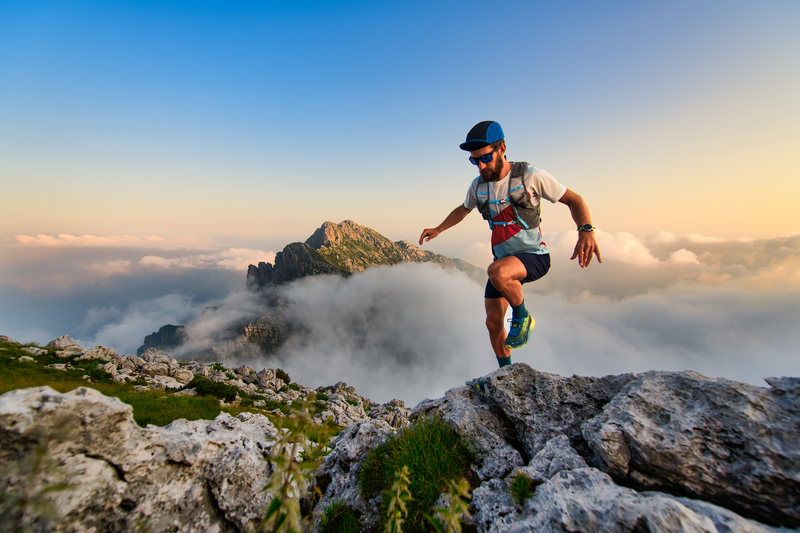
94% of researchers rate our articles as excellent or good
Learn more about the work of our research integrity team to safeguard the quality of each article we publish.
Find out more
ORIGINAL RESEARCH article
Front. Immunol. , 16 August 2023
Sec. Autoimmune and Autoinflammatory Disorders: Autoinflammatory Disorders
Volume 14 - 2023 | https://doi.org/10.3389/fimmu.2023.1195553
This article is part of the Research Topic Genetic and Molecular Determinants in Bone Health and Diseases View all 9 articles
Background: Studies of liver dysfunction in relation to bone and joint-related diseases are scarce, and its causality remains unclear. Our objective was to investigate whether serum liver enzymes are causally associated with bone and joint-related diseases using Mendelian randomization (MR) designs.
Methods: Genetic data on serum liver enzymes (alkaline phosphatase (ALP); alanine transaminase (ALT); gamma-glutamyl transferase (GGT)) and six common bone and joint-related diseases (rheumatoid arthritis (RA), osteoporosis, osteoarthritis (OA), ankylosing spondylitis, psoriatic arthritis, and gout) were derived from independent genome-wide association studies of European ancestry. The inverse variance-weighted (IVW) method was applied for the main causal estimate. Complementary sensitivity analyses and reverse causal analyses were utilized to confirm the robustness of the results.
Results: Using the IVW method, the positive causality between ALP and the risk of osteoporosis diagnosed by bone mineral density (BMD) at different sites was indicated (femoral neck, lumbar spine, and total body BMD, odds ratio (OR) [95% CI], 0.40 [0.23–0.69], 0.35 [0.19–0.67], and 0.33 [0.22–0.51], respectively). ALP was also linked to a higher risk of RA (OR [95% CI], 6.26 [1.69–23.51]). Evidence of potential harmful effects of higher levels of ALT on the risk of hip and knee OA was acquired (OR [95% CI], 2.48 [1.39–4.41] and 3.07 [1.49–6.30], respectively). No causal relationship was observed between GGT and these bone and joint-related diseases. The study also found that BMD were all negatively linked to ALP levels (OR [95% CI] for TBMD, FN-BMD, and LS-BMD: 0.993 [0.991–0.995], 0.993 [0.988–0.998], and 0.993 [0.989, 0.998], respectively) in the reverse causal analysis. The results were replicated via sensitivity analysis in the validation process.
Conclusions: Our study revealed a significant association between liver function and bone and joint-related diseases.
● The genetically predicted alkaline phosphatase (ALP) was positively related to the risk of osteoporosis diagnosed by bone mineral density (BMD) and rheumatoid arthritis (RA).
● Genetic evidence of the potential harmful effects of higher levels of alanine transaminase (ALT) on the risk of hip and knee osteoarthritis was indicated.
● There was little evidence supporting causality between gamma-glutamyl transferase (GGT) and diverse bone and joint-related diseases.
Bones and joints play a vital role in supporting weight and facilitating movement in the human body. However, there has been a notable surge in the global prevalence of bone and joint-related diseases, with joint disease ranking as the second most prevalent cause of disability worldwide (1, 2). Hence, it is crucial to delve into the unexplored mechanisms underlying bone and joint-related diseases. Recent research has highlighted the importance of a signaling axis between the liver and bone, which is critical in maintaining body homeostasis by interacting with other organs like the gut, brain, and endocrine organs (3–6). Among patients with chronic liver disease, 5% to 20% also suffer from bone and joint-related diseases such as osteoporosis and fractures (7). A large retrospective study including 931,193 fracture patients found that fracture patients with chronic liver disease had significantly higher mortality and hospitalization rates than those without chronic liver disease. Another cross-sectional study including 17,476 adults found that the risk of osteoarthritis (OA) was 1.479 times higher in the presence of metabolic-associated fatty liver disease (8). A meta-analysis of 30 studies (29 retrospective cohort studies and one cross-sectional study) demonstrated that liver transplant patients were at five times greater risk for fractures than patients who had not received liver transplants (9). Hepatic osteodystrophy is defined as poor bone metabolism and structure resulting from chronic liver disease (10), and its underlying mechanisms may be related to immunity (11), growth factor (12), hormones (13), and nutrients (14).
Our aim was to comprehensively address aspects of bone and joint-related diseases within the constraints of our study. However, it is important to acknowledge that it is unavailable to address all the genetic bone and joint-related diseases due to the absence of a comprehensive genome-wide association studies (GWAS) database. We were unable to incorporate them into our investigation. Therefore, we opted to focus on six bone and joint-related diseases (rheumatoid arthritis (RA), osteoporosis, OA, ankylosing spondylitis (AS), psoriatic arthritis (PsA), and gout) for which there is publicly available high-quality GWAS data. The selection of these six bone and joint-related diseases with a high incidence rate was primarily driven by the objective of investigating the potential causal relationship between bone and joint-related diseases and liver enzymes. Existing reports indicate that genetics might contribute to this association (15, 16).
Liver enzymes such as alanine aminotransferase (ALT), alkaline phosphatase (ALP), and gamma-glutamyl transferase (GGT) are major biomarkers that reflect liver function, and the levels of blood liver enzymes are usually significantly elevated in patients with hepatic impairment (17–19). Notably, liver enzyme levels have also been found to be elevated in patients with bone and joint-related diseases. For example, serum ALP is elevated in patients with AS and RA, and serum ALT is elevated in patients with PsA (20–22). Thus, elevated blood liver enzymes may be considered a potential risk factor for bone and joint-related disease. However, little is known about the causal relationships between liver enzymes and bone and joint-related diseases.
Well-designed randomized controlled trials (RCTs)—the gold standard to imply causality—can effectively deal with potential confounders. However, RCTs take considerable time and might be impractical due to ethical concerns and financial limitations. As an important complementary causal research approach, Mendelian randomization (MR) uses genetic variants associated with the exposure as instrumental variables to robustly assess the causality between exposure and outcome, given that certain assumptions, including the absence of pleiotropy are valid (23). Against this background, the purpose of this study was to investigate the causal relationships between serum liver enzymes and six common bone and joint-related diseases via MR.
An overview of the study design is shown in Figure 1. Three liver enzymes (ALT, ALP, and GGT) were regarded as exposure factors. Six bone and joint-related diseases, namely RA, osteoporosis, OA, AS, PsA, and gout, with 10 traits were considered the outcomes. The GWAS data of the exposure and outcome groups were collected. Using these data, MR analysis was performed to determine whether the liver enzymes have causal effects on the different bone and joint-related diseases. Three key assumptions must be met in a MR study: (1) the genetic variant is associated with the risk factor; (2) the genetic variant is not associated with confounders; and (3) the genetic variant influences the outcome only through the risk factor. All the data used in this study are published in GWAS databases; thus, additional ethical approval was not necessary. The study utilized various methods for statistical and sensitivity analysis, and the findings indicated that ALP was causally linked to decreased bone mineral density and a heightened risk of rheumatoid arthritis. Additionally, ALT was found to be causally associated with an increased risk of osteoarthritis and gout. However, GGT did not show any causal relation to the six bone and joint-related diseases studied.
Figure 1 Overview and analysis process of our research. SNP, single-nucleotide polymorphism; IVW, inverse variance-weighted; MR, Mendelian randomization; ALT, alanine aminotransferase; ALP, alkaline phosphatase; OA, osteoarthritis; RA, rheumatoid arthritis; T-BMD, total body bone mineral density; FN-BMD, femoral neck bone mineral density; LS-BMD, lumbar spine bone mineral density.
All the data utilized in this study was obtained from publicly available GWAS studies. The genetic analysis of liver enzymes was based on GWAS data for 753,010 European people from the UK Biobank and the Million Veteran Program (24). Osteoporosis was measured by bone mineral density (BMD). The data of total body BMD (T-BMD) were derived from a large GWAS meta-analysis involving about 66,628 European populations from the USA, Europe, and Australia (25). Femoral neck BMD (FN-BMD), lumbar spine BMD (LS-BMD), and forearm BMD (FA-BMD) data from a large whole genome sequencing, including 53,236 populations of European ancestry (26). BMD measurements were conducted using DXA according to standard manufacturer protocols. OA data were from the UK Biobank, including 77,052 cases and 378,169 controls (27). The diagnosis of OA was based on a radiological examination and clinical evaluation. The data for AS originates from the IEU OpenGWAS project database, with 1296 cases and 461,637 controls for the European population (28), and the data of RA comes from a large-scale GWAS meta-analysis involving 14,361 RA cases and 43,923 controls of European ancestry populations (29). The diagnosis of AS was defined by the code M16 in the International Classification of Diseases, Tenth Revision (ICD-10). All cases of RA met the 1987 criteria for RA diagnosis established by the American College of Rheumatology or were diagnosed by a professional rheumatologist. PsA data are from the FinnGen Biobank, involving 1,637 cases and 212,242 controls (30). The diagnosis of PsA also conformed to the ICD-10 code L40.5. Gout’s GWAS data come from a large GWAS meta-analysis involving 763,813 people (98.7% are of European ancestry) (31). Gout was identified based on serum urate levels, self-report, intake of urate-lowering medications, or the International Statistical Classification of Diseases and Related Health Problems (ICD) codes. The existing publications are listed in Table 1.
According to previous research, for each liver enzyme trait, the single-nucleotide polymorphisms (SNPs) were then filtered using the following steps (32, 33): (1) A genome-wide significance threshold p < 5 × 10−8 was used. (2) Linkage disequilibrium (LD) test was performed using PLINK, and LD r2 < 0.001 was adopted to ensure the independence of the selected genetic variants. For those variants missing from the outcome dataset, proxy SNPs with LD r2 > 0.8 were used. (3) The F-statistic of each SNP was calculated, and SNPs with F < 10 were eliminated to avoid weak instrument bias. The proportion of variation explained (R2) was also calculated to quantify the strength of genetic instruments with the following equation: R2 = [2 × Beta2 × (1 − EAF) × EAF]/[2 × Beta2 × (1 − EAF) × EAF + 2 × SE2 × N × (1 − EAF) × EAF], where Beta indicates the genetic effect of each SNP, EAF is effect allele frequency, SE is the standard error, and N is the sample size. To assess the strength of the selected SNPs, the F-statistic was calculated using the following equation for each SNP: F = R2 (N − k − 1)/k(1 − R2), where R2 represents the exposure variance explained by the selected SNPs, N is the sample size, and k represents the number of included instrumental variables. Weak instruments with F-statistics less than 10 were removed. The remaining independent instruments were used for subsequent MR analysis. (4) The SNPs that were incompatible or palindromic with intermediate allele frequencies were removed in the process of harmonizing. (5) Mendelian randomization pleiotropy residual sum and outlier test were performed to detect outliers and adjust for horizontal pleiotropy. If horizontal pleiotropy was detected among the instrumental variables, the outliers were removed.
Each liver enzyme trait was matched with each bone and joint-related disease for the causal estimate. The inverse variance-weighted (IVW) method was first used to evaluate potential causal effects (34). The weighted median (WM) method (35) and the MR-Egger method (36) were then applied for sensitivity analyses. The causal effect estimates reflect the increase in outcome trait risk per 1-unit-higher standard deviation (SD) of each liver enzyme in the natural scale and presented as odds ratios (ORs) with their 95% confidence interval (CI). To correct for multiple comparisons for multiple hypotheses, the significance of causal inference (Bonferroni adjusted p-value) was set to less than 0.05/10 = 0.005 in the main IVW MR analyses (37).
Potential heterogeneity was quantified and tested by Cochran’s Q test (38), and directional pleiotropy was estimated by the MR-Egger regression test (36). Leave-one-out analysis was conducted to identify any potential outliers that independently influence the observed causal relationship. To eliminate the influence of confounding factors, we searched Phenoscanner (http://www.phenoscanner.medschl.cam.ac.uk/) to determine whether the selected SNPs are associated with other confounding risk factors. After excluding the confounder-related SNPs, we rechecked that the causal relationship remained significant. The statistical analyses above were mainly completed using the two-sample MR package (version 0.5.5) of R software (version 4.0.2). All reported p-values were two-tailed, and p < 0.05 was considered to indicate a significant difference.
To further explore the biological connection between liver enzymes and bone and joint-related diseases, we performed Kyoto Encyclopedia of Genes and Genomes (KEGG) enrichment analyses using the nearest genes for each lead SNP. Comprehensive gene list annotation and analysis were performed in Metascape (http://metascape.org/gp/index.html), a customer-friendly web-based portal. Enrichment dot bubbles were plotted using https://www.bioinformatics.com.cn, a free online platform for data analysis and visualization.
To investigate the potential reverse causal relationship between liver enzymes and specific bone and joint-related diseases, we conducted a reverse causal relationship analysis. Since there might be significant causality between liver enzymes and these diseases, we reversed the exposure and outcome. We followed the standard procedure to select appropriate genetic instruments and utilized IVW methods for the main analysis. Additionally, we performed sensitivity analyses to assess the robustness of our findings.
All the remaining SNPs and their related data, namely beta (β), standard error (SE), effect allele/other allele, and p-value, utilized to conduct the subsequent causal analysis are listed in Supplementary File 1 (Tables S1–S7). Among them is effect size (β) for a SNP by modeling its association with the trait through a regression model, such as linear regression for a quantitative trait (each liver enzyme) or logistic regression for a qualitative outcome trait, assuming a linear trend per copy of an allele. The regression coefficients for quantitative traits (each liver enzyme) are presented with per 1-unit-higher SD, and for qualitative outcome traits, they are presented with per 1-unit-higher log odds, so that they are comparable across traits. The F-statistics of all the SNPs were greater than 10, indicating sufficient correlation strength between the instrumental variables and exposure traits.
The results of the preliminary analyses of the effects of genetically predicted liver enzymes on the risk of different bone and joint-related diseases are listed in Supplementary File 2 (Table S8; Figure 2). Among them, a genetically predicted higher serum level of ALP was associated with a higher risk of RA (OR: 6.26, 95% CI: 1.69 to 23.15, p = 5.97 × 10−3). The positive causality between ALP and risk of osteoporosis diagnosed by bone mineral density (BMD) at different sites was indicated (TBMD, OR: 0.33, 95% CI: 0.22 to 0.51, p = 5.53 × 10−7; FN-BMD, OR: 0.40, 95% CI: 0.23 to 0.69, p = 8.44 × 10−4; LS-BMD, OR: 0.35, 95% CI: 0.19 to 0.67, p = 1.31 × 10−3). ALT showed positive causal relationships with the risk of OA and gout (knee OA, OR: 2.48, 95% CI: 1.39 to 4.41; p = 2.06 × 10−3; hip OA, OR: 3.07, 95% CI: 1.49–6.30; p = 2.27 × 10−3; gout, OR: 4.94, 95% CI: 1.98–12.37; p = 6.36 × 10−4). Figure 3 shows a scatter plot of the causal estimates of the effects of each liver enzyme trait on the risk of different bone and joint-related diseases. No causal relationship could be supported based on our estimates between GGT and these bone and joint-related diseases.
Figure 2 A panorama of all the liver enzyme features and their causal estimate on diverse phenotypes of bone and joint-related diseases identified at the nominal significance based on p-value of IVW methods. T-BMD, total body bone mineral density; FA-BMD, forearm bone mineral density; FN-BMD, femoral neck bone mineral density; LS-BMD, lumbar spine bone mineral density; OA, osteoarthritis; RA, rheumatoid arthritis; AS, ankylosing spondylitis; PsA, psoriatic arthritis; ALT, alanine aminotransferase; ALP, alkaline phosphatase; GGT, gamma-glutamyl transferase; IVW, inverse variance-weighted.
Figure 3 Scatter plot showing the effect sizes (beta) of the SNP effects on outcomes (y-axes) and the live enzymes (x-axes) with 95% confidence intervals. Each dot represents one of the SNPs used as the genetic instrument. The slopes indicate the estimate for each of the three different MR tests. SNP effects for each live enzyme are presented with per 1‐unit‐higher standard deviation (SD), and for outcome (diverse phenotypes of bone and joint-related diseases), they are presented with per 1‐unit‐higher log odds. (A–D) The estimated effect sizes of ALP on RA, total BMD, femoral neck BMD, and lumbar spine BMD. (E–G) SNP effects for estimated effect sizes of ALT on knee OA, hip OA, and gout. IVW, inverse variance-weighted; MR, Mendelian randomization; SNP, single-nucleotide polymorphism; RA, rheumatoid arthritis; BMD, body bone mineral density; ALP, alkaline phosphatase; ALT, alanine aminotransferase; OA, osteoarthritis.
To avoid excessive bias, a series of sensitivity analyses were conducted to test the reliability of the MR analysis and detect potential pleiotropy. As shown in Table 2, the intercept of the MR-Egger regression showed no sign of directional pleiotropy (p > 0.05) of all causality estimates. Cochran’s Q test indicated that considerable heterogeneity could not be excluded with confidence. The leave-one-out analysis conducted between ALP and gout detected three SNPs (rs174564, rs55714927, and rs6680628) that would drive the result, making the causal estimate unreliable. In the other leave-one-out analyses, no fundamental causal effects were changed, regardless of which SNP was removed, reflecting the robustness of our MR analysis (Supplementary File 3; Supplementary Tables S9–S16). As shown in Table 3, after removing the SNPs associated with confounding factors detected on Phenoscanner (listed in Supplementary File 4; Supplementary Table S17), the positive causal relationships found in primary analyses remained significant except the estimate between ALT and the risk of gout (OR (95% CI): 2.38 (0.77–7.34), p = 0.131, after adjustment), indicating that confounding factors are thought to be responsible for the finding between ALT and the risk of gout. MR power calculation showed strong power (100%) to detect a significant causal effect.
KEGG pathway enrichment analysis indicated significant enrichment in the 20 most important regulation pathways (Supplementary Figures S1–S6). Several of these pathways may be involved in disease pathogenesis and are thus worthy of attention. Among them, RUNX2 transcriptional regulation and DNA methylation may be involved in the mechanism by which ALP reduces BMD and increases the risk of RA. Lipid homeostasis and circulatory system regulation involve multiple biological processes and molecular functions that may contribute to the essentiality of the association between ALT and the risk of OA.
In primary analysis, ALP was found to be causally associated with a higher risk of osteoporosis and RA, while ALT was associated with a higher risk of OA. In the reverse causal relationship analysis, we conducted IVW analyses to explore the potential causal effect of BMD on ALP and OA on ALT (Supplementary File 5; Supplementary Table S18). The results showed evidence for a causal effect of BMD on ALP levels. Specifically, BMD was inversely associated with ALP levels. The ORs and their corresponding 95% CIs were as follows: TBMD (OR: 0.993, 95% CI: 0.991 to 0.995, p = 1.06 × 10−8), FN-BMD (OR: 0.993, 95% CI: 0.988 to 0.998, p = 4.28 × 10−3), and LS-BMD (OR: 0.993, 95% CI: 0.989 to 0.998, p = 2.57 × 10−3) (Table 4). The estimates obtained using the weighted median method were consistent with these results. Furthermore, we conducted a MR-Egger regression analysis to assess directional pleiotropy. The intercepts of the MR-Egger regression indicated no evidence of directional pleiotropy for all causality estimates (p > 0.05) (Table 5). Although Cochran’s Q test suggested the presence of considerable heterogeneity, the leave-one-out analysis demonstrated that the fundamental causal effects remained unchanged regardless of the SNP removed, indicating the robustness of our MR analysis.
Table 4 Main causal relationships detected by MR analysis with different methods in revise causality analysis.
Table 5 Main causal relationships detected by MR analysis with compensated sensitivity methods in revise causality analysis.
The mechanisms underlying the elevated risk of bone and joint-related diseases in patients with liver disease are complex, and the precise mechanisms remain unknown. Our study provides strong genetic evidence in support of potential causal links between liver enzymes and an increased risk of specific bone and joint-related diseases based on a validated structured MR analysis. Specifically, we found that ALP was causally associated with a higher risk of osteoporosis and RA, while ALT was associated with a higher risk of OA. However, no causal relationships were observed between GGT and the diverse bone and joint-related diseases in our study. These results were significant in the main MR analyses and consistent across follow-up sensitivity analyses. These findings reveal causal relationships between liver enzymes and bone and joint-related diseases, suggesting that liver enzymes may serve as potential biomarkers for specific bone and joint-related diseases.
Serum ALP mainly comes from the liver and bones, with a minor amount coming from the intestine (39). Serum ALP is elevated when liver dysfunction develops (40). A study including 6,334 adults found that total serum ALP was inversely associated with BMD (41). Experimental high-ALP rat models established through bile-duct ligation exhibited skeletal fragility and impaired osteoblastogenesis, resulting in lower bone maximal force and stiffness (42). Naylor and Eastell found that increased ALP activity was associated with increased bone turnover (43). Interestingly, studies have shown that some liver cell membrane repair and protective agents can inhibit bone loss and promote bone formation (44, 45). For instance, ursodeoxycholic acid, another commonly used clinical drug for improving bile stasis, can promote mesenchymal stem cells to differentiate into osteoblasts, inhibit adipocyte differentiation, inhibit the production of inflammatory factors, and reduce inflammation-related side effects to promote bone formation (45, 46). These studies suggest that liver function improvement may regulate bone and joint-related metabolism. There are only a few clinical reports on the effect of ursodeoxycholic acid on bone mineral density. One of the studies pointed out that there was no significant effect on bone mineral density in patients with primary biliary cirrhosis (47). However, another study pointed out that the therapeutic effect of ursodeoxycholic acid on patients with primary biliary cirrhosis was related to the original vitamin D3 level of the patients, and the ALP level did not improve in patients with low vitamin D3 levels (48). Therefore, further comprehensive research is needed to understand the effect of ursodeoxycholic acid on the bone and joint systems of patients with hepatitis.
Studies of serum ALP concentration in relation to RA are scarce. In some observational studies, the ALP levels in the serum and synovia of RA patients are significantly elevated, and ALP is positively correlated with RA disease activity (49, 50). Meanwhile, patients with RA have high bone turnover and a high proportion of osteoporosis, which may explain the causal association between ALP and RA to some extent (49, 51).
The results of the KEGG analysis revealed other possible related mechanisms. RUNX2 is a classical regulatory transcription factor that promotes the differentiation of mesenchymal cells into osteoblasts (52–54). Interestingly, treatment with an ALP inhibitor reduced RUNX2 expression, a master transcriptional factor in osteoblasts, suggesting that the causal relationship between ALP and BMD may be driven through the RUNX2-associated signaling pathway (55). DNA methyltransferases can catalyze the methylation of DNACpG islands, thereby impairing genome stability and reducing the activity of gene transcription (56). DNA methylation has been shown to play an important role in the pathogenesis of osteoporosis (57–59). For example, the methylation of the osteoclast activation signal RANKL gene promoter region is significantly reduced in patients with osteoporosis, resulting in increased RANKL expression and increased osteoclast activity (60). In our study, ALP-related SNPs were enriched in DNA methylation pathways, suggesting that ALP may increase osteoclast activity by affecting DNA methylation levels. Further verified analyses should be applied in the future.
ALT is another important biomarker of liver function. Elevated serum ALT levels indicate impaired liver function (61). The relationship between ALT and OA has not been well investigated in epidemiology. However, our MR analysis suggested a definitive causal relationship between ALT and OA. We also obtained some hints from KEGG pathway analysis, suggesting that the mechanism may be related to circulatory system processes and lipid homeostasis. Circulation plays an important role in the pathogenesis of OA. Subchondral vascular hyperplasia, decreased arterial blood flow, and venous stasis have been reported in patients with knee osteoarthritis (62–64). Subchondral hyperemia in patients with OA leads to osteoblast hypoxia, alters the gene expression profile of cytokines, and ultimately leads to subchondral remodeling and chondrocyte degeneration, which are recognized as major components of the pathogenesis of OA (30, 65–68). Epidemiological studies suggest that ALT may significantly affect an individual’s circulatory system. For example, a retrospective study of 11,324 patients found that elevated plasma ALT levels were significantly associated with a higher risk of abnormal myocardial perfusion and myocardial infarction (69). The liver is the regulatory center of lipid metabolism in the body and maintains lipid homeostasis. In addition, serum desmosterol levels and the desmosterol/cholesterol ratio (a marker of cholesterol synthesis) were positively associated with ALT in a random population-based sample of 717 men (70). The concentration of cholesterol in synovial fluid is significantly elevated in individuals with OA (71). Choi et al. found that cholesterol and its metabolites directly activate retinoic acid-related orphan alpha receptors on chondrocytes, upregulate matrix-degrading enzymes, and increase the risk of OA (72). Notably, Maximos et al. demonstrated that the hepatic triglyceride content in patients with liver disease is positively correlated with the plasma ALT level (73). Based on a cohort study, Chen et al. found that the risk of new-onset metabolic fatty liver disease increased concurrently with increasing ALT levels (74). Hypertriglyceridemia is predominantly associated with worse pain in OA through central obesity (75). The relationship between ALT and the risk of OA could not be well established based on the present observational studies; thus, sufficient supporting evidence was needed from mechanical research.
The results of the reverse causal relationship suggested that BMD was inversely associated with ALP levels, but there was no reverse causal relationship between OA and ALT. These results were consistent across follow-up sensitivity analyses. Currently, no studies have clarified how BMD affects the liver enzyme. However, it is crucial to note that studies conducted in the last 10 years have revealed that the skeletal joint system is more than just a simple load-bearing structure. It is also a significant endocrine organ, and the cytokines it secretes govern numerous organs throughout the body, including the liver (76). For instance, a prospective analysis of 2,055 community populations discovered that women with lower levels of the typical bone-derived cytokine osteocalcin had a higher risk of nonalcoholic fatty liver disease, and further animal studies revealed that osteocalcin therapy for mice reduced hepatic steatosis (77). In addition, bone contains a lot of mesenchymal stem cells, and it has been discovered that these cells could regulate CD4 T-cell differentiation to lessen nonalcoholic liver steatosis in mouse models (78). Even though more direct studies are required, the aforementioned indirect findings may help partially explain the weak reverse causality relationship between BMD and ALP.
Our study has several limitations. First, there is an overlap between our disease GWAS data, like the presence of the UK Biobank in both the liver enzyme and OA databases. The challenge of resolving sample overlap across multiple GWAS databases remains unresolved due to the use of summary-level data that cannot be extracted individually. Certainly, it is important to exercise caution when drawing conclusions due to the potential inflation of causal associations resulting from sample overlap. Nevertheless, the strong instruments used in our study (i.e., F-statistic >> 10) could minimize potential bias due to the duplication of samples. Second, given that the summary-level statistics were obtained from the publicly available GWAS data, we could not perform other subgroup analyses to address associations with study-specific factors (e.g., age, gender, and other risk factors). The detailed characteristics like the effect allele frequency of genetic variants identified through GWAS study, can vary significantly across different ethnic backgrounds. These variations can influence the prevalence, genetic factors, and disease mechanisms of bone and joint-related diseases. Therefore, findings from a study conducted exclusively on European populations may not necessarily apply to other populations with distinct genetic backgrounds. Further research involving diverse populations is necessary to obtain a more comprehensive understanding of the genetic factors contributing to bone and joint-related diseases worldwide. Third, Cochran’s Q test indicated that considerable heterogeneity could not be excluded with confidence. However, based on the results of the IVW and weighted median methods, we believe that the robustness of our study was not biased by heterogeneity. Moreover, MR-Egger regression ruled out directional pleiotropy, indicating that heterogeneity did not cause pleiotropy or bias.
To our knowledge, this is the first study to comprehensively investigate the causal relationships between liver enzymes and bone and joint-related diseases based on publicly available genetic data via MR analysis. Although earlier studies have demonstrated that the risk of bone and joint-related illnesses will rise when liver function is poor for an extended period of time, no studies have yet suggested which specific liver function markers may raise the risk of certain bone and joint disorders. As a result, clinical recommendations are ambiguous, meaning that although medical professionals believe that patients with liver function impairment have an increased risk of developing bone and joint disorders, they are unsure of which specific bone and joint-related diseases the patient is more likely to develop. In this study, we discovered for patients with liver disease, blood ALP may be a potential biomarker of osteoporosis and RA, and blood ALT may be a potential biomarker of OA and gout. This finding strongly suggests the significant role of the liver in regulating bone and joint-related diseases. In patients with long-term liver disease, it is important to be mindful of screening for bone and joint-related diseases to facilitate early detection and treatment. The results may provide a new strategy to understand the relationships between liver and bone and guide clinical decisions related to disease management. Certainly, additional evidence is needed to further support this conclusion.
The original contributions presented in the study are included in the article/Supplementary Material. Further inquiries can be directed to the corresponding author.
GH and WCL collected data, conducted the MR analysis, and wrote the manuscript. GH, WCL, and YZ contributed to the conceptualization, methodology, data acquisition and curation, formal analysis, visualization, writing, and editing. GH, WML, and ZZ contributed to the methodology, interpretation of data, writing, and editing. All authors reviewed the manuscript. ZZ takes full responsibility for the integrity of the study. All authors contributed to the article and approved the submitted version.
This study was supported by the National Natural Science Foundation of China (81972049, 82172467, 82172406), the First Affiliated Hospital of Sun Yat-Sen University Ke Ling Funding Program for Novel and Distinguished Talents (R07005), and the Guangdong Natural Science Funds for Distinguished Young Scholar of China (2021B1515020008).
We thank the consortium studies for making the summary association statistics data publicly available.
The authors declare that the research was conducted in the absence of any commercial or financial relationships that could be construed as a potential conflict of interest.
All claims expressed in this article are solely those of the authors and do not necessarily represent those of their affiliated organizations, or those of the publisher, the editors and the reviewers. Any product that may be evaluated in this article, or claim that may be made by its manufacturer, is not guaranteed or endorsed by the publisher.
The Supplementary Material for this article can be found online at: https://www.frontiersin.org/articles/10.3389/fimmu.2023.1195553/full#supplementary-material
Supplementary Figure 1 | KEGG pathway enrichment analysis of nearest genes for single-nucleotide polymorphisms used on the causality inference for ALP on the risk of rheumatoid arthritis. (A) Enriched ontology clusters. Each cluster is represented in a single color and shown as a circle. (B) Enrichment dot bubble where count means enriched number of genes.
Supplementary Figure 2 | KEGG pathway enrichment analysis of nearest genes for single-nucleotide polymorphisms used on the causality inference for ALP on the risk of total body BMD. (A) Enriched ontology clusters. Each cluster is represented in a single color and shown as a circle. (B) Enrichment dot bubble where count means enriched number of genes.
Supplementary Figure 3 | KEGG pathway enrichment analysis of nearest genes for single-nucleotide polymorphisms used on the causality inference for ALP on the risk of femoral neck BMD. (A) Enriched ontology clusters. Each cluster is represented in a single color and shown as a circle. (B) Enrichment dot bubble where count means enriched number of genes.
Supplementary Figure 4 | KEGG pathway enrichment analysis of nearest genes for single-nucleotide polymorphisms used on the causality inference for ALP on the risk of Lumbar spine BMD. (A) Enriched ontology clusters. Each cluster is represented in a single color and shown as a circle. (B) Enrichment dot bubble where count means enriched number of genes.
Supplementary Figure 5 | KEGG pathway enrichment analysis of nearest genes for single-nucleotide polymorphisms used on the causality inference for ALT on the risk of knee OA. (A) Enriched ontology clusters. Each cluster is represented in a single color and shown as a circle. (B) Enrichment dot bubble where count means enriched number of genes.
Supplementary Figure 6 | KEGG pathway enrichment analysis of nearest genes for single-nucleotide polymorphisms used on the causality inference for ALT on the risk of hip OA. (A) Enriched ontology clusters. Each cluster is represented in a single color and shown as a circle. (B) Enrichment dot bubble where count means enriched number of genes.
Supplementary Figure 7 | Plots of “leave-one-out” analyses (A–C) for MR analyses of the reverse causal relationship between BMD and ALP.
1. Sebbag E, Felten R, Sagez F, Sibilia J, Devilliers H, Arnaud L. The world-wide burden of musculoskeletal diseases: a systematic analysis of the World Health Organization Burden of Diseases Database. Ann Rheumatic Dis (2019) 78(6):844–8. doi: 10.1136/annrheumdis-2019-215142
2. Shen Y, Huang X, Wu J, Lin X, Zhou X, Zhu Z, et al. The Global Burden of Osteoporosis, Low Bone Mass, and Its Related Fracture in 204 Countries and Territories, 1990-2019. Front Endocrinol (2022) 13:882241. doi: 10.3389/fendo.2022.882241
3. Wang F, So KF, Xiao J, Wang H. Organ-organ communication: The liver's perspective. Theranostics (2021) 11(7):3317–30. doi: 10.7150/thno.55795
4. Emdin CA, Khera AV, Kathiresan S. Mendelian Randomization. Jama (2017) 318(19):1925–6. doi: 10.1001/jama.2017.17219
5. Yan L, Wei JA, Yang F, Wang M, Wang S, Cheng T, et al. Physical Exercise Prevented Stress-Induced Anxiety via Improving Brain RNA Methylation. Adv Sci (Weinheim Baden-Wurttemberg Germany) (2022) 9(24):e2105731. doi: 10.1002/advs.202105731
6. Lu K, Shi TS, Shen SY, Shi Y, Gao HL, Wu J, et al. Defects in a liver-bone axis contribute to hepatic osteodystrophy disease progression. Cell Metab (2022) 34(3):441–57.e7. doi: 10.1016/j.cmet.2022.02.006
7. Jeong HM, Kim DJ. Bone Diseases in Patients with Chronic Liver Disease. Int J Mol Sci (2019) 20(17). doi: 10.3390/ijms20174270
8. Han AL. Association between metabolic associated fatty liver disease and osteoarthritis using data from the Korean national health and nutrition examination survey (KNHANES). Inflammopharmacology (2021) 29(4):1111–8. doi: 10.1007/s10787-021-00842-7
9. Lim WH, Ng CH, Ow ZGW, Ho OTW, Tay PWL, Wong KL, et al. A systematic review and meta-analysis on the incidence of osteoporosis and fractures after liver transplant. Transplant Int (2021) 34(6):1032–43. doi: 10.1111/tri.13863
10. Ehnert S, Aspera-Werz RH, Ruoß M, Dooley S, Hengstler JG, Nadalin S, et al. Hepatic Osteodystrophy-Molecular Mechanisms Proposed to Favor Its Development. Int J Mol Sci (2019) 20(10). doi: 10.3390/ijms20102555
11. Choi M, Park S, Yi JK, Kwon W, Jang S, Kim SY, et al. Overexpression of hepatic serum amyloid A1 in mice increases IL-17-producing innate immune cells and decreases bone density. J Biol Chem (2021) 296:100595. doi: 10.1016/j.jbc.2021.100595
12. Liu Z, Han T, Werner H, Rosen CJ, Schaffler MB, Yakar S. Reduced Serum IGF-1 Associated With Hepatic Osteodystrophy Is a Main Determinant of Low Cortical but Not Trabecular Bone Mass. J Bone mineral Res (2018) 33(1):123–36. doi: 10.1002/jbmr.3290
13. Deng N, Mallepally N, Peng FB, Kanji A, Marcelli M, Hernaez R. Serum testosterone levels and testosterone supplementation in cirrhosis: A systematic review. Liver Int (2021) 41(10):2358–70. doi: 10.1111/liv.14938
14. Roman-Garcia P, Quiros-Gonzalez I, Mottram L, Lieben L, Sharan K, Wangwiwatsin A, et al. Vitamin B12-dependent taurine synthesis regulates growth and bone mass. J Clin Invest (2014) 124(7):2988–3002. doi: 10.1172/JCI72606
15. Mosca A, Fintini D, Scorletti E, Cappa M, Paone L, Zicari AM, et al. Relationship between non-alcoholic steatohepatitis, PNPLA3 I148M genotype and bone mineral density in adolescents. Liver Int (2018) 38(12):2301–8. doi: 10.1111/liv.13955
16. Huang X, Lv Y, He P, Wang Z, Xiong F, He L, et al. HDL impairs osteoclastogenesis and induces osteoclast apoptosis via upregulation of ABCG1 expression. Acta Biochim Biophys Sin (2018) 50(9):853–61. doi: 10.1093/abbs/gmy081
17. Giannini EG, Testa R, Savarino V. Liver enzyme alteration: a guide for clinicians. CMAJ (2005) 172(3):367–79. doi: 10.1503/cmaj.1040752
18. Abdulla S, Hussain A, Azim D, Abduallah EH, Elawamy H, Nasim S, et al. COVID-19-Induced Hepatic Injury: A Systematic Review and Meta-Analysis. Cureus (2020) 12(10):e10923. doi: 10.7759/cureus.10923
19. Mohamed MF, Wadhavkar N, Elfanagely Y, Marino D, Beran A, Abdallah M, et al. Etiologies and Outcomes of Transaminase Elevation > 1000 IU/L: A Systematic Review and Meta-Analysis. Dig Dis Sci (2023) 68(7):2843–52. doi: 10.1007/s10620-023-07962-w
20. Kang KY, Hong YS, Park SH, Ju JH. Increased serum alkaline phosphatase levels correlate with high disease activity and low bone mineral density in patients with axial spondyloarthritis. Semin Arthritis Rheumatism (2015) 45(2):202–7. doi: 10.1016/j.semarthrit.2015.03.002
21. Curtis JR, Beukelman T, Onofrei A, Cassell S, Greenberg JD, Kavanaugh A, et al. Elevated liver enzyme tests among patients with rheumatoid arthritis or psoriatic arthritis treated with methotrexate and/or leflunomide. Ann Rheumatic Dis (2010) 69(1):43–7. doi: 10.1136/ard.2008.101378
22. Nanke Y, Kotake S, Akama H, Kamatani N. Alkaline phosphatase in rheumatoid arthritis patients: possible contribution of bone-type ALP to the raised activities of ALP in rheumatoid arthritis patients. Clin Rheumatol (2002) 21(3):198–202. doi: 10.1007/s10067-002-8285-4
23. Boehm FJ, Zhou X. Statistical methods for Mendelian randomization in genome-wide association studies: A review. Comput Struct Biotechnol J (2022) 20:2338–51. doi: 10.1016/j.csbj.2022.05.015
24. Pazoki R, Vujkovic M, Elliott J, Evangelou E, Gill D, Ghanbari M, et al. Genetic analysis in European ancestry individuals identifies 517 loci associated with liver enzymes. Nat Commun (2021) 12(1):2579. doi: 10.1038/s41467-021-22338-2
25. Medina-Gomez C, Kemp JP, Trajanoska K, Luan J, Chesi A, Ahluwalia TS, et al. Life-Course Genome-wide Association Study Meta-analysis of Total Body BMD and Assessment of Age-Specific Effects. Am J Hum Genet (2018) 102(1):88–102. doi: 10.1016/j.ajhg.2017.12.005
26. Zheng HF, Forgetta V, Hsu YH, Estrada K, Rosello-Diez A, Leo PJ, et al. Whole-genome sequencing identifies EN1 as a determinant of bone density and fracture. Nature (2015) 526(7571):112–7. doi: 10.1038/nature14878
27. Tachmazidou I, Hatzikotoulas K, Southam L, Esparza-Gordillo J, Haberland V, Zheng J, et al. Identification of new therapeutic targets for osteoarthritis through genome-wide analyses of UK Biobank data. Nat Genet (2019) 51(2):230–6. doi: 10.1038/s41588-018-0327-1
28. IEU OpenGWAS project. Available at: https://gwas.mrcieu.ac.uk/.
29. Okada Y, Wu D, Trynka G, Raj T, Terao C, Ikari K, et al. Genetics of rheumatoid arthritis contributes to biology and drug discovery. Nature (2014) 506(7488):376–81. doi: 10.1038/nature12873
30. Tsai PH, Lee HS, Siow TY, Wang CY, Chang YC, Lin MH, et al. Abnormal perfusion in patellofemoral subchondral bone marrow in the rat anterior cruciate ligament transection model of post-traumatic osteoarthritis: a dynamic contrast-enhanced magnetic resonance imaging study. Osteoarthritis Cartilage (2016) 24(1):129–33. doi: 10.1016/j.joca.2015.07.015
31. Tin A, Marten J, Halperin Kuhns VL, Li Y, Wuttke M, Kirsten H, et al. Target genes, variants, tissues and transcriptional pathways influencing human serum urate levels. Nat Genet (2019) 51(10):1459–74. doi: 10.1038/s41588-019-0504-x
32. Tan JS, Liu NN, Guo TT, Hu S, Hua L. Genetically predicted obesity and risk of deep vein thrombosis. Thromb Res (2021) 207:16–24. doi: 10.1016/j.thromres.2021.08.026
33. Wang W, Tan JS, Hua L, Zhu S, Lin H, Wu Y, et al. Genetically Predicted Obesity Causally Increased the Risk of Hypertension Disorders in Pregnancy. Front Cardiovasc Med (2022) 9:888982. doi: 10.3389/fcvm.2022.888982
34. Burgess S, Small DS, Thompson SG. A review of instrumental variable estimators for Mendelian randomization. Stat Methods Med Res (2017) 26(5):2333–55. doi: 10.1177/0962280215597579
35. Bowden J, Davey Smith G, Haycock PC, Burgess S. Consistent Estimation in Mendelian Randomization with Some Invalid Instruments Using a Weighted Median Estimator. Genet Epidemiol (2016) 40(4):304–14. doi: 10.1002/gepi.21965
36. Burgess S, Thompson SG. Interpreting findings from Mendelian randomization using the MR-Egger method. Eur J Epidemiol (2017) 32(5):377–89. doi: 10.1007/s10654-017-0255-x
37. Curtin F, Schulz P. Multiple correlations and Bonferroni's correction. Biol Psychiatry (1998) 44(8):775–7. doi: 10.1016/S0006-3223(98)00043-2
38. Cohen JF, Chalumeau M, Cohen R, Korevaar DA, Khoshnood B, Bossuyt PM. Cochran's Q test was useful to assess heterogeneity in likelihood ratios in studies of diagnostic accuracy. J Clin Epidemiol (2015) 68(3):299–306. doi: 10.1016/j.jclinepi.2014.09.005
39. Haarhaus M, Cianciolo G, Barbuto S, La Manna G, Gasperoni L, Tripepi G, et al. Alkaline Phosphatase: An Old Friend as Treatment Target for Cardiovascular and Mineral Bone Disorders in Chronic Kidney Disease. Nutrients (2022) 14(10). doi: 10.3390/nu14102124
40. Ponsioen CY, Chapman RW, Chazouillères O, Hirschfield GM, Karlsen TH, Lohse AW, et al. Surrogate endpoints for clinical trials in primary sclerosing cholangitis: Review and results from an International PSC Study Group consensus process. Hepatol (Baltimore Md) (2016) 63(4):1357–67. doi: 10.1002/hep.28256
41. Shu J, Tan A, Li Y, Huang H, Yang J. The correlation between serum total alkaline phosphatase and bone mineral density in young adults. BMC Musculoskeletal Disord (2022) 23(1):467. doi: 10.1186/s12891-022-05438-y
42. Weinreb M, Pollak RD, Ackerman Z. Experimental cholestatic liver disease through bile-duct ligation in rats results in skeletal fragility and impaired osteoblastogenesis. J Hepatol (2004) 40(3):385–90. doi: 10.1016/j.jhep.2003.11.032
43. Naylor K, Eastell R. Bone turnover markers: use in osteoporosis. Nat Rev Rheumatol (2012) 8(7):379–89. doi: 10.1038/nrrheum.2012.86
44. Tao ZS, Li TL, Wei S. Silymarin prevents iron overload induced bone loss by inhibiting oxidative stress in an ovariectomized animal model. Chemico-biol Interact (2022) 366:110168. doi: 10.1016/j.cbi.2022.110168
45. Arai Y, Park H, Park S, Kim D, Baek I, Jeong L, et al. Bile acid-based dual-functional prodrug nanoparticles for bone regeneration through hydrogen peroxide scavenging and osteogenic differentiation of mesenchymal stem cells. J Control Release (2020) 328:596–607. doi: 10.1016/j.jconrel.2020.09.023
46. Cha BH, Jung MJ, Moon BK, Kim JS, Ma Y, Arai Y, et al. Administration of tauroursodeoxycholic acid enhances osteogenic differentiation of bone marrow-derived mesenchymal stem cells and bone regeneration. Bone (2016) 83:73–81. doi: 10.1016/j.bone.2015.10.011
47. Angulo P, Therneau TM, Jorgensen A, DeSotel CK, Egan KS, Dickson ER, et al. Bone disease in patients with primary sclerosing cholangitis: prevalence, severity and prediction of progression. J Hepatol (1998) 29(5):729–35. doi: 10.1016/S0168-8278(98)80253-5
48. Guo GY, Shi YQ, Wang L, Ren X, Han ZY, Guo CC, et al. Serum vitamin D level is associated with disease severity and response to ursodeoxycholic acid in primary biliary cirrhosis. Aliment Pharmacol Ther (2015) 42(2):221–30. doi: 10.1111/apt.13244
49. Wheater G, Elshahaly M, Naraghi K, Tuck SP, Datta HK, van Laar JM. Changes in bone density and bone turnover in patients with rheumatoid arthritis treated with rituximab, results from an exploratory, prospective study. PloS One (2018) 13(8):e0201527. doi: 10.1371/journal.pone.0201527
50. Aida S. Alkaline phosphatase isoenzyme activities in rheumatoid arthritis: hepatobiliary enzyme dissociation and relation to disease activity. Ann Rheumatic Dis (1993) 52(7):511–6. doi: 10.1136/ard.52.7.511
51. Adami G, Saag KG. Osteoporosis Pathophysiology, Epidemiology, and Screening in Rheumatoid Arthritis. Curr Rheumatol Rep (2019) 21(7):34. doi: 10.1007/s11926-019-0836-7
52. Kim WJ, Shin HL, Kim BS, Kim HJ, Ryoo HM. RUNX2-modifying enzymes: therapeutic targets for bone diseases. Exp Mol Med (2020) 52(8):1178–84. doi: 10.1038/s12276-020-0471-4
53. Shu HS, Liu YL, Tang XT, Zhang XS, Zhou B, Zou W, et al. Tracing the skeletal progenitor transition during postnatal bone formation. Cell Stem Cell (2021) 28(12):2122–36.e3. doi: 10.1016/j.stem.2021.08.010
54. Kim HJ, Lee S, Park JM, Cho HB, Park JI, Park JS, et al. Development of a three-layer consecutive gene delivery system for enhanced bone regeneration. Biomaterials (2021) 277:121104. doi: 10.1016/j.biomaterials.2021.121104
55. Jo S, Han J, Lee YL, Yoon S, Lee J, Wang SE, et al. Regulation of osteoblasts by alkaline phosphatase in ankylosing spondylitis. Int J Rheumatic Dis (2019) 22(2):252–61. doi: 10.1111/1756-185X.13419
56. Schübeler D. Function and information content of DNA methylation. Nature (2015) 517(7534):321–6. doi: 10.1038/nature14192
57. Peng S, Gao Y, Shi S, Zhao D, Cao H, Fu T, et al. LncRNA-AK137033 inhibits the osteogenic potential of adipose-derived stem cells in diabetic osteoporosis by regulating Wnt signaling pathway via DNA methylation. Cell Prolif (2022) 55(1):e13174. doi: 10.1111/cpr.13174
58. Morris JA, Tsai PC, Joehanes R, Zheng J, Trajanoska K, Soerensen M, et al. Epigenome-wide Association of DNA Methylation in Whole Blood With Bone Mineral Density. J Bone Miner Res (2017) 32(8):1644–50. doi: 10.1002/jbmr.3148
59. Visconti VV, Cariati I, Fittipaldi S, Iundusi R, Gasbarra E, Tarantino U, et al. DNA Methylation Signatures of Bone Metabolism in Osteoporosis and Osteoarthritis Aging-Related Diseases: An Updated Review. Int J Mol Sci (2021) 22(8). doi: 10.3390/ijms22084244
60. Wang P, Cao Y, Zhan D, Wang D, Wang B, Liu Y, et al. Influence of DNA methylation on the expression of OPG/RANKL in primary osteoporosis. Int J Med Sci (2018) 15(13):1480–5. doi: 10.7150/ijms.27333
61. Montanari NR, Ramírez R, Aggarwal A, van Buuren N, Doukas M, Moon C, et al. Multi-parametric analysis of human livers reveals variation in intrahepatic inflammation across phases of chronic hepatitis B infection. J Hepatol (2022) 77(2):332–43. doi: 10.1016/S0168-8278(22)00762-0
62. Aaron RK, Racine JR, Voisinet A, Evangelista P, Dyke JP. Subchondral bone circulation in osteoarthritis of the human knee. Osteoarthritis Cartilage (2018) 26(7):940–4. doi: 10.1016/j.joca.2018.04.003
63. Su W, Liu G, Liu X, Zhou Y, Sun Q, Zhen G, et al. Angiogenesis stimulated by elevated PDGF-BB in subchondral bone contributes to osteoarthritis development. JCI Insight (2020) 5(8). doi: 10.1172/jci.insight.135446
64. Ching K, Houard X, Berenbaum F, Wen C. Hypertension meets osteoarthritis - revisiting the vascular aetiology hypothesis. Nat Rev Rheumatol (2021) 17(9):533–49. doi: 10.1038/s41584-021-00650-x
65. Arnoldi CC, Linderholm H, Müssbichler H. Venous engorgement and intraosseous hypertension in osteoarthritis of the hip. J Bone Joint Surg Br volume (1972) 54(3):409–21. doi: 10.1302/0301-620X.54B3.409
66. Kiaer T, Pedersen NW, Kristensen KD, Starklint H. Intra-osseous pressure and oxygen tension in avascular necrosis and osteoarthritis of the hip. J Bone Joint Surg Br volume (1990) 72(6):1023–30. doi: 10.1302/0301-620X.72B6.2246284
67. Warren SM, Steinbrech DS, Mehrara BJ, Saadeh PB, Greenwald JA, Spector JA, et al. Hypoxia regulates osteoblast gene expression. J Surg Res (2001) 99(1):147–55. doi: 10.1006/jsre.2001.6128
68. Aaron RK, Racine J, Dyke JP. Contribution of Circulatory Disturbances in Subchondral Bone to the Pathophysiology of Osteoarthritis. Curr Rheumatol Rep (2017) 19(8):49. doi: 10.1007/s11926-017-0660-x
69. Yardeni D, Toledano R, Novack V, Shalev A, Wolak A, Rotman Y, et al. The Association of Alanine Aminotransferase Levels With Myocardial Perfusion Imaging and Cardiovascular Morbidity. J Cardiovasc Pharmacol Ther (2022) 27:10742484221074585. doi: 10.1177/10742484221074585
70. Simonen M, Männistö V, Leppänen J, Kaminska D, Kärjä V, Venesmaa S, et al. Desmosterol in human nonalcoholic steatohepatitis. Hepatology (2013) 58(3):976–82. doi: 10.1002/hep.26342
71. Zhang K, Ji Y, Dai H, Khan AA, Zhou Y, Chen R, et al. High-Density Lipoprotein Cholesterol and Apolipoprotein A1 in Synovial Fluid: Potential Predictors of Disease Severity of Primary Knee Osteoarthritis. Cartilage (2021) 13(1_suppl):1465s–73s. doi: 10.1177/19476035211007919
72. Choi W-S, Lee G, Song W-H, Koh J-T, Yang J, Kwak J-S, et al. The CH25H–CYP7B1–RORα axis of cholesterol metabolism regulates osteoarthritis. Nature (2019) 566(7743):254–8. doi: 10.1038/s41586-019-0920-1
73. Maximos M, Bril F, Portillo Sanchez P, Lomonaco R, Orsak B, Biernacki D, et al. The role of liver fat and insulin resistance as determinants of plasma aminotransferase elevation in nonalcoholic fatty liver disease. Hepatol (Baltimore Md) (2015) 61(1):153–60. doi: 10.1002/hep.27395
74. Chen JF, Qin Q, Wu ZQ, Yan S, Song XQ, Ding SY. [A cohort study on the correlation between alanine aminotransferase trajectories and new-onset metabolic fatty liver disease]. Zhonghua liu xing bing xue za zhi = Zhonghua liuxingbingxue zazhi (2022) 43(2):234–40.
75. Pan F, Tian J, Cicuttini F, Jones G. Metabolic syndrome and trajectory of knee pain in older adults. Osteoarthritis Cartilage (2020) 28(1):45–52. doi: 10.1016/j.joca.2019.05.030
76. Zheng XQ, Lin JL, Huang J, Wu T, Song CL. Targeting aging with the healthy skeletal system: The endocrine role of bone. Rev Endocrine Metab Disord (2023) 24(4):695–711. doi: 10.1007/s11154-023-09812-6
77. Xia M, Rong S, Zhu X, Yan H, Chang X, Sun X, et al. Osteocalcin and Non-Alcoholic Fatty Liver Disease: Lessons From Two Population-Based Cohorts and Animal Models. J Bone Miner Res (2021) 36(4):712–28. doi: 10.1002/jbmr.4227
Keywords: Mendelian randomization, liver enzyme, bone and joint-related diseases, causality, genome-wide association studies
Citation: Huang G, Li W, Zhong Y, Liao W and Zhang Z (2023) Mendelian randomization to evaluate the causal relationship between liver enzymes and the risk of six specific bone and joint-related diseases. Front. Immunol. 14:1195553. doi: 10.3389/fimmu.2023.1195553
Received: 28 March 2023; Accepted: 24 July 2023;
Published: 16 August 2023.
Edited by:
Andrea Del Fattore, Bambino Gesù Children’s Hospital (IRCCS), ItalyReviewed by:
Ya-Li Chen, Second Hospital of Hebei Medical University, ChinaCopyright © 2023 Huang, Li, Zhong, Liao and Zhang. This is an open-access article distributed under the terms of the Creative Commons Attribution License (CC BY). The use, distribution or reproduction in other forums is permitted, provided the original author(s) and the copyright owner(s) are credited and that the original publication in this journal is cited, in accordance with accepted academic practice. No use, distribution or reproduction is permitted which does not comply with these terms.
*Correspondence: Zhiqi Zhang, emh6aGlxaUBtYWlsLnN5c3UuZWR1LmNu
†These authors have contributed equally to this work
Disclaimer: All claims expressed in this article are solely those of the authors and do not necessarily represent those of their affiliated organizations, or those of the publisher, the editors and the reviewers. Any product that may be evaluated in this article or claim that may be made by its manufacturer is not guaranteed or endorsed by the publisher.
Research integrity at Frontiers
Learn more about the work of our research integrity team to safeguard the quality of each article we publish.