- 1College of Pharmacy, Binzhou Medical University, Yantai, China
- 2College of Life Science, Yantai University, Yantai, China
Background: Systemic lupus erythematosus (SLE) is an autoimmune disease characterized by loss of tolerance to self-antigen, autoantibody production, and abnormal immune response. Cuproptosis is a recently reported cell death form correlated with the initiation and development of multiple diseases. This study intended to probe cuproptosis-related molecular clusters in SLE and constructed a predictive model.
Methods: We analyzed the expression profile and immune features of cuproptosis-related genes (CRGs) in SLE based on GSE61635 and GSE50772 datasets and identified core module genes associated with SLE occurrence using the weighted correlation network analysis (WGCNA). We selected the optimal machine-learning model by comparing the random forest (RF) model, support vector machine (SVM) model, generalized linear model (GLM), and the extreme gradient boosting (XGB) model. The predictive performance of the model was validated by nomogram, calibration curve, decision curve analysis (DCA), and external dataset GSE72326. Subsequently, a CeRNA network based on 5 core diagnostic markers was established. Drugs targeting core diagnostic markers were acquired using the CTD database, and Autodock vina software was employed to perform molecular docking.
Results: Blue module genes identified using WGCNA were highly related to SLE initiation. Among the four machine-learning models, the SVM model presented the best discriminative performance with relatively low residual and root-mean-square error (RMSE) and high area under the curve (AUC = 0.998). An SVM model was constructed based on 5 genes and performed favorably in the GSE72326 dataset for validation (AUC = 0.943). The nomogram, calibration curve, and DCA validated the predictive accuracy of the model for SLE as well. The CeRNA regulatory network includes 166 nodes (5 core diagnostic markers, 61 miRNAs, and 100 lncRNAs) and 175 lines. Drug detection showed that D00156 (Benzo (a) pyrene), D016604 (Aflatoxin B1), D014212 (Tretinoin), and D009532 (Nickel) could simultaneously act on the 5 core diagnostic markers.
Conclusion: We revealed the correlation between CRGs and immune cell infiltration in SLE patients. The SVM model using 5 genes was selected as the optimal machine learning model to accurately evaluate SLE patients. A CeRNA network based on 5 core diagnostic markers was constructed. Drugs targeting core diagnostic markers were retrieved with molecular docking performed.
1 Introduction
Systemic lupus erythematosus (SLE) is an autoimmune disease (1) (AID) featuring loss of tolerance to self-antigen, autoantibody production, and abnormal immune response. It affects multiple organs, such as skin, joints, kidneys, lungs, and hearts (2), severely interrupting work, normal routine, and physical and mental health. The pathogenesis of SLE is not clear. Genetic and environmental factors and viral infection are considered possible pathogenic factors (3–5). So far, specific drugs for SLE are very scarce, and SLE patients are still treated with traditional anti-inflammatory drugs, immunoregulatory drugs, and corticosteroids, often accompanied by adverse events (AE). Therefore, investigating molecular characteristics and mechanisms of SLE has substantial implications for providing new strategies for SLE diagnosis and treatment.
Copper serves as a cofactor in many enzymes and has important physiological functions in vital activities (6). Normal cells have a quite low copper concentration, and they prevent the accumulation of free intracellular copper ions mainly by active transport mechanisms, thereby sustaining copper homeostasis (7, 8). Copper imbalance leads to oxidative stress (9), aberrant autophagy (10), etc., thereby inducing various copper/copper ion-associated diseases. Cuproptosis is a copper-dependent programmed cell death form, and its mechanism is different from apoptosis, pyroptosis, necrosis, and autophagy. In cuproptosis, copper directly binds with lipoylated proteins in the tricarboxylic acid (TCA) cycle, leading to lipoylated protein aggregation and following iron-sulfur cluster loss, inducing proteotoxic stress and eventually cell death (11). Research showed that ferroptosis in neutrophils leads to the occurrence of SLE, and the mechanism is by promoting cAMP response element modulator CREM binding with glutathione peroxidase 4 (GPX4) promoter to downregulate GPX4 expression (12). The significance of copper homeostasis in immune infiltration has been reported in relevant studies recently. It was reported that copper chelation in macrophages can eliminate lysyl oxidase-like 4-mediated programmed death-ligand 1 presentation, thereby suppressing cell immune escape (13). However, currently, cuproptosis’s role in the initiation and development of SLE is still not clear. Hence, elucidating cuproptosis’s role in SLE has considerable implications.
Machine learning is being widely applied in the medical field, particularly in disease diagnosis, prediction, and treatment. With its high efficiency in thousands of types of diseases, machine learning can be divided into three main types: supervised learning (14), semi-supervised learning (15), and unsupervised learning (16) each being used to train artificial intelligence models with different types of data in different circumstances. Among these, the prediction of disease biomarkers is one important application of machine learning in disease prediction, which can provide doctors with more accurate diagnostics and treatment decisions, ultimately increasing the cure rate and prognosis of the disease. The future of medicine will place increasing emphasis on machine learning. For instance, it could be used to quickly and accurately detect lung cancer in chest CT scans, improving patient survival rates (17). Machine learning can also predict the risk of heart disease in electrocardiograms (18), detect early retinal lesions using semi-supervised learning techniques (19), and assist in diagnosing Alzheimer’s disease (20). By incorporating machine learning, we can gain better insight into the underlying mechanisms of diseases and provide potential targets for future treatments. However, machine learning is just a tool, and we still need to combine it with practical disease research to effectively address specific problems.
In this study, we analyzed differentially expressed cuproptosis-related genes (CRGs) between healthy people and SLE patients using the Gene Expression Omnibus (GEO) database and conducted a bioinformatics analysis of immune characteristics. Based on the CRG expression profile, we assigned SLE patients to two cuproptosis-related clusters and further compared the CRGs of the two clusters. Subsequently, we identified key modules associated with SLE initiation using the weighted gene co-expression network analysis (WGCNA) algorithm. Furthermore, we constructed a predictive model that can reveal the prognoses of patients with different molecular clusters by comparing multiple machine-learning methods. Nomogram, calibration curve, decision curve analysis (DCA), and an external dataset were adopted to verify the performance of the predictive model. Additionally, we established a competing endogenous RNA (CeRNA) regulation network, and selected drugs that act on key biomarkers using the CTD database, which were used as candidate drugs for SLE.
2 Materials and methods
2.1 Data acquisition and preprocessing
The flow chart of this study is shown in Figure 1. The GSE61635 (21), GSE50772 (22), and GSE72326 (23) datasets were retrieved from the GEO database (Table 1), and CRGs (11) were collected from published studies. GSE61635 and GSE50772 were merged as one dataset (GSEM) serving as the training cohort since the two datasets were obtained from the same platform, and the GSE72326 dataset was used as the validation cohort. The raw data were normalized and annotated with background subtracted, and batch effects from the merged dataset were removed using the “SVA” package.
2.2 WGCNA
WGCNA was performed using the R “WGCN”package (24). The optimal value of the weighting parameter in the adjacent function was obtained using the pickSoftThreshold function and served as soft-thresholding power for following network construction (25). Subsequently, weighted adjacency matrices were established, and gene modules were created by hierarchical clustering based on a 1-TOM dissimilarity matrix (26). Each module was assigned a unique color identifier, and the module eigengene represents the expression profile of the entire module. Module–disease state relationships represent module significance (MS), and gene significance describes a gene’s correlation with a phenotype.
2.3 Predictive model construction based on multiple machine-learning methods
Machine-learning predictive models include the support vector machine (SVM) model, random forest (RF) model, generalized linear model (GLM), and extreme gradient boosting (XGB) model. The SVM algorithm seeks the separating hyperplane that yields the maximal margin to discriminate positive instances from negative instances (27). The RF is an ensemble learning method yielding several independent decision trees to predict classification or regression (28). The GLM is an extension of the multiple linear regression model, and it can flexibly assess the relationship between normally-distributed dependent characteristics and continuous or categorical independent characteristics (29). XGB is a collection of gradient-boosted trees that can carefully compare and analyze complexity and classification error (30). The four machine learning models were explained using the “DALEX” package, and residual distribution and feature importance among the models were visualized. The AUC of the ROC curve was visualized using the “pROC” R package. Eventually, we confirmed the optimal machine learning model and selected the top 5 factors as SLE-related key predictors.
2.4 Nomogram construction and validation
A nomogram was established to evaluate SLE occurrence in clusters using the “RMS” R package. Each predictor contributes to a score, and the “total score” represents the sum of the score of the above predictors. The calibration curve and DCA were adopted to evaluate the predictive performance of the nomogram.
2.5 Immune cell infiltration analysis
The CIBERSORT algorithm (https://cibersort.stanford.edu/) was performed to estimate the relative abundance of 22 kinds of immune cells for each sample using the LM22 signature matrix and gene expression data. CIBERSORT uses Monte Carlo sampling to obtain a p‐value for the deconvolution of each sample. Only samples with P < 0.05 were considered to have accurate immune cell fractions, and the sum of the 22 immune cell compositions in each sample was 1 (31).
2.6 lncRNA-miRNA-mRNA CeRNA network construction
miRNA-miRNA interactions were predicted using TargetScan (http://www.targetscan.org), miRDB (http://www.mirdb.org/), and miRanda (http://www.microrna.org/) databases, and miRNA-lncRNAs were predicted using the SpongeScan database (http://spongescan.rc.ufl.edu/). Based on lncRNA-miRNA-mRNA interactions, a ceRNA network was constructed using Cytoscape software (3.8.2).
2.7 Identification of candidate small-molecule drugs
Drugs corresponding to those diagnostic biomarkers were retrieved using the CTD database (http://ctdbase.org/) to confirm potential SLE drugs. Drug-gene network was constructed and visualized. Molecular docking was performed between selected drugs and sites of key biomarkers using Autodock vina software V1.1.2, and the results were visualized using Pymol V3.9.2.
3 Results
3.1 CRG expression and immune infiltration analysis in SLE patients
We evaluated the expression profile of 17 CRGs in SLE and normal Control samples using the merged dataset GSEM to investigate CRGs’ biological functions in SLE patients. SLE samples presented higher expression of NFE2L2, NLRP3, ATP7A, MTF1, and CDKN2A genes and lower expression of LIAS, LIPT1, DLD, DLAT, PDHA1, PDHB, GLS, DBT, and DLST genes versus the Control group (Figures 2A, B). Subsequently, we conducted correlation analysis for differentially expressed CRGs (Figure 2C) to probe CRGs’ role in SLE development. Notably, some CRGs like PDHB and PDHA1 exhibited synergistic effects (R = 0.62). Meanwhile, NFE2L2 and DLST displayed significant antagonistic effects (R = 0.53). Moreover, we performed an immune infiltration analysis to illustrate the difference in immune systems between the normal Control group and SLE patients. The CIBERSORT algorithm revealed a significant distinction in the proportions of 22 kinds of immune cells between the Control and SLE groups such as neutrophils, plasma cells, CD8+ T cells, naive CD8+ lymphocytes, M1 macrophages, activated dendritic cells (DC), resting mast cells, etc. (Figures 2D, E), suggesting that immune system alteration might be the primary cause of SLE occurrence. Additionally, correlation analysis demonstrated that neutrophils and CD8+ T cells were correlated with cuproptosis (Figure 2F).
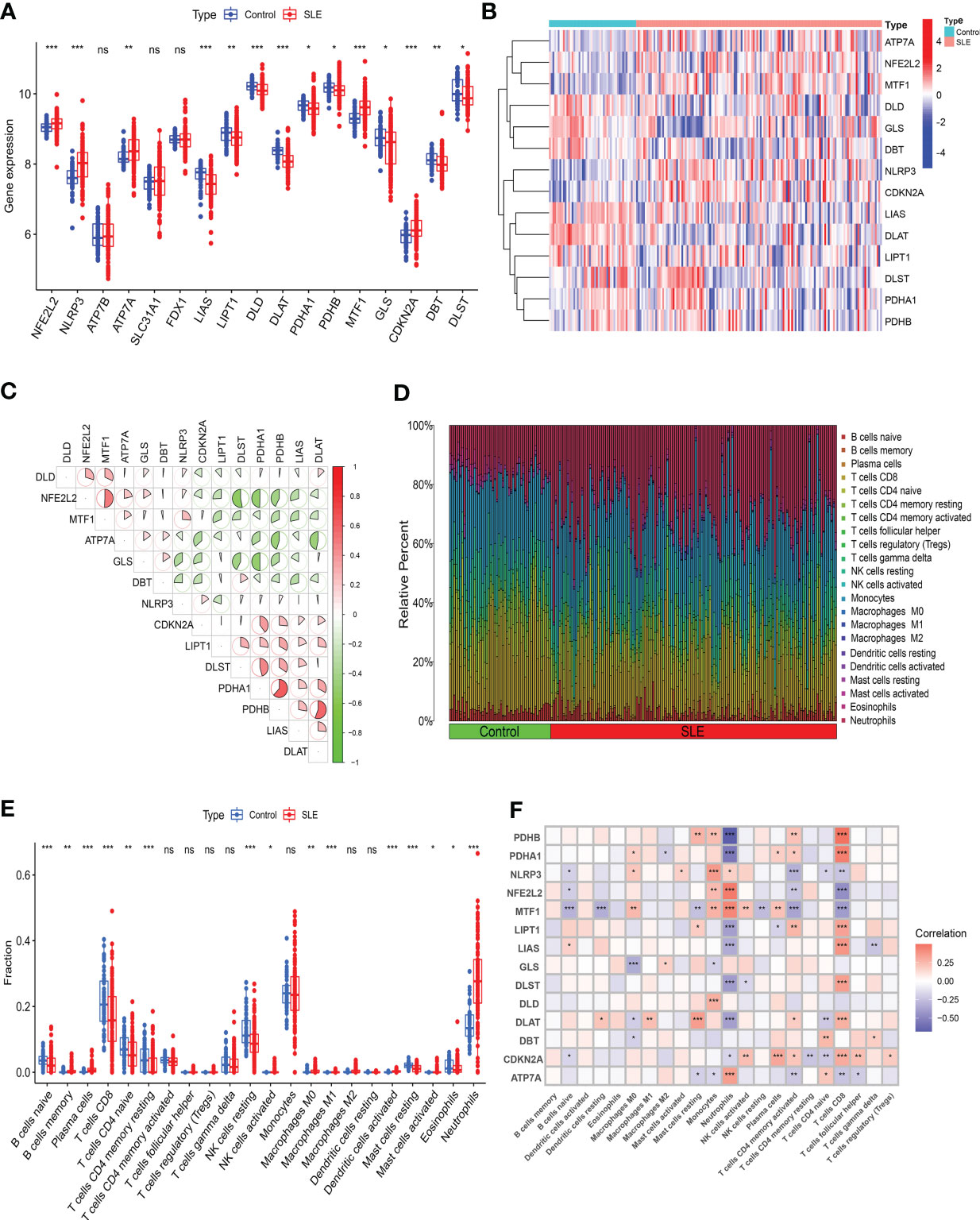
Figure 2 Identification of the expression of CRGs and Immune infiltration analysis in SLE. (A) Boxplots showed the expression of 14 CRGs between SLE and Control. (B) The expression patterns of 14 CRGs were presented in the heatmap. (C) The correlation of CRGs. (D) The relative abundance of immune cells between SLE and Control. (E) Boxplots showed the difference of Immune infiltration in SLE and Control. (F) The correlation analysis between 14 CRGs and immune cells. (* p≤ 0.05, ** p≤ 0.01, *** p≤ 0.001, ns, no significance).
3.2 Identification of SLE cuproptosis cluster
To understand the expression patterns of CRGs in SLE, we conducted a consensus clustering analysis with the expression of the 14 CRGs, and the consensus index fluctuated within a minimal range of 0.2-0.6 (Figures 3A, B, Figure S1). When k = 2-9, the area under the CDF curve is presented as the difference between two CDF curves (k and k-1) (Figure 3C). Furthermore, only when k = 2, the consistency score of all subtypes was> 0.9 (Figure 3D). Combining the consensus matrix heatmap, we divided 141 patients into two clusters, including Cluster 1 (n = 98) and Cluster 2 (n = 43) (Figure 3E). Patients were clustered by t-distributed stochastic neighbor embedding (t-SNE), showing a significant difference between the two clusters (Figure 3F). We generally evaluated the expression difference in 14 CRGs between Clusters 1 and 2 to investigate the molecular characteristics of clusters. Distinct CRG expression patterns were observed between two Clusters. Cluster 1 showed high expression of FDX1, DLD, DLAT, PDHA1, PDHB, and GLS, while Cluster 2 showed enhanced expression of LIPT1, MTF1, CDKN2A, and SLC31A1 (Figure 3G). Next, our analysis focused on the immune cell infiltration differences between the two groups, revealing distinct levels of four immune cells. T cells CD8 and M0 macrophages were found to be higher in cluster 2, while neutrophils and M2 macrophages were lower (Figures S2, 3). Further analysis using GSVA revealed functional differences in cluster-specific DEGs between the two clusters. Cluster 1 showed up-regulation in Glycine serine and threonine metabolism, Cysteine and methionine metabolism, and Pathogenic Escherichia coli infection signal activity, whereas cluster 2 showed enhancement in inflammation, metabolism, immune response, and TGF-β signal activity (Figure S4). Additionally, the functional enrichment results revealed that cluster 1 was significantly associated with positive regulation of ATPase complex of proton transport, mitosis, vitamin D metabolism, and smooth muscle cell apoptosis. In contrast, H3K9me2 demethylase activity, aminophospholipid transferase activity, regulation of RNA binding, and negative regulation of cytoplasmic translation were enriched in cluster 2 (Figure S5).
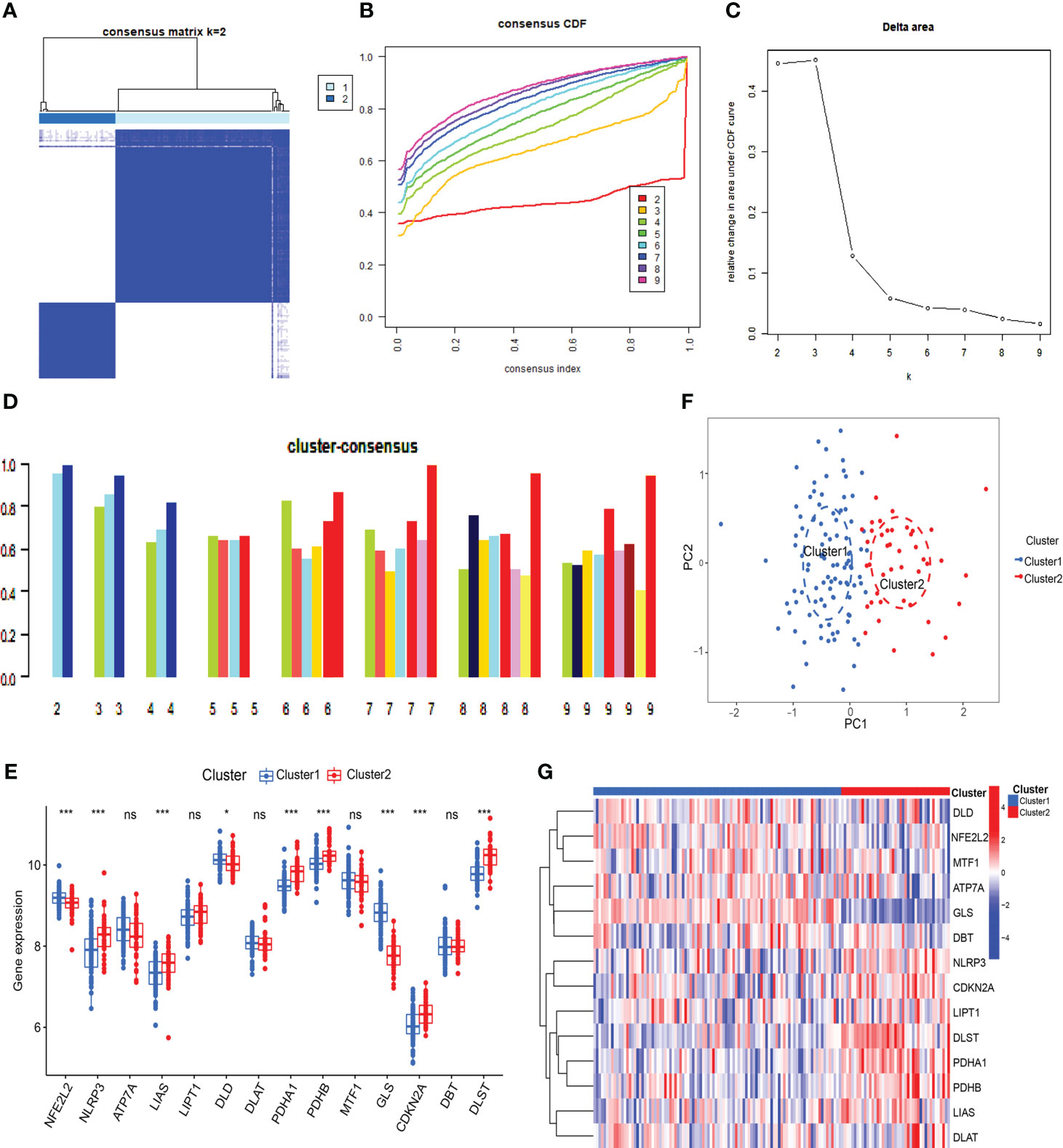
Figure 3 Identification of cuproptosis-related molecular clusters in SLE. (A) Consensus clustering matrix when k=2. (B, C) Representative cumulative distribution function (CDF) curves (B), CDF delta area curves (C), the score of consensus clustering (D). (E) T-SNE visualizes the distribution of two subtypes. (F) Boxplots showed the expression of 14 CRGs between two cuproptosis clusters. (G) The expression patterns of 14 CRGs were presented in the heatmap. (* p ≤ 0.05,*** p ≤ 0.001, ns, no significance).
3.3 Weighted co-expression network construction and core module selection
Co-expression network and module were constructed for the Control and SLE groups using the WGCNA algorithm to identify SLE-related core gene modules. We calculated the variance of gene expression in the GSEM dataset and selected the top 25% of genes with the highest variance for further analysis. When Soft was set to 7, scale-free R2 = 0.9, and co-expression modules were identified (Figure 4A). Altogether 9 co-expression modules with different colors were obtained using the dynamic cut-tree algorithm, and TOM Heatmap was generated (Figures 4B–D). Subsequently, genes were consecutively applied in 9 color modules with module-clinical characteristics (Control and SLE) co-expression similarity and adjacency analyzed. The blue module presented the strongest correlation with SLE, including 263 genes (Figure 4E, Table S1). We also observed the correlation between modules and module-related genes (Figure 4F, Table S2).
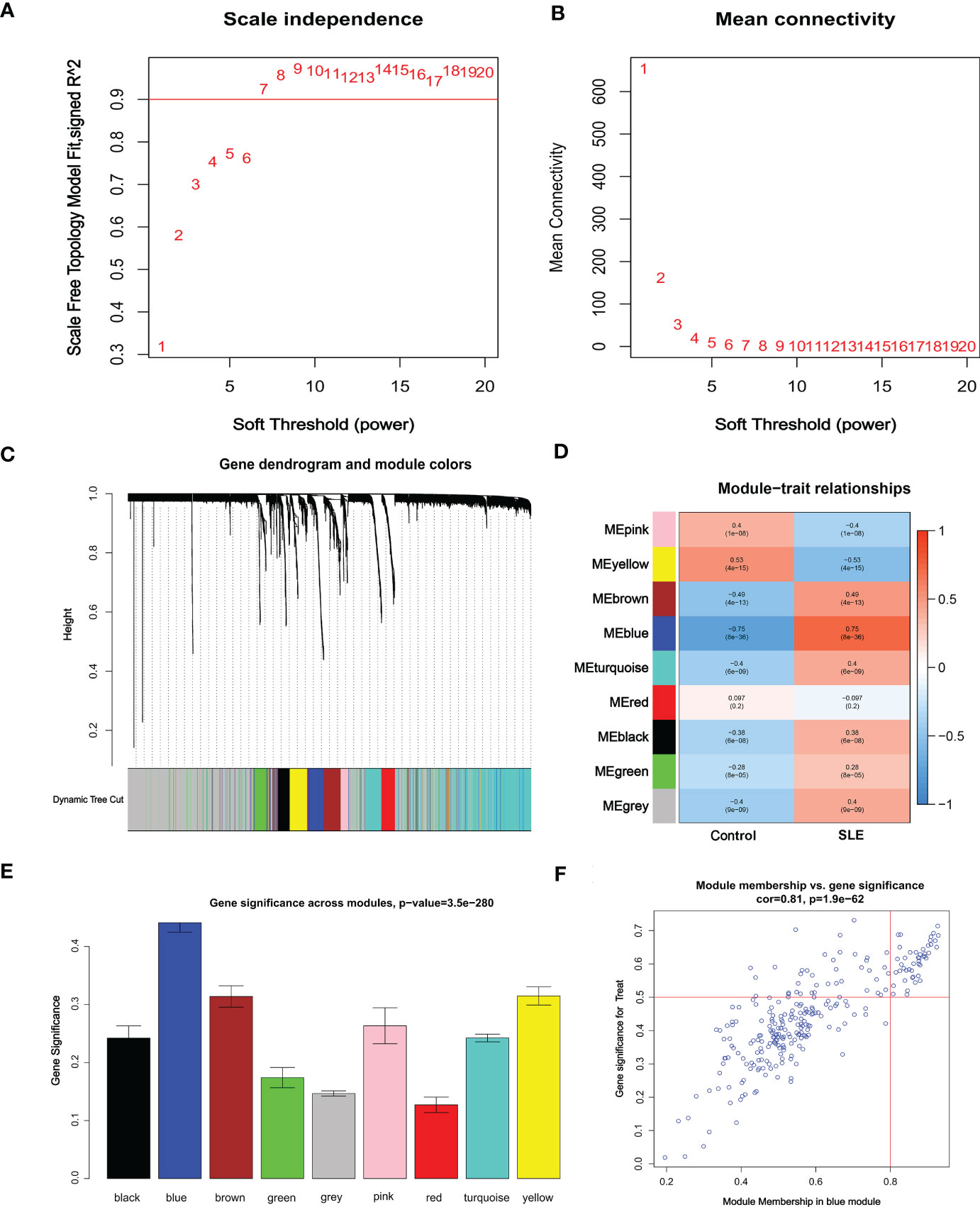
Figure 4 Identification of weighted gene co-expression network modules associated with SLE in GSEM. (A, B) Soft threshold selection. (C) Dynamic shearing tree merging similar module genes. (D) Correlation analysis between module eigengenes and clinical status. (E) The correlation between genes and traits between modules. (F) The correlation between module membership and genetic importance. cor represents the correlation between GS and MM.
3.4 Machine learning model construction and evaluation
To further identify critical markers with high diagnostic value, we established 4 machine-learning models based on the blue core module expression profile, including SVM, RF, GLM, and XGB models. The 4 models were explained using the “DALEX” package, and residuals from each model in the training cohort were plotted. The SVM model had a relatively low residual (Figures 5A, B). The top 10 important variables of each model were obtained according to root-mean-square error (RMSE) (Figure 5C). Moreover, ROC curves of the 5-fold cross-validation were plotted to appraise the diagnostic performance of the 4 machine learning algorithms in the training cohort. SVM model had the highest AUC (SVM, AUC = 0.998; RF, AUC = 0.976; XGB, AUC = 0.960; GLM, AUC = 0.943, Figure 5D). Combining those results, the SVM model had the best performance in distinguishing patients from different clusters. The top 5 variables (IFIT3, PLSCR1, CCR1, IL1RN, and ETV7) were selected from the SVM model as critical predictive markers for the following analysis.
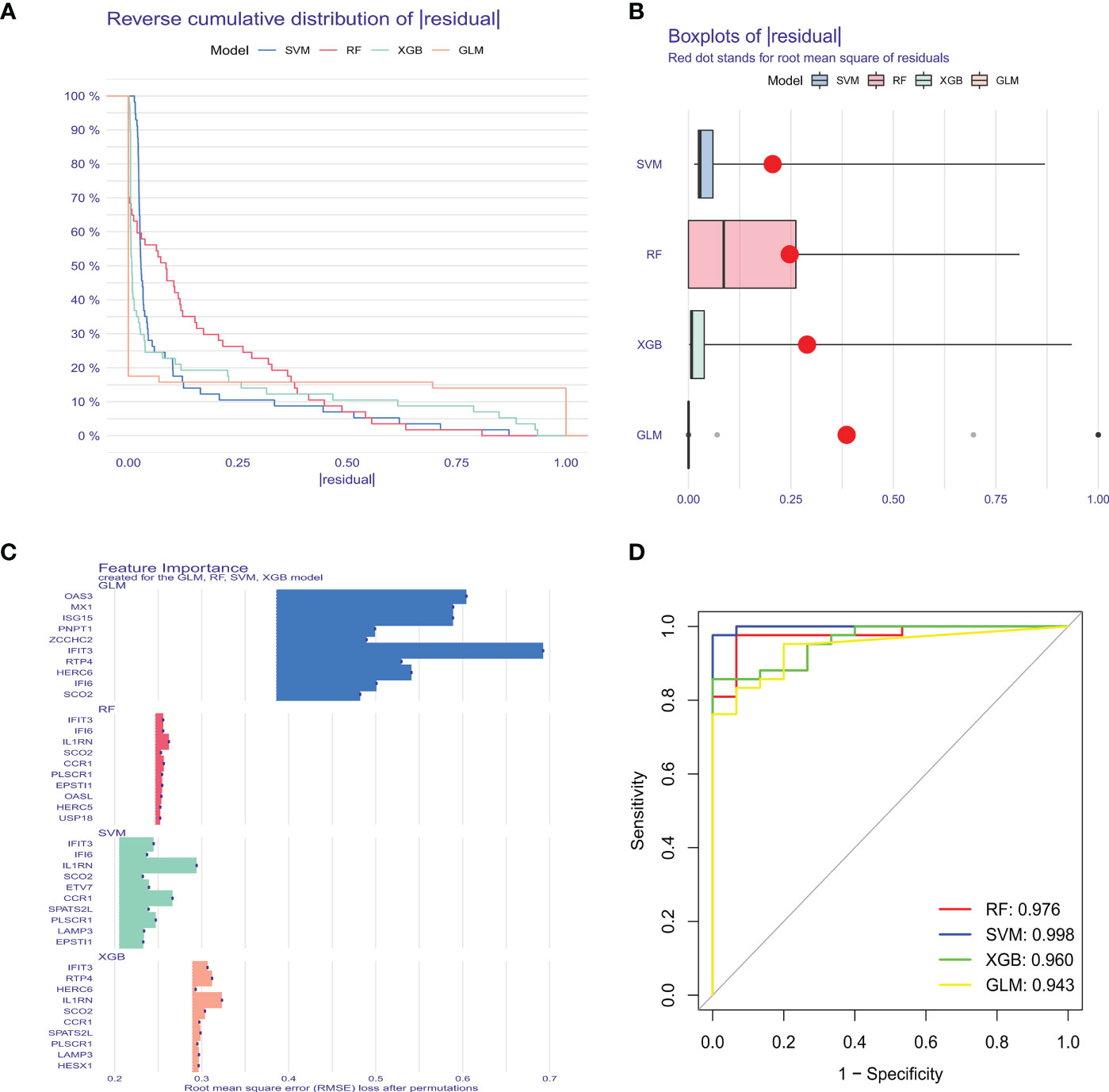
Figure 5 Construction and evaluation of SVM, RF, GLM, and XGB machine models. (A) Cumulative residual distribution of each machine learning model. (B) Boxplots showed the residuals of each machine learning model. Red dot represented the root mean square of residuals (RMSE). (C) The important features in SVM, RF, GLM, and XGB machine models. (D) ROC analysis of four machine learning models based on 5-fold cross-validation in the testing cohort.
A nomogram was constructed to assess the predictive efficiency of the SVM model using 141 SLE cases to predict the risk of cuproptosis aggregation (Figure 6A). The predictive efficiency of the nomogram was assessed using the calibration curve and DCA. According to the calibration curve, in the SLE cluster, the error between the actual risk and the predicted risk was very small (Figure 6B). DCA revealed that the nomogram had high accuracy that can provide evidence for clinical decisions (Figure 6C). Subsequently, we validated the predictive capability of the 5 core markers using the validation cohort GSE72326. The ROC curve revealed that the predictive model with 5 core markers had a favorable performance with an AUC of 0.943 (Figure 6D), suggesting that our diagnostic model can effectively discriminate SLE patients from normal cases. Meanwhile, the diagnostic value of single markers was verified as well, and IL1RN had the highest AUC (AUC = 0.918, Figure 6E). The expression of the 5 core diagnostic markers was all upregulated in SLE patients (Figures 6F–J).
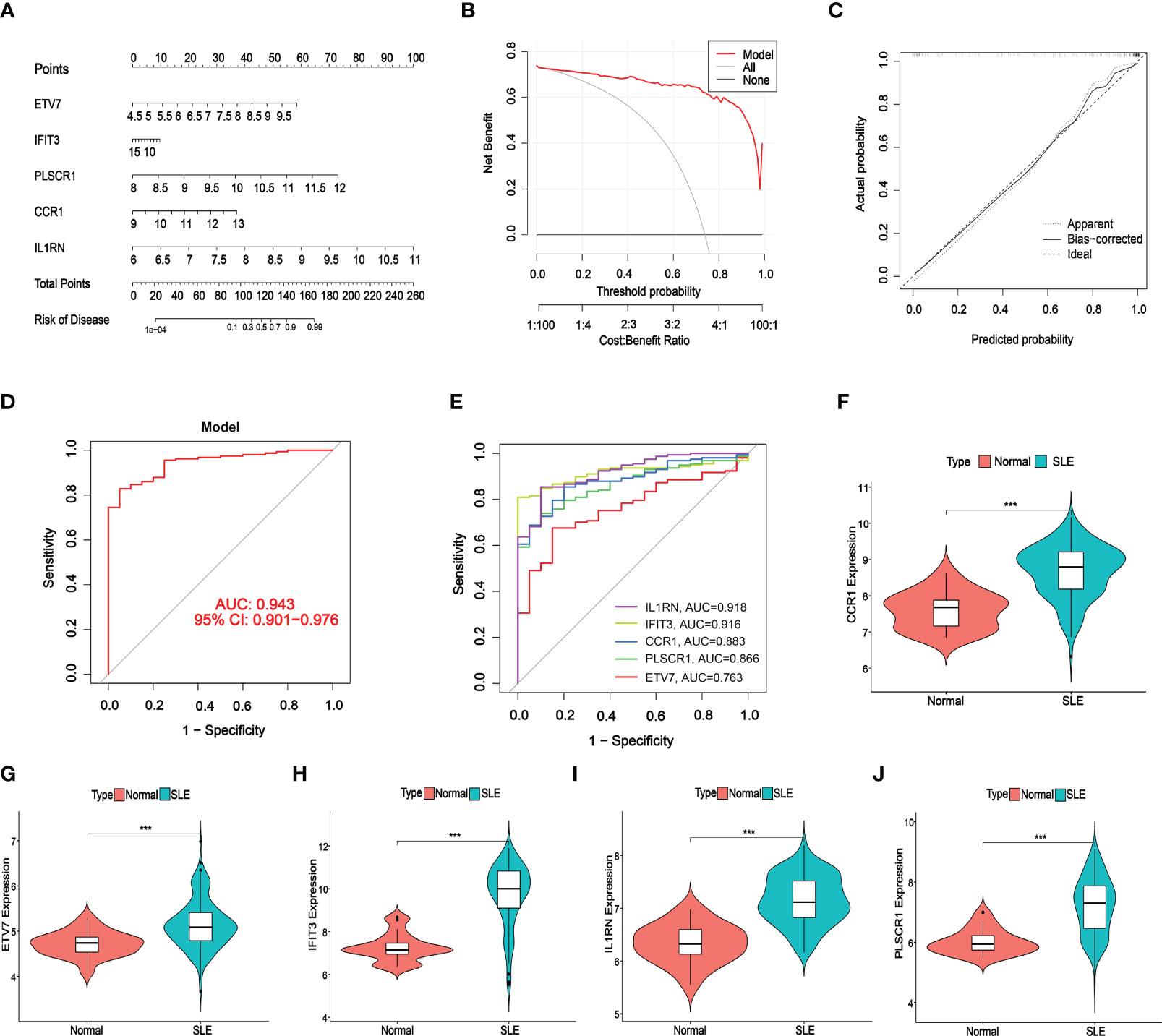
Figure 6 Validation of the 5-gene-based SVW model. (A) Construction of a nomogram for predicting the risk of SLE clusters based on the 5-gene-based SVW model. (B, C) Construction of calibration curve (B) and DCA (C) for assessing the predictive efficiency of the nomogram model. (D, E) ROC analysis of the 5-gene-based SVW model based on 5-fold cross-validation in GSE72326. (F-J) The expression levels of 5-genes were verified with validated dataset GSE72326. (*** p ≤ 0.001).
3.5 CeRNA network establishment of core diagnostic markers
A CeRNA network was constructed using miRanda, targetScan, miRDB, and SpongeScan databases with 5 core diagnostic markers. The CeRNA network contains 166 nodes (5 core diagnostic markers, 61 miRNAs, and 100 lncRNAs) and 175 lines (Figure 7). Eventually, 47 lncRNAs can competitively bind with IL1RN regulated by hsa-miR-650, hsa-miR-515-5p, hsa-miR-377-3p, hsa-miR-185-3p, and hsa-miR-1205, among which lncRNA SNHG14 can simultaneously target hsa-miR-515-5p and hsa-miR-185-3p. 23 lncRNA can target IFIT3 regulated by hsa-miR-876-3p, hsa-miR-127-5p, hsa-miR-34a-3p, hsa-miR-143-3p, hsa-miR-1207-5p, and hsa-miR-876-5p. Additionally, 19 lncRNA can regulate CCR1 expression by competitively binding with hsa-miR-149-3p. In the ceRNA network of PLSCR1, LINC00662 can bind with hsa-miR-28-3p and hsa-miR-708-3p to regulate PLSCR1. 8 lncRNAs competing with hsa-miR-342-5p to regulate ETV7 expression.
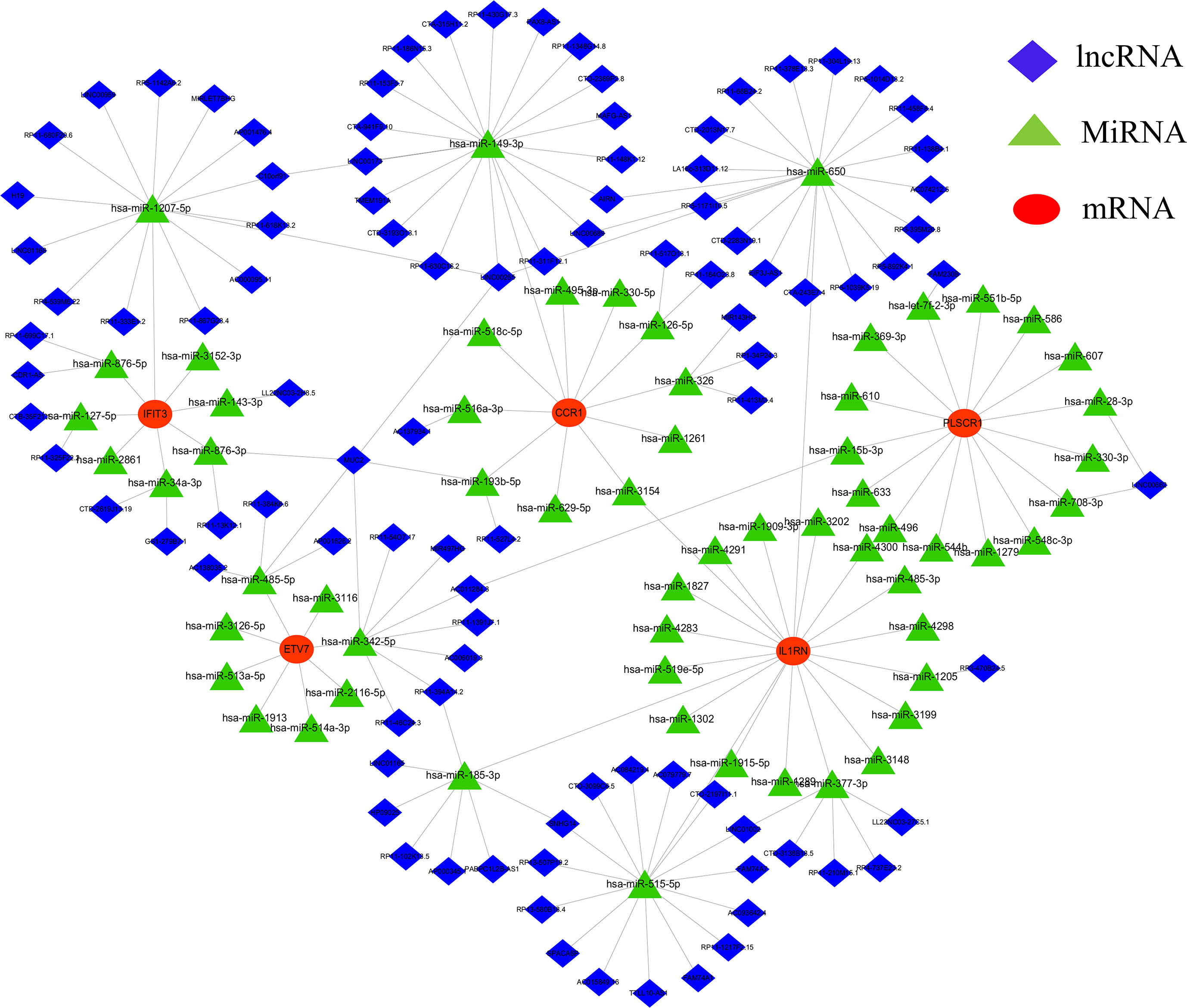
Figure 7 lncRNA-miRNA-mRNA regulatory network. The red represent the mRNAs, the green represent the miRNAs and the blue represent the lncRNAs.
3.6 Prediction of targeted drugs for core diagnostic markers
We further predicted drugs of the 5 core diagnostic markers using the CTD database, extracted drug-marker interactions, and constructed a drug-marker network containing 226 knots and 319 edges, in which 5 core diagnostic markers and 221 drugs were included. The results were visualized using the Cytoscape software (Figure 8A, Table S3). Drug detection showed that D00156 (Benzo (a) pyrene), D016604 (Aflatoxin B1), D014212 (Tretinoin), and D009532 (Nickel) could simultaneously act on the 5 core diagnostic markers. And a molecular docking was performed between drugs and predicted molecular targets (Figures 8B–E, Figure S7, Table S4).
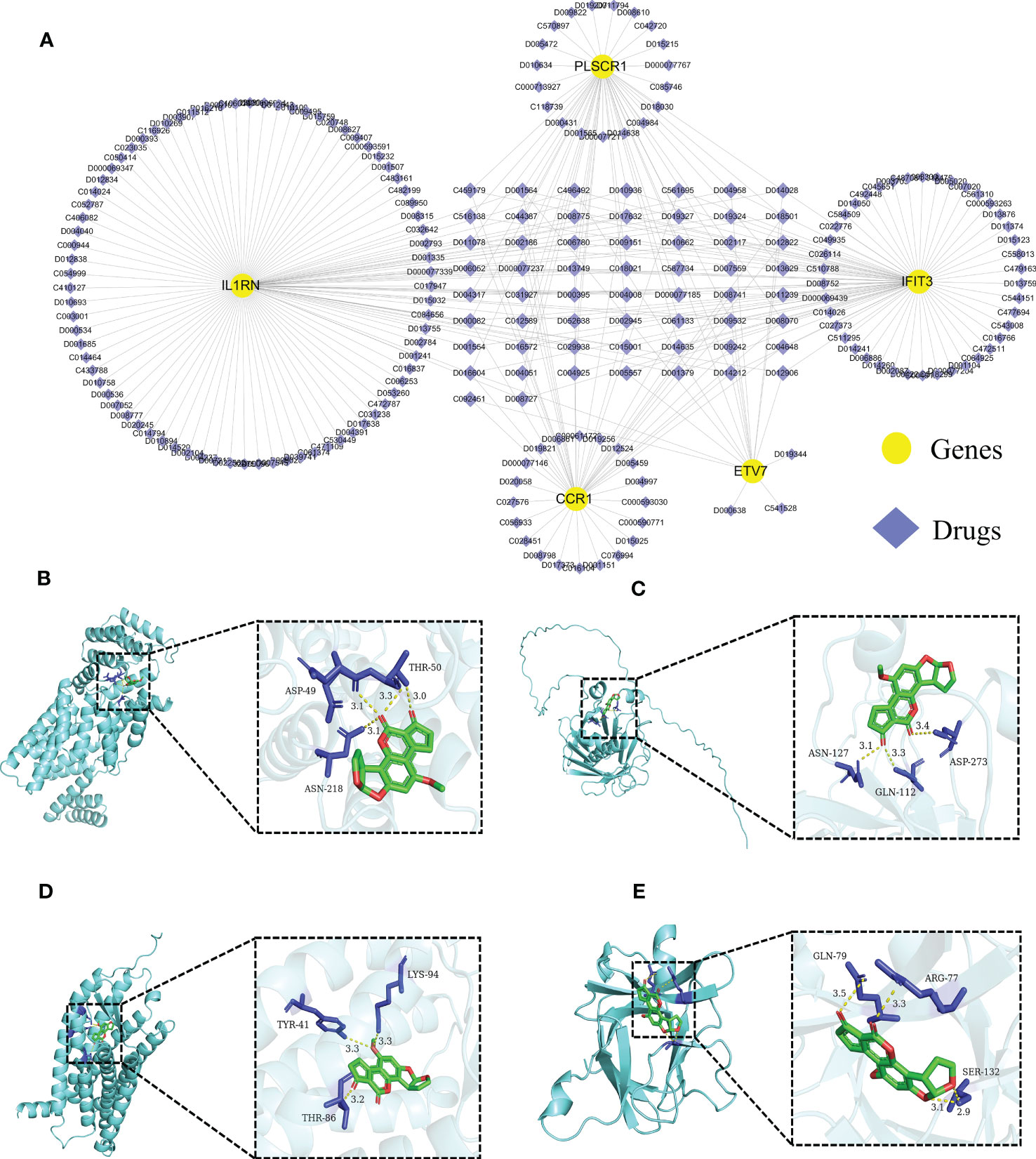
Figure 8 Predicting the drugs targeting the diagnostic biomarkers. (A) Drug-gene network. (B- E) Molecular docking analysis. Aflatoxin B1 was docked with IFIT3, PLSCR1, CCR1, and IL1RN.
4 Discussion
At present, the pathogenesis of SLE has not been fully elucidated. It is widely accepted that SLE develops on a specific genetic background and epigenetic modifications upset the immune system balance, leading to aberrant immune cell proliferation, massive production of autoantibodies, and eventually multiple organ damage (32, 33). However, a single causal gene has not been identified. Conversely, currently, mounting studies reported that multigenic interactions were closely related to SLE initiation and multi-organ involvement (34, 35). Therefore, searching for core molecular clusters is crucial to instruct SLE diagnosis and individualized treatment. Cuproptosis is a newly reported copper-dependent cell death form, mainly manifesting mitochondrial aggrecanase lipoylation, and is closely associated with disease progression (36). However, the specific mechanism and regulatory role of cuproptosis in various diseases have not been further delved into. Hence, we endeavored to elucidate the role of CRGs in SLE phenotype and immune microenvironment.In this study, we analyzed the expression profile of CRGs in the peripheral whole blood of SLE patients. The aberrant gene expression level in SLE patients was higher than in normal individuals, suggesting that CRGs play a significant role in SLE initiation. The correlation among CRGs was calculated to reveal the relationship between CRGs and SLE. Significant synergistic or antagonistic effects were identified among CRGs. Meanwhile, differentiation was observed in immune cell abundance between the control group and SLE patients. SLE patients exhibited a higher infiltration level of neutrophils, memory B cells, plasma cells, and activated DC. Neutrophils play a pathogenic role in multiple AIDs, including SLE (37). Neutrophils can induce plasmacytoid dendritic cells (pDC) to generate interferon (IFN), thereby advancing disease progression (38). Furthermore, the complex genetic background of SLE patients could provide multiple amplification steps for the perpetuation and subsequent pathogenicity of neutrophil-pDC interactions (39). ISG15 in neutrophils may also induce the production of Th1 lymphocytes with pro-inflammatory properties (40). Moreover, by applying unsupervised clustering analysis, we confirmed two distinct clusters based on CRG expression to illustrate the different regulatory patterns of SLE patients. These results demonstrated that CRGs might be the key factors that regulate SLE occurrence and immune infiltration status.
Machine learning is a multidisciplinary discipline, and modeling using the machine learning method can explore the underlying value of data. Additionally, machine learning plays an indispensable role in effectively utilizing data and supporting clinical decisions. In this study, we compared the predictive performance of the 4 machine learning methods (SVM, RF, GLM, and XGB), and constructed a predictive model based on SVM (best performance, AUC = 0.998), suggesting that the SVM model had favorable performance when predicting SLE. An SVM model was established using the 5 important factors (IFIT3, PLSCR1, CCR1, IL1RN, and ETV7). Research showed that IFIT3 belongs to the interferon-induced protein family and is an anti-viral protein (41). IFIT3 can block the synthesis of type I IFN and other inflammatory cytokines via the cGAS/STING pathway (42). PLSCR1 shows increased expression in multiple systemic AIDs, such as primary antiphospholipid syndrome, rheumatoid arthritis, idiopathic inflammatory myopathies, and SLE (43, 44). A correlation was identified between PLSCR1 expression and type I interferon-stimulated genes (45), and PLSCR1 is highly expressed in neutrophils, DC, and macrophages (46). CCR1 is a member of the β-chemokine receptor family and can interact with numerous ligands, such as CCL5, and suppressing CCR1 could improve lupus nephritis progression in New Zealand black/white mice (47). IL1RN is a natural IL-1 inhibitor that can regulate multiple IL-1-related immune and inflammatory responses. IL1RN polymorphism is a factor that affects SLE severity, and IL1RN might be a potential biomarker for SLE (48). Transcription factor ETV7 exhibited elevated expression in SLE (49), which might be induced by IFN-α/γ (50, 51). The SVM model accurately predicted SLE in the validation cohort (AUC = 0.943), providing new insights for SLE diagnosis. More importantly, a nomogram was plotted based on IFIT3, PLSCR1, CCR1, IL1RN, and ETV7 for diagnosing SLE subtypes. The nomogram displayed significant predictive value, suggesting that the model has clinical utility.
In addition, we constructed a CeRNA network using the 5 core diagnostic markers to explore the regulatory mechanism of the core markers. MicroRNA (miRNA) is one of the major epigenetic regulators of SLE-related genes. Remarkable research progress has been made in miRNA-based biomarkers and therapies (52). The CeRNA network illustrated that lncRNA SNHG14 could simultaneously interact with hsa-miR-515-5p and hsa-miR-185-3p, lncRNA SNHG14 could participate in the production of proinflammatory cytokines in rheumatoid arthritis by regulating the MINK1/JNK pathway (53). It was reported that hsa-miR-515-5p regulated WISP1 expression, inhibited the TLR4/JNK signaling pathway, and reduced apoptosis in fibroblast-like synoviocytes (RAFLS) of rheumatoid arthritis (54). The hsa-miR-185-3p modulating transcription factor Foxo1 plays a foremost role in AIDs and can serve as a diagnostic marker of SLE (55–57). We also predicted diagnostic markers-associated drugs using the CTD database, constructed the drug-gene network, and predicted targets of action by constructing molecular docking models. This offered a reference for devising new protocols or investigating potential pathogenic factors for SLE (58). For instance, tretinoin simultaneously targets 5 core diagnostic markers and is a reactive derivative of vitamin A, which can regulate cellular proliferation, differentiation, and maturation (59). Previous studies have indicated that the imbalance of Th17/Treg cells was closely related to the pathogenesis and disease activity of SLE (60). Tretinoin can regulate the balance of Th17/Treg cells by downregulating IL-6Ra expression, which affects the binding of IL-6 to IL-6Ra and gp130, leading to the recruitment of STAT3 and promotion of its phosphorylation to induce ROR γt expression, and ultimately inhibits Th17 cell differentiation and promotes Treg cell proliferation (61, 62). Tretinoin is a potent inhibitor of Pin1, effectively blocking the TLR-7/TLR-9/Pin1/IRAK-1/IRF-7 signaling pathway by inhibiting and degrading activated Pin1, making it an attractive candidate for treating SLE, as Pin1 plays a key role in preventing the progression of the disease (63). Although the potential therapeutic effects of tretinoin are still being explored in this field, it has gained increasing attention, and more research can be carried out to explore its potential therapeutic effects and bring more medical progress.
Nevertheless, there are some limitations to this study. First, this current study is based on bioinformatics, and additional clinical data and experiments are required to verify CRG expression levels. Second, more detailed clinical characteristics are required to identify the performance of the predictive model, and more SLE samples are required to demonstrate the accuracy of the CRG-based model. The correlation between CRGs and immune response needs to be further explored.
Data availability statement
The original contributions presented in the study are included in the article/Supplementary Material. Further inquiries can be directed to the corresponding author.
Author contributions
WL and YS conceived the project and designed the study. YoW and XG analyzed the data and wrote the manuscript. YL and YuW interpreted the data and provided practical resources. MY revised the paper. All authors contributed to the article and approved the submitted version.
Funding
This work was supported by Provincial Natural Science Foundation of Shandong Province (Grant No. ZR2021MH082). This work was also supported by the National Natural Science Foundation of China (Grant No.81801192 and 91639102) and the funding of Taishan Scholars of Shan-dong Province to Binzhou Medical University.
Conflict of interest
The authors declare that the research was conducted in the absence of any commercial or financial relationships that could be construed as a potential conflict of interest.
Publisher’s note
All claims expressed in this article are solely those of the authors and do not necessarily represent those of their affiliated organizations, or those of the publisher, the editors and the reviewers. Any product that may be evaluated in this article, or claim that may be made by its manufacturer, is not guaranteed or endorsed by the publisher.
Supplementary material
The Supplementary Material for this article can be found online at: https://www.frontiersin.org/articles/10.3389/fimmu.2023.1157196/full#supplementary-material
References
1. Tsokos GC, Lo MS, Reis PC, Sullivan KE. New insights into the immunopathogenesis of systemic lupus erythematosus. Nat Rev Rheumatol (2016) 12(12):716–30. doi: 10.1038/nrrheum.2016.186
2. Hermansen ML, Lindhardsen J, Torp-Pedersen C, Faurschou M, Jacobsen S. The risk of cardiovascular morbidity and cardiovascular mortality in systemic lupus erythematosus and lupus nephritis: a Danish nationwide population-based cohort study. Rheumatol (Oxford) (2017) 56(5):709–15. doi: 10.1093/rheumatology/kew475
3. Grammatikos AP, Kyttaris VC, Kis-Toth K, Fitzgerald LM, Devlin A, Finnell MD, et al. A T cell gene expression panel for the diagnosis and monitoring of disease activity in patients with systemic lupus erythematosus. Clin Immunol (2014) 150(2):192–200. doi: 10.1016/j.clim.2013.12.002
4. Kang SC, Hwang SJ, Chang YS, Chou CT, Tsai CY. Characteristics of comorbidities and costs among patients who died from systemic lupus erythematosus in Taiwan. Arch Med Sci (2012) 8(4):690–6. doi: 10.5114/aoms.2012.30293
5. Wakeland EK, Liu K, Graham RR, Behrens TW. Delineating the genetic basis of systemic lupus erythematosus. Immunity (2001) 15(3):397–408. doi: 10.1016/S1074-7613(01)00201-1
6. Kim BE, Nevitt T, Thiele DJ. Mechanisms for copper acquisition, distribution and regulation. Nat Chem Biol (2008) 4(3):176–85. doi: 10.1038/nchembio.72
7. Ge EJ, Bush AI, Casini A, Cobine PA, Cross JR, DeNicola GM, et al. Connecting copper and cancer: from transition metal signalling to metalloplasia. Nat Rev Cancer (2022) 22(2):102–13. doi: 10.1038/s41568-021-00417-2
8. Lutsenko S. Human copper homeostasis: a network of interconnected pathways. Curr Opin Chem Biol (2010) 14(2):211–7. doi: 10.1016/j.cbpa.2010.01.003
9. Guo HR, Wang YQ, Cui HM, Ouyang YJ, Yang TY, Liu CY, et al. Copper induces spleen damage through modulation of oxidative stress, apoptosis, DNA damage, and inflammation. Biol Trace Elem Res (2022) 200(2):669–77. doi: 10.1007/s12011-021-02672-8
10. Jian Z, Guo H, Liu H, Cui H, Fang J, Zuo Z, et al. Oxidative stress, apoptosis and inflammatory responses involved in copper-induced pulmonary toxicity in mice. Aging (Albany NY) (2020) 12(17):16867–86. doi: 10.18632/aging.103585
11. Tsvetkov P, Coy S, Petrova B, Dreishpoon M, Verma A, Abdusamad M, et al. Copper induces cell death by targeting lipoylated TCA cycle proteins. Science (2022) 375(6586):1254–61. doi: 10.1126/science.abf0529
12. Li P, Jiang M, Li K, Li H, Zhou Y, Xiao X, et al. Glutathione peroxidase 4-regulated neutrophil ferroptosis induces systemic autoimmunity. Nat Immunol (2021) 22(9):1107–17. doi: 10.1038/s41590-021-00993-3
13. Tan HY, Wang N, Zhang C, Chan YT, Yuen MF, Feng Y. Lysyl oxidase-like 4 fosters an immunosuppressive microenvironment during hepatocarcinogenesis. Hepatology (2021) 73(6):2326–41. doi: 10.1002/hep.31600
15. Oliver A, Odena A, Raffel CA, Cubuk ED, Goodfellow IJ. Realistic evaluation of deep semi-supervised learning algorithms. 32nd Conference on Neural Information Processing Systems (2018). doi: 10.48550/arXiv.1804.09170
16. Hinton GE, Osindero S, Teh YW. A fast learning algorithm for deep belief nets. Neural Comput (2006) 18(7):1527–54. doi: 10.1162/neco.2006.18.7.1527
17. Li X, Guo F, Zhou Z, Zhang F, Wang Q, Peng Z, et al. [Performance of deep-learning-based artificial intelligence on detection of pulmonary nodules in chest CT]. Zhongguo fei ai za zhi = Chin J Lung Cancer (2019) 22(6):336–40. doi: 10.3779/j.issn.1009-3419.2019.06.02
18. Feeny AK, Chung MK, Madabhushi A, Attia ZI, Cikes M, Firouznia M, et al. Artificial intelligence and machine learning in arrhythmias and cardiac electrophysiology. Circ Arrhythm Electrophysiol (2020) 13(8):e007952. doi: 10.1161/CIRCEP.119.007952
19. Schlegl T, Seeböck P, Waldstein SM, Schmidt-Erfurth U, Langs G. (2017). Unsupervised anomaly detection with generative adversarial networks to guide marker discovery. Information processing in medical imaging, in: 25th International Conference, IPMI 2017, Boone, NC, USA, June 25-30, 2017. pp. 146–57. Proceedings, Springer. doi: 10.48550/arXiv.1703.05921
20. Choi H, Jin KH, Alzheimer’s Disease Neuroimaging Initiative. Predicting cognitive decline with deep learning of brain metabolism and amyloid imaging. Behav Brain Res (2018) 344:103–9. doi: 10.1016/j.bbr.2018.02.017
21. Carpintero MF, Martinez L, Fernandez I, Romero AC, Mejia C, Zang YJ, et al. Diagnosis and risk stratification in patients with anti-RNP autoimmunity. Lupus (2015) 24(10):1057–66. doi: 10.1177/0961203315575586
22. Kennedy WP, Maciuca R, Wolslegel K, Tew W, Abbas AR, Chaivorapol C, et al. Association of the interferon signature metric with serological disease manifestations but not global activity scores in multiple cohorts of patients with SLE. Lupus Sci Med (2015) 2(1):e000080. doi: 10.1136/lupus-2014-000080
23. Chiche L, Jourde-Chiche N, Whalen E, Presnell S, Gersuk V, Dang K, et al. Modular transcriptional repertoire analyses of adults with systemic lupus erythematosus reveal distinct type I and type II interferon signatures. Arthritis Rheumatol (Hoboken N.J.) (2014) 66(6):1583–95. doi: 10.1002/art.38628
24. Langfelder P, Horvath S. WGCNA: an r package for weighted correlation network analysis. BMC Bioinf (2008) 9:559. doi: 10.1186/1471-2105-9-559
25. Wang FZ, Wang B, Long JB, Wang FM, Wu P. Identification of candidate target genes for endometrial cancer, such as ANO1, using weighted gene co-expression network analysis. Exp Ther Med (2019) 17(1):298–306. doi: 10.3892/etm.2018.6965
26. Ravasz E, Somera AL, Mongru DA, Oltvai ZN, Barabasi AL. Hierarchical organization of modularity in metabolic networks. Science (2002) 297(5586):1551–5. doi: 10.1126/science.1073374
27. Gold C, Sollich P. Model selection for support vector machine classification. Neurocomputing (2003) 55(1):221–49. doi: 10.1016/S0925-2312(03)00375-8
29. Nelder JA, Wedderburn RWM. Generalized linear models. J R Stat Soc (1972) 135(3):370–84. doi: 10.2307/2344614
30. Chen T, He T, Benesty M, Khotilovich V, Tang Y, Cho H, et al. Xgboost: extreme gradient boosting. The annals of statistics (2015) 1(4):1–4.
31. Newman AM, Liu CL, Green MR, Gentles AJ, Feng W, Xu Y, et al. Robust enumeration of cell subsets from tissue expression profiles. Nat Methods (2015) 12(5):453–7. doi: 10.1038/nmeth.3337
32. Bakshi J, Segura BT, Wincup C, Rahman A. Unmet needs in the pathogenesis and treatment of systemic lupus erythematosus. Clin Rev Allergy Immunol (2018) 55(3):352–67. doi: 10.1007/s12016-017-8640-5
33. Gordon C, Amissah-Arthur MB, Gayed M, Brown S, Bruce IN, D'Cruz D, et al. The British society for rheumatology guideline for the management of systemic lupus erythematosus in adults. Rheumatol (Oxford) (2018) 57(1):e1–e45. doi: 10.1093/rheumatology/kex286
34. Zakharova MY, Belyanina TA, Sokolov AV, Kiselev IS, Mamedov AE. The contribution of major histocompatibility complex class II genes to an association with autoimmune diseases. Acta Naturae (2019) 11(4):4–12. doi: 10.32607/20758251-2019-11-4-4-12
35. Luque A, Serrano I, Ripoll E, Malta C, Goma M, Blom AM, et al. Noncanonical immunomodulatory activity of complement regulator C4BP(beta-) limits the development of lupus nephritis. Kidney Int (2020) 97(3):551–66. doi: 10.1016/j.kint.2019.10.016
36. Tang D, Chen X, Kroemer G. Cuproptosis: a copper-triggered modality of mitochondrial cell death. Cell Res (2022) 32(5):417–8. doi: 10.1038/s41422-022-00653-7
37. Papayannopoulos V. Neutrophil extracellular traps in immunity and disease. Nat Rev Immunol (2018) 18(2):134–47. doi: 10.1038/nri.2017.105
38. Chasset F, Arnaud L. Targeting interferons and their pathways in systemic lupus erythematosus. Autoimmun Rev (2018) 17(1):44–52. doi: 10.1016/j.autrev.2017.11.009
39. Moser KL, Kelly JA, Lessard CJ, Harley JB. Recent insights into the genetic basis of systemic lupus erythematosus. Genes Immun (2009) 10(5):373–9. doi: 10.1038/gene.2009.39
40. Carrillo-Vazquez DA, Jardon-Valadez E, Torres-Ruiz J, Juarez-Vega G, Maravillas-Montero JL, Meza-Sanchez DE, et al. Conformational changes in myeloperoxidase induced by ubiquitin and NETs containing free ISG15 from systemic lupus erythematosus patients promote a pro-inflammatory cytokine response in CD4(+) T cells. J Transl Med (2020) 18(1):429. doi: 10.1186/s12967-020-02604-5
41. Fleith RC, Mears HV, Leong XY, Sanford TJ, Emmott E, Graham SC, et al. IFIT3 and IFIT2/3 promote IFIT1-mediated translation inhibition by enhancing binding to non-self RNA. Nucleic Acids Res (2018) 46(10):5269–85. doi: 10.1093/nar/gky191
42. Wang JH, Dai M, Cui YG, Hou GJ, Deng J, Gao X, et al. Association of abnormal elevations in IFIT3 with overactive cyclic GMP-AMP Synthase/Stimulator of interferon genes signaling in human systemic lupus erythematosus monocytes. Arthritis Rheumatol (2018) 70(12):2036–45. doi: 10.1002/art.40576
43. Bernales I, Fullaondo A, Marin-Vidalled MJ, Ucar E, Martinez-Taboada V, Lopez-Hoyos M, et al. Innate immune response gene expression profiles characterize primary antiphospholipid syndrome. Genes Immun (2008) 9(1):38–46. doi: 10.1038/sj.gene.6364443
44. O'Hanlon TP, Rider LG, Gan L, Fannin R, Paules RS, Umbach DM, et al. Gene expression profiles from discordant monozygotic twins suggest that molecular pathways are shared among multiple systemic autoimmune diseases. Arthritis Res Ther (2011) 13(2):R69. doi: 10.1186/ar3330
45. Deng Y, Zheng Y, Li D, Hong Q, Zhang M, Li Q, et al. Expression characteristics of interferon-stimulated genes and possible regulatory mechanisms in lupus patients using transcriptomics analyses. EBioMedicine (2021) 70:103477. doi: 10.1016/j.ebiom.2021.103477
46. Bing PF, Xia W, Wang L, Zhang YH, Lei SF, Deng FY. Common marker genes identified from various sample types for systemic lupus erythematosus. PloS One (2016) 11(6):e0156234. doi: 10.1371/journal.pone.0156234
47. Bignon A, Gaudin F, Hemon P, Tharinger H, Mayol K, Walzer T, et al. CCR1 inhibition ameliorates the progression of lupus nephritis in NZB/W mice. J Immunol (2014) 192(3):886–96. doi: 10.4049/jimmunol.1300123
48. Tsai LJ, Lan JL, Lin CY, Hsiao SH, Tsai LM, Tsai JJ. The different expression patterns of interleukin-1 receptor antagonist in systemic lupus erythematosus. Tissue Antigens (2006) 68(6):493–501. doi: 10.1111/j.1399-0039.2006.00704.x
49. Idborg H, Zandian A, Sandberg AS, Nilsson B, Elvin K, Truedsson L, et al. Two subgroups in systemic lupus erythematosus with features of antiphospholipid or sjogren's syndrome differ in molecular signatures and treatment perspectives. Arthritis Res Ther (2019) 21:62. doi: 10.1186/s13075-019-1836-8
50. Irudayam JI, Contreras D, Spurka L, Subramanian A, Allen J, Ren S, et al. Characterization of type I interferon pathway during hepatic differentiation of human pluripotent stem cells and hepatitis c virus infection. Stem Cell Res (2015) 15(2):354–64. doi: 10.1016/j.scr.2015.08.003
51. Minutti CM, Garcia-Fojeda B, Saenz A, de Las Casas-Engel M, Guillamat-Prats R, de Lorenzo A, et al. Surfactant protein a prevents IFN-gamma/IFN-gamma receptor interaction and attenuates classical activation of human alveolar macrophages. J Immunol (2016) 197(2):590–8. doi: 10.4049/jimmunol.1501032
52. Hong SM, Liu C, Yin Z, Wu L, Qu B, Shen N. MicroRNAs in systemic lupus erythematosus: a perspective on the path from biological discoveries to clinical practice. Curr Rheumatol Rep (2020) 22(6):17. doi: 10.1007/s11926-020-00895-7
53. Zhang J, Lei H, Li X. LncRNA SNHG14 contributes to proinflammatory cytokine production in rheumatoid arthritis via the regulation of the miR-17-5p/MINK1-JNK pathway. Environ Toxicol (2021) 36(12):2484–92. doi: 10.1002/tox.23361
54. Cai D, Hong S, Yang J, San P. The effects of microRNA-515-5p on the toll-like receptor 4 (TLR4)/JNK signaling pathway and WNT1-Inducible-Signaling pathway protein 1 (WISP-1) expression in rheumatoid arthritis fibroblast-like synovial (RAFLS) cells following treatment with receptor activator of nuclear factor-kappa-B ligand (RANKL). Med Sci Monit (2020) 26:e920611. doi: 10.12659/MSM.920611
55. Zhou SL, Zhang J, Luan PF, Ma ZB, Dang J, Zhu H, et al. miR-183-5p is a potential molecular marker of systemic lupus erythematosus (vol 2021, 5547635, 2021). J Immunol Res (2021) 2021:5547635. doi: 10.1155/2021/5547635
56. Wan C, Ping CY, Shang XY, Tian JT, Zhao SH, Li L, et al. MicroRNA 182 inhibits CD4(+)CD25(+)Foxp3(+) treg differentiation in experimental autoimmune encephalomyelitis. Clin Immunol (2016) 173:109–16. doi: 10.1016/j.clim.2016.09.008
57. Yang X, He QY, Guo ZZ, Xiong F, Li Y, Pan Y, et al. MicroRNA-425 facilitates pathogenic Th17 cell differentiation by targeting forkhead box O1 (Foxo1) and is associated with inflammatory bowel disease. Biochem Bioph Res Co (2018) 496(2):352–8. doi: 10.1016/j.bbrc.2018.01.055
58. Chu Y, Zhao C, Zhang B, Wang X, Wang Y, An J, et al. Restoring T-helper 17 cell/regulatory T-cell balance and decreasing disease activity by rapamycin and all-trans retinoic acid in patients with systemic lupus erythematosus. Lupus (2019) 28(12):1397–406. doi: 10.1177/0961203319877239
59. Cassani B, Villablanca EJ, De Calisto J, Wang S, Mora JR. Vitamin a and immune regulation: role of retinoic acid in gut-associated dendritic cell education, immune protection and tolerance. Mol Aspects Med (2012) 33(1):63–76. doi: 10.1016/j.mam.2011.11.001
60. Ma J, Yu J, Tao X, Cai L, Wang J, Zheng SG. The imbalance between regulatory and IL-17-secreting CD4+ T cells in lupus patients. Clin Rheumatol (2010) 29:1251–8. doi: 10.1007/s10067-010-1510-7
61. Wang X, Wang W, Xu J, Wu S, Le Q. All-trans retinoid acid promotes allogeneic corneal graft survival in mice by regulating treg-Th17 balance in the presence of TGF-β. BMC Immunol (2015) 16(1):1–13. doi: 10.1186/s12865-015-0082-3
62. Ivanov II, Zhou L, Littman DR. Transcriptional regulation of Th17 cell differentiation. In: Seminars in immunology. Elsevier (2007). p. 409–17. doi: 10.1016/j.smim.2007.10.011
Keywords: systemic lupus erythematosus, WGCNA, machine learning, immune infiltration, biomarker
Citation: Li W, Guan X, Wang Y, Lv Y, Wu Y, Yu M and Sun Y (2023) Cuproptosis-related gene identification and immune infiltration analysis in systemic lupus erythematosus. Front. Immunol. 14:1157196. doi: 10.3389/fimmu.2023.1157196
Received: 17 February 2023; Accepted: 17 May 2023;
Published: 29 May 2023.
Edited by:
Evangelia Sarandi, University of Crete, GreeceReviewed by:
Uma Sriram, Temple University, United StatesPrathyusha Bachali, AMPEL BioSolutions, United States
Copyright © 2023 Li, Guan, Wang, Lv, Wu, Yu and Sun. This is an open-access article distributed under the terms of the Creative Commons Attribution License (CC BY). The use, distribution or reproduction in other forums is permitted, provided the original author(s) and the copyright owner(s) are credited and that the original publication in this journal is cited, in accordance with accepted academic practice. No use, distribution or reproduction is permitted which does not comply with these terms.
*Correspondence: Yeying Sun, c3VueXkyMWNuQGdtYWlsLmNvbQ==