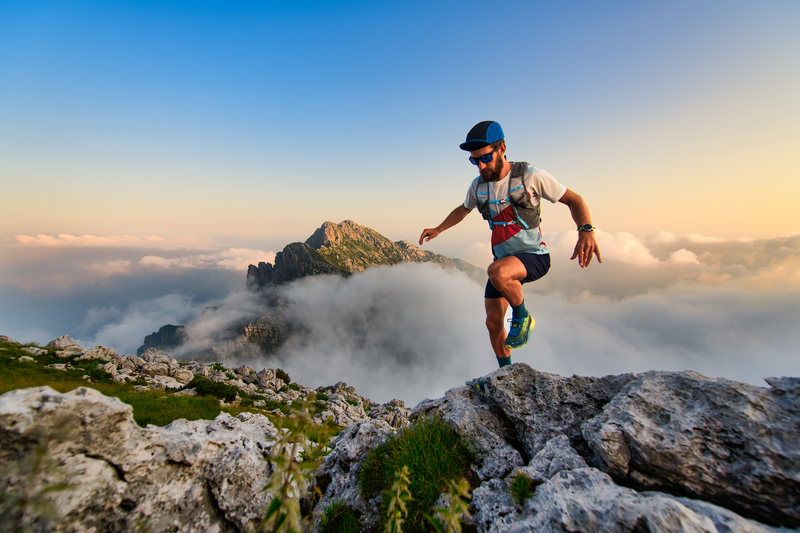
95% of researchers rate our articles as excellent or good
Learn more about the work of our research integrity team to safeguard the quality of each article we publish.
Find out more
ORIGINAL RESEARCH article
Front. Immunol. , 09 October 2023
Sec. Viral Immunology
Volume 14 - 2023 | https://doi.org/10.3389/fimmu.2023.1183489
This article is part of the Research Topic Immunogenetics in times of COVID-19 pandemic View all 20 articles
Background: Sepsis and COVID-19 have a well-established observable relationship. Whether COVID-19 increases the likelihood of developing sepsis and whether patients with sepsis are at increased risk for COVID-19 infection is unknown. Using a bidirectional 2-sample Mendelian randomization (TSMR) analysis techniques in sizable cohorts, we sought to answer this question.
Methods: The current study performed Mendelian randomization (MR) on publicly accessible genome-wide association study (GWAS) summary data in order to investigate the causal linkages between COVID-19 and sepsis. A Two-Sample MR(TSMR) analyses was performed. As instrumental variables, a COVID-19 dataset of single nucleotide polymorphisms (SNPs) with significance value smaller than 5*10-8 was employed and Sepsis dataset of SNPs with significance value smaller than 5*10-7was employed.
Results: The results suggested that Very severe respiratory confirmed COVID-19(VSRC), hospitalized COVID-19(HC) and Infected COVID-19(IC) had no causal influence on sepsis risk using the inverse variance weighted (IVW) technique (VSRC OR = 1.000, 95% CI, 0.956-1.046, P = 0.996, HC OR = 0.976, 95% CI, 0.920-1.036, P = 0.430, IC OR = 0.923, 95% CI, 0.796-1.071, P = 0.291) and there was no causal effect of sepsis on the risk of VSRC, HC and IC (VSRC OR = 0.955, 95% CI, 0.844-1.173, P = 0.953, HC OR = 0.993, 95% CI, 0.859-1.147, P = 0.921, IC OR = 1.001, 95% CI, 0.959-1.045, P = 0.961).
Conclusions: Our findings do not support a causal relationship between COVID-19 and sepsis risk, nor do they suggest a causal link between sepsis and COVID-19. The bidirectional relationship between COVID-19 and sepsis warrants further investigation in large cohorts.
The coronavirus disease 2019 (COVID19), also called as the severe acute respiratory syndrome coronavirus 2 (SARS-CoV2)-induced respiratory distress syndrome, was discovered in humans in late 2019 (1). SARS-CoV-2 infection is very heterogeneous in both susceptibility and severity, with clinical severity (2, 3) ranging from asymptomatic infection to fatal respiratory failure (4). Severe COVID-19 has been linked in several studies to older age, male sex, and a number of comorbidities, including obesity, type 2 diabetes, cardiovascular disease, and hypertension (5–12). Millions of people have been impacted by the ongoing COVID-19 epidemic, which poses a major risk to public health.
Sepsis is one of the main causes of infection-related death and is a severe, potentially fatal clinical syndrome. Bacterial pathogens are frequently implicated in the development of sepsis, but other pathogens such as viruses, fungi, and bacteria can also cause it (13). In the 2016 revision of the Surviving Sepsis Campaign(SSC), sepsis is now described as a potentially fatal organ failure brought on by a dysregulated host reaction to infection (14). According to information acquired from multiple cohorts, respiratory failure and the start of sepsis are the leading causes of mortality from COVID-19 (15). Sepsis has really been detected in nearly all patients who passed away in several of the groups that have been studied (16–18). While the sepsis statistics were inconsistently correlated with the bacterial discoveries in the microbiological work-up, recent research proposed SARS-CoV-2 as the causative agent of this systemic disease (19). Although the above study shows that there is an association between SARS-CoV-2 and sepsis, whether SARS-CoV-2 has a causal relationship with the occurrence and development of sepsis remains to be further verified.
The purpose of this study is to investigate the bidirectional causal effects between SARS-CoV-2 infection and sepsis development. Confounding factors are lessened by a method known as Mendelian randomization (MR) (20), which incorporates summary data from genome-wide association studies (GWAS). MR is a well-liked method for figuring out whether exposure and complicated outcomes have any causal relationships. The selection of instrumental variables (IVs) comprises genetic variations that are closely connected to exposure in order to infer causality (21). If the exposure is causal, the instrumental variables influencing the exposure will have an impact on the results. Elucidating the causal nature of this relationship is critical to understanding COVID-19 pathogenesis and improving treatment strategies.
We use COVID-19 severity including Very severe respiratory confirmed COVID-19(VSRC), hospitalized COVID-19(HC) and Infected COVID-19(IC) as “exposure,” respectively, and Sepsis as “outcome,” to screen out the instrumental variables for bidirectional Mendelian randomization analysis, To minimize type I error and assess heterogeneity, we adopted Bonferroni correction for significance thresholds and performed Cochran’s Q test. To validate the robustness of causal conclusions, we conducted sensitivity analyses including horizontal pleiotropy analysis using MR-Egger regression and leave-one-out analysis. We then performed reverse MR with Sepsis as “exposure” and COVID-19 severity as “outcomes.” Valid MR analysis requires the following key assumptions: (1) The genetic variants used as instrumental variables must demonstrate robust associations with the exposure; (2) The genetic instruments should not be correlated with any confounders of the exposure-outcome relationship; (3) The genetic variants shall affect the outcome only through the exposure, without exerting horizontal pleiotropic effects (Figure 1). In this study, bidirectional Mendelian randomization was used to evaluate the causal connection between COVID-19 severity and sepsis.
Figure 1 Two-sample bidirectional Mendelian randomization framework and assumptions of sepsis and COVID-19 severity.
The IEU Open GWAS with summary-level data, which includes 10,154 explicit sepsis cases and 454,764 controls, was used to obtain data on the genetic predisposition to sepsis. They were developed by the MRC Integrative Epidemiology Unit (MRC-IEU) consortium, which is based on the UK Biobank (http://www.nealelab.is/uk-biobank). We used information for COVID-19 from the COVID-19 Host Genetics Initiative (HGI, https://www.covid19hg.org/) Release 7, a worldwide partnership to support COVID-19 genetics research (22). To reduce the possibility of sample overlap with GWAS of sepsis, we chose the sets of GWAS summary statistics that did not include the UK Biobank (UKBB) sample. Three separate COVID-19 results were assessed: Hospitalized COVID-19 patients (n = 40929) compared with community-based controls (n = 1924400); Very severe respiratory confirmed COVID-19 patients (n = 17472) compared with population-based controls (n = 2357647); Infected COVID-19 population (n = 143839) compared with population based controls (n =2357647).
In order to ensure the accuracy and validity of the findings on the causal relationship between COVID-19 and sepsis, quality check techniques were used to choose the suitable instrument variables. A group of COVID-19 SNPs with P-values less than 5*10-8 and sepsis SNPs less than 5*10-7 were chosen as instrumental variables. Second, since considerable LD might result in skewed results, the lack of linkage disequilibrium (LD) among the chosen instrumental variable is a fundamental guiding principle of the MR technique. In the current study, the degree of LD between the additional SNPs was examined using the clumping approach [R2 0.01 and clump window = 10,000kb, with European reference panel from the 1000 Genomes Project (23)]. Third, a crucial component of MR is determining whether the effects of SNPs in exposure correspond to the same genotype as the effects on outcome. According to the notion, the instrumental variables wouldn’t contain palindromic SNPs. Fourth, in cases where GWAS data lacked SNPs associated with exposure, proxy SNPs with (R2 > 0.8) significantly associated with the relevant variations were chosen.
To reduce the effects of bias on the results, the MR technique must adhere to three key presumptions. First off, instrumental variables that alter exposure and result have no effect on other instrumental variables. Second, the variations of interest should be closely related to the study’s exposure. When evaluating how strongly instrumental variables and exposure relate to one another, the F value is frequently used. F = R2(n-k-1)/k(1-R2) is the formula for the F statistic (24). The sample size is N, the quantity of the instrumental variable is k, and the treatment variation, which the selected SNPs represent, is R2. When F value is less than 10, the relationship between the instrumental variable and the exposure is poor. Third, because instrumental variables only influence outcomes through exposure, there is no horizontal pleiotropy effect between instrumental variables and outcomes.
To evaluate the causal effects of exposures on outcomes, we used a number of complimentary methods in this work, including the inverse variance weighted (IVW), the MR-Egger regression, the weighted median, the simple mode, and the weighted mode methods. The primary analytical method was the IVW approach.
When all chosen SNPs were valid IVs, the IVW technique would produce the most accurate findings for basic causal estimations. The Wald ratio estimations are weighted averaged using the IVW technique (25). Using the premise of Instrument Strength Independent of Direct Effect, the MR-Egger regression runs a weighted linear regression and generates a consistent causal estimate even if all of the genetic IVs are incorrect (InSIDE) (26). It has a poor level of accuracy, though, and is vulnerable to genetic variants. As the weighted median regression technique does not require the InSIDE hypothesis, it is immune to horizontal pleiotropic bias and provides a weighted median of the Wald ratio estimates (27). It has been established that the Weighted Median technique outperforms the MR-Egger regression in various ways, offering reduced type I error and more causal estimate power. By grouping the SNPs into subsets based on similarities in their causal effects, the causal effect of the subgroup with the most SNPs is calculated using the Weighted Mode approach (28). The simple mode approach is also less biased than other methods while being less precise since it can reduce bias (28).
To assess the potential for horizontal pleiotropy, we used the MR-Egger regression. The intercept term of MR-Egger regression represents the average pleiotropic impact of the IVs (26). To determine whether pleiotropy existed, the MR Pleiotropy REsidual Sum and Outlier (MR-PRESSO) test was also performed (29). Its tasks include identifying horizontal pleiotropy, eliminating outliers to account for horizontal pleiotropy, and determining whether there are any discernible variations in the causal effects between the periods before and after outlier removal. We used the MR-Egger regression and the IVW technique to identify heterogeneity, and Cochran’s Q was used to measure the heterogeneities. To prevent horizontal pleiotropy brought on by a single SNP, a leave-one-out analysis was carried out, which systematically drops one SNP at a time.
Multiple comparisons were taken into consideration using the Bonferroni method, and a p-value of less than 0.05 demonstrated significant support for causal links. With three sets of SNPs analyzed, this resulted in a adjusted significance threshold of 0.05/3 = 0.0167. Only p-values below this Bonferroni-corrected cut-off of 0.0167 were considered statistically significant. The packages “TwoSampleMR” (30) and “MRPRESSO” in R version 4.2.1 were used for every analysis.
We incorporated 30, 36, and 17 independent SNPs with significant p-value less than 5×10-8 as IV SNPs for VSRC, HC and IC and Supplementary Table S1 contains extensive information on the instrumental variables. Our TSMR analysis revealed that sepsis and COVID-19 do not appear to be significantly causally related. When setting COVID traits as the exposure, COVID-19 was no causally associated with sepsis, as shown in Figure 2. The results of IVW analyses demonstrated that VSRC (odds ratio (OR) = 1.000, 95% confidence interval (CI),0.956-1.046, P = 0.996) HC (OR = 0.976, 95% CI, 0.920-1.036, P = 0.430) and IC (OR = 0.923, 95% CI, 0.796-1.071, P = 0.291) were no significantly associated with the risk of sepsis. The MR-Egger, Weighted Median, Simple Mode, MR-PRESSO, and Weighted Mode approaches also produced reliable findings. There was no heterogeneity between the individual SNPs, according to the heterogeneity test. The results of the MR-Egger regression and MR-PRESSO global test indicated that it was unlikely that horizontal pleiotropy would skew the cause of sepsis in COVID-19 (Table 1). Leave-one-out analysis revealed that no one SNP was responsible for the causative estimates of COVID-19 and sepsis. In Supplementary Figure S1, the leave-one-out analysis plots were displayed.
When setting COVID traits as the outcome, 11 independent SNPs with significant p-value less than 5×10-7 were extracted as IV. Detailed information on these IVs is provided in Supplementary Table S2. Sepsis was not causally associated with COVID-19, as shown in Figure 3. There was no evidence of a connection between sepsis and the risk of COVID-19 based on the IVW approach (VSRC: OR=0.995, 95% CI: 0.844-1.173, P = 0.953; HC: OR=0.993, 95% CI: 0.859-1.147, P = 0.921; IC: OR=1.001, 95% CI: 0.959-1.045, P = 0.961). The MR-Egger, Weighted Median, Simple Mode, MR-PRESSO, and Weighted Mode approaches also produced consistent findings (Table 2 and Supplementary Figure S2).
This was the first research to use a variety of complementary MR techniques to examine the bidirectional causal relationship between sepsis and COVID-19. A genetically anticipated COVID-19 association with sepsis in people of European ancestry was not seen in our two-sample MR investigation. The reverse MR study also showed no proof that COVID-19 and genetic susceptibility to sepsis were connected.
There have been earlier epidemiological studies that found a connection between sepsis and COVID-19. According to a recent meta-analysis, a sizable majority of hospitalized patients have COVID-19-related sepsis based on Sepsis-3; 77.9% of adult patients in the ICU had viral sepsis (31). A prospectively recruited, multicentric study’s retrospective analysis also revealed that during the COVID-19 pandemic, the raw 30-day death rate for sepsis patients rose (32). Nevertheless, this cohort study has drawbacks, such as a limited patient cohort and recruitment bias, which might prevent the detection of important effects. As a result, drawing conclusions about the causes of COVID-19 and sepsis merely from past research is challenging.
Several reports have shown since the beginning of the pandemic that while COVID-19 has certain distinctive characteristics, many of its acute signs are comparable to sepsis brought on by other viruses (19, 33–35). The correlation between COVID-19 and sepsis in observational studies can be explained by a number of factors. As a result of a number of potential processes, including immunological dysregulation, respiratory failure that results in hypoxemia, and metabolic acidosis brought on by circulatory malfunction, research has shown that the virus itself probably produces a sepsis syndrome (36). The most likely explanation is that sepsis-like cytokine storms are linked to COVID-19 illness (33, 37). In sepsis, there are two phases that occur one after the other. The first phase is a hyper-inflammatory phase, and the second is an immunosuppressive phase (38). Several indicators, including C-reactive protein (CRP), procalcitonin, tumor necrosis factors (TNF), interleukin (IL) 1 and IL-6, are present throughout the hyperinflammatory phase. Pro-inflammatory cytokines TNF, IL-1, and IL-6 are generated as part of the body’s early response to injury or illness (39). The liver produces CRP in response to infection and is induced to do so by IL-6. The body produces procalcitonin, which is regarded as the most effective sign of severe systemic inflammation. A number of these indicators are recognized as sepsis biomarkers and can be utilized to help diagnose and treat sepsis patients (40). Similar to this, it has been shown that these markers are elevated in patients with severe COVID-19 (19, 41–43). This highlights that the exact mechanisms leading to hyperinflammation and organ damage in COVID-19 remain incompletely understood. More research is still needed to elucidate the complex immunopathology of severe COVID-19 illness.
Our study conclusions indicate clinicians should be cautious about relying solely on interventions targeting sepsis to improve COVID-19 outcomes. While managing secondary sepsis is still important, our findings underscore the need to better understand the distinct mechanisms leading to critical COVID-19 pneumonia apart from sepsis. Ultimately, our results point to the need for further investigation into the biological pathways underlying progression to acute respiratory distress syndrome and multiple organ failure in COVID-19.
The inference of causation between COVID-19 and sepsis in both directions was ensured by the bidirectional analysis, which is a strength of the current bidirectional MR study. Compared to previous MR studies that largely examined COVID-19 hospitalization or mortality, our study outcome of severe vs. mild COVID-19 phenotypes allows us to detect more nuanced effects on disease progression. We also conducted thorough sensitivity analyses to assess pleiotropy that were not performed uniformly across prior works. There are a few restrictions with this study, though. The research group with European ancestry was included in the MR analysis, to start. As a result, it is still unknown whether the findings can be considered representative of the entire population. Second, although it is difficult to assess the degree of sample overlap, individuals in the exposure and outcome research likely overlapped. Luckily, the powerful instruments used in this study (F statistic significantly higher than 10) should reduce any potential bias resulting from sample overlap (44). Third, Pleiotropic effects of genetic variants cannot be fully ruled out, although sensitivity analyses were conducted to assess this. Fourth, variants associated with VSRC showed some evidence of bias based on MR Egger and IVW heterogeneity test P-values <0.05. This suggests pleiotropy and linkage disequilibrium may impact these VSRC results. Though we attempted to minimize confounding through outlier removal and robust methods, some residual bias cannot be excluded. Further analyses in larger datasets and with additional quality control steps could provide more definitive results. Fifth, previous observational studies have suggested an association between sepsis and COVID-19 (45), whereas our Mendelian randomization analysis found no evidence for a causal relationship; synthesizing these complementary lines of evidence across the broader literature highlights the need for multiple approaches when assessing causality, and our MR findings alone cannot definitively rule out a causal effect. Finally, the sepsis GWAS may capture susceptibility to infections beyond just bacterial sepsis.
In conclusion, our findings do not support a causal relationship between COVID-19 and sepsis risk, nor do they suggest a causal link between sepsis and COVID-19. Verification of the study’s findings will require updated MR analysis based on more genetic instruments and larger scale GWAS summary data.
Publicly available datasets were analyzed in this study. This data can be found here: UK Biobank (http://www.nealelab.is/uk-biobank). COVID-19 Host Genetics Initiative Release 7 (HGI, https://www.covid19hg.org/).
YC and MZ conceived the presented idea. HL and YC performed the manuscript writing. YC and MZ was involved in acquisition and processing of data. HL was involved in interpretation of data. YC and HL have contributed equally to this work and share first authorship. All authors contributed to the article and approved the submitted version.
This research did not receive any specific grant from funding agencies in the public, commercial, or not-for-profit sectors.
We gratefully acknowledge all the studies and databases that made GWAS summary data available.
The authors declare that the research was conducted in the absence of any commercial or financial relationships that could be construed as a potential conflict of interest.
All claims expressed in this article are solely those of the authors and do not necessarily represent those of their affiliated organizations, or those of the publisher, the editors and the reviewers. Any product that may be evaluated in this article, or claim that may be made by its manufacturer, is not guaranteed or endorsed by the publisher.
The Supplementary Material for this article can be found online at: https://www.frontiersin.org/articles/10.3389/fimmu.2023.1183489/full#supplementary-material
1. Rai P, Kumar BK, Deekshit VK, Karunasagar I, Karunasagar I. Detection technologies and recent developments in the diagnosis of COVID-19 infection. Appl Microbiol Biotechnol (2021) 105(2):441–55. doi: 10.1007/s00253-020-11061-5
2. Rothe C, Schunk M, Sothmann P, Bretzel G, Froeschl G, Wallrauch C, et al. Transmission of 2019-nCoV infection from an asymptomatic contact in Germany. N Engl J Med (2020) 382(10):970–1. doi: 10.1056/NEJMc2001468
3. Bai Y, Yao L, Wei T, Tian F, Jin DY, Chen L, et al. Presumed asymptomatic carrier transmission of COVID-19. Jama (2020) 323(14):1406–7. doi: 10.1001/jama.2020.2565
4. Fan S, He P, Guan J, Song W, Zhi H, Wang L. No association between interleukin-18 levels and risk of cardiovascular disease: A Mendelian randomization study. Hereditas (2020) 157(1):12. doi: 10.1186/s41065-020-00121-5
5. Rapp JL, Lieberman-Cribbin W, Tuminello S, Taioli E. Male sex, severe obesity, older age, and chronic kidney disease are associated with COVID-19 severity and mortality in New York City. Chest (2021) 159(1):112–5. doi: 10.1016/j.chest.2020.08.2065
6. Zhang JJ, Cao YY, Tan G, Dong X, Wang BC, Lin J, et al. Clinical, radiological, and laboratory characteristics and risk factors for severity and mortality of 289 hospitalized COVID-19 patients. Allergy (2021) 76(2):533–50. doi: 10.1111/all.14496
7. Ebinger JE, Achamallah N, Ji H, Claggett BL, Sun N, Botting P, et al. Pre-existing traits associated with Covid-19 illness severity. PloS One (2020) 15(7):e0236240. doi: 10.1371/journal.pone.0236240
8. Gao F, Zheng KI, Wang XB, Sun QF, Pan KH, Wang TY, et al. Obesity is a risk factor for greater COVID-19 severity. Diabetes Care (2020) 43(7):e72–4. doi: 10.2337/dc20-0682
9. Dennis JM, Mateen BA, Sonabend R, Thomas NJ, Patel KA, Hattersley AT, et al. Type 2 diabetes and COVID-19-related mortality in the critical care setting: A national cohort study in England, March-July 2020. Diabetes Care (2021) 44(1):50–7. doi: 10.2337/dc20-1444
10. Clift AK, Coupland CAC, Keogh RH, Diaz-Ordaz K, Williamson E, Harrison EM, et al. Living risk prediction algorithm (QCOVID) for risk of hospital admission and mortality from coronavirus 19 in adults: national derivation and validation cohort study. Bmj (2020) 371:m3731. doi: 10.1136/bmj.m3731
11. Williamson EJ, Walker AJ, Bhaskaran K, Bacon S, Bates C, Morton CE, et al. Factors associated with COVID-19-related death using OpenSAFELY. Nature (2020) 584(7821):430–6. doi: 10.1038/s41586-020-2521-4
12. Li X, Xu S, Yu M, Wang K, Tao Y, Zhou Y, et al. Risk factors for severity and mortality in adult COVID-19 inpatients in Wuhan. J Allergy Clin Immunol (2020) 146(1):110–8. doi: 10.1016/j.jaci.2020.04.006
13. Lin GL, McGinley JP, Drysdale SB, Pollard AJ. Epidemiology and immune pathogenesis of viral sepsis. Front Immunol (2018) 9:2147. doi: 10.3389/fimmu.2018.02147
14. American college of chest physicians/society of critical care medicine consensus conference: definitions for sepsis and organ failure and guidelines for the use of innovative therapies in sepsis. Crit Care Med (1992) 20(6):864–74.
15. Ripa M, Galli L, Poli A, Oltolini C, Spagnuolo V, Mastrangelo A, et al. Secondary infections in patients hospitalized with COVID-19: incidence and predictive factors. Clin Microbiol Infect (2021) 27(3):451–7. doi: 10.1016/j.cmi.2020.10.021
16. Zhou F, Yu T, Du R, Fan G, Liu Y, Liu Z, et al. Clinical course and risk factors for mortality of adult inpatients with COVID-19 in Wuhan, China: a retrospective cohort study. Lancet (2020) 395(10229):1054–62. doi: 10.1016/S0140-6736(20)30566-3
17. Chen T, Wu D, Chen H, Yan W, Yang D, Chen G, et al. Clinical characteristics of 113 deceased patients with coronavirus disease 2019: retrospective study. Bmj (2020) 368:m1091. doi: 10.1136/bmj.m1091
18. Chao JY, Derespina KR, Herold BC, Goldman DL, Aldrich M, Weingarten J, et al. Clinical characteristics and outcomes of hospitalized and critically ill children and adolescents with coronavirus disease 2019 at a tertiary care medical center in New York City. J Pediatr (2020) 223:14–19.e2. doi: 10.1016/j.jpeds.2020.05.006
19. Li H, Liu L, Zhang D, Xu J, Dai H, Tang N, et al. SARS-CoV-2 and viral sepsis: observations and hypotheses. Lancet (2020) 395(10235):1517–20. doi: 10.1016/S0140-6736(20)30920-X
20. Smith GD, Ebrahim S. ‘Mendelian randomization’: can genetic epidemiology contribute to understanding environmental determinants of disease? Int J Epidemiol (2003) 32(1):1–22. doi: 10.1093/ije/dyg070
21. Dan YL, Wang P, Cheng Z, Wu Q, Wang XR, Wang DG, et al. Circulating adiponectin levels and systemic lupus erythematosus: a two-sample Mendelian randomization study. Rheumatol (Oxford) (2021) 60(2):940–6. doi: 10.1093/rheumatology/keaa506
22. Mohus RM, Flatby H, Liyanarachi KV, DeWan AT, Solligård E, Damås JK, et al. Iron status and the risk of sepsis and severe COVID-19: a two-sample Mendelian randomization study. Sci Rep (2022) 12(1):16157. doi: 10.1038/s41598-022-20679-6
23. Abecasis GRD, Altshuler A, Auton LD, Brooks RM, Durbin RA, Gibbs, et al. A map of human genome variation from population-scale sequencing Nature, (2010). 467(7319):1061–73.
24. Burgess S, Thompson SG. Avoiding bias from weak instruments in Mendelian randomization studies. Int J Epidemiol (2011) 40(3):755–64. doi: 10.1093/ije/dyr036
25. Burgess S, Butterworth A, Thompson SG. Mendelian randomization analysis with multiple genetic variants using summarized data. Genet Epidemiol (2013) 37(7):658–65. doi: 10.1002/gepi.21758
26. Bowden J, Davey Smith G, Burgess S. Mendelian randomization with invalid instruments: effect estimation and bias detection through Egger regression. Int J Epidemiol (2015) 44(2):512–25. doi: 10.1093/ije/dyv080
27. Bowden J, Davey Smith G, Haycock PC, Burgess S. Consistent estimation in mendelian randomization with some invalid instruments using a weighted median estimator. Genet Epidemiol (2016) 40(4):304–14. doi: 10.1002/gepi.21965
28. Hartwig FP, Davey Smith G, Bowden J. Robust inference in summary data Mendelian randomization via the zero modal pleiotropy assumption. Int J Epidemiol (2017) 46(6):1985–98. doi: 10.1093/ije/dyx102
29. Verbanck M, Chen CY, Neale B, Do R. Detection of widespread horizontal pleiotropy in causal relationships inferred from Mendelian randomization between complex traits and diseases. Nat Genet (2018) 50(5):693–8. doi: 10.1038/s41588-018-0099-7
30. Hemani G, Zheng J, Elsworth B, Wade KH, Haberland V, Baird D, et al. The MR-Base platform supports systematic causal inference across the human phenome. Elife (2018) 7:e34408. doi: 10.7554/eLife.34408
31. Karakike E, Giamarellos-Bourboulis EJ, Kyprianou M, Fleischmann-Struzek C, Pletz MW, Netea MG, et al. Coronavirus disease 2019 as cause of viral sepsis: A systematic review and meta-analysis. Crit Care Med (2021) 49(12):2042–57. doi: 10.1097/CCM.0000000000005195
32. Unterberg M, Rahmel T, Rump K, Wolf A, Haberl H, von Busch A, et al. The impact of the COVID-19 pandemic on non-COVID induced sepsis survival. BMC Anesthesiol (2022) 22(1):12. doi: 10.1186/s12871-021-01547-8
33. Patil M, Singh S, Henderson J, Krishnamurthy P. Mechanisms of COVID-19-induced cardiovascular disease: Is sepsis or exosome the missing link? J Cell Physiol (2021) 236(5):3366–82. doi: 10.1002/jcp.30109
34. Prescott HC, Girard TD. Recovery from severe COVID-19: leveraging the lessons of survival from sepsis. Jama (2020) 324(8):739–40. doi: 10.1001/jama.2020.14103
35. Lin HY. The severe COVID-19: A sepsis induced by viral infection? And its immunomodulatory therapy. Chin J Traumatol (2020) 23(4):190–5. doi: 10.1016/j.cjtee.2020.06.002
36. Wang F, Suo XG, Wang C, Wang JN, He XY, Wang FC, et al. Highly pathogenic coronaviruses and the kidney. Biomed Pharmacother (2022) 156:113807. doi: 10.1016/j.biopha.2022.113807
37. Kellum JA, Nadim MK, Forni LG. Sepsis-associated acute kidney injury: is COVID-19 different? Kidney Int (2020) 98(6):1370–2. doi: 10.1016/j.kint.2020.08.009
38. Rello J, Valenzuela-Sánchez F, Ruiz-Rodriguez M, Moyano S. Sepsis: A review of advances in management. Adv Ther (2017) 34(11):2393–411. doi: 10.1007/s12325-017-0622-8
39. Zeng G, Chen D, Zhou R, Zhao X, Ye C, Tao H, et al. Combination of C-reactive protein, procalcitonin, IL-6, IL-8, and IL-10 for early diagnosis of hyperinflammatory state and organ dysfunction in pediatric sepsis. J Clin Lab Anal (2022) 36(7):e24505. doi: 10.1002/jcla.24505
40. Riedel S, Melendez JH, An AT, Rosenbaum JE, Zenilman JM. Procalcitonin as a marker for the detection of bacteremia and sepsis in the emergency department. Am J Clin Pathol (2011) 135(2):182–9. doi: 10.1309/AJCP1MFYINQLECV2
41. Huang C, Wang Y, Li X, Ren L, Zhao J, Hu Y, et al. Clinical features of patients infected with 2019 novel coronavirus in Wuhan, China. Lancet (2020) 395(10223):497–506. doi: 10.1016/S0140-6736(20)30183-5
42. Liu J, Li S, Liu J, Liang B, Wang X, Wang H, et al. Longitudinal characteristics of lymphocyte responses and cytokine profiles in the peripheral blood of SARS-CoV-2 infected patients. EBioMedicine (2020) 55:102763. doi: 10.1016/j.ebiom.2020.102763
43. Satış H, Özger HS, Aysert Yıldız P, Hızel K, Gulbahar Ö, Erbaş G, et al. Prognostic value of interleukin-18 and its association with other inflammatory markers and disease severity in COVID-19. Cytokine (2021) 137:155302. doi: 10.1016/j.cyto.2020.155302
44. Pierce BL, Burgess S. Efficient design for Mendelian randomization studies: subsample and 2-sample instrumental variable estimators. Am J Epidemiol (2013) 178(7):1177–84. doi: 10.1093/aje/kwt084
Keywords: COVID-19, sepsis, Mendelian randomization, immune response, genetics
Citation: Lu H, Cao Y and Zhong M (2023) No causal association between COVID-19 and sepsis: a bidirectional two-sample Mendelian randomization study. Front. Immunol. 14:1183489. doi: 10.3389/fimmu.2023.1183489
Received: 10 March 2023; Accepted: 18 September 2023;
Published: 09 October 2023.
Edited by:
Gabriela Angélica Martínez-Nava, National Institute of Rehabilitation Luis Guillermo Ibarra Ibarra, MexicoReviewed by:
Laura Edith Martínez-Gómez, National Institute of Rehabilitation Luis Guillermo Ibarra Ibarra, MexicoCopyright © 2023 Lu, Cao and Zhong. This is an open-access article distributed under the terms of the Creative Commons Attribution License (CC BY). The use, distribution or reproduction in other forums is permitted, provided the original author(s) and the copyright owner(s) are credited and that the original publication in this journal is cited, in accordance with accepted academic practice. No use, distribution or reproduction is permitted which does not comply with these terms.
*Correspondence: Ming Zhong, emhvbmdtaW5nX29yYWxAYWxpeXVuLmNvbQ==
†These authors have contributed equally to this work and share first authorship
Disclaimer: All claims expressed in this article are solely those of the authors and do not necessarily represent those of their affiliated organizations, or those of the publisher, the editors and the reviewers. Any product that may be evaluated in this article or claim that may be made by its manufacturer is not guaranteed or endorsed by the publisher.
Research integrity at Frontiers
Learn more about the work of our research integrity team to safeguard the quality of each article we publish.