caused by inhibition of CD96 function among some other signal pathways. The proteolytic activity of MALT1 paracaspase is required for T cell activation (87). It also plays role in reactivation of HIV-1 (88). It was demonstrated that MATLT1 inactivates NEDD4-binding protein 1 (N4BP1) that inhibits HIV-1 development in primary T cells and macrophages (88).
Therefore, the identified molecules can be considered as the biomarkers for the severity of viral disease progression. Also targeting molecules by direct interaction or modulation by gene expression may be considered as one of novel strategies for search for non-specific antiviral agents.
Since the observed transcriptional changes are associated with well-known immunopathogenesis of studied viral infections, we searched for MRs, which are the proteins at the top of signaling networks and are responsible for the maintenance of observed transcriptional changes. Our study focused on receptors because their interaction with corresponding ligands is the first of the consequent events leading to gene transcription changes. We identified many cytokines and growth factors receptors, which are common for studied viral infections (Figure 4). According to Human Proteins Atlas data7, all of them are expressed in PBMC: lymphocytes, monocytes, or NK cells. The revealed receptors are up- or down-regulated themselves in part of viral infection-related groups (Figure 4). It means that they are part of positive feedback loops and are extremely important to maintain the observed transcription profiles.
The revealed receptors differ in the novelty of their relationships with the functional states of studied immune cells, they can be divided into three groups by this criterion. The receptors of the first group that include interferons alpha and gamma, and interleukins 1-10, have a clear relationship with functional states of PBMC. They are essential for cell activation, differentiation, survival, and proliferation. The role in immune response of the receptors belonging to the second group is under active investigation. Such receptors include immunoglobulin gamma and epsilon receptors and C-X-C chemokine receptors type 1 and 2 (receptors for interleukin 8). For example, CXCR1 and CXCR2 genes encode receptors for interleukin 8, which is well known as a potent neutrophil chemotactic factor. The CXCR1 receptor is also expressed on the cell surface of T cells, and interleukin 8 treatment results in a reduction in the activation status of both effector memory T cells and terminally differentiated effector T cells (89). Interleukin 8 is also an essential chemotactic factor for NK cells (90) and reduced expression of CXCR1 is associated with decreased counts of NK cells in lymph nodes and incomplete control of HIV viral replication during the early stages of the disease (91). The FCGR2A gene encodes low-affinity immunoglobulin gamma Fc region receptor II-a that binds to the Fc region of immunoglobulins gamma and promotes phagocytosis of opsonized viral particles (92, 93). Polymorphisms in the FCGR2A gene are associated with severity and outcome of viral infections such as COVID-19 (94), Influenza A (95), and HIV infection (96, 97). Surprisingly, we also identified high-affinity immunoglobulin epsilon receptor, which is well known to be expressed in mast cells and basophils and is responsible for initiating the allergic response. However, it was recently shown that CD4(+) T cells also express immunoglobulin epsilon receptors that induces production of interleukin 6 and interferon-gamma but reduces production of interleukin 10 (98). Polymorphism in the FCER1A gene, which encodes the alpha subunit of the receptor, is associated with the efficacy of HBV infection treatment by peginterferon alfa-2a (99).
The third group of the revealed receptors are not specific to the immune system, and their associations with immune response are not studied enough. For instance, the role of Ephrin type-A receptor 4 is well described for the nervous system; however, it is also expressed on memory T cells and is responsible for their migration (100). The insulin receptor is essential for normal adaptive immune function through modulating T cell nutrient uptake and associated glycolytic and respiratory capacities (101). Moreover, following insulin binding, the insulin receptor translocates to the nucleus, where it plays a crucial role in regulating the transcription of various immune-related genes, including pathways involved in viral infections. Therefore, diabetes with insulin resistance can directly contribute to an inadequate immune response, e.g., observed during COVID-19 (102) and some other severe acute viral infections. Prolactin and its receptors play an important role in regulating innate and adaptive immune responses (103), particularly in T lymphocyte growth and activation (104, 105). Stimulation of angiotensin AT1 receptors is important for T cell activation and adhesion/transmigration through the basal endothelial membrane, whereas angiotensin AT2 receptors limit this effect (106, 107). AMFR gene encodes the receptor for autocrine motility factor, the protein with multiple functions. It is little known about its functions in the immune cell. Particularly, the autocrine motility factor stimulates immunoglobulin secretion by cultured human PBMC (108).
We revealed new untrivial associations between the human genes and alterations in immune response, which are common for several viral infections. Nevertheless, this study has some limitations. First, the transcription datasets used in the study (Table 1) are highly heterogenic. They may contain unknown or hidden confounders that may influence the results. Such demographic characteristics as age, gender and geographic region cannot be taken into account in our study because the corresponding information is not available for the most of used datasets. Some datasets were coming from RNAseq studies, some others - from microarrays of different platforms allowing measuring transcription of different numbers of genes. This issue might be solved using normalization procedure, however different number of target genes in microarray and RNAseq studies comprises the limitation of the study. The heterogeneity of datasets may have introduced some biases in the obtained results. Second, the results of the analysis represent rather statistical than causal relationships. The causal nature of revealed relationships can be analysed using additional experiments in cell cultures or animals. Third, the results were obtained at the level of transcripts, however the analysis of the host response to viral infections at the protein level is currently restricted due to the lack of proteome experimental data. Nevertheless, identified DEGs and MRs can be considered as the key features common for several viral infections and can be used as a basis for the development of novel therapeutic strategies for pathogenesis-directed antiviral therapy. New therapeutic strategies developed using the results of computational analysis may include the use of drugs with pathogenesis-based mechanisms of action, including those that can be found by drug repurposing. In this case, identification of human genes and corresponding proteins, as well as the molecular mechanisms controlled by them, may be useful in the search for new potential therapeutic strategies. Furthermore, identifying common mechanisms among various viral infections may help to understand the possible role of viral infections in the development of other diseases, such as cancer.
5 Conclusions
Since many different viruses currently exist and new viral infections, such as COVID-19, suddenly become spreading among human population, it is challenging to create direct therapeutics for each virus. Additionally, complications can develop rapidly after a person contacts a virus. In such cases pathogenesis-directed therapy may be beneficial for treatment and cure of severe viral infections. Even though significant differences between viral diseases exist, most of them are associated with the dysfunction of the immune system. As a result, the immune system cannot effectively eliminate viruses, which increase disease severity and duration and susceptibility to secondary infections. Thus, it is crucial to identify mechanisms of immune system disruption that are common for different viral infections. In our study, we applied network-based transcriptomics analysis to identify mechanisms of immune function disruption caused by nine different viral infections at the levels of pathways, cellular processes, and master regulators, which are the key proteins responsible for the observed immune states. Analysis of revealed pathways, small groups of functionally related genes and individual differentially expressed genes demonstrated that nine studied viral infections cause immune activation, exhaustion, cell proliferation, and increased susceptibility to apoptosis for peripheral blood mononuclear cells. Additionally, we performed a search for receptors – master regulators, which are at the top of immune cells signalling networks and may be responsible for the maintenance of observed transcriptional changes, and, thus, accountable for the observed changes in immune cell state. Besides well-characterized receptors for interleukins and interferons, we identified receptors whose relationships with virus-induced immune disfunction have not been shown earlier. The receptors for autocrine motility factor, insulin, prolactin, angiotensin II, and immunoglobulin epsilon may be essential for the normal functioning of immune cells during anti-viral response. They regulate immune cell activation, growth, metabolism, migration and secretion of immunoglobulins and cytokines, and may be involved in the maintenance of gene transcription changes, induced by different viral infections and associated with altered immune response. The pharmacological modulation of the receptors may change transcription profiles in immune cells, which may lead to recovery of immune functions and increase the efficacy of anti-viral response. Thus, the revealed receptors may be investigated as potential targets for treating severe viral infections.
Data availability statement
The original contributions presented in the study are included in the article/Supplementary Material. Further inquiries can be directed to the corresponding author.
Ethics statement
As publicly available data was used in this analysis, ethical approval was not required.
Author contributions
SI, OT, and VP contributed to conception and design of the study, and reviewed and prepared the final version of the manuscript. SI and OT prepared transcription datasets. SI wrote R scripts and performed analysis with Genome Enhancer pipeline. SI and OT wrote the first draft of the manuscript. SI, OT and VP reviewed and prepared the final version of the manuscript. All authors contributed to the article and approved the submitted version.
Funding
The study was performed in the framework of the Program for Basic Research in the Russian Federation for a long-term period (2021-2030) (project No. 121102900156-6).
Acknowledgments
We are grateful to the geneXplain GmbH for providing access to the Genome Enhancer pipeline available online at https://ge.genexplain.com.
Conflict of interest
The authors declare that the research was conducted in the absence of any commercial or financial relationships that could be construed as a potential conflict of interest.
Publisher’s note
All claims expressed in this article are solely those of the authors and do not necessarily represent those of their affiliated organizations, or those of the publisher, the editors and the reviewers. Any product that may be evaluated in this article, or claim that may be made by its manufacturer, is not guaranteed or endorsed by the publisher.
Supplementary material
The Supplementary Material for this article can be found online at: https://www.frontiersin.org/articles/10.3389/fimmu.2023.1199482/full#supplementary-material
Supplementary Table 1 | Differentially expressed genes found for particular viral infection-related groups.
Supplementary Table 2 | Summary on differentially expressed genes.
Supplementary Table 3 | The list of 50 interferon-stimulated genes.
Supplementary Table 4 | The full lists of KEGG pathways found for particular viral infection-related groups.
Supplementary Table 5 | Transcription factors are potentially responsible for observed gene expression changes in particular viral infection-related groups.
Supplementary Table 6 | Master regulators are potentially responsible for observed gene expression changes in particular viral infection-related groups.
Footnotes
- ^ https://www.ncbi.nlm.nih.gov/geo
- ^ https://www.ebi.ac.uk/arrayexpress
- ^ https://ge.genexplain.com
- ^ https://www.genome.jp/kegg/pathway.html
- ^ https://CRAN.R-project.org/package=pheatmap
- ^ https://www.uniprot.org
- ^ https://www.proteinatlas.org
References
1. Poropatich K, Sullivan DJ Jr. Human immunodeficiency virus type 1 long-term non-progressors: the viral, genetic and immunological basis for disease non-progression. J Gen Virol (2011) 92:247–68. doi: 10.1099/vir.0.027102-0
2. Lopez-Galindez C, Pernas M, Casado C, Olivares I, Lorenzo-Redondo R. Elite controllers and lessons learned for HIV-1 cure. Curr Opin Virol (2019) 38:31–6. doi: 10.1016/j.coviro.2019.05.010
3. Fadl N, Ali E, Salem TZ. COVID-19: risk factors associated with infectivity and severity. Scand J Immunol (2021) 93:e13039. doi: 10.1111/sji.13039
4. Zhang JJ, Dong X, Liu GH, Gao YD. Risk and protective factors for COVID-19 morbidity, severity, and mortality. Clin Rev Allergy Immunol (2022) 64:90–107. doi: 10.1007/s12016-022-08921-5
5. Bonagura VR, Rosenthal DW. Infections that cause secondary immune deficiency. In: Sullivan KE, Stiehm ER, editors. Stiehm's Immune Deficiencies, 2nd ed. Cambridge, Massachusetts, USA: Academic Press (2020). p. 1035–58. doi: 10.1016/B978-0-12-816768-7.00049-1
6. Griffiths P, Baraniak I, Reeves M. The pathogenesis of human cytomegalovirus. J Pathol (2015) 235:288–97. doi: 10.1002/path.4437
7. Thorley-Lawson DA. EBV persistence–introducing the virus. Curr Top Microbiol Immunol (2015) 390:151–209. doi: 10.1007/978-3-319-22822-8_8
8. Kannagi M, Hasegawa A, Nagano Y, Kimpara S, Suehiro Y. Impact of host immunity on HTLV-1 pathogenesis: potential of tax-targeted immunotherapy against ATL. Retrovirology (2019) 16:23. doi: 10.1186/s12977-019-0484-z
9. Brites C, Grassi MF, Quaresma JAS, Ishak R, Vallinoto ACR. Pathogenesis of HTLV-1 infection and progression biomarkers: an overview. Braz J Infect Dis (2021) 25:101594. doi: 10.1016/j.bjid.2021.101594
10. Ivanov S, Lagunin A, Filimonov D, Tarasova O. Network-based analysis of OMICs data to understand the HIV-host interaction. Front Microbiol (2020) 11:1314. doi: 10.3389/fmicb.2020.01314
11. Guzman MG, Gubler DJ, Izquierdo A, Martinez E, Halstead SB. Dengue infection. Nat Rev Dis Primers (2016) 2:16055. doi: 10.1038/nrdp.2016.55
12. Li C, He Q, Qian H, Liu J. Overview of the pathogenesis of COVID-19 (Review). Exp Ther Med (2021) 22:1011. doi: 10.3892/etm.2021.10444
13. Kausar S, Said Khan F, Ishaq Mujeeb Ur Rehman M, Akram M, Riaz M, Rasool G, et al. A review: Mechanism of action of antiviral drugs. Int J Immunopathol Pharmacol (2021) 35:20587384211002620. doi: 10.1177/20587384211002621
14. Kayali Z, Schmidt WN. Finally sofosbuvir: an oral anti-HCV drug with wide performance capability. Pharmgenomics Pers Med (2014) 7:387–98. doi: 10.2147/PGPM.S52629
15. Ferenci P. New anti-HCV drug combinations: who will benefit? Lancet Infect Dis (2017) 17:1008–9. doi: 10.1016/S1473-3099(17)30486-3
16. Menéndez-Arias L, Delgado R. Update and latest advances in antiretroviral therapy. Trends Pharmacol Sci (2022) 43:16–29. doi: 10.1016/j.tips.2021.10.004
17. Gilmore JC, Serghides L, Bendayan R. Differential effects of antiretroviral drug toxicity in male versus female children who are HIV-exposed but uninfected. AIDS (2021) 35:1–14. doi: 10.1097/QAD.0000000000002707
18. Guimarães NN, de Andrade HHR, Lehmann M, Dihl RR, Cunha KS. The genetic toxicity effects of lamivudine and stavudine antiretroviral agents. Expert Opin Drug Saf (2010) 9:771–81. doi: 10.1517/14740331003702384
19. Fowler MG. Follow-up of children exposed to perinatal antiretrovirals. Teratology (2000) 61:395–6. doi: 10.1002/(SICI)1096-9926(200005)61:5<395::AID-TERA15>3.0.CO;2-H
20. Atta MG, De Seigneux S, Lucas GM. Clinical pharmacology in HIV therapy. Clin J Am Soc Nephrol (2019) 14:435–44. doi: 10.2215/CJN.02240218
21. Nachega JB, Morroni C, Chaisson RE, Goliath R, Efron A, Ram M, et al. Impact of immune reconstitution inflammatory syndrome on antiretroviral therapy adherence. Patient Prefer Adherence (2012) 6:887–91. doi: 10.2147/PPA.S38897
22. Castillo JP, Kowalik TF. HCMV infection: modulating the cell cycle and cell death. Int Rev Immunol (2004) 23:113–39. doi: 10.1080/08830180490265565
23. Malavige GN, Huang LC, Salimi M, Gomes L, Jayaratne SD, Ogg GS. Cellular and cytokine correlates of severe dengue infection. PLoS One (2012) 7:e50387. doi: 10.1371/journal.pone.0050387
24. Antoine P, Olislagers V, Huygens A, Lecomte S, Liesnard C, Donner C, et al. Functional exhaustion of CD4+ T lymphocytes during primary cytomegalovirus infection. J Immunol (2012) 189:2665–72. doi: 10.4049/jimmunol.1101165
25. Slyker JA, Rowland-Jones SL, Dong T, Reilly M, Richardson B, Emery VC, et al. Acute cytomegalovirus infection is associated with increased frequencies of activated and apoptosis-vulnerable T cells in HIV-1-infected infants. J Virol (2012) 86:11373–9. doi: 10.1128/JVI.00790-12
26. Heim MH, Thimme R. Innate and adaptive immune responses in HCV infections. J Hepatol (2014) 61:S14–25. doi: 10.1016/j.jhep.2014.06.035
27. Gogoi D, Borkakoty B, Biswas D, Mahanta J. Activation and exhaustion of adaptive immune cells in hepatitis B infection. Viral Immunol (2015) 28:348–53. doi: 10.1089/vim.2014.0115
28. McLane LM, Abdel-Hakeem MS, Wherry EJ. CD8 T cell exhaustion during chronic viral infection and cancer. Annu Rev Immunol (2019) 37:457–95. doi: 10.1146/annurev-immunol-041015-055318
29. Schönrich G, Raftery MJ. The PD-1/PD-L1 axis and virus infections: A delicate balance. Front Cell Infect Microbiol (2019) 9:207. doi: 10.3389/fcimb.2019.00207
30. Fenwick C, Joo V, Jacquier P, Noto A, Banga R, Perreau M, et al. T-cell exhaustion in HIV infection. Immunol Rev (2019) 292:149–63. doi: 10.1111/imr.12823
31. Luo XH, Zhu Y, Mao J, Du RC. T cell immunobiology and cytokine storm of COVID-19. Scand J Immunol (2021) 93:e12989. doi: 10.1111/sji.12989
32. Yuan S, Jiang SC, Zhang ZW, Fu YF, Hu J, Li ZL. Quantification of cytokine storms during virus infections. Front Immunol (2021) 12:659419. doi: 10.3389/fimmu.2021.659419
33. Holm GH, Gabuzda D. Distinct mechanisms of CD4+ and CD8+ T-cell activation and bystander apoptosis induced by human immunodeficiency virus type 1 virions. J Virol (2005) 79:6299–311. doi: 10.1128/JVI.79.10.6299-6311.2005
34. Álvarez-Mon M, Ortega MA, Gasulla Ó, Fortuny-Profitós J, Mazaira-Font FA, Saurina P, et al. A predictive model and risk factors for case fatality of COVID-19. J Pers Med (2021) 11:36. doi: 10.3390/jpm11010036
35. Dunmire SK, Odumade OA, Porter JL, Reyes-Genere J, Schmeling DO, Bilgic H, et al. Primary EBV infection induces an expression profile distinct from other viruses but similar to hemophagocytic syndromes. PLoS One (2014) 9:e85422. doi: 10.1371/journal.pone.0085422
36. Zhai Y, Franco LM, Atmar RL, Quarles JM, Arden N, Bucasas KL, et al. Host transcriptional response to influenza and other acute respiratory viral infections–A prospective cohort study. PLoS Pathog (2015) 11:e1004869. doi: 10.1371/journal.ppat.1004869
37. Afroz S, Giddaluru J, Abbas MM, Khan N. Transcriptome meta-analysis reveals a dysregulation in extra cellular matrix and cell junction associated gene signatures during dengue virus infection. Sci Rep (2016) 6:33752. doi: 10.1038/srep33752
38. Moni MA, Quinn JMW, Sinmaz N, Summers MA. Gene expression profiling of SARS-CoV-2 infections reveal distinct primary lung cell and systemic immune infection responses that identify pathways relevant in COVID-19 disease. Brief Bioinform (2021) 22:1324–37. doi: 10.1093/bib/bbaa376
39. Nienhold R, Ciani Y, Koelzer VH, Tzankov A, Haslbauer JD, Menter T, et al. Two distinct immunopathological profiles in autopsy lungs of COVID-19. Nat Commun (2020) 11:5086. doi: 10.1038/s41467-020-18854-2
40. Vavougios GD. Overlapping host pathways between SARS-CoV-2 and its potential copathogens: an in silico analysis. Infect Genet Evol (2020) 86:104602. doi: 10.1016/j.meegid.2020.104602
41. Zhou Y, Hou Y, Shen J, Mehra R, Kallianpur A, Culver DA, et al. A network medicine approach to investigation and population-based validation of disease manifestations and drug repurposing for COVID-19. PloS Biol (2020) 18:e3000970. doi: 10.1371/journal.pbio.3000970
42. Ng DL, Granados AC, Santos YA, Servellita V, Goldgof GM, Meydan C, et al. A diagnostic host response biosignature for COVID-19 from RNA profiling of nasal swabs and blood. Sci Adv (2021) 7:eabe5984. doi: 10.1126/sciadv.abe5984
43. McClain MT, Constantine FJ, Henao R, Liu Y, Tsalik EL, Burke TW, et al. Dysregulated transcriptional responses to SARS-CoV-2 in the periphery. Nat Commun (2021) 12:1079. doi: 10.1038/s41467-021-21289-y
44. Barral-Arca R, Gómez-Carballa A, Cebey-López M, Bello X, Martinón-Torres F, Salas AA. Meta-analysis of multiple whole blood gene expression data unveils a diagnostic host-response transcript signature for respiratory syncytial virus. Int J Mol Sci (2020) 21:E1831. doi: 10.3390/ijms21051831
45. Gómez-Carballa A, Barral-Arca R, Cebey-López M, Bello X, Pardo-Seco J, Martinón-Torres F, et al. Identification of a minimal 3-transcript signature to differentiate viral from bacterial infection from best genome-wide host RNA biomarkers: A multi-cohort analysis. Int J Mol Sci (2021) 22:3148. doi: 10.3390/ijms22063148
46. Tsalik EL, Fiorino C, Aqeel A, Liu Y, Henao R, Ko ER, et al. The host response to viral infections reveals common and virus-specific signatures in the peripheral blood. Front Immunol (2021) 12:741837. doi: 10.3389/fimmu.2021.741837
47. Unterman A, Sumida TS, Nouri N, Yan X, Zhao AY, Gasque V, et al. Single-cell multi-omics reveals dyssynchrony of the innate and adaptive immune system in progressive COVID-19. Nat Commun (2022) 13:440. doi: 10.1038/s41467-021-27716-4
48. Tang Z, Fan W, Li Q, Wang D, Wen M, Wang J, et al. MVIP: multi-omics portal of viral infection. Nucleic Acids Res (2022) 50:D817–27. doi: 10.1093/nar/gkab958
49. Arunachalam PS, Wimmers F, Mok CKP, Perera RAPM, Scott M, Hagan T, et al. Systems biological assessment of immunity to mild versus severe COVID-19 infection in humans. Science (2020) 369:1210–20. doi: 10.1126/science.abc6261
50. Bolen CR, Robek MD, Brodsky L, Schulz V, Lim JK, Taylor MW, et al. The blood transcriptional signature of chronic hepatitis C virus is consistent with an ongoing interferon-mediated antiviral response. J Interferon Cytokine Res (2013) 33:15–23. doi: 10.1089/jir.2012.0037
51. Kobayashi S, Nakano K, Watanabe E, Ishigaki T, Ohno N, Yuji K, et al. CADM1 expression and stepwise downregulation of CD7 are closely associated with clonal expansion of HTLV-I-infected cells in adult T-cell leukemia/lymphoma. Clin Cancer Res (2014) 20:2851–61. doi: 10.1158/1078-0432.CCR-13-3169
52. Nascimento EJ, Braga-Neto U, Calzavara-Silva CE, Gomes AL, Abath FG, Brito CA, et al. Gene expression profiling during early acute febrile stage of dengue infection can predict the disease outcome. PLoS One (2009) 4:e7892. doi: 10.1371/journal.pone.0007892
53. Reghunathan R, Jayapal M, Hsu LY, Chng HH, Tai D, Leung BP, et al. Expression profile of immune response genes in patients with Severe Acute Respiratory Syndrome. BMC Immunol (2005) 6:2. doi: 10.1186/1471-2172-6-2
54. Singh S, Toor JS, Sharma A, Arora SK. Signature genes associated with immunological non-responsiveness to anti-retroviral therapy in HIV-1 subtype-c infection. PloS One (2020) 15:e0234270. doi: 10.1371/journal.pone.0234270
55. Zhang J, Lin D, Li K, Ding X, Li L, Liu Y, et al. Transcriptome analysis of peripheral blood mononuclear cells reveals distinct immune response in asymptomatic and re-detectable positive COVID-19 patients. Front Immunol (2021) 12:716075. doi: 10.3389/fimmu.2021.716075
56. Kel A, Boyarskikh U, Stegmaier P, Leskov LS, Sokolov AV, Yevshin I, et al. Walking pathways with positive feedback loops reveal DNA methylation biomarkers of colorectal cancer. BMC Bioinf (2019) 20:119. doi: 10.1186/s12859-019-2687-7
57. Matys V, Kel-Margoulis OV, Fricke E, Liebich I, Land S, Barre-Dirrie A, et al. TRANSFAC and its module TRANSCompel: transcriptional gene regulation in eukaryotes. Nucleic Acids Res (2006) 34:D108–10. doi: 10.1093/nar/gkj143
58. Kel A, Konovalova T, Waleev T, Cheremushkin E, Kel-Margoulis O, Wingender E. Composite Module Analyst: a fitness-based tool for identification of transcription factor binding site combinations. Bioinformatics (2006) 22:1190–7. doi: 10.1093/bioinformatics/btl041
59. Krull M, Pistor S, Voss N, Kel A, Reuter I, Kronenberg D, et al. TRANSPATH: an information resource for storing and visualizing signaling pathways and their pathological aberrations. Nucleic Acids Res (2006) 34:D546–51. doi: 10.1093/nar/gkj107
60. Koschmann J, Bhar A, Stegmaier P, Kel AE, Wingender E. "Upstream analysis": an integrated promoter-pathway analysis approach to causal interpretation of microarray data. Microarrays (Basel) (2015) 4:270–86. doi: 10.3390/microarrays4020270
61. Kel AE, Stegmaier P, Valeev T, Koschmann J, Poroikov V, Kel-Margoulis OV, et al. Multi-omics "upstream analysis" of regulatory genomic regions helps identifying targets against methotrexate resistance of colon cancer. EuPA Open Proteom (2016) 13:1–13. doi: 10.1016/j.euprot.2016.09.002
62. Türei D, Korcsmáros T, Saez-Rodriguez J. OmniPath: guidelines and gateway for literature-curated signaling pathway resources. Nat Methods (2016) 13:966–7. doi: 10.1038/nmeth.4077
63. Khatri P, Sirota M, Butte AJ. Ten years of pathway analysis: current approaches and outstanding challenges. PloS Comput Biol (2012) 8:e1002375. doi: 10.1371/journal.pcbi.1002375
64. Kuleshov MV, Jones MR, Rouillard AD, Fernandez NF, Duan Q, Wang Z, et al. Enrichr: A comprehensive gene set enrichment analysis web server 2016 update. Nucleic Acids Res (2016) 44:W90–97. doi: 10.1093/nar/gkw377
65. Riou R, Bressollette-Bodin C, Boutoille D, Gagne K, Rodallec A, Lefebvre M, et al. Severe symptomatic primary human cytomegalovirus infection despite effective innate and adaptive immune responses. J Virol (2017) 91:e02245–16. doi: 10.1128/JVI.02245-16
66. Schoggins JW, Rice CM. Interferon-stimulated genes and their antiviral effector functions. Curr Opin Virol (2011) 1:519–25. doi: 10.1016/j.coviro.2011.10.008
67. Gozman L, Perry K, Nikogosov D, Klabukov I, Shevlyakov A, Baranova A. A role of variance in interferon genes to disease severity in COVID-19 patients. Front Genet (2021) 12:709388. doi: 10.3389/fgene.2021.709388
68. Zhou H, Tang Y-D, Zheng C. Revisiting IRF1-mediated antiviral innate immunity. Cytokine Growth Factor Rev (2022) 64:1–6. doi: 10.1016/j.cytogfr.2022.01.004
69. Zhao C, Zhao W. TANK-binding kinase 1 as a novel therapeutic target for viral diseases. Expert Opin Ther Targets (2019) 23:437–46. doi: 10.1080/14728222.2019.1601702
70. Salaun B, Romero P, Lebecque S. Toll-like receptors’ two-edged sword: when immunity meets apoptosis. Eur J Immunol (2007) 37:3311–8. doi: 10.1002/eji.200737744
71. Collins-McMillen D, Chesnokova L, Lee B-J, Fulkerson HL, Brooks R, Mosher BS, et al. HCMV infection and apoptosis: how do monocytes survive HCMV infection? Viruses (2018) 10:533. doi: 10.3390/v10100533
72. Ivanov S, Filimonov D, Tarasova O. A computational analysis of transcriptional profiles from CD8(+) T lymphocytes reveals potential mechanisms of HIV/AIDS control and progression. Comput Struct Biotechnol J (2021) 19:2447–59. doi: 10.1016/j.csbj.2021.04.056
73. Poon B, Grovit-Ferbas K, Stewart SA, Chen IS. Cell cycle arrest by Vpr in HIV-1 virions and insensitivity to antiretroviral agents. Science (1998) 281:266–9. doi: 10.1126/science.281.5374.266
74. Yoon JC, Yang CM, Song Y, Lee JM. Natural killer cells in hepatitis C: current progress. World J Gastroenterol (2016) 22:1449–60. doi: 10.3748/wjg.v22.i4.1449
75. Boldeanu MV, Siloşi I, Bărbulescu AL, Sandu RE, Geormăneanu C, Pădureanu V, et al. Host immune response in chronic hepatitis C infection: involvement of cytokines and inflammasomes. Rom J Morphol Embryol (2020) 61:33–43. doi: 10.47162/RJME.61.1.04
76. Peloponese J-M, Kinjo T, Jeang K-T. Human T-cell leukemia virus type 1 Tax and cellular transformation. Int J Hematol (2007) 86:101–6. doi: 10.1532/IJH97.07087
77. Kuklina EM. T lymphocytes as targets for SARS-CoV-2. Biochem (Mosc) (2022) 87:566–76. doi: 10.1134/S0006297922060086
78. Yu J, Liu SL. Emerging role of LY6E in virus-host interactions. Viruses (2019) 11:1020. doi: 10.3390/v11111020
79. Pfaender S, Mar KB, Michailidis E, Kratzel A, Boys IN, V'kovski P, et al. LY6E impairs coronavirus fusion and confers immune control of viral disease. Nat Microbiol (2020) 5:1330–9. doi: 10.1038/s41564-020-0769-y
80. Larrubia JR, Benito-Martínez S, Calvino M, Sanz-de-Villalobos E, Parra-Cid T. Role of chemokines and their receptors in viral persistence and liver damage during chronic hepatitis C virus infection. World J Gastroenterol (2008) 14:7149–59. doi: 10.3748/wjg.14.7149
81. Brownell J, Polyak SJ. Molecular pathways: hepatitis C virus, CXCL10, and the inflammatory road to liver cancer. Clin Cancer Res (2013) 19:1347–52. doi: 10.1158/1078-0432.CCR-12-0928
82. Kesmez Can F, Özkurt Z, Öztürk N, Sezen S. Effect of IL-6, IL-8/CXCL8, IP-10/CXCL 10 levels on the severity in COVID 19 infection. Int J Clin Pract (2021) 75:e14970. doi: 10.1111/ijcp.14970
83. Li MT, Di W, Xu H, Yang YK, Chen HW, Zhang FX, et al. Negative regulation of RIG-I-mediated innate antiviral signaling by SEC14L1. J Virol (2013) 87:10037–46. doi: 10.1128/JVI.01073-13
84. Yan Y, Chen R, Wang X, Hu K, Huang L, Lu M, et al. CCL19 and CCR7 expression, signaling pathways, and adjuvant functions in viral infection and prevention. Front Cell Dev Biol (2019) 7:212. doi: 10.3389/fcell.2019.00212
85. Grosche L, Knippertz I, König C, Royzman D, Wild AB, Zinser E, et al. The CD83 molecule - an important immune checkpoint. Front Immunol (2020) 11:721. doi: 10.3389/fimmu.2020.00721
86. Chan CJ, Martinet L, Gilfillan S, Souza-Fonseca-Guimaraes F, Chow MT, Town L, et al. The receptors CD96 and CD226 oppose each other in the regulation of natural killer cell functions. Nat Immunol (2014) 15:431–8. doi: 10.1038/ni.2850
87. Rebeaud F, Hailfinger S, Posevitz-Fejfar A, Tapernoux M, Moser R, Rueda D, et al. The proteolytic activity of the paracaspase MALT1 is key in T cell activation. Nat Immunol (2008) 9:272–81. doi: 10.1038/ni1568
88. Yamasoba D, Sato K, Ichinose T, Imamura T, Koepke L, Joas S, et al. N4BP1 restricts HIV-1 and its inactivation by MALT1 promotes viral reactivation. Nat Microbiol (2019) 4:1532–44. doi: 10.1038/s41564-019-0460-3
89. Meniailo ME, Malashchenko VV, Shmarov VA, Gazatova ND, Melashchenko OB, Goncharov AG, et al. Direct effects of interleukin-8 on growth and functional activity of T lymphocytes. Int Immunopharmacol (2017) 50:178–85. doi: 10.1016/j.intimp.2017.06.023
90. Maghazachi AA. Role of chemokines in the biology of natural killer cells. Curr Top Microbiol Immunol (2010) 341:37–58. doi: 10.1007/82_2010_20
91. Luteijn R, Sciaranghella G, van Lunzen J, Nolting A, Dugast AS, Ghebremichael MS, et al. Early viral replication in lymph nodes provides HIV with a means by which to escape NK-cell-mediated control. Eur J Immunol (2011) 41:2729–40. doi: 10.1002/eji.201040886
92. Neidich SD, Fong Y, Li SS, Geraghty DE, Williamson BD, Young WC, et al. Antibody fc effector functions and IgG3 associate with decreased HIV-1 risk. J Clin Invest (2019) 129:4838–49. doi: 10.1172/JCI126391
93. Bohländer F, Riehl D, Weißmüller S, Gutscher M, Schüttrumpf J, Faust S. Immunomodulation: immunoglobulin preparations suppress hyperinflammation in a COVID-19 model via FcγRIIA and FcαRI. Front Immunol (2021) 12:700429. doi: 10.3389/fimmu.2021.700429
94. Fricke-Galindo I, Falfán-Valencia R. Genetics insight for COVID-19 susceptibility and severity: A review. Front Immunol (2021) 12:622176. doi: 10.3389/fimmu.2021.622176
95. Zúñiga J, Buendía-Roldán I, Zhao Y, Jiménez L, Torres D, Romo J, et al. Genetic variants associated with severe pneumonia in A/H1N1 influenza infection. Eur Respir J (2012) 39:604–10. doi: 10.1183/09031936.00020611
96. Li SS, Gilbert PB, Carpp LN, Pyo CW, Janes H, Fong Y, et al. Fc gamma receptor polymorphisms modulated the vaccine effect on HIV-1 risk in the HVTN 505 HIV vaccine trial. J Virol (2019) 93:e02041–18. doi: 10.1128/JVI.02041-18
97. Carapito R, Mayr L, Molitor A, Verniquet M, Schmidt S, Tahar O, et al. A FcɣRIIa polymorphism has a HLA-B57 and HLA-B27 independent effect on HIV disease outcome. Genes Immun (2020) 21:263–8. doi: 10.1038/s41435-020-0106-8
98. Wang J, Lindholt JS, Sukhova GK, Shi MA, Xia M, Chen H, et al. IgE actions on CD4+ T cells, mast cells, and macrophages participate in the pathogenesis of experimental abdominal aortic aneurysms. EMBO Mol Med (2014) 6:952–69. doi: 10.15252/emmm.201303811
99. Wei L, Pavlovic V, Bansal AT, Chen X, Foster GR, He H, et al. Genetic variation in FCER1A predicts peginterferon Alfa-2a-induced hepatitis B surface antigen clearance in East Asian patients with chronic hepatitis B. J Viral Hepat (2019) 26:1040–9. doi: 10.1111/jvh.13107
100. Holen HL, Nustad K, Aasheim HC. Activation of EphA receptors on CD4+CD45RO+ Memory cells stimulates migration. J Leukoc Biol (2010) 87:1059–68. doi: 10.1189/jlb.0709497
101. Tsai S, Clemente-Casares X, Zhou AC, Lei H, Ahn JJ, Chan YT, et al. Insulin receptor-mediated stimulation boosts T cell immunity during inflammation and infection. Cell Metab (2018) 28:922–34.e4. doi: 10.1016/j.cmet.2018.08.003
102. van Niekerk G, van der Merwe M, Engelbrecht AM. Diabetes and susceptibility to infections: implication for COVID-19. Immunology (2021) 164:467–75. doi: 10.1111/imm.13383
103. Pereira Suarez AL, López-Rincón G, Martínez Neri PA, Estrada-Chávez C. Prolactin in inflammatory response. Adv Exp Med Biol (2015) 846:243–64. doi: 10.1007/978-3-319-12114-7_11
104. Xu D, Lin L, Lin X, Huang Z, Lei Z. Immunoregulation of autocrine prolactin: suppressing the expression of costimulatory molecules and cytokines in T lymphocytes by prolactin receptor knockdown. Cell Immunol (2010) 263:71–8. doi: 10.1016/j.cellimm.2010.02.018
105. Ewerman L, Landberg E, Hellberg S, Hovland M, Sundin A, Jenmalm MC, et al. Immunomodulating effects depend on prolactin levels in patients with hyperprolactinemia. Horm Metab Res (2020) 52:228–35. doi: 10.1055/a-1126-4272
106. Silva-Filho JL, Souza MC, Henriques M das G, Morrot A, Savino W, Nunes MP, et al. AT1 receptor-mediated angiotensin II activation and chemotaxis of T lymphocytes. Mol Immunol (2011) 48:1835–43. doi: 10.1016/j.molimm.2011.05.008
107. Crowley SD, Rudemiller NP. Immunologic effects of the renin-angiotensin system. J Am Soc Nephrol (2017) 28:1350–61. doi: 10.1681/ASN.2016101066
Keywords: viral infections, transcriptomics, immune response, master regulators, peripheral blood mononuclear cells, COVID-19
Citation: Ivanov SM, Tarasova OA and Poroikov VV (2023) Transcriptome-based analysis of human peripheral blood reveals regulators of immune response in different viral infections. Front. Immunol. 14:1199482. doi: 10.3389/fimmu.2023.1199482
Received: 03 April 2023; Accepted: 01 September 2023;
Published: 19 September 2023.
Edited by:
Gennady Bocharov, Marchuk Institute of Numerical Mathematics (RAS), RussiaReviewed by:
Valeria De Giorgi, National Institutes of Health (NIH), United StatesShetty Ravi Dyavar, Adicet Bio, Inc, United States
Copyright © 2023 Ivanov, Tarasova and Poroikov. This is an open-access article distributed under the terms of the Creative Commons Attribution License (CC BY). The use, distribution or reproduction in other forums is permitted, provided the original author(s) and the copyright owner(s) are credited and that the original publication in this journal is cited, in accordance with accepted academic practice. No use, distribution or reproduction is permitted which does not comply with these terms.
*Correspondence: Sergey M. Ivanov, smivanov7@gmail.com