- 1Laboratory of Metabolites and Proteins in Translational Research, Istituto di Ricerche Farmacologiche Mario Negri IRCCS, Milan, Italy
- 2Department of Biotechnologies and Biosciences, Università degli Studi Milano Bicocca, Milan, Italy
- 3Hepatobiliary Immunopathology Laboratory, IRCCS Humanitas Research Hospital, Milan, Italy
- 4Department of Electronics, Information and Bioengineering, Politecnico di Milano, Milan, Italy
- 5Department of Biomedical Sciences, Humanitas University, Milan, Italy
- 6Department of Hepatobiliary and General Surgery, IRCCS Humanitas Research Hospital, Milan, Italy
- 7Department of Health Sciences, Università del Piemonte Orientale, Novara, Italy
- 8Department of General Surgery, University Maggiore Hospital, Novara, Italy
Tumor-associated macrophages (TAMs) represent one of the main tumor-infiltrating immune cell types and are generally categorized into either of two functionally contrasting subtypes, namely classical activated M1 macrophages and alternatively activated M2 macrophages. TAMs showed different activation states that can be represent by the two extremes of the complex profile of macrophages biology, the M1-like phenotype (pro-inflammatory activity) and the M2-like phenotype (anti-inflammatory activity). Based on the tumor type, and grades, TAMs can acquire different functions and properties; usually, the M1-like phenotype is typical of early tumor stages and is associated to an anti-tumor activity, while M2-like phenotype has a pro-inflammatory activity and is related to a poor patients’ prognosis. The classification of macrophages into M1/M2 groups based on well-defined stimuli does not model the infinitely more complex tissue milieu where macrophages (potentially of different origin) would be exposed to multiple signals in different sequential order. This review aims to summarize the recent mass spectrometry-based (MS-based) metabolomics findings about the modifications of metabolism in TAMs polarization in different tumors. The published data shows that MS-based metabolomics is a promising tool to help better understanding TAMs metabolic phenotypes, although it is still poorly applied for TAMs metabolism. The knowledge of key metabolic alterations in TAMs is an essential step for discovering TAMs polarization novel biomarkers and developing novel therapeutic approaches targeting TAM metabolism to repolarize TAMs towards their anti-tumor phenotype.
Introduction
Macrophages are immune cells essential component of the innate immune system, with a wide distribution in lymphoid and non-lymphoid tissues throughout the body that play a pivotal role in innate immunity, tissue homeostasis, and response to adverse signals, such as pathogenic infections or inflammatory stimuli (1–3).
Macrophages principally originate from monocyte precursors mainly generated from hematopoietic stem cells placed in the bone marrow; then, monocytes migrate to several tissues and differentiate into tissue-specific macrophages (3, 4). Instead, tissue-resident macrophages such as Kupffer cells (liver), microglia (central nervous system), and Langerhans cells (skin) originate from the yolk sac and fetal liver during primitive and definitive hematopoiesis and they are responsible for the innate immunity (5, 6).
Circulating monocytes are recruited by chemotactic signals generated upon injury, infection etc. and migrate to the target tissues where they differentiate and polarize into mature macrophages depending on the microenvironment (5, 7, 8). It is possible to identify two main activation states that represent the two extremes of the complex profile of macrophage biology (9). The current classification into M1-type (classically activated macrophage) and M2-type (alternatively activated macrophage) is based upon macrophage polarization and describe macrophages different behaviors (10). One phenotype, the pro-inflammatory or classically activated M1 phenotype, allows the host to fight infections and pathogens and exhibits anti-tumoral activity. The second phenotype, the anti-inflammatory or alternatively activated M2 phenotype, displays the capability to repair damaged tissues but also presents a pro-tumoral functions (Figure 1A) (11–15).
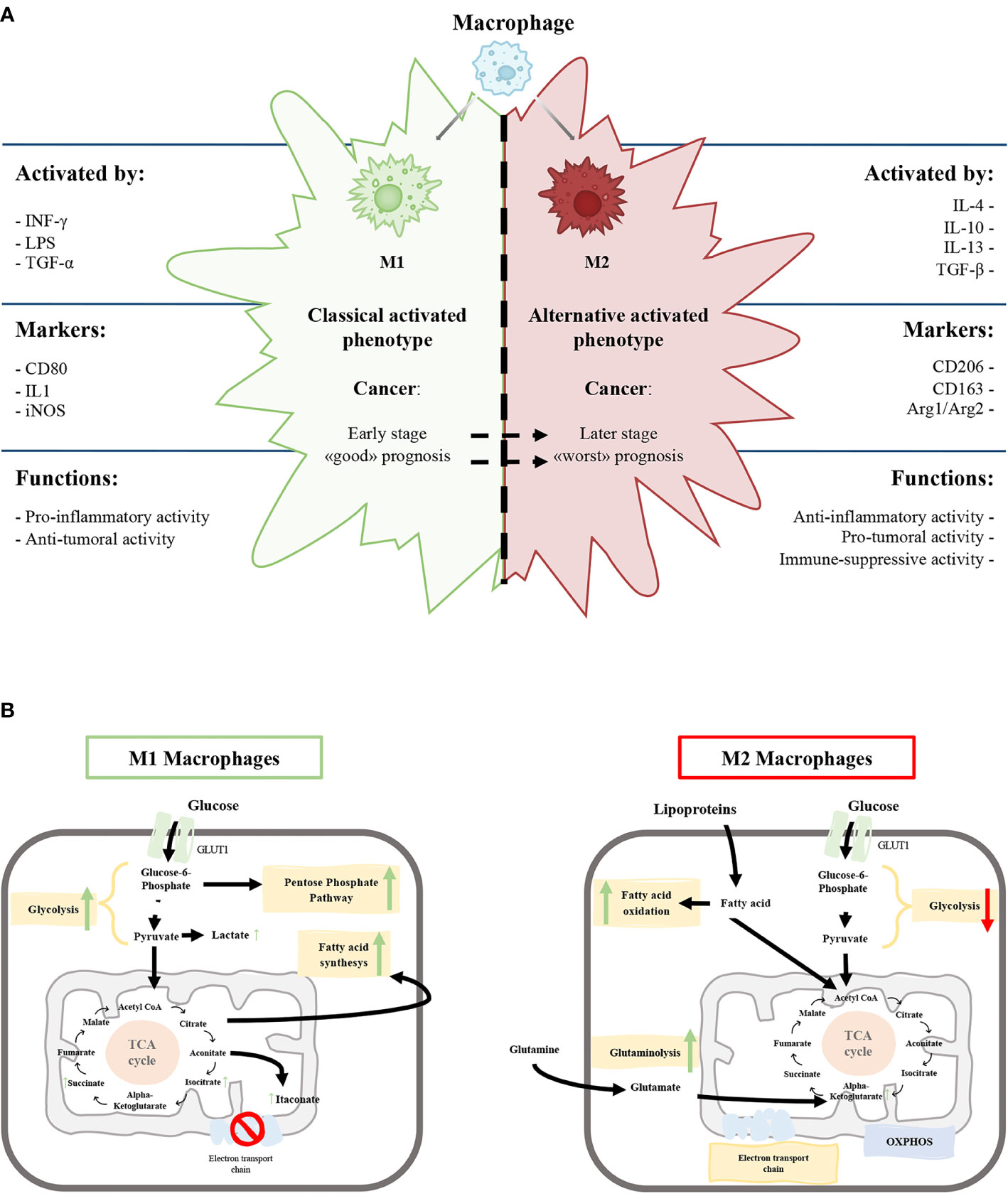
Figure 1 (A) Schematic representation of the characteristics of M1 and M2 macrophages phenotypes. (B) Schematic view of M1 and M2 macrophages metabolism. Green and red arrows indicate an up-regulation and down-regulation of a specific pathway respectively.
The M1 phenotype is induced by microbial products like lipopolysaccharide and cytokines secreted by Th1 lymphocytes, such as Interferon-γ (IFN-γ) and tumor necrosis factor-alpha (TNF-α). On the other hand, anti-inflammatory molecules, such as glucocorticoid hormones and interleukin (IL)-4, IL-10, IL-13, secreted by Th2 lymphocytes, induce the M2 phenotype (Figure 1A) (5, 16, 17).
M1 macrophages express high levels of inducible nitric oxide synthetase (iNOS) that promote the release of nitric oxide, reactive oxygen species and pro-inflammatory cytokines (IL-1β, IL-6, IL-12) promoting Th1 response (2, 13). The high production of anti-microbial and anti-tumoral molecules gives to M1 macrophages the capability to kill intracellular pathogens (5, 18). Classically activated macrophages express specific surface proteins, such as CD68, CD80, and CD86 (Figure 1A).
In contrast, M2 macrophages produce a high amount of anti-inflammatory cytokines (IL-10, TGF-β), low levels of pro-inflammatory cytokines (IL-12) and diminished expression of the NOS enzyme. M2 macrophages have a strong phagocytosis capacity and induce angiogenesis and lymphangiogenesis (2, 19, 20). Furthermore, the arginase pathway induces increased production of ornithine, collagen, and polyamines with an elevated expression of arginase-1 (Arg1) (Figure 1A).
From a biochemical point of view, the metabolism of M1 and M2 activated macrophages were extensively characterized. M1 macrophages metabolism is based on anaerobic glycolysis, truncated tricarboxylic acid cycle (itaconate production), fatty acid synthesis and pentose phosphate pathway (Figure 1B) with, a consequent decrease in oxidative phosphorylation (OXPHOS) and fatty acid oxidation (5, 21–23)
M2 macrophages have a poorly glycolytic profile with higher arginine metabolism and preferential fatty acid oxidation (oxidative glucose metabolism), glutamine metabolism and OXPHOS (Figure 1B) (5, 18, 19, 24). Glycolysis is not essential for M2 phenotype, and glucose is mainly employed to sustain OXPHOS (25–27).
In tumors, monocytes from bone marrow and circulating monocytes are recruited through the release of cytokines and macrophages were functionally polarized in tumor-associated macrophages (TAMs) (9, 28). Within the tumor microenvironment (TME), TAMs exhibit high plasticity and undergo specific functional metabolic alterations according to the availability of tumor tissue oxygen and nutrients, thus further contributing to tumorigenesis and cancer progression.
In this review, we describe the relationship between TAMs metabolic profile and activation states and their involvement in cancer pathologies. We focus on mass spectrometry (MS)-based metabolomics analysis that is able to detect, quantify and map a huge number of molecules for recapitulate the different TAMs metabolic phenotypes (29).
TAMs density and polarization as prognostic marker in cancer pathologies: state of the art
TAMs are one of the most abundant immune myeloid populations in the TME (30). Meta-Analyses indicates that high density of TAM (CD68+) could be associated with worse prognosis in almost all cancers (31–35), contradictory in gastric cancer (32, 36) or should be linked to a favorable prognosis in colorectal cancer (37, 38).
TAMs could exhibit pro-tumoral or anti-tumoral roles depending on the stimuli generated by the TME and can acquire different functions and properties depending on cancer type, grade, and during tumor progression (39). Several studies are committed to quantify and characterize the M1 and M2 TAM phenotype to better refine the prognostic significance of TAMs.
M1-like TAMs as prognostic marker
TAMs can show a M1-like phenotype, in particular during the early stages of tumor development (2, 20, 39, 40), promoting antineoplastic and phagocytose activity. During the tumor progression, the TME produce a large amount of growth factors and anti-inflammatory mediators that induce a shift in TAMs phenotype.
In ovarian cancer, two prospective observational studies (140 Italian and 112 Chinese patients) showed that intratumoral M1/M2 TAM ratio decreased as the cancer stage increased (41) with a significant association between higher M1/M2 TAMs ratio and the longer overall survival and progression-free survival (42),
Similar findings were obtained in Non-Small Cell Lung Cancer (NSCLC) studies, where high infiltration of M1 like TAMs (41 UK and 80 Lithuanian subjects) were associated with a better survival outcomes (43, 44). Consistently with these studies, a high density of iNOS+ M1-like macrophages predicted the improved survival rate in a cohort (40 Finland patients) of HER2+ breast cancer subjects (45). Also considering TAMs morphologies, M1-like macrophages more frequently appear round and flattened, as opposed to M2-like cells that present an elongated morphology. A higher percentage of TAMs with small dimensions and regular shape were associated with a better prognosis in colorectal liver metastasis patients (101 Italian patients) (46).
Overall, these data point out that M1-like TAMs infiltrate may be associated with a favorable survival rate in several cancer pathologies.
M2-like TAMs as prognostic marker
The alternative activated M2-like phenotype promote cancer cells growth through the secretion of pro-angiogenic factors, like vascular endothelial growth factors, and immunosuppressive factors, as IL-10 and TGF-β (47). This M2-like phenotype is considered as pro-tumoral increasing neovascularization, angiogenesis, infiltration and consequently metastatization; has therefore an immune-suppressive activity and antitumor adaptive immune response (14, 16, 26, 48).
High number of M2-like TAMs were observed associated with tumor growth and poor patient prognosis in breast (562 Finland, 144 Sweden patients), NSCLC (349 Korean patients) and melanoma (184 Finland, 94 Italian patients) cancers (49–52).
Overall, these studies highlight that M1 and M2 TAMs may be useful markers of patient prognosis (M1-like better and M2-like worst) regardless of tumor type and patient ethnicity. However, meta-analyses considering prospective studies employing standardized methodology and larger sample sizes are still needed to validate the prognostic significance of M1 and M2 TAMs infiltration.
Metabolism as modulator of macrophage functionality driving the balance between M1-like and M2-like TAMs might lead to different strategies for chemotherapeutic and/or immunotherapeutic approaches.
Mass spectrometry-based metabolomics approaches in cancer research
Metabolomics is the large-scale study of endogenous small molecules of low molecular weight molecules (<1000 Da), commonly known as metabolites, within cells, biofluids, tissues, or organisms. Metabolomics together with genomics, transcriptomics, and proteomics, drive the connection between the genotype and the phenotype in both physiological and pathological processes in order to be a powerful tool in biomarker discovery, developing drugs, and personalized medicine (53–55). Metabolomics is an essential tool for the simultaneous measurement and quantification of thousands of small metabolites from biological matrices, with the possibility to be hypothesis-generating, without a priori knowledge, or hypothesis-driven, with a priori knowledge about the metabolites present in the sample. The applicability of metabolomics in identifying metabolic dysregulation has been demonstrated in a wide range of human diseases, including cardiovascular diseases, diabetes, obesity, and cognitive disorders, but also cancers (54). For this reason, metabolomics could be considered a promising approach for the identification of metabolites acting as biomarkers to allow early diagnosis, to follow pathological processes and progression or response to treatments.
The two main analytical techniques employed for metabolomics profiling are Nuclear Magnetic Resonance (NMR) and Mass Spectrometry (MS), which allow the achievement of both qualitative and quantitative information. We will focus on the role of MS as a powerful tool for the identification of a large set of ionized analytes based on their mass-to-charge ratio (m/z) (53, 56).
Analytical methods for MS-based metabolomics
MS is usually coupled with a chromatographic separation system (hyphenated techniques), such as gas chromatography (GC)-MS, liquid chromatography (LC)-MS [both high-performance LC (HPLC) and ultra-performance LC (UPLC)], and capillary electrophoresis (CE)-MS, but a sample could be also directly injected into the MS (flow-injection analysis-MS) (56, 57). GC-MS is employed for the identification of volatile compounds, like fatty acids and organic acids, requiring a derivatization step before the analysis (58). Electron impact (EI) and chemical ionization (CI) sources are commonly used with a GC separation system (59). However, LC-MS does not require any derivatization and allows the detection of more analytes (60), and it is usually combined with an electrospray (ESI) and CI sources.
CE-MS allows the separation of polar and charged metabolites based on their electrophoretic mobility, an intrinsic property that depends on the size and charge of the molecule (61). To date, MS-based metabolomics strategies are widely employed in the tumor research area to identify biomarkers for prediction, diagnosis, and prognosis (62, 63).
Metabolomics approaches
Untargeted and targeted MS are the two methods used for the identification of endogenous small molecules (58). The untargeted strategy allows the identification of a huge number of metabolites with high accuracy using high-resolution MS, without knowing basic information about the metabolome of the sample.
Orbitrap and Time-of-flight (ToF) are the preferred mass analyzers for high-resolution untargeted metabolomics analysis. Ionized molecules in the orbitrap analyzer are forced to move in complex spiral patterns by the electrostatic field, combining axial oscillations with rotation around the central axis (56). Different frequencies of oscillation are then associated with different m/z. In the ToF analyzer, ions with initial kinetic energy enter a field-free drift region of known length and are dispersed in time based on their different m/z. Ions with the larger m/z arrive at the end of the drift length after ions with a smaller m/z (64).
Then, after the final ion detection, the use of database is required for metabolites identification and annotation [HMDB (65), METLIN (66)]. This annotation is performed by comparing the experimental mass to libraries within a mass tolerance frame, depending on the mass spectrometer used for the analysis (58, 60, 67). On the other hand, the information should be incomplete cause of employed solvents, pH, chromatographic separation, ionization techniques; and the detection of chemical unknowns with no annotation in database. MS/MS fragmentation experiments are then required to confirm the metabolites’ structure, especially to distinguish co-eluting isobaric species (67).
Targeted metabolomics is a hypothesis-driven strategy that allows the identification of a specific set of metabolites from a panel of interest with high accuracy, selectivity and sensitivity (59).
Triple quadrupole (QQQ) and quadrupole ion trap are useful mass analyzers for targeted metabolomics (56, 58). QQQ is the most common type of tandem MS, the first and the third quadrupole are used as mass filters, while the second quadrupole is used as a collision cell to generate fragment ions. Into the Q, ions with a particular range of m/z values have stable periodic trajectories imposed by the direct current and the radio frequency potential of the four rod electrodes composing the Q that periodically changes in time (56, 68). The voltage polarity between two adjacent rods is opposite (64). The quadrupole is often combined with other mass analyzers to filter ions to remove matrix ions such as in Q-Trap.
The ion trap analyzer is a modification of the Q with improved sensibility. The operating principle of Q and ion trap is the same: ions of different m/z are selectively ejected from the analyzer by varying the radio frequency potential (68).
Targeted metabolomics allows the quantification and semi-quantification using internal standards (69) and the Multiple Reaction Monitoring (MRM) approach, performed on QQQ or Q-ion trap, is used for the exact identification of the molecules of interest, selecting specific precursor and product ions.
The number of publications in PubMed related to cancer metabolomics shows that the MS-based approach has been largely preferred over NMR with more than 4000 results obtained for the search query “cancer metabolomics AND mass spectrometry” and less than 1500 results obtained for the search “cancer metabolomics AND NMR”, supporting the higher impact of MS-based techniques on cancer research.
MS-based metabolomics limitations and future perspective
Mass spectrometry (MS) techniques, because of their sensitivity and selectivity, have become methods of choice to characterize the human metabolome, being able to detect and quantify many thousands of metabolite features simultaneously. Because the metabolite composition is central to every living organism the in-depth biological insides, metabolomics can advance research across a variety of scientific areas. However many challenges still exist for a successful metabolomics study. Much has been discussed on this issue elsewhere (67, 70, 71), here we address few of the unique challenges for metabolomics. The first one is analytical, due to the chemical diversity of the metabolites, their wide dynamic range and the confidence for their unambiguous identification. To this purpose, implementation of analytical and bioinformatic tools for the accurate and standardized metabolites identification is a current goal to be achieve for a large-scale coverage of metabolites and to facilitate data processing. Although there is no single analytical platform strategy that provides the complete coverage for the whole metabolome, there are common experimental criteria to all strategies that need to be addressed. Robust metabolomics results hinge on further key elements such as a proper study design (e.g. number of observations, power size, and sampling storage), method optimization, data processing and final validation. The application of this virtuous workflow will help not only in assigning biological meaning to metabolites but also to move towards finding robust mechanism of diseases. Metabolomics as for the other omics, is not a separate discipline, and the implementation of bioinformatic algorithms and computational strategies for multi-omics integration is fundamental to place metabolites in a biological context and to associate metabolites with phenotype causality. This promotes the understanding of metabolome in a system-wide level in order to develop personalized treatments and help in early diagnosis of disease. It is believed that the continuous progress in technologies together with bioinformatics and computational tools will feed metabolomics research to guide not only biomarkers discovery but also to delve and discover mechanisms of disease development and progression.
The MS-based metabolomics profile of TAMs in cancer pathologies
Exploring the biochemical mechanisms underlying TAMs polarization during tumor development and progression could contribute to the development of new therapeutic approaches. In the last few years, metabolism of M1 and M2-like macrophages was mainly studied in in-vitro polarized TAMs (72, 73). Nevertheless, the in-vivo metabolic phenotypes of resident TAMs directly isolated from fresh tumor specimens are not largely explored. Considering the heterogeneity of activation stimuli from TME, deciphering the TAMs metabolism in the tissue has a vital importance in cancer research.
We summarized the scientific analysis of TAMs metabolism obtained by MS-based metabolomics studies in different cancer pathologies. MS-based studies on TAMs metabolism could determinate the metabolic alterations in different metabolic pathways such as glycolysis, mitochondrial machinery, amino acids, and lipid metabolism. These information are critical for identifying new biochemical pathways underlying tumor resident TAMs polarization useful to influence overall patient survival.
Central cellular biochemical pathways in TAMs
Untargeted metabolomics by using CE-ToF-MS of in-vivo sorted murine TAMs from a colon adenocarcinoma xenograft model in both early (14 days after tumor implantation) and late tumor stage (28 days after tumor implantation) showed an increasing of different cellular metabolic pathways (glycolysis, methionine metabolism, TCA cycle, and glutamine and glutamate metabolism) in the tumor resident TAMs relative to myeloid-derived suppressor cells (74). This metabolic asset [increase in oxidative phosphorylation (OXPHOS) and glycolysis] of TAMs was confirmed in peritoneal Mϕ macrophages isolated from naive and ID8 tumor-bearing mice (75). The untargeted metabolomics (GC-TOF) identified itaconic acid, the product of the catabolism of mitochondrial cis-aconitate, the most highly upregulated metabolites in Mϕ macrophages of tumor-bearing mice (75). The high glycolytic activity of TAMs was also observed by untargeted metabolomics (by means of UHPLC-Orbitrap) on in-vitro generated human pancreatic ductal adenocarcinoma (PDAC) TAMs. The mixed M1/M2 TAMs generated from monocytes exposed to PDAC conditioned medium were characterized by elevated glycolysis, increased lactate production and reduced OXPHOS compared to normal macrophages (76).
The reliance on glycolysis of pancreatic cancer derived TAMs was confirmed by untargeted metabolomics (using both LC-ToF-MS and CE-ToF-MS) on TAMs deleted for the Glucose Transporter 1 (GLUT1). Tumor resident GLUT1 deleted TAMs showed the decrement of glycolytic intermediates such as glucose-6-phosphate and fructose 1,6-biphosphate with no substantial difference of the TCA cycle relative to control (77).
For the characterization of metabolic pattern characteristic of M1 and M2-like TAMs, a metabolic flux analysis (13C-labeling experiments coupled with GC-MS) on in-vivo sorted NSCLC resident TAMs (3LL-R Lewis lung murine model) was used. In this model M1-like TAMs (major histocompatibility complex (MHC)-IIhi) display a hampered tricarboxylic acid (TCA) cycle, while M2-like TAMs (MHC-IIlo) show higher OXPHOS and glycolytic metabolism (78). Another metabolic profiling (through UPLC QQQ tandem MS) of in-vitro conditioned PDAC TAMs indicated that nucleoside metabolism is the principal biochemical pathway able to distinguish TAM and M2 macrophages from the M1 (72).
Metabolism of M1 was also studied in ex vivo NSCLC tumor slices. Slices were treated with the macrophage activator β-glucan, and metabolic changes were monitored by metabolic flux analysis (13C6-glucose LC–MS). MS highlighted a higher synthesis of itaconate and higher levels of NADPH in M1-like generated TAMs relative to the general TAMs population (79).
Lipid metabolism in TAMs
Lipidomics is focused on the identification of lipid species present within a cell, organ, or biological system. The lipidome compartment in the cells is composed of several lipid categories (e.g., fatty acids, glycerolipids, sphingolipids, and sterol lipids). Among the metabolic alterations observed in TAMs, lipid metabolism seems to directly affected TAMs polarization and function (80).
Eicosanoids quantification (Target lipodomics by using LC-QQQ tandem MS) on in-vivo sorted tumor resident TAMs populations (resident alveolar macrophages MacA and M2-like TAMs MacB) in orthotropic lung cancer model, showed that M2-like TAMs had low level of eicosanoids production relative to resident alveolar macrophages (81).
Global lipidomics analysis (by using LC-HRMS with Orbitrap) on in-vitro generated TAMs (monocytes exposure to gastric cancer conditioned medium) showed almost 10-fold higher triacylglycerol levels relative to control macrophages (73). This data was also supported by the high amount of total lipid found in in-vivo TAM sorted from both murine and human gastric cancer specimens. The lipid accumulation seems to occur by uptakes of lipids released by cancer cells, that drives TAMs polarization toward an M2‐like profile (73). Finally, target lipidomics (by QTrap analyzer) on conditioned medium from in-vivo sorted TAMs reveal that M2-like TAMs (CD163+CD206+) actively released of PLA2G7 and autotaxin that play an essential role in ovarian cancer invasiveness and metastatic spread (82).
Overall, mass spectrometry-based metabolic profiling of TAMs converges to define the increased glycolytic and OXPHOS activity of TAMs relative to control macrophages also considering the in-vivo sorted and in vitro conditioned TAMs (Table 1). Considering the heterogeneity of the TAMs populations in the cancer microenvironment and the different experimental settings for determining TAMs subpopulation, it is difficult to speculate the attribution of the augmented metabolism at a particular TAMs populations. It is possible to speculate that M2-like TAMs identified as CD11b+Ly6G−Ly6ClowMHC-IIhigh (78) rely on glycolysis, mitochondrial machinery, and lipid metabolism to sustain their functionality (Table 1). On the contrary, M1-like TAMs, when compared to the general control macrophages cells, seem be less metabolic active with a hampered OXPHOS capacity (Table 1). These data only partially agree with the metabolic characteristics of M1, and M2 polarized macrophages. Indeed, the M2 phenotype is mainly defined by a poor glycolytic profile with higher arginine metabolism and preferential fatty acid oxidation (oxidative glucose metabolism) and OXPHOS (5, 19) (Figure 1).
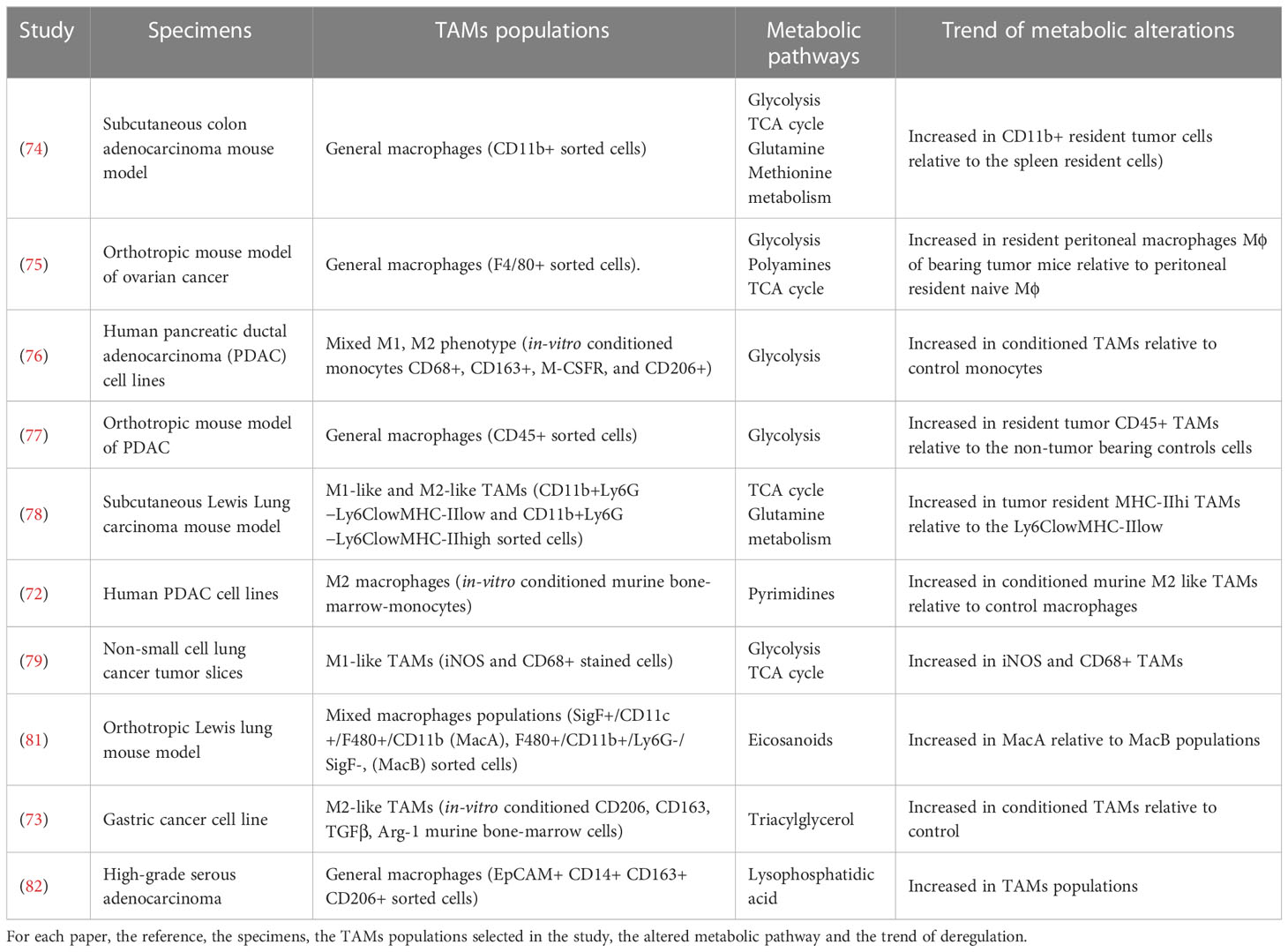
Table 1 List of papers employing mass spectrometry based metabolomics approaches to describe the metabolic state of TAMs populations.
Discussion
TAMs are one of the most common immune cells in the TME and they are characterized by a great plasticity and adaptability to the environment they infiltrate by re-programming their activation state towards a pro- (M1) or an anti- (M2) inflammatory phenotype (26). Macrophages polarization in various malignancies appears to be complex, and the M1 to M2 phenotype switching can occur during cancer progression. M1-like and M2-like TAMs can be co-expressed inside the tumor environment and their ratio can change during cancer progression. A high amount of M1-like TAMs is typical of the early stages of tumors development and it is associated with good prognosis in several malignancies, while M2-like TAMs have a strong correlation with worst prognosis and advanced tumor stage. Understanding and deciphering the complexity of metabolic mechanisms involved in TAMs polarization could be a useful strategy to target cancer. Mass spectrometry-based metabolomics/lipidomics provide the capability to profile and detail the metabolic alterations in TAMs providing new mechanistic hypothesis for find more effective TAMs-targeted therapy. The collected results point to the fact that there are few articles dissecting the metabolic characteristics of the in-vivo derived human TAMs populations and up to now it is only possible to state that in-vivo patient derived TAMs populations (mixed M1- M2-like phenotypes) had an augmented metabolic activity that not completely recapitulate the M1, M2 macrophages metabolic peculiarity. Of note, current evidence based on MS-metabolomics studies are still limited and mostly focused in comparing TAMs metabolism relative to non-tumor counterparts, thus not allowing an in-depth metabolic characterization of TAMs subset. So far, metabolic profiling is mainly related to the general description of metabolic pathways and does not go deeply into the definition of the enzyme activity to discover new fragile metabolic points that might be pharmacologically targeted. Considering the technological advances in the field of cytofluorimetry and mass spectrometry, it is urgent and feasible to investigate the metabolic structure of TAMs sorted directly from patients, without having to analyze orthotropic mouse models or in vitro conditioned monocytes.
Therefore, more effort is needed to implement bulk metabolic analyses such as targeted metabolomics, 13C-label-tracing, and extracellular flux analysis to provide the complete metabolic picture and address the therapeutic implications of TAMs metabolism.
Author contributions
Conceptualization, all authors. Project administration, MD. Resources, MD. Supervision, LB. Writing original draft, GD and LB. Review and editing, all authors. All authors contributed to the article and approved the submitted version.
Funding
This study was supported by Italian Ministry of Health grant number RF-2018-12367150.
Conflict of interest
The authors declare that the research was conducted in the absence of any commercial or financial relationships that could be construed as a potential conflict of interest.
Publisher’s note
All claims expressed in this article are solely those of the authors and do not necessarily represent those of their affiliated organizations, or those of the publisher, the editors and the reviewers. Any product that may be evaluated in this article, or claim that may be made by its manufacturer, is not guaranteed or endorsed by the publisher.
References
1. Castegna A, Gissi R, Menga A, Montopoli M, Favia M, Viola A, et al. Pharmacological targets of metabolism in disease: opportunities from macrophages. Pharmacol Ther (2020) 210:107521. doi: 10.1016/j.pharmthera.2020.107521
2. Petty AJ, Yang Y. Tumor-associated macrophages: implications in cancer immunotherapy. Immunotherapy (2017) 9(3):289–302. doi: 10.2217/imt-2016-0135
3. Chanmee T, Ontong P, Konno K, Itano N. Tumor-associated macrophages as major players in the tumor microenvironment. Cancers (2014) 6(3):1670–90. doi: 10.3390/cancers6031670
4. Murray PJ, Wynn TA. Protective and pathogenic functions of macrophage subsets. Nat Rev Immunol (2011) 11(11):723–37. doi: 10.1038/nri3073
5. Viola A, Munari F, Sánchez-Rodríguez R, Scolaro T, Castegna A. The metabolic signature of macrophage responses. Front Immunol (2019) 10:1462. doi: 10.3389/fimmu.2019.01462
6. Dey A, Allen J, Hankey-Giblin PA. Ontogeny and polarization of macrophages in inflammation: blood monocytes versus tissue macrophages. Front Immunol (2015) 5:683/abstract. doi: 10.3389/fimmu.2014.00683/abstract
7. Cassetta L, Pollard JW. Tumor-associated macrophages. Curr Biol (2020) 30(6):R246–8. doi: 10.1016/j.cub.2020.01.031
8. Cassetta L, Fragkogianni S, Sims AH, Swierczak A, Forrester LM, Zhang H, et al. Human tumor-associated macrophage and monocyte transcriptional landscapes reveal cancer-specific reprogramming, biomarkers, and therapeutic targets. Cancer Cell (2019) 35(4):588–602.e10. doi: 10.1016/j.ccell.2019.02.009
9. Joyce JA, Fearon DT. T Cell exclusion, immune privilege, and the tumor microenvironment. Science (2015) 348(6230):74–80. doi: 10.1126/science.aaa6204
10. Biswas SK, Mantovani A. Macrophage plasticity and interaction with lymphocyte subsets: cancer as a paradigm. Nat Immunol (2010) 11(10):889–96. doi: 10.1038/ni.1937
11. Cortese N, Donadon M, Rigamonti A, Marchesi F. Macrophages at the crossroads of anticancer strategies. Front Biosci Landmark Ed (2019) 24(7):1271–83. doi: 10.2741/4779
12. Murray PJ. Macrophage polarization. Annu Rev Physiol (2017) 79(1):541–66. doi: 10.1146/annurev-physiol-022516-034339
13. Mosser DM, Edwards JP. Exploring the full spectrum of macrophage activation. Nat Rev Immunol (2008) 8(12):958–69. doi: 10.1038/nri2448
14. Mantovani A, Sozzani S, Locati M, Allavena P, Sica A. Macrophage polarization: tumor-associated macrophages as a paradigm for polarized M2 mononuclear phagocytes. Trends Immunol (2002) 23(11):549–55. doi: 10.1016/S1471-4906(02)02302-5
15. Kodelja V, Müller C, Tenorio S, Schebesch C, Orfanos CE, Goerdt S. Differences in angiogenic potential of classically vs alternatively activated macrophages. Immunobiology (1997) 197(5):478–93. doi: 10.1016/S0171-2985(97)80080-0
16. Mojsilovic SS, Mojsilovic S, Villar VH, Santibanez JF. The metabolic features of tumor-associated macrophages: opportunities for immunotherapy? Anal Cell Pathol (2021) 2021:1–12. doi: 10.1155/2021/5523055
17. Mantovani A, Sica A, Sozzani S, Allavena P, Vecchi A, Locati M. The chemokine system in diverse forms of macrophage activation and polarization. Trends Immunol (2004) 25(12):677–86. doi: 10.1016/j.it.2004.09.015
18. Anders CB, Lawton TMW, Smith HL, Garret J, Doucette MM, Ammons MCB. Use of integrated metabolomics, transcriptomics, and signal protein profile to characterize the effector function and associated metabotype of polarized macrophage phenotypes. J Leukoc Biol (2021), 6A1120–744R. doi: 10.1101/2020.03.10.985788
19. Shapouri-Moghaddam A, Mohammadian S, Vazini H, Taghadosi M, Esmaeili S, Mardani F, et al. Macrophage plasticity, polarization, and function in health and disease. J Cell Physiol (2018) 233(9):6425–40. doi: 10.1002/jcp.26429
20. Sica A, Mantovani A. Macrophage plasticity and polarization: in vivo veritas. J Clin Invest (2012) 122(3):787–95. doi: 10.1172/JCI59643
21. Xia Y, Brown ZJ, Huang H, Tsung A. Metabolic reprogramming of immune cells: shaping the tumor microenvironment in hepatocellular carcinoma. Cancer Med (2021) 10(18):6374–83. doi: 10.1002/cam4.4177
22. Zhu L, Zhao Q, Yang T, Ding W, Zhao Y. Cellular metabolism and macrophage functional polarization. Int Rev Immunol (2015) 34(1):82–100. doi: 10.3109/08830185.2014.969421
23. Van den Bossche J, O’Neill LA, Menon D. Macrophage immunometabolism: where are we (Going)? Trends Immunol (2017) 38(6):395–406. doi: 10.1016/j.it.2017.03.001
24. Noe JT, Rendon BE, Geller AE, Conroy LR, Morrissey SM, Young LEA, et al. Lactate supports a metabolic-epigenetic link in macrophage polarization. Sci Adv (2021) 7(46):eabi8602. doi: 10.1126/sciadv.abi8602
25. Zhang Q, Wang J, Yadav DK, Bai X, Liang T. Glucose metabolism: the metabolic signature of tumor associated macrophage. Front Immunol (2021) 12:702580. doi: 10.3389/fimmu.2021.702580
26. Vitale I, Manic G, Coussens LM, Kroemer G, Galluzzi L. Macrophages and metabolism in the tumor microenvironment. Cell Metab (2019) 30(1):36–50. doi: 10.1016/j.cmet.2019.06.001
27. Locati M, Curtale G, Mantovani A. Diversity, mechanisms, and significance of macrophage plasticity. Annu Rev Pathol Mech Dis (2020) 15(1):123–47. doi: 10.1146/annurev-pathmechdis-012418-012718
28. Zhou J, Tang Z, Gao S, Li C, Feng Y, Zhou X. Tumor-associated macrophages: recent insights and therapies. Front Oncol (2020) 10:188. doi: 10.3389/fonc.2020.00188
29. Cui L, Lu H, Lee YH. Challenges and emergent solutions for LC-MS/MS based untargeted metabolomics in diseases. Mass Spectrom Rev (2018) 37(6):772–92. doi: 10.1002/mas.21562
30. Rabold K, Aschenbrenner A, Thiele C, Boahen CK, Schiltmans A, Smit JWA, et al. Enhanced lipid biosynthesis in human tumor-induced macrophages contributes to their protumoral characteristics. J Immunother Cancer (2020) 8(2):e000638. doi: 10.1136/jitc-2020-000638
31. Chen YL. Prognostic significance of tumor-associated macrophages in patients with nasopharyngeal carcinoma: a meta-analysis. Med (Baltimore) (2020) 99(39):e21999. doi: 10.1097/MD.0000000000021999
32. Wang XL, Jiang JT, Wu CP. Prognostic significance of tumor-associated macrophage infiltration in gastric cancer: a meta-analysis. Genet Mol Res (2016) 15(4). doi: 10.4238/gmr15049040
33. Guo B, Cen H, Tan X, Ke Q. Meta-analysis of the prognostic and clinical value of tumor-associated macrophages in adult classical Hodgkin lymphoma. BMC Med (2016) 14(1):159. doi: 10.1186/s12916-016-0711-6
34. Wu P, Wu D, Zhao L, Huang L, Chen G, Shen G, et al. Inverse role of distinct subsets and distribution of macrophage in lung cancer prognosis: a meta-analysis. Oncotarget (2016) 7(26):40451–60. doi: 10.18632/oncotarget.9625
35. Zhang Qw, Liu L, Gong Cy, Shi Hs, Zeng Yh, Wang X, et al. Prognostic significance of tumor-associated macrophages in solid tumor: a meta-analysis of the literature. hoque MO. PloS One (2012) 7(12):e50946. doi: 10.1371/journal.pone.0050946
36. Liu JY, Yang XJ, Geng XF, Huang CQ, Yu Y, Li Y. Prognostic significance of tumor-associated macrophages density in gastric cancer: a systemic review and meta-analysis. Minerva Med (2016) 107(5):314–21.
37. Li J, Li L, Li Y, Long Y, Zhao Q, Ouyang Y, et al. Tumor-associated macrophage infiltration and prognosis in colorectal cancer: systematic review and meta-analysis. Int J Colorectal Dis (2020) 35(7):1203–10. doi: 10.1007/s00384-020-03593-z
38. Cortese N, Soldani C, Franceschini B, Barbagallo M, Marchesi F, Torzilli G, et al. Macrophages in colorectal cancer liver metastases. Cancers (2019) 11(5):633. doi: 10.3390/cancers11050633
39. Sica A, Erreni M, Allavena P, Porta C. Macrophage polarization in pathology. Cell Mol Life Sci (2015) 72(21):4111–26. doi: 10.1007/s00018-015-1995-y
40. Movahedi K, Laoui D, Gysemans C, Baeten M, Stangé G, Van den Bossche J, et al. Different tumor microenvironments contain functionally distinct subsets of macrophages derived from Ly6C(high) monocytes. Cancer Res (2010) 70(14):5728–39. doi: 10.1158/0008-5472.CAN-09-4672
41. Zhang M, He Y, Sun X, Li Q, Wang W, Zhao A, et al. A high M1/M2 ratio of tumor-associated macrophages is associated with extended survival in ovarian cancer patients. J Ovarian Res (2014) 7(1):19. doi: 10.1186/1757-2215-7-19
42. Macciò A, Gramignano G, Cherchi MC, Tanca L, Melis L, Madeddu C. Role of M1-polarized tumor-associated macrophages in the prognosis of advanced ovarian cancer patients. Sci Rep (2020) 10(1):6096. doi: 10.1038/s41598-020-63276-1
43. Garrido-Martin EM, Mellows TWP, Clarke J, Ganesan AP, Wood O, Cazaly A, et al. M1 hot tumor-associated macrophages boost tissue-resident memory T cells infiltration and survival in human lung cancer. J Immunother Cancer (2020) 8(2):e000778. doi: 10.1136/jitc-2020-000778
44. Jackute J, Zemaitis M, Pranys D, Sitkauskiene B, Miliauskas S, Vaitkiene S, et al. Distribution of M1 and M2 macrophages in tumor islets and stroma in relation to prognosis of non-small cell lung cancer. BMC Immunol (2018) 19(1):3. doi: 10.1186/s12865-018-0241-4
45. Honkanen TJ, Tikkanen A, Karihtala P, Mäkinen M, Väyrynen JP, Koivunen JP. Prognostic and predictive role of tumour-associated macrophages in HER2 positive breast cancer. Sci Rep (2019) 9(1):10961. doi: 10.1038/s41598-019-47375-2
46. Donadon M, Torzilli G, Cortese N, Soldani C, Di Tommaso L, Franceschini B, et al. Macrophage morphology correlates with single-cell diversity and prognosis in colorectal liver metastasis. J Exp Med (2020) 217(11):e20191847. doi: 10.1084/jem.20191847
47. Ostuni R, Kratochvill F, Murray PJ, Natoli G. Macrophages and cancer: from mechanisms to therapeutic implications. Trends Immunol (2015) 36(4):229–39. doi: 10.1016/j.it.2015.02.004
48. Shu Y, Cheng P. Targeting tumor-associated macrophages for cancer immunotherapy. Biochim Biophys Acta BBA - Rev Cancer (2020) 1874(2):188434. doi: 10.1016/j.bbcan.2020.188434
49. Sousa S, Brion R, Lintunen M, Kronqvist P, Sandholm J, Mönkkönen J, et al. Human breast cancer cells educate macrophages toward the M2 activation status. Breast Cancer Res (2015) 17(1):101. doi: 10.1186/s13058-015-0621-0
50. Medrek C, Pontén F, Jirström K, Leandersson K. The presence of tumor associated macrophages in tumor stroma as a prognostic marker for breast cancer patients. BMC Cancer (2012) 12(1):306. doi: 10.1186/1471-2407-12-306
51. Salmi S, Siiskonen H, Sironen R, Tyynelä-Korhonen K, Hirschovits-Gerz B, Valkonen M, et al. The number and localization of CD68+ and CD163+ macrophages in different stages of cutaneous melanoma. Melanoma Res (2019) 29(3):237–47. doi: 10.1097/CMR.0000000000000522
52. Falleni M, Savi F, Tosi D, Agape E, Cerri A, Moneghini L, et al. M1 and M2 macrophages’ clinicopathological significance in cutaneous melanoma. Melanoma Res (2017) 27(3):200–10. doi: 10.1097/CMR.0000000000000352
53. Ciocan-Cartita CA, Jurj A, Buse M, Gulei D, Braicu C, Raduly L, et al. The relevance of mass spectrometry analysis for personalized medicine through its successful application in cancer “Omics”. Int J Mol Sci (2019) 20(10):2576. doi: 10.3390/ijms20102576
54. Hu R, Li Y, Yang Y, Liu M. Mass spectrometry-based strategies for single-cell metabolomics. Mass Spectrom Rev (2021) 42(1):67–94. doi: 10.1002/mas.21704
55. Griffiths WJ, Koal T, Wang Y, Kohl M, Enot DP, Deigner HP. Targeted metabolomics for biomarker discovery. Angew Chem Int Ed (2010) 49(32):5426–45. doi: 10.1002/anie.200905579
57. Chen JH, Singer S. Chromatographic and electrophoretic separations combined with mass spectrometry for metabonomics. In: The handbook of metabonomics and metabolomics. Elsevier (2007). p. 149–69. Available at: https://www.sciencedirect.com/book/9780444528414/the-handbook-of-metabonomics-and-metabolomics.
58. Johnson CH, Ivanisevic J, Siuzdak G. Metabolomics: beyond biomarkers and towards mechanisms. Nat Rev Mol Cell Biol (2016) 17(7):451–9. doi: 10.1038/nrm.2016.25
59. Dudley E, Yousef M, Wang Y, Griffiths WJ. Targeted metabolomics and mass spectrometry. In: Advances in protein chemistry and structural biology. Elsevier (2010). p. 45–83. Available at: https://linkinghub.elsevier.com/retrieve/pii/B9780123812643000023.
60. Cheung PK, Ma MH, Tse HF, Yeung KF, Tsang HF, Chu MKM, et al. The applications of metabolomics in the molecular diagnostics of cancer. Expert Rev Mol Diagn (2019) 19(9):785–93. doi: 10.1080/14737159.2019.1656530
61. Zhang W, Hankemeier T, Ramautar R. Next-generation capillary electrophoresis–mass spectrometry approaches in metabolomics. Curr Opin Biotechnol (2017) 43:1–7. doi: 10.1016/j.copbio.2016.07.002
62. Gao P, Huang X, Fang XY, Zheng H, Cai SL, Sun AJ, et al. Application of metabolomics in clinical and laboratory gastrointestinal oncology. World J Gastrointest Oncol (2021) 13(6):536–49. doi: 10.4251/wjgo.v13.i6.536
63. Gonzalez-Covarrubias V, Martínez-Martínez E, del Bosque-Plata L. The potential of metabolomics in biomedical applications. Metabolites (2022) 12(2):194. doi: 10.3390/metabo12020194
64. Li C, Chu S, Tan S, Yin X, Jiang Y, Dai X, et al. Towards higher sensitivity of mass spectrometry: a perspective from the mass analyzers. Front Chem (2021) 9:813359. doi: 10.3389/fchem.2021.813359
65. Wishart DS, Guo A, Oler E, Wang F, Anjum A, Peters H, et al. HMDB 5.0: the human metabolome database for 2022. Nucleic Acids Res (2022) 50(D1):D622–31. doi: 10.1093/nar/gkab1062
66. Guijas C, Montenegro-Burke JR, Domingo-Almenara X, Palermo A, Warth B, Hermann G, et al. METLIN: a technology platform for identifying knowns and unknowns. Anal Chem (2018) 90(5):3156–64. doi: 10.1021/acs.analchem.7b04424
67. Schrimpe-Rutledge AC, Codreanu SG, Sherrod SD, McLean JA. Untargeted metabolomics strategies–challenges and emerging directions. J Am Soc Mass Spectrom (2016) 27(12):1897–905. doi: 10.1007/s13361-016-1469-y
68. Mirzaei H, Carrasco M. Modern proteomics – sample preparation, analysis and practical applications. In: Advances in experimental medicine and biology (2016). vol. 919. Cham: Springer International Publishing. doi: 10.1007/978-3-319-41448-5
69. Roberts LD, Souza AL, Gerszten RE, Clish CB. Targeted metabolomics. Curr Protoc Mol Biol (2012) 98(1):1–24. doi: 10.1002/0471142727.mb3002s98
70. Zhou J, Zhong L. Applications of liquid chromatography-mass spectrometry based metabolomics in predictive and personalized medicine. Front Mol Biosci (2022) 9:1049016. doi: 10.3389/fmolb.2022.1049016
71. Rampler E, Abiead YE, Schoeny H, Rusz M, Hildebrand F, Fitz V, et al. Recurrent topics in mass spectrometry-based metabolomics and lipidomics-standardization, coverage, and throughput. Anal Chem (2021) 93(1):519–45. doi: 10.1021/acs.analchem.0c04698
72. Halbrook CJ, Pontious C, Kovalenko I, Lapienyte L, Dreyer S, Lee HJ, et al. Macrophage-released pyrimidines inhibit gemcitabine therapy in pancreatic cancer. Cell Metab (2019) 29(6):1390–1399.e6. doi: 10.1016/j.cmet.2019.02.001
73. Luo Q, Zheng N, Jiang L, Wang T, Zhang P, Liu Y, et al. Lipid accumulation in macrophages confers protumorigenic polarization and immunity in gastric cancer. Cancer Sci (2020) 111(11):4000–11. doi: 10.1111/cas.14616
74. Umemura N, Sugimoto M, Kitoh Y, Saio M, Sakagami H. Metabolomic profiling of tumor-infiltrating macrophages during tumor growth. Cancer Immunol Immunother (2020) 69(11):2357–69. doi: 10.1007/s00262-020-02622-8
75. Weiss JM, Davies LC, Karwan M, Ileva L, Ozaki MK, Cheng RY, et al. Itaconic acid mediates crosstalk between macrophage metabolism and peritoneal tumors. J Clin Invest (2018) 128(9):3794–805. doi: 10.1172/JCI99169
76. Penny HL, Sieow JL, Adriani G, Yeap WH, See Chi Ee P, San Luis B, et al. Warburg metabolism in tumor-conditioned macrophages promotes metastasis in human pancreatic ductal adenocarcinoma. OncoImmunology (2016) 5(8):e1191731. doi: 10.1080/2162402X.2016.1191731
77. Penny HL, Sieow JL, Gun SY, Lau MC, Lee B, Tan J, et al. Targeting glycolysis in macrophages confers protection against pancreatic ductal adenocarcinoma. Int J Mol Sci (2021) 22(12):6350. doi: 10.3390/ijms22126350
78. Geeraerts X, Fernández-Garcia J, Hartmann FJ, de Goede KE, Martens L, Elkrim Y, et al. Macrophages are metabolically heterogeneous within the tumor microenvironment. Cell Rep (2021) 37(13):110171. doi: 10.1016/j.celrep.2021.110171
79. Fan TWM, Warmoes MO, Sun Q, Song H, Turchan-Cholewo J, Martin JT, et al. Distinctly perturbed metabolic networks underlie differential tumor tissue damages induced by immune modulator β-glucan in a two-case ex vivo non-small-cell lung cancer study. Mol Case Stud (2016) 2(4):a000893. doi: 10.1101/mcs.a000893
80. Li Y. Lipid metabolism in tumor immunity. In: Advances in experimental medicine and biology, vol. 1316. . Singapore: Springer Singapore (2021). doi: 10.1007/978-981-33-6785-2
81. Poczobutt JM, Gijon M, Amin J, Hanson D, Li H, Walker D, et al. Eicosanoid profiling in an orthotopic model of lung cancer progression by mass spectrometry demonstrates selective production of leukotrienes by inflammatory cells of the microenvironment. PloS One (2013) 8(11):e79633. doi: 10.1371/journal.pone.0079633
Keywords: tumor associated macrophages (TAMs), prognostic markers, metabolism, mass spectrometry, TAM polarization
Citation: De Simone G, Soldani C, Morabito A, Franceschini B, Ferlan F, Costa G, Pastorelli R, Donadon M and Brunelli L (2023) Implication of metabolism in the polarization of tumor-associated-macrophages: the mass spectrometry-based point of view. Front. Immunol. 14:1193235. doi: 10.3389/fimmu.2023.1193235
Received: 24 March 2023; Accepted: 22 June 2023;
Published: 12 July 2023.
Edited by:
Soumya R. Mohapatra, KIIT University, IndiaReviewed by:
Remya Raja, Mayo Clinic Arizona, United StatesCopyright © 2023 De Simone, Soldani, Morabito, Franceschini, Ferlan, Costa, Pastorelli, Donadon and Brunelli. This is an open-access article distributed under the terms of the Creative Commons Attribution License (CC BY). The use, distribution or reproduction in other forums is permitted, provided the original author(s) and the copyright owner(s) are credited and that the original publication in this journal is cited, in accordance with accepted academic practice. No use, distribution or reproduction is permitted which does not comply with these terms.
*Correspondence: Laura Brunelli, bGF1cmEuYnJ1bmVsbGlAbWFyaW9uZWdyaS5pdA==; Matteo Donadon, bWF0dGVvLmRvbmFkb25AdW5pdXBvLml0
†These authors have contributed equally to this work and share senior authorship