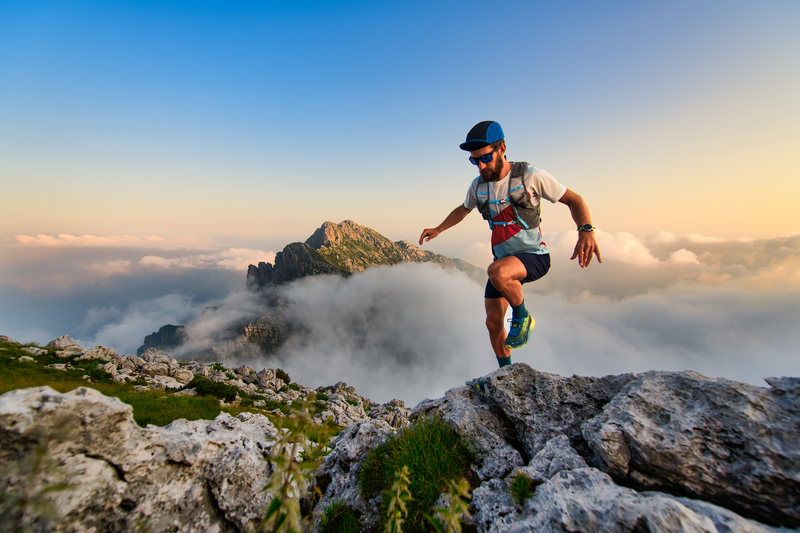
94% of researchers rate our articles as excellent or good
Learn more about the work of our research integrity team to safeguard the quality of each article we publish.
Find out more
REVIEW article
Front. Immunol. , 14 April 2023
Sec. Immunological Memory
Volume 14 - 2023 | https://doi.org/10.3389/fimmu.2023.1127470
This article is part of the Research Topic Women in Immunological Memory View all 4 articles
Antigen-specific T cells play a central role in the adaptive immune response and come in a wide range of phenotypes. T cell receptors (TCRs) mediate the antigen-specificities found in T cells. Importantly, high-throughput TCR sequencing provides a fingerprint which allows tracking of specific T cells and their clonal expansion in response to particular antigens. As a result, many studies have leveraged TCR sequencing in an attempt to elucidate the role of antigen-specific T cells in various contexts. Here, we discuss the published approaches to studying antigen-specific T cells and their specific TCR repertoire. Further, we discuss how these methods have been applied to study the TCR repertoire in various diseases in order to characterize the antigen-specific T cells involved in the immune control of disease.
The innate and adaptive arm of the immune system work in coordination to elicit host immune responses against a variety of pathogens. The adaptive response, a crucial component of the immune system, relies on antigen-specificity, and mediates action via B and T cells (1). These cells have a diverse and finely tuned repertoire of receptors with the ability to discriminate between self and non-self-antigens (2, 3). Upon antigen-specific activation individual T and B cells undergo clonal expansion to produce a population of identical antigen-reactive cells (4–6). While B cells are responsible for antibody mediated responses, T cells disseminate their action via a plethora of cell mediated responses (7). T cells are also critical effector cells for providing protection against wide range of pathogens and cancer, as well as maintaining self-tolerance. This broad range of functions is enabled by the diversity of T cell phenotypes and antigen specificities. T cell phenotypes range from highly cytotoxic effector cells to regulatory T cells that fight inflammation. These effector cells include the CD4 and CD8 lineage. Unlike CD4 T cells, which normally focus on protein antigens sourced from the extracellular environment, CD8 T cells preferentially identify antigens that are biosynthesized by infected or altered host cells. Both CD4 and CD8 T cells differentiate into independent memory cell lineages that release various and frequently mutually exclusive sets of cytokines in response to antigen contact and the proper co-stimulatory cues (8, 9). There are several different CD4 T helper (Th) subsets that have been found, but the Th1, Th2, and Th17 lineages are the most well-known. However, other CD4 Th cell subsets, such as the Th1*, especially in the context of mycobacterial infection (10–12), and Th9 and Th22 subsets that release IL-9 and IL-22, have become more well-known in recent years (13). The wide diversity of T cell phenotypes is a direct result of the wide repertoire of T cell receptors (TCR) and range of T cell antigen-specificities. In this review, we will be focusing on antigen-specificity in the context of T cells and their TCRs and discussing it in detail.
T cells are key mediators in mounting an effective cell mediated immune response. During T cell development, T cell precursors travel to the thymus, where they develop into mature T cells and are exported to the periphery where they can be activated by antigens and differentiate into effector and memory cells. T cell development is largely dependent on T cell receptor interactions which facilitates the transition of double positive T cell progenitors (CD4+ and CD8+) to single positive cells (CD4+ or CD8+) after thymal selection. During this selection, T cells bearing TCR with a high affinity for self-peptide Major Histocompatibility Complexes (MHC) undergo apoptosis (negative selection), whereas those bearing low-affinity TCR for self-peptides survive and differentiate into mature T cells (positive selection). This ensures that only those T cells that are self-tolerant survive while eliminating the self-reactive T cells. The T cells leaving the thymus are functional cells expressing unique and specific T-cell receptors (TCRs) that are both tolerant to self-antigens and restricted to self-MHC (14). These cells have the ability to responds to new antigens with a wide array of antigen specificities due to highly diverse TCRs. An immune response is initiated when naïve single positive T cells encounter processed antigen presented by antigen presenting cells (APCs). The TCR of naïve single positive T cells binds to the antigen-MHC complex which results in the proliferation and differentiation of antigen-specific effector cells that migrate to diverse sites and aid in pathogen clearance (15). The activated cells are short lived, although a subset of cells survive as memory T cell maintaining long term immunity (8, 16). It is very important to study these antigen-specific T cells as they play a central role in the adaptive immune response in various pathologies. In cancer, tumor-specific T cells are involved in effective anti-tumor immunity, whereas in infectious diseases, pathogen-specific T cells coordinate specific defense mechanisms. Apart from pathological contexts, antigen-specific T cells are also crucial for the formation of immunological memory (e.g., after vaccination) or for the maintenance of tolerance to self-antigens. The various applications of antigen-specific T cells and their TCRs will also be discussed in detail in this review.
T cells provide protection by recognizing processed antigens presented on Major Histocompatibility (MHC) molecules using its highly specific TCR. The ensemble of TCRs that are present within an individual at a particular time is called the TCR repertoire which are generated by the process of random recombination and selection. Each T cell expresses a unique TCR on its surface which facilitates antigen recognition and subsequent immune responses, as well as adding another dimension of variation within T cell populations.
The core TCR complex contains two TCR chains and six complementarity-determining regions (CDR). There are four TCR genes in the human genome; TCRα, TCRβ, TCRγ, and TCRδ. The majority of T cells express α and β isoforms to form a heterodimer i.e., αβ T cells or more generally referred to as just T cells. The α/β TCRs bind to antigenic peptides presented in molecular grooves on the surface of MHC I or MHC II molecules present on APCs (17–20). Only a small proportion of T cells express TCRγ and TCRδ isoforms known as γδ T cells. TCR chains are made up of an extracellular region, a transmembrane region and a short cytoplasmic tail. The extracellular region consists of a variable domain (V) that serves as antigen binding site and a constant domain (C) used for interaction with CD3 chains (21). The V domain typically has a marked sequence variation while the rest of the chain remains conserved. Each V domain has three Complementarity-determining regions (CDR). Various studies from the past few decades showed that the CDR1 and CDR2 regions of the TCR interact with MHC while the CDR3 region interacts with the antigenic peptide (22–25). The TCR is glycosylated, and recent studies have shown that the amount of glycosylation may vary depending on the level of T cell activation (26, 27). The generation of T cell diversity arises from genetic recombination of DNA encoded segments by combinatorial somatic V (variable), D (diversity) and J (joining) recombination using RAG1 and RAG2 recombinases. The α chain is generated by VJ recombination while the β chain is generated by VDJ recombination. Theoretically, a TCR repertoire consists of 2x1019 unique TCRαβ pairs generated by the recombination process along with non-templated addition or deletion of nucleotides between spliced gene segments. Therefore, each T cell repertoire is shaped by both genetically determined biases, as well as immune exposures. However, the actual diversity is likely lower, which can potentially be explained by the selection process and number of T cells present. However, it has not yet been thoroughly investigated how much genetic background of an individual influences the diversity of their TCR repertoire.
In recent years, high-throughput TCR profiling has been widely used to define the interaction between TCRs and the matching peptide/MHC complexes. A TCR sequence can be used as a unique identifier of T cell clones and can be used for measuring antigen-driven clonal expansion of T cells. Characterizing the TCR repertoire can describe T cell dynamics in a wide range of diseases, including malignancies, autoimmune disorders, and infectious diseases. With the help of TCR sequencing, some studies have demonstrated that T cell populations change after numerous immunization cycles or pathogen exposure (28, 29). This change is crucial for the understanding of disease pathology and when designing therapeutic strategies. Studying the TCR diversity in patients across time can also shed light on the kinetics of T cell clones that may correlate with clinically relevant features or ongoing treatment. In this review, we highlight both TCR-sequence dependent and independent techniques of identifying antigen-specificity ex vivo and in vitro and their potential applications in different diseases.
Antigen-specificity of a T cell can be deciphered directly using peptide-MHC (pMHC) multimers, and/or indirectly using surrogate markers of antigen-specificity.
The gold standard technique to identify antigen-specific T cells is the use of peptide-MHC (pMHC) multimers, which are stable complexes of several identical pMHC monomers that can specifically bind to a given TCR (30, 31). The seminal and still most commonly used scaffold type is a tetramer (i.e., containing 4 pMHC) (30), but other multimers exist such as dimers, pentamers and dextramers (32, 33). The pMHC multimers are typically conjugated to a fluorochrome, and thus enable for direct visualization and isolation of antigen-specific T cells using flow cytometry. The pMHC multimers can be used for the study of both MHC-I restricted (CD8) and MHC-II restricted (CD4) T cell populations. Their use is more prevalent for the analysis of antigen-specific CD8 T cells, as pMHC-I multimers are associated with greater TCR affinity compared to pMHC-II multimers (34), and epitope/MHC restriction prediction tools generally perform better for MHC-I than for MHC-II epitopes (35). A myriad of studies, that have been reviewed previously (36–38), has used pMHC multimers for identifying and monitoring the frequency, phenotype and function of antigen-specific T cells in the context of infectious diseases, vaccination, auto-immunity, allergy, and cancer.
The main advantage of pMHC multimers is their usability to identify antigen-specific T cells ex vivo. Thus, unlike assays requiring in vitro stimulation that will significantly affect the cell transcriptome and phenotype, tetramers can give information on the phenotype of antigen-specific T cells in vivo at the time of sampling. Additionally, since pMHC multimers directly bind antigen-specific T cells, the risk of contamination with bystander activated T cells is lower compared to indirect antigen-specific T cell profiling techniques, for instance those based on the production of pro-inflammatory cytokines after in vitro stimulation (39). Lastly, a key advantage of pMHC multimers is that they can be used without the presence of Antigen presenting cells (APC), for instance on T cell clones derived from tumor infiltrating lymphocytes or TCR transduced T cell lines (40). The major limitation of pMHC multimers is that the exact epitope sequence and its associated MHC restriction are required for each individual antigen-specific T cell population targeted to study, limiting their application to already well-characterized epitopes. Another limitation of pMHC multimers is that the frequency of binding T cells is generally very low, and therefore requires analysis of large sample volumes. Moreover, due to low sensitivity, staining protocols need to be carefully optimized for each pMHC multimer to achieve maximum specificity and sensitivity (40–42).
Since their first application in 1996, significant advances have been made to tackle the limitations of pMHC multimers. New technologies use heavy metal or DNA labelled pMHC multimers that enable the simultaneous interrogation of hundreds to thousands of epitope specificities in a single sample at the single-cell level (43, 44). More recently, the development of spheromers, a multivalent self-assembly system that simultaneously displays six pMHC dimers, allows for the detection of antigen-specific CD4 and CD8 T cells at much greater specificity and sensitivity compared to their tetramer and dextramers counterparts (45) (Figure 1). Furthermore, the development of MHC epitope prediction tools, as well as community-based epitope databases such as the Immune Epitope Database (IEDB) (46), were instrumental to identify new epitope/MHC combinations that can be used for multimers. More recently, the discovery of non-classical T cells and their ligands has initiated the development of non-classical MHC multimers tailored for the study of antigen-specific NKT, γδT or MAIT cells (47). Despite all these advances, it remains questionable whether the pMHC multimer technology will ever be sufficient to create a complete list of all classically restricted epitopes and ligands of non-conventional T cells that are being recognized in the global human population.
Figure 1 Methods to identify antigen-specificity. pMHC multimers allows the direct identification of antigen-specific T cells, while indirect methods rely on cytokine production, surface marker expression or cell proliferation upon in vitro stimulation. Created with BioRender.com.
In conclusion, pMHC multimers can be used to directly label antigen-specific T cells and bypass the need for in vitro stimulation. Due to their limitations in design and optimal use, they are best suited for studies restricted to a few well-characterized antigen-specific T cell populations.
Indirect analysis of T cell antigen specificity requires in vitro stimulation to measure specific T cell features. Most notable/commonly used features are cytokine production, proliferation and the expression of activation induced markers (AIM) (Figure 1). The complexity and duration of in vitro stimuli used in these assays vary from single peptides, multiepitope pools (so called “megapools”) to whole pathogen preparations that can last from a few hours to several days. Longer stimulations are preferred when the aim is to enrich cell populations that are low in numbers, while shorter stimulations limit the possibility of bystander activation.
The most commonly used cytokine to define antigen-specificity is IFN-γ. Along with TNF-α and IL2, it is typically used to define Th1 polarized antigen-specific T cells. However, using different cytokine combinations, many other T helper cell subsets can be defined, such as Th2 (producing IL-4, IL-5, and IL-13) or Th17 (producing IFN-γ, IL-17) cells. The two main methods to measure cytokine production after antigen-specific stimulation are ELISpot/FluoroSpot and intracellular cytokine staining (ICS).
The ELISpot assay is highly validated (48) and currently one of the most frequently used assays (49) to detect and quantify antigen-specific T cell responses in peripheral blood in clinical trials (50). Whereas these assays were originally designed to capture only one cytokine, the FluoroSpot technology can simultaneously measure up to four cytokines and this number is likely to increase in the future (51, 52). ELISpot/FluoroSpot assays are powerful due to is their high sensitivity. Less than 100 cytokine molecules can be detected and the lower detection limit can be less than 10 cytokine-producing cells per million cells (53). Their major limitation is the inability to identify/phenotype the exact cells responsible for the response.
In ICS, antigen-stimulated cells are fixed and stained with intracellular fluorescently labeled cytokine-targeting antibodies and subsequently analyzed via flow cytometry (54, 55). The main advantage of ICS is that cytokine production can be measured per cell. This method allows for the quantification of the cells that produce the targeted cytokine/s (56), as well as deducing their exact phenotype (e.g., memory/naïve phenotype). The main disadvantage of the ICS is that it requires cell fixation, which can preclude downstream analysis. Cytokine-based assays are thus easily performed and are ideal for routine analysis in many clinical trials. They can be used to study antigen-specific T cell populations with a well-defined cytokine polarization profile.
Another widely used technique for capturing antigen-specificity is through cell proliferation assays. Typically, these assays use cell proliferation dyes, such as CFSE or Cell Trace Violet, to fluorescently label all cells prior to stimulation (57, 58). Upon division, each daughter cell will receive half of the fluorescence intensity from the original cell which enables tracking the number of divisions each cell has undergone via flow cytometry. Similar to ICS, this assay offers single-cell resolution and can be used to determine not only the frequency but also the phenotype of dividing cells. This assay is also particularly well suited for the identification of rare T cell populations, such as allergen- (59) or autoantigen- (60) specific T cells. Proliferation dyes are limited by their toxicity and requires careful protocol optimization to obtain a stable and homogenous fluorescence intensity in the originally labelled cell population. Dying cells also lose fluorescence intensity, but newer versions that overcome these challenges are constantly emerging (58, 61).
The co-expression of surface protein activation markers upon in vitro stimulation is measured via flow cytometry and used to identify antigen-specific T cells (62, 63). Well defined activation markers are: CD20, CD25, CD40L, CD69, CD107a, CD137, CCR7 CXCR5, OX40, PD-1, and PD-L1. Unlike cytokine assays, AIM assays are not limited to a handful of cytokines, and are also more sensitive. Using the AIM assay, Bowyer et al. detected more antigen-specific T cells compared to ELISpot and ICS with comparable (CD4+) or even lower (CD8+) background signal (64). AIM assays have been successfully applied to identify antigen-specific T cells in the context of infection and vaccination (65–67) and their popularity is increasingly growing. A variation of AIM is the ARTE (Antigen Reactive T cell Enrichment) assay. Upon interaction with APCs, CD154 expression peaks at 6-8 hours on CD4 T cells and are captured by magnetic enrichment prior to stimulation. This method provides a reliably way to isolate rare populations of T cells thus making it a popular choice for studying antigen-specificity (68–71).
The TCR is composed of two different chains, each containing variable and constant regions. The CDR3 region of each chain is associated with the highest number of recombination events, and is directly in contact with the epitope, thus critical for antigen recognition. A given TCR sequence will be shared by all cells originating from the same T cell, and all T cells from that clone will share the same antigen specificity. Thus, the association of TCR sequences to antigen specificity could, in theory, be used to analyze the entire antigen-specific T cell repertoire in a given individual, without prior knowledge of the MHC sequence/restriction (as needed with tetramers). Additionally, TCR sequences could be used to trace the fate of clones over time and pre-post stimulation. This technique could be applied on longitudinal samples of a disease cohort undergoing treatment, pre-post vaccination, and also before and after in vitro stimulation (discussed below). In this section, we review the current techniques of TCR sequencing and how it can be linked to antigen-specificity in T cells.
TCR sequencing techniques can be broadly divided into two categories: i) direct techniques that perform targeted sequencing of the TCR, and ii) indirect techniques that employ bioinformatic algorithms to derive TCR sequences or specificities from bulk or single-cell RNA sequencing data.
Targeted TCR sequencing is typically done using first a targeted amplification step with specific primers spanning the CDR3 region (72, 73). For targeted TCR sequencing, the starting material can be either genomic DNA or cDNA depending on the context and has its own pros and cons (74). The first TCR repertoire analyses were done using bulk TCR sequencing. Pioneer studies include the ones from Freeman et al. (75) and Robins et al. (76), which identified tens of thousands of distinct TCR beta chain sequences in the peripheral blood of healthy individuals, revealed a higher repertoire diversity than previously expected. With rapid technological advances, targeted TCR sequencing can now be performed at the single-cell level, in combination with single-cell RNA sequencing (72, 73). It is even possible to get the entire TCR repertoire of a given sample at the single-cell level, in one single experiment (77). The advantage of bulk over single-cell TCR sequencing is that it is less expensive, and can be done on a larger number of cells, so it is a great option for mining the entire TCR repertoire of a given sample. Conversely, the advantage of single-cell TCR sequencing is that it offers chain pairing, and can provide functional information on a given TCR cell clone (e.g., transcriptome).
Since TCR αβT cells represent the majority of T cells in humans, and have been extensively studied for their MHC/peptide mediated antigen-specificity, the vast majority of TCR sequencing tools available are tailored for their use. Bulk TCR sequencing is almost exclusively done on the TCRβ-chain, as it contains a higher diversity compared to the α-chain (78). More recently, novel tools have been developed for the study of the TCR repertoire of non-conventional T cells. For instance, using custom designed primers, direct sequencing of TCRγ and TCRδ chains from γδT cells can be done at both bulk (79) and single-cell level (80). The study of antigen-specific TCRs in non-conventional T cells is reviewed in more detail in the following sections.
Repertoire sequencing can be expensive and consumes samples that may be available in limited supply. Repertoire construction tools offer an alternative to this problem by mining RNA-seq data for TCR and BCR sequences. Algorithms that can infer the full-length sequence of the immune receptor are preferred as it can facilitate better receptor-antigen interaction modeling. However, computational methods such as V’DJer (81), MiXCR (82), CATT (83) and ImRep (84) can only reconstruct CDR3, and are thus limited in their use to assemble full-length V(D)J receptor sequences. For single-cell platforms such as SMART-seq, pre-existing tools such as BALDR (85), BASIC (86), and VDJPuzzle (87), and even MiXCR have now been developed to construct full-length paired TCR or BCRs (88). Another tool that has gained popularity recently is TRUST4 which is a redesign of the TRUST algorithm but with substantially enhanced features and performance for αβ/γδ T cells and B cells from both bulk and single-cell RNA-seq (89). TRUST4 has proved to be far superior to other comparable methods such as MiXCR and CATT in both performance, sensitivity and speed.
Both direct and indirect techniques produce TCR sequences which can be used in conjunction with other methods to infer antigen-specificity either at bulk or single-cell level. For e.g., cells expressing AIM markers could be RNA sequenced and their respective TCR repertoires can be generated for downstream analysis. This is a powerful method to associate both cellular phenotypes with specific TCR sequences and thus can shed light on clonality of antigen-specific T cells. In this section we discuss how this can be achieved using both experimental and/or computational methods (Table 1).
A relatively straight-forward technique to determine the antigen-specific TCR repertoire is using in vitro cultures to investigate the specific expansion of antigen-reactive clonotypes (90). This method used by our group has successfully determined both vaccine-antigen and auto-antigen specific TCRs (90) (Figure 2). PBMCs were stimulated with antigens of interest for 14-days in vitro after which the TCR repertoire was determined. Statistical variation was controlled for by culture replicates. Bystander activated cells and/or cells already activated by antigens before the sample was taken was controlled for by including an unrelated antigen and excluding TCRs that are expanded under multiple conditions. To determine which TCRs that expanded the productive repertoire was compared to an ex vivo sample of T cells from the same participant. This allowed identification of the TCRs that were antigen-specific, and subsequent computational analysis of public clonotypes and clonotype groups (as described below). We have implemented this method at the single-cell level in a group of TB patients undergoing treatment to isolate ex vivo antigen-specificity. Our goal is to 1) identify all cell phenotypes associated with antigen-specificity and 2) trace their fate from diagnosis/pre-treatment to treatment success. For in vitro stimulation component we employed megapools, which are synthetic peptides designed to carry proteins or epitopes of interest (107–111). They can be selected based on MHC binding to target either CD4 or CD8 T cell responses (35, 112). The advantage of megapools is the high rate of reproducibility of results due to limited variation between batches. Our group designed a Mtb-specific peptide pool of 300 MHC class II restricted epitopes (MTB300) which we and others have validated (113–116) for this purpose. Due to the overlap of epitopes recognized by MHC of multiple species, MTB300 has shown to capture T cell reactivity in mice and non-human primates, attesting to its versatility (115, 117–120).
Figure 2 Antigen-specific TCR repertoire using in vitro culture. Cells are stimulated with antigens or epitope pools. Only those clonotypes that expanded upon stimulation compared to ex vivo T cell samples across both replicates are analyzed further. The clonotypes are overlapped to obtain antigen-specific clonotypes. Clonotypes that expand in response to multiple unrelated stimuli are excluded.
Combining tetramer technology with single-cell sequencing has enabled the interrogation of antigen-specificity directly ex vivo. Tetramer-associated TCR sequencing (tetTCR-seq) allows to simultaneously profile TCR sequences, cognate antigen specificities, gene and surface protein expression at the single-cell level (91, 92). As highlighted by Zhang et al., large library of fluorescently labeled, DNA-barcoded pMHC tetramers were constructed using in vitro transcription/translation (IVTT). Next, tetramer-stained cells were single-cell sorted and the DNA-BC and TCR αβ genes were amplified by RT-PCR. A molecular identifier was included in the DNA-barcode to provide absolute counting of the copy number for each species of tetramers bound to the cell. Finally, nucleotide-based cell barcodes were used to link multiple peptide specificities with their bound TCRαβ sequences. DNA-barcoded pMHC tetramers were compatible with isolation of rare antigen-binding precursor T cells (121), making tetTCR-Seq a versatile platform to analyze both clonally expanded and precursor T cells.
Microfluidic techniques such as MATE-seq uses magnetic nanoparticle-barcoded pMHC tetramers linked to photocleavable TCR-specific primers to capture both TCR sequence and antigen-identity within droplets (93). T cells are incubated with a library of nanoparticle-barcoded pMHCs and purified magnetically. The single cells are captured as droplets and lysed, and the nanoparticle-barcoded pMHCs are exposed to UV light, releasing RT-PCR primers targeting TCR αβ C regions. Because these primers are linked to a DNA barcode corresponding to the pMHC, the TCR sequence and antigen specificity can be coupled at the single-cell level even after pooling and sequencing. There are two major limitations to this method. 1) This method is limited to a few pMHC tetramers due to limitations in library construction, 2) It can only be applied to T cells with known antigen-specificities (72).
ENTER-seq (lentiviral-mediated cell entry by engineered ligand-receptor interaction) uses engineered lentiviruses at multiple levels to systematically deorphanize TCR-pMHC interactions. GFP fused viruses with single chain of MHC infused with beta 2 microglobulin and covalently linked peptides are used to determine if the viruses can specifically bind to target cells (94). While this method is theoretically similar to DNA-barcoded library of pMHC tetramers, ENTER-seq has several advantages (122). 1) Libraries can be prepared parallelly by DNA synthesis, and thus does not require the synthesis of individual peptides to be loaded on to the pMHC molecules. 2) By leveraging lentivirus biology there is more uniform barcode oligonucleotide loading during the conjugation reaction (123). 3) ENTER-seq can be more sensitive due to the ability of the virus to display more envelope proteins per viral particle unlike pMHC tetramers which are four linked molecules by definition (124).
While tetramers are preferred, they are limited by their use as described in previous sections. There are ways to overcome these challenges and bypassing the use of tetramers when interrogating antigen-specificity. This method requires samples to be sequenced before and after stimulation. The TCRs present before and after can be used as a barcode to link antigen-specificity and to reverse phenotype the targeted cells (95). Using this method Fischer et al., found antigen-reactive clonotypes and they validated reactive TCRs by transgenic T cells using CRISPR/Cas9-mediated OTR. Furthermore using in vitro stimulation, they were able to decipher states of T cell activation/reactivity and associated it with severe or mild disease.
In another method, tumor infiltrating lymphocytes were co-cultured with tandem minigene transfected or peptide pulsed autologous APCs before single-cell RNA-seq. Multiple TCR sequences associated with cells expressing high levels of IFN-γ and IL-2 were identified (96). The identified TCRs were transduced into donor T cells using cloned retroviral vectors, and these transduced cells were able to specifically recognize neoantigens present by autologous APCs (97). This approach is an efficient procedure to isolate neoantigen-specific TCRs for clinical applications and basic translational research.
Unlike with tetramers, single cell sequencing after differential antigen stimulation offers the ability to identify and characterize antigen-reactive T cells by their states of reactivity which is not always possible with multimers. It thereby contributes to understanding the adaptive immune response which will provide a guide to enhance and accelerate development of therapies and vaccines for existing and emerging pathogens.
Another challenge of identifying antigen-specificity is determining which antigens are recognized by a particular TCR. Computational methods of epitope prediction are relatively new (23, 125–127) and it involves training a supervised machine learning model on TCR-antigen pairs to classify and predict antigen specificities of unknown TCR sequences. Unfortunately, the accuracy of the prediction rate on full TCR repertoires are low (23, 125) and models must be trained separately on different epitopes or sets of epitopes. Despite the advances in computationally driven algorithms for better epitope prediction, antigen-specific responses are complicated by cross-reactive TCRs which interfere with precise linking of TCR to biological function (128, 129). Thus, epitope prediction models require an experimental validation method to determine TCR specificity.
Algorithms which cluster TCRs, exploit similarity between TCRs with the aim to identify antigen-specificity. This works with the assumption that TCRs belonging to a specific group should recognize the same pMHC and this is achieved in two ways. 1) comparing global similarities across whole TCRs or CDR3 regions, 2) local similarities focusing on small amino acid motifs. ImmunoMap algorithm is an example of sequence alignment approach for assessing global similarities and relies on PAM10 matrix, large gap penalties and hierarchical clustering to group similar CDR3s (98). TCRdist was developed as a more focused approach to cluster TCRs based on a distance-based metric on both α and β chain of the receptor (99). It is a similarity weighted mismatch distance using alignment with BLOSUM62 substitution matrix to calculate similarity between CDR regions (100). Gap penalties are assigned to CDRs based on conserved short length motifs. Generally, gap penalties are low for CDR1 and CDR2, but increase for CDR3 as it is responsible for binding. Distance between two TCRs is calculated by summing over scores for each CDR on both chains, as well as an additional variable loop (CDR2.5). The CDR3 loop scores on both chains is upweighted in the sum, and TCRs are clustered using TCR distance. TCRdist is the first specialized single-cell TCR similarity measure which combined both α and β chains. However, it must be noted that this metric has not been evaluated on complex repertoires originating from responses from multiple epitopes. CDRdist uses a similar approach but only takes CDR3 sequences into account using local alignment and a substantial gap penalty with BLOSUM45 (100, 101). This combination generates longer matching substrings in alignment allowing for a larger physico-chemical diversity.
An alternative to the scoring approaches described above is to identify short motifs within TCR sequences. The underlying hypothesis of this approach is hot spot interaction, which states that only short stretches of similar amino acid residues are responsible for epitope binding (130–132). Thus, using short stretches of amino acids of length k (k-mers) to evaluate TCR receptor similarity could help reduce noise that is generally associated with using entire sequences. K-mers allows researchers to pinpoint dominant motifs driving TCR-epitope specificity rather than expanded clones. Thomas et al, demonstrates this on murine CD4 T cells following M. tuberculosis immunization (133, 134). Every CDRβ3 sequence was encoded as k-mers of length 3, and each triplet was encoded as a set of Atchley factors that corresponded to its physico-chemical properties (135). The authors generated a code book that reduced the set of representative triplets to describe the complete pooled dataset. This is done by pooling and subsampling triplets from all samples, and grouping them by k means clustering. A single representative triplet is selected to represent each cluster. Each murine repertoire is assigned a triplet vector based on the most similar triplet in the codebook. The repertoire representation is converted into a feature vector that is used for hierarchical clustering and Support Vector Machine (SVM) analysis. Both these methods could distinguish between immunized and non-immunized mice, but time points following immunization were not distinguishable. A major finding from this study is that the results strengthen the importance of TCR repertoire diversity as many private TCRs contribute to T cell responses to the same antigen in generically identical mice.
GLIPH (Grouping of Lymphocyte Interactions by Paratope Hotspots) combines both global similarity metrics and local amino acid motifs to cluster TCRs and predict their HLA restriction (24, 102). One study evaluated the efficiency of GLIPH by using publicly available CDR3 with known specificities, as well their own pMHC tetramer sorted human CD4 and CD8 data (24). Using GLIPH they searched for enriched conserved TCR motifs of length 2, 3 and 4 within TCR multimer repertoires in the CDRβ3 region. The distance metric was calculated by combining global and local TCR sequence similarity, V gene usage, CDR3 length bias, structural peptide antigen contact propensity and other features. GLIPH grouped 94% of the clustered TCRs together with others of the same specificity. In another evaluation with CD4 Mtb-specific T cells from 22 individuals with LTBI, showed that enrichment of motifs can organize TCRs within or across individuals. The authors state that GLIPH can be used independently of knowing epitope specificity. A major drawback of GLIPH was that it lost efficiency and accuracy when analyzing >10,000 TCRs. GLIPH2 was designed to process millions of TCR sequences overcoming this challenge (102).
There is no one single tool that outperforms the rest in its ability to classify TCR repertoire specificity. Biology is not simple and complexities such as cross reactive TCRs that bind to multiple antigens introduce challenges to computational models. TCR binding in itself is not sufficient to elicit a T cell response, and these methods do not take into account binding affinity, stability, co-stimulatory signals that interplay to regulate T cell activation (136). This greatly hampers the intended use of these methods in disease outcome predictions. With the rise in available TCR sequencing data offers the opportunity for researchers to improve methods of epitope prediction and specificity identification. Over the recent years, numerous TCR-antigen specificity predictions tools have been developed, including TCRMatch, NetTCR-2.0, Deep TCR, and TCRAI.
TCRMatch takes advantage of the ever-growing data available to researchers in the Immune Epitope Database (IEDB) (103) that have been experimentally determined to be recognized by T cells and have their specificity information available (137, 138). This tool takes the TCR β chain CDR3 sequences and matches it against the existing sequences in the IEDB to identify specificity of each hit. TCRMatch performs well on independent and small datasets of paired CDR3αβ sequences and is available as a web server tool. However, the performance TCRMatch is affected by the accuracy and limitations in the publicly available data.
NetTCR-2.0 was developed to specifically address the limitations associated with simpler sequenced based models (104). NetTCR-2.0 is utilizes a complex convolutional neural network (CNN) to predict TCR-pMHC interactions based on the amino acid sequences of the peptide and CDR3 region of the TCR chains. CNN is a deep learning method that extracts important features from sequenced data (105). The main advantage of CNNs is that it can learn sequence motifs through objective functions provided to the network. These motifs can be used by the deep learning model to either describe the data or to classify it. The 1D CNN model used in NetTCR-2.0 was found to outperform simpler sequence-based models such as TCRMatch and TCRdist (104). However, the accuracy of the CNN relied on being trained on paired TCRα- and β-chains. Due to the small number of training peptides, the model can only be applied to the limited set of peptides included in the training. NetTCR-2.1 is an extension of NetTCR-2.0 covering more peptides and all CDRs in the binding prediction (139).
DeepTCR is a platform for both supervised and unsupervised deep learning that can be applied at both the individual TCR level and repertoire level (105). The aim of this method is to learn patterns in data that can be used to describe or predict sequence motifs. However, this method also runs into the same problems as NetTCR due to the limitations in the training data.
TCRAI utilizes a similar neural network as DeepTCR, and both methods outperformed TCRdist and NetTCR (106). TCRAI was also more balanced in terms of sensitivity and specificity compared to GLIPH2, NetTCR, TCRdist and DeepTCR. The flexible architecture, ID convolutions, batch normalization of CDR3 sequences and lower dimensional representations for the genes forced TCRAI to learn stronger gene associations making it a stronger performer compared to its rival DeepTCR.
As constantly highlighted in the above methods, a major drawback of deep learning models is that they do not perform as well when tested on different datasets that do not belong to the same source as the training data (140). In order to make machine learning models applicable for real-world applications, they would need to be trained on larger-scale datasets while exploring different feature representations for unseen TCRs and/or epitopes (141).
An individuals’ TCR repertoire is incredibly diverse, however conditions including autoimmune disease, allergy, cancer, and infections can lead to clonal expansion of antigen specific T cells. Using the methods described in this review, the TCR repertoires of clonally expanded cells can be studied in different disease states to better understand antigen specificity. Here, we review recent findings using TCR repertoires to define antigen-specific T cells.
Autoimmune and allergic diseases are defined by a breakdown of tolerance. In the case of autoimmunity, antigen specific T cells bind to antigen presenting cells (APC) presenting self-peptides; whereas in allergy, they recognize APC presenting harmless environmental agents. The recognition of these antigens by T cells leads to downstream inflammatory cascades and results in numerous forms of disease affecting almost every part of the body. T cells are known to play a significant role in these diseases in their recognition of self-antigen followed by downstream activation of B cells and infiltration of tissues leading to immunopathology (142, 143). Many autoimmune diseases are linked to specific HLA genes, and the identification and characterization of clonally expanded T cells, by defining their TCR repertoire, and their respective antigens will enable us to better understand the development and pathogenesis and ultimately treat patients with these diseases (144). The major challenge with this has been the low abundance of these cells in circulation, however the advent of single cell technologies and paired transcriptome/TCR analyses has opened the door to new studies on these populations (145).
Studies have examined TCR repertoires in autoimmune diseases (144, 146) including Crohn’s Disease (147), systemic lupus erythematosus (SLE) (148, 149), rheumatoid arthritis (RA) (150–152), celiac disease (CD) (153, 154), type 1 diabetes (T1D) (155, 156), and Lofgren’s Syndrome (LS) (157). This large body of work shows that antigen specific T cells are critical for disease pathology, expand clonally during disease, can be tracked in tissue and blood, and have broad shifts in disease-specific TCR repertoires (144, 146). More recent studies have begun to comprehensively characterize these cells using paired single cell RNA-Seq and TCR sequencing. One study examined patients with psoriatic arthritis to show predominantly CD8+ clonal expansions in the joint fluid, pointing to a critical role for these cells in disease (158). Another study examined skin inflammatory diseases, finding differences in the transcriptional signatures and clonal expansion of T cells in psoriasis versus atopic dermatitis (159). A third study showed clonal expansion of activated, cytotoxic T cells in cerebrospinal fluid in patients with multiple sclerosis (MS) (160). In another study, TCRs expressing disease associated public β-chain variable region BV9–CDR3β motif were isolated from individuals with ankylosing spondylitis and acute anterior uveitis (161). Using HLA-B*27:05 yeast display peptide libraries, authors identified shared self-peptides and microbial peptides that activated T cells expressing both ankylosing spondylitis and acute anterior uveitis disease-specific TCRs. Their structural analysis revealed cross-reactivity to be rooted in shared binding motifs present in both self-antigens and microbial antigens that engages the BV9–CDR3β TCRs. More studies targeted at antigen-specific cells are needed, which is dependent on the discovery of antigens and epitopes associated with autoimmune diseases. Importantly, studies in mice have shown that TCR affinity plays a role in the activity of autoreactive T cells, making it critical for us to understand the strength of interactions between TCRs and their epitopes (162). Further, there is translational potential in targeting these antigen-specific cells for use in therapy by immune suppression (163, 164).
Allergic diseases encompass a wide range of pathologies, but are mediated by immune responses to environmental agents including aeroallergens and foods. While it is known that T cells play a role in allergy, specifically activated T helper type 2 (Th2) cells, the mechanisms leading to the break of tolerance and development of disease remain unknown. The identification and characterization of allergen-specific T cells, their TCR sequences, and their reactive epitopes is critical for our ability to better treat patients with allergies (145). Studies on various tissue compartments and blood have broadly shown skewing of T cell repertoire usage with allergic disease (165–169). More targeted studies have examined the TCR repertoires and transcriptional profiles of antigen-specific T cells. A study on dog-allergen specific T cells showed heterogeneity in Th2 cells along with less clonality in allergic individuals (170). Alternately, studies on peanut allergy have shown TCR convergence in antigen-specific cells from allergic patients (171, 172). A recent study on eosinophilic esophagitis (EoE) analyzed esophageal, peripheral blood, and duodenal samples, showing clonal expansion of a pathogenic effector Th2 (peTh2) population in these compartments with EoE (173). These studies suggest specific antigens elicit T cell responses in allergy, but require more studies defining and validating T cell epitopes in the allergens. Additionally, the significant heterogeneity in individual responses and in responses to different types of allergens require more studies examining the TCR repertoires of antigen-specific T cells in allergy and other diseases.
Cancer is a disease of the genome- cells that are unable to prevent or repair oncogenic mutations can metastasize and develop into tumors (174). The same mutations that drive oncogenesis generate peptides that can be processed and presented as epitopes on the surface of cancer and antigen presenting cells. Importantly, T cells can recognize said epitopes through their TCRs in order to initiate an anti-tumor immune response (175, 176). This phenomenon led to the development of T cell-based therapies for cancer. Adoptive cell therapy, T cell epitope vaccines, and immune checkpoint blockade therapy all aim to magnify the number of cancer-specific T cells in order to bolster a patient’s immune response. Unlike traditional cancer treatments (i.e. chemotherapy, radiation, etc.), T cell based cancer therapy can be designed to specifically target cancer cells and thus limit off-target toxicities (177). For this reason, much work has been done within the T cell field to identify cancer-specific epitopes (i.e., neoepitopes), neoepitope-specific T cells, and their respective TCRs. Many previous works have discussed current neoepitope prediction tools that harness tumor and blood sequencing to identify tumor-specific epitopes (178–182). In this section, we will highlight work that uses RNA and TCR sequencing to characterize tumor-specific T cells.
Many studies within this either infer or identify antigen specificity of T cells and characterize the phenotype of the predicted tumor-specific cells (183–185). Work done by Li et al. provides an example of this. Their study used single cell RNA and TCR sequencing of 25 melanoma patient tumors in order to investigate the T cell subsets present within tumors at different stages of the disease. It was observed that the T cells present in the tumors of these patients expressed genes associated with cell dysfunction. When the intratumoral cells were characterized in more detail, it was found that they are present in a spectrum of dysfunctionality: cells either expressed genes associated with transitional, early or high dysfunctionality. Importantly, the highly dysfunctional cells were exclusively present in the tumor of the patients and not in the peripheral blood. By including the clonality information gained through TCR sequencing, it was discovered that clone size was significantly increased in the dysfunctional cells that were exclusively present within the tumor. Thus, leading to the conclusion that these clonally expanded T cells that exhibit a highly dysfunctional gene program within the tumor are potentially tumor-specific (186). Other work has also classified tumor-specific cells as dysfunctional. In particular, recent work by Lowery et al. employed single cell RNA sequencing of 10 metastatic human tumors to generate a UMAP of 12 phenotypically distinct clusters of cells. This study also isolated neoantigen-specific T cells by culturing tumor infiltrating lymphocytes (TILs) from the same 10 patients with peptide or tandem-minigene pulsed dendritic cells and sorting for activated cells. Integrating the TCRs of the epitope specific cells onto the UMAP revealed that the majority of the epitope-specific cells congregated within the dysfunctional CD4 and CD8 phenotypic cluster. This led to the identification of a dysfunctional gene signature comprising 283 genes that were associated with the neoepitope-specific dysfunctional cells which, in turn, resulted in the identification of additional neoepitope-specific T cells (187). Lastly, Gros et al. found that PD-1 expression, a gene associated with dysfunction and exhaustion, could be used to narrow down the identification of cancer-specific T cells. PD-1 expression was found in 36% of the TIL isolated from 18 tumors while only 4% of peripheral cells expressed PD-1. Both CD8+PD1+ and CD8+PD1- populations were sorted from patient PBMC and expanded with neoepitope candidates, which showed that CD8+PD1- cells had limited reactivity in comparison to their PD1+ counterparts. Importantly, there was an overlap in the TCR sequences of CD8+PD1+ TILs and circulating cells but not in the PD1- population (188). This suggests that PD1+ cells within the tumor may be antigen-specific and that PD1 expression within the periphery may be circulating clones of the tumor-specific cells. Overall, this body of work provides an example of how antigen-specificity is employed to better understand the critical players within an anti-tumor immune response and develop concrete phenotypes, such as PD1 expression and/or dysfunctionality, of tumor-specificity.
Work has been done to characterize tumor-specific T cells outside of the expression of a dysfunctional phenotype and PD1. In particular, aspects of the TCR repertoire have been examined. For example, Reuben et al. studied the relationship between TCR repertoire overlap in the tumor tissue of 236 early-stage non-small cell lung cancer (NSCLC) patients and their adjacent uninvolved lung. Through TCR sequencing of the CDR3 β region in the tumor and adjacent lung, this group found an overlap of the TCR repertoire present within the tumor and adjacent uninvolved lung. Importantly, relapsed patients had a higher TCR repertoire overlap than non-relapse patients. This indicates that the presence of a larger repertoire of tumor-specific than shared T cell clones (i.e., less overlap) could be used as a prognostic marker for NSCLC patients (184).
Lastly, a few key studies have used the TCR sequence as a molecular barcode alongside single cell RNA sequencing to identify additional genes potentially relevant for tumor-specific cells. Zheng et al. used single cell RNA and TCR sequencing on tumor specific CD4 T cells in human melanoma. They found the TCRs of neoantigen-specific CD4 T cells and used this barcode to determine that these cells had significant expression of the genes HOPX and ADGRG1 and CXCL13 (189). Further, Pauken et al. characterized “tumor-matching” T cells in the peripheral blood (i.e., T cells in the blood expressing tumor-specific TCRs) as cells that expressed a more effector phenotype with a decreased expression of genes GYPC, CCR7, LTB, and FLT3LG (190). Overall, these provide an example of the work that has been done to utilize tumor-specific TCRs to identify additional markers outside the traditional exhausted and dysfunctional phenotype.
In addition to autoimmunity, allergy, and cancer, the TCR sequences of antigen-specific cells within the realm of infectious disease have also been investigated. This section will focus in particular on Mycobacterium tuberculosis (Mtb), Epstein-Barr Virus (EBV), and SARS-CoV-2.
Mtb is an infectious disease predicted to affect about ⅓ of the world’s population. Mtb is characterized by a spectrum of disease stats ranging from a latent, controlled version of infection (LTBI) to an active infection state (ATB) in which a person becomes contagious. A significant limitation in the effective treatment of this disease is the lack of effective diagnostic tools that can accurately identify individuals with LTBI who are at risk of developing ATB (191). For this reason, many groups have utilized TCR and RNA sequencing to study the repertoire of Mtb-specific T cells in order to get a more in depth understanding (192). Single cell TCR and RNA sequencing, calculating the frequencies of different TRBV, TRBD, and TRBJ comparing the tuberculosis pleural effusion (TPE) and blood in ATB patients revealed an increased expression of TRBV4-1 as well as genes related to TCR signaling, T cell activation, glycolysis and differentiation (193). Gideon et al. studied the role of different T cell subsets present within Mtb granulomas, a prominent feature of Mtb infection, in which immune activity can promote bacterial clearance or persistence. Single-cell RNA sequencing of granulomas derived from cynomolgus macaques infected with a low dose of Mtb revealed one particular cell cluster negatively correlated with bacterial burden - the T/NK cell cluster. This cluster (the so-called Type1-Type17 cluster) was enriched for a Th1 and Th17 phenotype, CD4, increased cytotoxic production, cytokines, and heat shock protein. However, these cells within this cluster were also enriched for common CDR3 sequences suggesting limited clonal expansion (194). Lastly, our own work characterizing the phenotype of antigen-specific cells using bulk RNA sequencing, revealed that HLA-DR expression is specific to recently divided Mtb-specific cells in ATB patients (195). However, additional work must be done to connect this phenotype to antigen-specific cells expressing specific TCRs.
The study of antigen-specific TCRs to characterize T cell responses is also applied to EBV and SARS-CoV-2 specific T cells. EBV is a gamma-herpesvirus that infects more than 80% of humans over the age of 20. EBV is known to infect B cells and EBV-specific immune responses are driven by T cells (196). EBV infection has been proven to precede multiple sclerosis onset (MS), therefore TCR sequencing has been used to analyze the TCR repertoire overlap in EBV and MS patients. Published antigen-specific TCRs derived from EBV, cytomegalovirus (CMV), influenza A, and SARS-CoV-2 were quantified in the blood of MS patients and MS-negative controls. This revealed a significantly larger number of EBV-specific TCRs in MS patients compared to healthy controls while none of the other infectious TCRs had a notable trend. Interestingly, MS patients that had undergone treatment that causes sequestration of T cells in the peripheral had an increase in EBV-specific T cells present. This indicates that there are EBV-specific cells creating an immune reaction within the CNS of MS patients that is removed upon treatment. The analysis of the transcriptome of EBV-specific T cells in MS patients and healthy controls determined that the T cells with EBV matching TCRs were enriched for an effector memory phenotype including the expression of PDCD1, CD28, KLRK1/NKG2D, TIGIT, NAM1, and CD244. Thus, this study identified and characterized EBV-specific T cells that may be implicated in MS symptom onset (197). Similar work has been done to study SARS-CoV-2. SARS-CoV-2 is the virus responsible for the COVID-19 pandemic and resulted in hundreds of thousands of deaths. Previous work has clearly shown the importance of T cell responses in COVID-19 related immunity and vaccination (198–201). For example, combining pMHC multimers to identify epitopes and TCR sequencing in a group of individuals with acute COVID-19 showed an enrichment of TRBV27 in epitope-specific T cells. These epitope-specific T cells were unable to produce cytokines and downregulated genes associated with activation, migration, and proliferation. Thus, Gangaev et al. was able to identify SARS-CoV-2 specific T cells and their overall phenotype, which gave insight into the characteristics of the antigen-specific T cells in acute disease (202).
Unconventional T cells are a relatively rare and understudied subset compared to canonical CD4 and CD8 T cells. Unconventional T cells are innate-like lymphocytes that have features of both innate and adaptive immune cells (203). They are not MHC-restricted like conventional T cells and are considered donor-unrestricted as they recognize monomorphic ligands that are shared across diverse human populations unlike MHC-restricted T cells. There are many unconventional T cell subsets, here we focus on mucosal-associated invariant T (MAIT), natural killer T (NKT), and γδT cells.
MAIT cells comprise only 2-5% of T cells in circulation and 10% of CD8+ T cells, but can be found at higher frequencies in tissues such as the liver (204, 205). Most MAIT cells express α-chain rearrangements with the genes TRAV1-2-TRAJ33/20/12 paired with a limited TCRβ-chain repertoire of Vβ2 or Vβ13 (TRBV6 or TRBV20, respectively). These pairings make up the vast majority of MAIT TCR clonotypes in circulation. MAIT cells recognize antigens presented by MR1, a non-polymorphic MHC I-like antigen-presenting molecule. There are other MR1-reactive T cell subsets described elsewhere (206), but classical TRAV1-2+ MAIT cells will be the main focus here.
MAIT cell antigens include those that are riboflavin-based, whereas MR1-restricted T cell antigens comprise a wide array of small molecules, which are reviewed elsewhere (206, 207). Riboflavin pathways are not present in mammals, so MAIT cells can respond to a broad array of microbially derived riboflavin intermediates. These are typically vitamin B metabolites derived from bacteria and yeast, of which the most frequently described and utilized is 5-(2-oxopropylideneamino)-6-D-ribitylaminouracil (5-OP-RU).
A common method to identify MAIT cells is through using MR1/5-OP-RU tetramers, which is sensitive and specific for this cell subset. However, CD4+ MAIT cells have an increased TCR diversity and only roughly one-third of this population binds to MR1/5-OP-RU tetramers (208).
There are two main groups of NKT cells that can be separated based on the expression of specific TCRs and reactivity to different sets of antigens: Type I NKT and Type II NKT. Both NKT subsets recognize the antigen-presenting molecule CD1d, a monomorphic MHC class I-like molecule.
Type I NKT cells, also known as invariant NKT (iNKT) cells, is the more well-studied of the two NKT subsets. iNKT cells constitute roughly 0.1% of T cells in circulation and 1% of liver mononuclear cells in humans (205). iNKT cells have a single α-chain, TRAV10-TRAJ18, that pairs with a limited set of β-chains (209). The β-chain diversity dictates the antigen specificity of iNKT cells (209). iNKT cells recognize lipid antigens such as the prototypic iNKT antigen α-galactosylceramide (α-GalCer). Other iNKT cell antigens include other microbial glycolipids and self-lipids such as phosphatidylinositol (210).
Compared to iNKT cells, Type II NKT cells are more prominent in humans but are less well understood (211, 212). Type II NKT cells have a more diverse TCR repertoire. Type II NKT cells can recognize self, non-self, and non-self and non-microbial antigens (e.g., pollen) presented by CD1d (213). These antigens are largely either sphingolipids and glycerolipids or phospholipids, and include the self-lipid sulfatide identified in mice (214). Type II NKT cell TCRs can be specific to various antigens or promiscuous, i.e. different TCRs can recognize the same antigens (215).
Antigen recognition can be directly measured through the use of tetramers involving CD1d loaded with antigens of interest (216). CD1d tetramers loaded with αGalCer are typically used to identify iNKT cells (217). Sulfatide can also be loaded on CD1d to identify Type II NKT cells. Lipid-loaded CD1d tetramers have been utilized in numerous studies to identify reactive type II NKT cells in multiple diseases, including Type 1 Diabetes, Gaucher’s disease, and cancer (218). However, some antigens will be unable to form stable complexes with CD1d molecules, in which case tetramers cannot be used to identify reactive NKT cells.
γδT cells express γδ TCRs instead of αβ TCRs that the other cell subsets discussed thus far express. γδT cells in total constitute roughly 5% of circulating T cells and up to 16% of T cells in tissues (219). γδT cells recognize viral, bacterial, tumor, and (stress-induced) self-antigens (220), but the antigens they recognize are not fully elucidated (219, 221). γδT cells are primarily segregated into different subsets based on the expression of one of eight δ chains, with Vδ1 and Vδ2 being the two most prominent subsets.
Vδ2 cells are the most prominent γδT cell subset in circulation and can make up 1-10% of T cells in the blood (222, 223). They predominantly express the Vγ9 chain, but can express other γ chains to a lesser extent (224, 225). Vγ9δ2 T cells typically represent roughly 4% of T cells in adult blood (205). They recognize phosphoantigens presented by butyrophilin molecules BTN3A1 and BTN2A1 (204, 205). The canonical antigen used to activate and expand Vγ9δ2 cells is (E)-4-hydroxy-3-methyl-but-2-enyl pyrophosphate (HMBPP) (226) or (E)-4-hydroxy-dimethylallyl pyrophosphate (HDMAPP) (227). These are intermediates of the non-mevalonate pathway and are used somewhat interchangeably. On the other hand, Vγ9-Vδ2+ cells do not respond to phosphoantigens, including HDMAPP (224). However, this subset has been found to clonally expand in response to CMV infection (228).
Vδ1 cells are less common in the blood but can be found more frequently in tissues such as the skin and mucosa. They can express a range of γ chains, and TCRγ chain usage is different at distinct locations within the body (229). Vδ1 cells recognize a variety of antigen-presenting molecules, including CD1b, CD1c, CD1d, and MR1 (221). The antigens recognized by Vδ1 cells are mainly lipids presented by CD1 molecules, though the melanoma-derived peptide MART-1 presented by HLA-A2 has also been found to be associated with Vδ1 response (230).
Other γδT cell subsets have also been studied albeit to a lesser extent. Vδ3, for example, has been shown to recognize MR1 independent of the antigen presented by the molecule (231). Additional Vδ3 antigens include annexin A2, a stress-induced ligand (221). There are even fewer studies on other γδT cell subsets, but there is some evidence of antigen-specific Vδ4 T cells in S. aureus infection and leukemia (232, 233).
Identifying antigen-specific γδT cells can be challenging because the antigens they recognize are not fully elucidated. Some of the difficulties in this field have been presented elsewhere (221), but these include that antigens could be derived from all groups of macromolecules (e.g., lipids, carbohydrates) and could be on the cell surface or in the extracellular space, both of which do not apply to canonical T cells. Similarly, TCR sequencing may not facilitate the identification of antigen-specific γδT cells due to these reasons. However, there has been progress in these efforts, including using tetramers with known antigens to identify reactive γδT cells (234) and unbiased biochemical screens to identify novel γδT cell antigens (235). Many studies have noted expression of specific markers associated with antigen recognition and γδTCR clonal expansion in numerous contexts, such as Mtb and HIV infection, which suggests antigen reactivity (219). Future work will continue contributing to our understanding of γδT cell antigen recognition and identification of antigen-specific cells.
Measuring antigen-specific T cell responses and associated phenotypes helps to deepen our understanding of many different diseases. There is value in examining and understanding the repertoire of antigen-specific T cells, rather than focusing on individual epitopes or antigens. Isolating antigen-specificity allows researchers to better understand T cell biology in disease and ultimately to develop more targeted therapeutics and vaccines. Methods, highlighted in this review are utilized to study antigen-specificities and their associated phenotypes in a variety of contexts. However, there is a gap in these techniques and our knowledge to address issues of multi-epitope-specificity, and also MHC diversity and cross-reactivity. Thus, newer methods are constantly evolving surrounding this need and will continue to develop ushering in the next generation of tools better adapted to analyze complex repertoires and their responses to multiple epitopes.
All authors contributed to the conceptualization, writing, editing and literature review. All authors contributed to the article and approved the submitted version.
This work was supported by the National Institute of Allergy and Infectious Diseases division of the National Institutes of Health under award number U19 AI118626 and 75N93019C00067.
The authors declare that the research was conducted in the absence of any commercial or financial relationships that could be construed as a potential conflict of interest.
All claims expressed in this article are solely those of the authors and do not necessarily represent those of their affiliated organizations, or those of the publisher, the editors and the reviewers. Any product that may be evaluated in this article, or claim that may be made by its manufacturer, is not guaranteed or endorsed by the publisher.
The content is solely the responsibility of the authors and does not necessarily represent the official views of the National Institutes of Health.
1. Eisen HN, Chakraborty AK. Evolving concepts of specificity in immune reactions. Proc Natl Acad Sci USA (2010) 107(52):22373–80. doi: 10.1073/pnas.1012051108
2. Schwartz RS. Diversity of the immune repertoire and immunoregulation. N Engl J Med (2003) 348(11):1017–26. doi: 10.1056/NEJMsa022766
3. Lemke H. Immune response regulation by antigen receptors’ clone-specific nonself parts. Front Immunol (2018) 9:1471. doi: 10.3389/fimmu.2018.01471
4. Burnet FM. A modification of jerne’s theory of antibody production using the concept of clonal selection. CA Cancer J Clin (1976) 26(2):119–21. doi: 10.3322/canjclin.26.2.119
5. van Stipdonk MJ, Lemmens EE, Schoenberger SP. Naïve CTLs require a single brief period of antigenic stimulation for clonal expansion and differentiation. Nat Immunol (2001) 2(5):423–9. doi: 10.1038/87730
6. Adams NM, Grassmann S, Sun JC. Clonal expansion of innate and adaptive lymphocytes. Nat Rev Immunol (2020) 20(11):694–707. doi: 10.1038/s41577-020-0307-4
7. Bonilla FA, Oettgen HC. Adaptive immunity. J Allergy Clin Immunol (2010) 125(2 Suppl 2):S33–40. doi: 10.1016/j.jaci.2009.09.017
8. Kaech SM, Ahmed R. Memory CD8+ T cell differentiation: Initial antigen encounter triggers a developmental program in naïve cells. Nat Immunol (2001) 2(5):415–22. doi: 10.1038/87720
9. Raphael I, Joern RR, Forsthuber TG. Memory CD4+ T cells in immunity and autoimmune diseases. Cells (2020) 9(3):531. doi: 10.3390/cells9030531
10. Lindestam Arlehamn CS, Gerasimova A, Mele F, Henderson R, Swann J, Greenbaum JA, et al. Memory T cells in latent mycobacterium tuberculosis infection are directed against three antigenic islands and largely contained in a CXCR3+CCR6+ Th1 subset. PloS Pathog (2013) 9(1):e1003130. doi: 10.1371/journal.ppat.1003130
11. Arlehamn CL, Seumois G, Gerasimova A, Huang C, Fu Z, Yue X, et al. Transcriptional profile of tuberculosis antigen-specific T cells reveals novel multifunctional features. J Immunol (2014) 193(6):2931–40. doi: 10.4049/jimmunol.1401151
12. Burel JG, Lindestam Arlehamn CS, Khan N, Seumois G, Greenbaum JA, Taplitz R, et al. Transcriptomic analysis of CD4+ T cells reveals novel immune signatures of latent tuberculosis. J Immunol (2018) 200(9):3283–90. doi: 10.4049/jimmunol.1800118
13. Luckheeram RV, Zhou R, Verma AD, Xia B. CD4+T cells: Differentiation and functions. Clin Dev Immunol (2012) 2012:925135. doi: 10.1155/2012/925135
14. Stritesky GL, Jameson SC, Hogquist KA. Selection of self-reactive T cells in the thymus. Annu Rev Immunol (2012) 30:95–114. doi: 10.1146/annurev-immunol-020711-075035
15. Jenkins MK, Khoruts A, Ingulli E, Mueller DL, McSorley SJ, Reinhardt RL, et al. In vivo activation of antigen-specific CD4 T cells. Annu Rev Immunol (2001) 19:23–45. doi: 10.1146/annurev.immunol.19.1.23
16. Sallusto F, Geginat J, Lanzavecchia A. Central memory and effector memory T cell subsets: Function, generation, and maintenance. Annu Rev Immunol (2004) 22:745–63. doi: 10.1146/annurev.immunol.22.012703.104702
17. Adams EJ, Luoma AM. The adaptable major histocompatibility complex (MHC) fold: structure and function of nonclassical and MHC class I-like molecules. Annu Rev Immunol (2013) 31:529–61. doi: 10.1146/annurev-immunol-032712-095912
18. Clements CS, Dunstone MA, Macdonald WA, McCluskey J, Rossjohn J. Specificity on a knife-edge: The alphabeta T cell receptor. Curr Opin Struct Biol (2006) 16(6):787–95. doi: 10.1016/j.sbi.2006.09.004
19. Garboczi DN, Ghosh P, Utz U, Fan QR, Biddison WE, Wiley DC. Structure of the complex between human T-cell receptor, viral peptide and HLA-A2. Nature (1996) 384(6605):134–41. doi: 10.1038/384134a0
20. Rudolph MG, Stanfield RL, Wilson IA. How TCRs bind MHCs, peptides, and coreceptors. Annu Rev Immunol (2006) 24:419–66. doi: 10.1146/annurev.immunol.23.021704.115658
21. Garcia KC, Degano M, Stanfield RL, Brunmark A, Jackson MR, Peterson PA, et al. An alphabeta T cell receptor structure at 2.5 a and its orientation in the TCR-MHC complex. Science (1996) 274(5285):209–19. doi: 10.1126/science.274.5285.209
22. Cole DK, Miles KM, Madura F, Holland CJ, Schauenburg AJA, Godkin AJ, et al. T-Cell receptor (TCR)-peptide specificity overrides affinity-enhancing TCR-major histocompatibility complex interactions. J Biol Chem (2014) 289(2):628–38. doi: 10.1074/jbc.M113.522110
23. Fischer DS, Wu Y, Schubert B, Theis FJ. Predicting antigen specificity of single T cells based on TCR CDR3 regions. Mol Syst Biol (2020) 16(8):e9416. doi: 10.15252/msb.20199416
24. Glanville J, Huang H, Nau A, Hatton O, Wagar LE, Rubelt F, et al. Identifying specificity groups in the T cell receptor repertoire. Nature (2017) 547(7661):94–8. doi: 10.1038/nature22976
25. Sharon E, Sibener LV, Battle A, Fraser HB, Garcia KC, Pritchard JK. Genetic variation in MHC proteins is associated with T cell receptor expression biases. Nat Genet (2016) 48(9):995–1002. doi: 10.1038/ng.3625
26. De Bousser E, Meuris L, Callewaert N, Festjens N. Human T cell glycosylation and implications on immune therapy for cancer. Hum Vaccin Immunother (2020) 16(10):2374–88. doi: 10.1080/21645515.2020.1730658
27. Kuball J, Hauptrock B, Malina V, Antunes E, Voss RH, Wolfl M, et al. Increasing functional avidity of TCR-redirected T cells by removing defined n-glycosylation sites in the TCR constant domain. J Exp Med (2009) 206(2):463–75. doi: 10.1084/jem.20082487
28. Pan YG, Aiamkitsumrit B, Bartolo L, Wang Y, Lavery C, Marc A, et al. Vaccination reshapes the virus-specific T cell repertoire in unexposed adults. Immunity (2021) 54(6):1245–1256.e5. doi: 10.1016/j.immuni.2021.04.023
29. Simpson J, Starke CE, Ortiz AM, Ransier A, Darko S, Douek DC, et al. Multiple modes of antigen exposure induce clonotypically diverse epitope-specific CD8+ T cells across multiple tissues in nonhuman primates. PloS Pathog (2022) 18(7):e1010611. doi: 10.1371/journal.ppat.1010611
30. Altman JD, Moss PAH, Goulder PJR, Barouch DH, McHeyzer-Williams MG, Bell JI, et al. Phenotypic analysis of antigen-specific T lymphocytes. Science (1996) 274(5284):94–6. doi: 10.1126/science.274.5284.94
31. Davis MM, Altman JD, Newell EW. Interrogating the repertoire: Broadening the scope of peptide–MHC multimer analysis. Nat Rev Immunol (2011) 11(8):551–8. doi: 10.1038/nri3020
32. Bakker AH, Schumacher TN. MHC multimer technology: Current status and future prospects. Curr Opin Immunol (2005) 17(4):428–33. doi: 10.1016/j.coi.2005.06.008
33. Batard P, Peterson DA, Devêvre E, Guillaume P, Cerottini JC, Rimoldi D, et al. Dextramers: New generation of fluorescent MHC class i/peptide multimers for visualization of antigen-specific CD8+ T cells. J Immunol Methods (2006) 310(1–2):136–48. doi: 10.1016/j.jim.2006.01.006
34. Cole DK, Pumphrey NJ, Boulter JM, Sami M, Bell JI, Gostick E, et al. Human TCR-binding affinity is governed by MHC class restriction. J Immunol (2007) 178(9):5727–34. doi: 10.4049/jimmunol.178.9.5727
35. Paul S, Lindestam Arlehamn CS, Scriba TJ, Dillon MBC, Oseroff C, Hinz D, et al. Development and validation of a broad scheme for prediction of HLA class II restricted T cell epitopes. J Immunol Methods (2015) 422:28–34. doi: 10.1016/j.jim.2015.03.022
36. Klenerman P, Cerundolo V, Dunbar PR. Tracking T cells with tetramers: new tales from new tools. Nat Rev Immunol (2002) 2(4):263–72. doi: 10.1038/nri777
38. Sims S, Willberg C, Klenerman P. MHC–peptide tetramers for the analysis of antigen-specific T cells. Expert Rev Vaccines (2010) 9(7):765–74. doi: 10.1586/erv.10.66
39. Martin MD, Jensen IJ, Ishizuka AS, Lefebvre M, Shan Q, Xue HH, et al. Bystander responses impact accurate detection of murine and human antigen-specific CD8+ T cells. J Clin Invest (2019) 129(9):3894–908. doi: 10.1172/JCI124443
40. Dolton G, Tungatt K, Lloyd A, Bianchi V, Theaker SM, Trimby A, et al. More tricks with tetramers: a practical guide to staining T cells with peptide-MHC multimers. Immunology (2015) 146(1):11–22. doi: 10.1111/imm.12499
41. Chattopadhyay PK, Melenhorst JJ, Ladell K, Gostick E, Scheinberg P, Barrett AJ, et al. Techniques to improve the direct ex vivo detection of low frequency antigen-specific CD8 + T cells with peptide-major histocompatibility complex class I tetramers: detection of low frequency antigen-specific CD8 + T cells. Cytometry (2008) 73A(11):1001–9. doi: 10.1002/cyto.a.20642
42. Wooldridge L, Lissina A, Cole DK, van den Berg HA, Price DA, Sewell AK. Tricks with tetramers: how to get the most from multimeric peptide-MHC. Immunology (2009) 126(2):147–64. doi: 10.1111/j.1365-2567.2008.02848.x
43. Bentzen AK, Marquard AM, Lyngaa R, Saini SK, Ramskov S, Donia M, et al. Large-Scale detection of antigen-specific T cells using peptide-MHC-I multimers labeled with DNA barcodes. Nat Biotechnol (2016) 34(10):1037–45. doi: 10.1038/nbt.3662
44. Newell EW, Sigal N, Nair N, Kidd BA, Greenberg HB, Davis MM. Combinatorial tetramer staining and mass cytometry analysis facilitate T-cell epitope mapping and characterization. Nat Biotechnol (2013) 31(7):623–9. doi: 10.1038/nbt.2593
45. Mallajosyula V, Ganjavi C, Chakraborty S, McSween AM, Pavlovitch-Bedzyk AJ, Wilhelmy J, et al. CD8 + T cells specific for conserved coronavirus epitopes correlate with milder disease in patients with COVID-19. Sci Immunol (2021) 6(61):eabg5669. doi: 10.1126/sciimmunol.abg5669
46. Martini S, Nielsen M, Peters B, Sette A. The immune epitope database and analysis resource program 2003–2018: reflections and outlook. Immunogenetics (2020) 72(1–2):57–76. doi: 10.1007/s00251-019-01137-6
47. Chang J. MHC multimer: a molecular toolbox for immunologists. MolCells (2021) 44(5):328–34. doi: 10.14348/molcells.2021.0052
48. Sundararaman S, Karulin A, Ansari T, BenHamouda N, Gottwein J, Laxmanan S, et al. High reproducibility of ELISPOT counts from nine different laboratories. Cells (2015) 4(1):21–39. doi: 10.3390/cells4010021
49. Möbs C, Schmidt T. Research techniques made simple: Monitoring of T-cell subsets using the ELISPOT assay. J Invest Dermatol (2016) 136(6):e55–9. doi: 10.1016/j.jid.2016.04.009
50. Czerkinsky CC, Nilsson LÅ, Nygren H, Ouchterlony Ö, Tarkowski A. A solid-phase enzyme-linked immunospot (ELISPOT) assay for enumeration of specific antibody-secreting cells. J Immunol Methods (1983) 65(1–2):109–21. doi: 10.1016/0022-1759(83)90308-3
51. Dillenbeck T, Gelius E, Fohlstedt J, Ahlborg N. Triple cytokine FluoroSpot analysis of human antigen-specific IFN-γ, IL-17A and IL-22 responses. Cells (2014) 3(4):1116–30. doi: 10.3390/cells3041116
52. Jahnmatz P, Bengtsson T, Zuber B, Färnert A, Ahlborg N. An antigen-specific, four-color, b-cell FluoroSpot assay utilizing tagged antigens for detection. J Immunol Methods (2016) 433:23–30. doi: 10.1016/j.jim.2016.02.020
53. Asai T, Storkus WJ, Whiteside TL. Evaluation of the modified ELISPOT assay for gamma interferon production in cancer patients receiving antitumor vaccines. Clin Diagn Lab Immunol (2000) 7(2):145–54. doi: 10.1128/CDLI.7.2.145-154.2000
54. Jung T, Schauer U, Heusser C, Neumann C, Rieger C. Detection of intracellular cytokines by flow cytometry. J Immunol Methods (1993) 159(1–2):197–207. doi: 10.1016/0022-1759(93)90158-4
55. Lovelace P, Maecker HT. Multiparameter intracellular cytokine staining. In: Hawley TS, Hawley RG, editors. Flow cytometry protocols, vol. 699. Totowa, NJ: Humana Press (2011). doi: 10.1007/978-1-61737-950-5_8
56. Darrah PA, Patel DT, De Luca PM, Lindsay RWB, Davey DF, Flynn BJ, et al. Multifunctional TH1 cells define a correlate of vaccine-mediated protection against leishmania major. Nat Med (2007) 13(7):843–50. doi: 10.1038/nm1592
57. Quah BJC, Parish CR. New and improved methods for measuring lymphocyte proliferation in vitro and in vivo using CFSE-like fluorescent dyes. J Immunol Methods (2012) 379(1–2):1–14. doi: 10.1016/j.jim.2012.02.012
58. Wallace PK, Tario JD, Fisher JL, Wallace SS, Ernstoff MS, Muirhead KA. Tracking antigen-driven responses by flow cytometry: Monitoring proliferation by dye dilution: tracking cell proliferation. Cytometry (2008) 73A(11):1019–34. doi: 10.1002/cyto.a.20619
59. Dunsterville C, Stephens AC, Lack G, Turcanu V. The use of dual-Cell-Tracker dye staining for the identification and characterization of peanut-specific T-cell subsets. In: Lympany P, Jones MG, editors. Allergy, vol. 2020 . New York, NY: Springer New York (2019). doi: 10.1007/978-1-4939-9591-2_10
60. Musthaffa Y, Nel HJ, Ramnoruth N, Patel S, Hamilton-Williams EE, Harris M, et al. Optimization of a method to detect autoantigen-specific T-cell responses in type 1 diabetes. Front Immunol (2020) 11:587469. doi: 10.3389/fimmu.2020.587469
61. Tario JD, Conway AN, Muirhead KA, Wallace PK. Monitoring cell proliferation by dye dilution: Considerations for probe selection. In: Hawley TS, Hawley RG, editors. Flow cytometry protocols, vol. 1678 . New York, NY: Springer New York (2018). p. 249–99. doi: 10.1007/978-1-4939-7346-0_12
62. Cook L, Zaunders JJ, Kelleher AD. Comment on “A cytokine-independent approach to identify antigen-specific human germinal center T follicular helper cells and rare antigen-specific CD4 + T cells in blood”. JI. (2016) 197(7):2557–8. doi: 10.4049/jimmunol.1601311
63. Dan JM, Lindestam Arlehamn CS, Weiskopf D, da Silva Antunes R, Havenar-Daughton C, Reiss SM, et al. A cytokine-independent approach to identify antigen-specific human germinal center T follicular helper cells and rare antigen-specific CD4 + T cells in blood. JI. (2016) 197(3):983–93. doi: 10.4049/jimmunol.1600318
64. Bowyer G, Rampling T, Powlson J, Morter R, Wright D, Hill A, et al. Activation-induced markers detect vaccine-specific CD4+ T cell responses not measured by assays conventionally used in clinical trials. Vaccines (2018) 6(3):50. doi: 10.3390/vaccines6030050
65. Barham MS, Whatney WE, Khayumbi J, Ongalo J, Sasser LE, Campbell A, et al. Activation-induced marker expression identifies Mycobacterium tuberculosis –specific CD4 T cells in a cytokine-independent manner in HIV-infected individuals with latent tuberculosis. IH (2020) 4(10):573–84. doi: 10.4049/immunohorizons.2000051
66. Herati RS, Muselman A, Vella L, Bengsch B, Parkhouse K, Del Alcazar D, et al. Successive annual influenza vaccination induces a recurrent oligoclonotypic memory response in circulating T follicular helper cells. Sci Immunol (2017) 2(8):eaag2152. doi: 10.1126/sciimmunol.aag2152
67. Painter MM, Mathew D, Goel RR, Apostolidis SA, Pattekar A, Kuthuru O, et al. Rapid induction of antigen-specific CD4+ T cells is associated with coordinated humoral and cellular immunity to SARS-CoV-2 mRNA vaccination. Immunity (2021) 54(9):2133–2142.e3. doi: 10.1016/j.immuni.2021.08.001
68. Bacher P, Heinrich F, Stervbo U, Nienen M, Vahldieck M, Iwert C, et al. Regulatory T cell specificity directs tolerance versus allergy against aeroantigens in humans. Cell (2016) 167(4):1067–1078.e16. doi: 10.1016/j.cell.2016.09.050
69. Bacher P, Schink C, Teutschbein J, Kniemeyer O, Assenmacher M, Brakhage AA, et al. Antigen-reactive T cell enrichment for direct, high-resolution analysis of the human naive and memory Th cell repertoire. J Immunol (2013) 190(8):3967–76. doi: 10.4049/jimmunol.1202221
70. Schmied S, Gostick E, Price DA, Abken H, Assenmacher M, Richter A. Analysis of the functional WT1-specific T-cell repertoire in healthy donors reveals a discrepancy between CD4(+) and CD8(+) memory formation. Immunology (2015) 145(4):558–69. doi: 10.1111/imm.12472
71. Tesch S, Abdirama D, Grießbach AS, Brand HA, Goerlich N, Humrich JY, et al. Identification and characterization of antigen-specific CD4+ T cells targeting renally expressed antigens in human lupus nephritis with two independent methods. Sci Rep (2020) 10(1):21312. doi: 10.1038/s41598-020-78223-3
72. Pai JA, Satpathy AT. High-throughput and single-cell T cell receptor sequencing technologies. Nat Methods (2021) 18(8):881–92. doi: 10.1038/s41592-021-01201-8
73. Rosati E, Dowds CM, Liaskou E, Henriksen EKK, Karlsen TH, Franke A. Overview of methodologies for T-cell receptor repertoire analysis. BMC Biotechnol (2017) 17(1):61. doi: 10.1186/s12896-017-0379-9
74. Mazzotti L, Gaimari A, Bravaccini S, Maltoni R, Cerchione C, Juan M, et al. T-Cell receptor repertoire sequencing and its applications: Focus on infectious diseases and cancer. IJMS (2022) 23(15):8590. doi: 10.3390/ijms23158590
75. Freeman JD, Warren RL, Webb JR, Nelson BH, Holt RA. Profiling the T-cell receptor beta-chain repertoire by massively parallel sequencing. Genome Res (2009) 19(10):1817–24. doi: 10.1101/gr.092924.109
76. Robins HS, Campregher PV, Srivastava SK, Wacher A, Turtle CJ, Kahsai O, et al. Comprehensive assessment of T-cell receptor β-chain diversity in αβ T cells. Blood (2009) 114(19):4099–107. doi: 10.1182/blood-2009-04-217604
77. McDaniel JR, DeKosky BJ, Tanno H, Ellington AD, Georgiou G. Ultra-high-throughput sequencing of the immune receptor repertoire from millions of lymphocytes. Nat Protoc (2016) 11(3):429–42. doi: 10.1038/nprot.2016.024
78. Watkins TS, Miles JJ. The human T-cell receptor repertoire in health and disease and potential for omics integration. Immunol Cell Biol (2021) 99(2):135–45. doi: 10.1111/imcb.12377
79. Sherwood AM, Desmarais C, Livingston RJ, Andriesen J, Haussler M, Carlson CS, et al. Deep sequencing of the human TCRγ and TCRβ repertoires suggests that TCRβ rearranges after αβ and γδ T cell commitment. Sci Transl Med (2011) 3(90):90ra61. doi: 10.1126/scitranslmed.3002536
80. Eggesbø LM, Risnes LF, Neumann RS, Lundin KEA, Christophersen A, Sollid LM. Single-cell TCR sequencing of gut intraepithelial γδ T cells reveals a vast and diverse repertoire in celiac disease. Mucosal Immunol (2020) 13(2):313–21. doi: 10.1038/s41385-019-0222-9
81. Mose LE, Selitsky SR, Bixby LM, Marron DL, Iglesia MD, Serody JS, et al. Assembly-based inference of b-cell receptor repertoires from short read RNA sequencing data with V’DJer. Bioinformatics (2016) 32(24):3729–34. doi: 10.1093/bioinformatics/btw526
82. Bolotin DA, Poslavsky S, Davydov AN, Frenkel FE, Fanchi L, Zolotareva OI, et al. Antigen receptor repertoire profiling from RNA-seq data. Nat Biotechnol (2017) 35(10):908–11. doi: 10.1038/nbt.3979
83. Chen SY, Liu CJ, Zhang Q, Guo AY. An ultra-sensitive T-cell receptor detection method for TCR-seq and RNA-seq data. Bioinformatics (2020) 36(15):4255–62. doi: 10.1093/bioinformatics/btaa432
84. Mandric I, Rotman J, Yang HT, Strauli N, Montoya DJ, van der Wey W, et al. Profiling immunoglobulin repertoires across multiple human tissues using RNA sequencing. Nat Commun (2020) 11(1):3126. doi: 10.1038/s41467-020-16857-7
85. Upadhyay AA, Kauffman RC, Wolabaugh AN, Cho A, Patel NB, Reiss SM, et al. BALDR: A computational pipeline for paired heavy and light chain immunoglobulin reconstruction in single-cell RNA-seq data. Genome Med (2018) 10(1):20. doi: 10.1186/s13073-018-0528-3
86. Canzar S, Neu KE, Tang Q, Wilson PC, Khan AA. BASIC: BCR assembly from single cells. Bioinformatics (2017) 33(3):425–7. doi: 10.1093/bioinformatics/btw631
87. Rizzetto S, Koppstein DNP, Samir J, Singh M, Reed JH, Cai CH, et al. B-cell receptor reconstruction from single-cell RNA-seq with VDJPuzzle. Bioinformatics (2018) 34(16):2846–7. doi: 10.1093/bioinformatics/bty203
88. Andreani T, Slot LM, Gabillard S, Strübing C, Reimertz C, Yaligara V, et al. Benchmarking computational methods for b-cell receptor reconstruction from single-cell RNA-seq data. NAR Genom Bioinform (2022) 4(3):lqac049. doi: 10.1093/nargab/lqac049
89. Song L, Cohen D, Ouyang Z, Cao Y, Hu X, Liu XS. TRUST4: Immune repertoire reconstruction from bulk and single-cell RNA-seq data. Nat Methods (2021) 18(6):627–30. doi: 10.1038/s41592-021-01142-2
90. Singhania A, Dubelko P, Kuan R, Chronister WD, Muskat K, Das J, et al. CD4+CCR6+ T cells dominate the BCG-induced transcriptional signature. EBioMedicine (2021) 74:103746. doi: 10.1016/j.ebiom.2021.103746
91. Kast F, Klein C, Umaña P, Gros A, Gasser S. Advances in identification and selection of personalized neoantigen/T-cell pairs for autologous adoptive T cell therapies. Oncoimmunology (2021) 10(1):1869389. doi: 10.1080/2162402X.2020.1869389
92. Zhang SQ, Ma KY, Schonnesen AA, Zhang M, He C, Sun E, et al. High-throughput determination of the antigen specificities of T cell receptors in single cells. Nat Biotechnol (2018) 36:1156–9. doi: 10.1101/457069
93. Ng AHC, Peng S, Xu AM, Noh WJ, Guo K, Bethune MT, et al. MATE-seq: Microfluidic antigen-TCR engagement sequencing. Lab Chip (2019) 19(18):3011–21. doi: 10.1039/C9LC00538B
94. Yu B, Shi Q, Belk JA, Yost KE, Parker KR, Li R, et al. Engineered cell entry links receptor biology with single-cell genomics. Cell (2022). 185(26) 4904–20.e22 doi: 10.1016/j.cell.2022.11.016
95. Fischer DS, Ansari M, Wagner KI, Jarosch S, Huang Y, Mayr CH, et al. Single-cell RNA sequencing reveals ex vivo signatures of SARS-CoV-2-reactive T cells through ‘reverse phenotyping’. Nat Commun (2021) 12(1):4515. doi: 10.1038/s41467-021-24730-4
96. Lu YC, Zheng Z, Lowery FJ, Gartner JJ, Prickett TD, Robbins PF, et al. Direct identification of neoantigen-specific TCRs from tumor specimens by high-throughput single-cell sequencing. J Immunother Canc (2021) 9(7):e002595. doi: 10.1136/jitc-2021-002595
97. Lu YC, Zheng Z, Robbins PF, Tran E, Prickett TD, Gartner JJ, et al. An efficient single-cell RNA-seq approach to identify neoantigen-specific T cell receptors. Mol Ther (2018) 26(2):379–89. doi: 10.1016/j.ymthe.2017.10.018
98. Sidhom JW, Bessell CA, Havel JJ, Kosmides A, Chan TA, Schneck JP. ImmunoMap: A bioinformatics tool for T-cell repertoire analysis. Cancer Immunol Res (2018) 6(2):151–62. doi: 10.1158/2326-6066.CIR-17-0114
99. Dash P, Fiore-Gartland AJ, Hertz T, Wang GC, Sharma S, Souquette A, et al. Quantifiable predictive features define epitope-specific T cell receptor repertoires. Nature (2017) 547(7661):89–93. doi: 10.1038/nature22383
100. Henikoff S, Henikoff JG. Amino acid substitution matrices from protein blocks. Proc Natl Acad Sci USA (1992) 89(22):10915–9. doi: 10.1073/pnas.89.22.10915
101. Thakkar N, Bailey-Kellogg C. Balancing sensitivity and specificity in distinguishing TCR groups by CDR sequence similarity. BMC Bioinf (2019) 20(1):241. doi: 10.1186/s12859-019-2864-8
102. Huang H, Wang C, Rubelt F, Scriba TJ, Davis MM. Analyzing the mycobacterium tuberculosis immune response by T-cell receptor clustering with GLIPH2 and genome-wide antigen screening. Nat Biotechnol (2020) 38(10):1194–202. doi: 10.1038/s41587-020-0505-4
103. Chronister WD, Crinklaw A, Mahajan S, Vita R, Koşaloğlu-Yalçın Z, Yan Z, et al. TCRMatch: Predicting T-cell receptor specificity based on sequence similarity to previously characterized receptors. Front Immunol (2021) 12:640725. doi: 10.3389/fimmu.2021.640725
104. Montemurro A, Schuster V, Povlsen HR, Bentzen AK, Jurtz V, Chronister WD, et al. NetTCR-2.0 enables accurate prediction of TCR-peptide binding by using paired TCRα and β sequence data. Commun Biol (2021) 4(1):1060. doi: 10.1038/s42003-021-02610-3
105. Sidhom JW, Larman HB, Pardoll DM, Baras AS. DeepTCR is a deep learning framework for revealing sequence concepts within T-cell repertoires. Nat Commun (2021) 12(1):1605. doi: 10.1038/s41467-021-21879-w
106. Zhang W, Hawkins PG, He J, Gupta NT, Liu J, Choonoo G, et al. A framework for highly multiplexed dextramer mapping and prediction of T cell receptor sequences to antigen specificity. Sci Adv (2021) 7(20):eabf5835. doi: 10.1126/sciadv.abf5835
107. Weiskopf D, Cerpas C, Angelo MA, Bangs DJ, Sidney J, Paul S, et al. Human CD8+ T-cell responses against the 4 dengue virus serotypes are associated with distinct patterns of protein targets. J Infect Dis (2015) 212(11):1743–51. doi: 10.1093/infdis/jiv289
108. Bancroft T, Dillon MBC, da Silva Antunes R, Paul S, Peters B, Crotty S, et al. Th1 versus Th2 T cell polarization by whole-cell and acellular childhood pertussis vaccines persists upon re-immunization in adolescence and adulthood. Cell Immunol (2016) 304–305:35–43. doi: 10.1016/j.cellimm.2016.05.002
109. Hinz D, Seumois G, Gholami AM, Greenbaum JA, Lane J, White B, et al. Lack of allergy to timothy grass pollen is not a passive phenomenon but associated with the allergen-specific modulation of immune reactivity. Clin Exp Allergy (2016) 46(5):705–19. doi: 10.1111/cea.12692
110. Grifoni A, Angelo MA, Lopez B, O’Rourke PH, Sidney J, Cerpas C, et al. Global assessment of dengue virus-specific CD4+ T cell responses in dengue-endemic areas. Front Immunol (2017) 8:1309. doi: 10.3389/fimmu.2017.01309
111. da Silva Antunes R, Paul S, Sidney J, Weiskopf D, Dan JM, Phillips E, et al. Definition of human epitopes recognized in tetanus toxoid and development of an assay strategy to detect ex vivo tetanus CD4+ T cell responses. PloS One (2017) 12(1):e0169086. doi: 10.1371/journal.pone.0169086
112. Paul S, Croft NP, Purcell AW, Tscharke DC, Sette A, Nielsen M, et al. Benchmarking predictions of MHC class I restricted T cell epitopes in a comprehensively studied model system. PloS Comput Biol (2020) 16(5):e1007757. doi: 10.1371/journal.pcbi.1007757
113. Lindestam Arlehamn CS, McKinney DM, Carpenter C, Paul S, Rozot V, Makgotlho E, et al. A quantitative analysis of complexity of human pathogen-specific CD4 T cell responses in healthy m. tuberculosis Infected South Africans PloS Pathog (2016) 12(7):e1005760. doi: 10.1371/journal.ppat.1005760
114. Scriba TJ, Carpenter C, Pro SC, Sidney J, Musvosvi M, Rozot V, et al. Differential recognition of mycobacterium tuberculosis-specific epitopes as a function of tuberculosis disease history. Am J Respir Crit Care Med (2017) 196(6):772–81. doi: 10.1164/rccm.201706-1208OC
115. Kauffman KD, Sallin MA, Hoft SG, Sakai S, Moore R, Wilder-Kofie T, et al. Limited pulmonary mucosal-associated invariant T cell accumulation and activation during mycobacterium tuberculosis infection in rhesus macaques. Infect Immun (2018) 86(12):e00431–18. doi: 10.1128/IAI.00431-18
116. Lindestam Arlehamn CS, Benson B, Kuan R, Dill-McFarland KA, Peterson GJ, Paul S, et al. T-Cell deficiency and hyperinflammatory monocyte responses associate with mycobacterium avium complex lung disease. Front Immunol (2022) 13:1016038. doi: 10.3389/fimmu.2022.1016038
117. Mothé BR, Lindestam Arlehamn CS, Dow C, Dillon MBC, Wiseman RW, Bohn P, et al. The TB-specific CD4(+) T cell immune repertoire in both cynomolgus and rhesus macaques largely overlap with humans. Tuberculosis (Edinb) (2015) 95(6):722–35. doi: 10.1016/j.tube.2015.07.005
118. Sallin MA, Kauffman KD, Riou C, Du Bruyn E, Foreman TW, Sakai S, et al. Host resistance to pulmonary mycobacterium tuberculosis infection requires CD153 expression. Nat Microbiol (2018) 3(11):1198–205. doi: 10.1038/s41564-018-0231-6
119. Patankar YR, Sutiwisesak R, Boyce S, Lai R, Lindestam Arlehamn CS, Sette A, et al. Limited recognition of mycobacterium tuberculosis-infected macrophages by polyclonal CD4 and CD8 T cells from the lungs of infected mice. Mucosal Immunol (2020) 13(1):140–8. doi: 10.1038/s41385-019-0217-6
120. Wood MP, Wood LF, Templeton M, Fisher B, Lippy A, Jones CI, et al. Transient immune activation in BCG-vaccinated infant rhesus macaques is not sufficient to influence oral simian immunodeficiency virus infection. J Infect Dis (2020) 222(1):44–53. doi: 10.1093/infdis/jiz382
121. Yu W, Jiang N, Ebert PJR, Kidd BA, Müller S, Lund PJ, et al. Clonal deletion prunes but does not eliminate self-specific αβ CD8(+) T lymphocytes. Immunity (2015) 42(5):929–41. doi: 10.1016/j.immuni.2015.05.001
122. Bentzen AK, Hadrup SR. Evolution of MHC-based technologies used for detection of antigen-responsive T cells. Cancer Immunol Immunother (2017) 66(5):657–66. doi: 10.1007/s00262-017-1971-5
124. Stano A, Leaman DP, Kim AS, Zhang L, Autin L, Ingale J, et al. Dense array of spikes on HIV-1 virion particles. J Virol (2017) 91(14):e00415–17. doi: 10.1128/JVI.00415-17
125. De Neuter N, Bittremieux W, Beirnaert C, Cuypers B, Mrzic A, Moris P, et al. On the feasibility of mining CD8+ T cell receptor patterns underlying immunogenic peptide recognition. Immunogenetics (2018) 70(3):159–68. doi: 10.1007/s00251-017-1023-5
126. Gielis S, Moris P, Bittremieux W, De Neuter N, Ogunjimi B, Laukens K, et al. Detection of enriched T cell epitope specificity in full T cell receptor sequence repertoires. Front Immunol (2019) 10:2820. doi: 10.3389/fimmu.2019.02820
127. Schneidman-Duhovny D, Khuri N, Dong GQ, Winter MB, Shifrut E, Friedman N, et al. Predicting CD4 T-cell epitopes based on antigen cleavage, MHCII presentation, and TCR recognition. PloS One (2018) 13(11):e0206654. doi: 10.1371/journal.pone.0206654
128. Mason D. A very high level of crossreactivity is an essential feature of the T-cell receptor. Immunol Today (1998) 19(9):395–404. doi: 10.1016/S0167-5699(98)01299-7
129. Sewell AK. Why must T cells be cross-reactive? Nat Rev Immunol (2012) 12(9):669–77. doi: 10.1038/nri3279
130. Clackson T, Wells JA. A hot spot of binding energy in a hormone-receptor interface. Science (1995) 267(5196):383–6. doi: 10.1126/science.7529940
131. Marks DS, Hopf TA, Sander C. Protein structure prediction from sequence variation. Nat Biotechnol (2012) 30(11):1072–80. doi: 10.1038/nbt.2419
132. Ovchinnikov S, Kamisetty H, Baker D. Robust and accurate prediction of residue-residue interactions across protein interfaces using evolutionary information. Elife (2014) 3:e02030. doi: 10.7554/eLife.02030.014
133. Atchley WR, Zhao J, Fernandes AD, Drüke T. Solving the protein sequence metric problem. Proc Natl Acad Sci USA (2005) 102(18):6395–400. doi: 10.1073/pnas.0408677102
134. Thomas N, Best K, Cinelli M, Reich-Zeliger S, Gal H, Shifrut E, et al. Tracking global changes induced in the CD4 T-cell receptor repertoire by immunization with a complex antigen using short stretches of CDR3 protein sequence. Bioinformatics (2014) 30(22):3181–8. doi: 10.1093/bioinformatics/btu523
135. Atchley WR, Fitch WM. A natural classification of the basic helix-loop-helix class of transcription factors. Proc Natl Acad Sci USA (1997) 94(10):5172–6. doi: 10.1073/pnas.94.10.5172
136. Gálvez J, Gálvez JJ, García-Peñarrubia P. Is TCR/pMHC affinity a good estimate of the T-cell response? an answer based on predictions from 12 phenotypic models. Front Immunol (2019) 10:349. doi: 10.3389/fimmu.2019.00349
137. Mahajan S, Vita R, Shackelford D, Lane J, Schulten V, Zarebski L, et al. Epitope specific antibodies and T cell receptors in the immune epitope database. Front Immunol (2018) 9:2688. doi: 10.3389/fimmu.2018.02688
138. Vita R, Mahajan S, Overton JA, Dhanda SK, Martini S, Cantrell JR, et al. The immune epitope database (IEDB): 2018 update. Nucleic Acids Res (2019) 47(D1):D339–43. doi: 10.1093/nar/gky1006
139. Montemurro A, Jessen LE, Nielsen M. NetTCR-2.1: lessons and guidance on how to develop models for TCR specificity predictions. Front Immunol (2022) 13:1055151. doi: 10.3389/fimmu.2022.1055151
140. Grazioli F, Mösch A, Machart P, Li K, Alqassem I, O’Donnell TJ, et al. On TCR binding predictors failing to generalize to unseen peptides. Front Immunol (2022) 13:1014256. doi: 10.3389/fimmu.2022.1014256
141. Weber A, Born J, Rodriguez Martínez M. TITAN: T-cell receptor specificity prediction with bimodal attention networks. Bioinformatics (2021) 37(Suppl_1):i237–44. doi: 10.1093/bioinformatics/btab294
142. Khan U, Ghazanfar H. T Lymphocytes and autoimmunity. Int Rev Cell Mol Biol (2018) 341:125–68. doi: 10.1016/bs.ircmb.2018.05.008
143. Platsoucas CD, Oleszak EL. Human autoimmune diseases are specific antigen-driven T-cell diseases: Identification of the antigens. Immunol Res (2007) 38(1–3):359–72. doi: 10.1007/s12026-007-0044-9
144. Mitchell AM, Michels AW. T Cell receptor sequencing in autoimmunity. J Life Sci (Westlake Village) (2020) 2(4):38–58. doi: 10.36069/JoLS/20201203
145. Lewis SA, Peters B. T-Cell epitope discovery and single-cell technologies to advance food allergy research. J Allergy Clin Immunol (2022) 151:S0091–6749(22)01483-X. doi: 10.1016/j.jaci.2022.10.025
146. Foth S, Völkel S, Bauersachs D, Zemlin M, Skevaki C. T Cell repertoire during ontogeny and characteristics in inflammatory disorders in adults and childhood. Front Immunol (2020) 11:611573. doi: 10.3389/fimmu.2020.611573
147. Chapman CG, Yamaguchi R, Tamura K, Weidner J, Imoto S, Kwon J, et al. Characterization of T-cell receptor repertoire in inflamed tissues of patients with crohn’s disease through deep sequencing. Inflammation Bowel Dis (2016) 22(6):1275–85. doi: 10.1097/MIB.0000000000000752
148. Ye X, Wang Z, Ye Q, Zhang J, Huang P, Song J, et al. High-throughput sequencing-based analysis of T cell repertoire in lupus nephritis. Front Immunol (2020) 11:1618. doi: 10.3389/fimmu.2020.01618
149. Liu X, Zhang W, Zhao M, Fu L, Liu L, Wu J, et al. T Cell receptor β repertoires as novel diagnostic markers for systemic lupus erythematosus and rheumatoid arthritis. Ann Rheum Dis (2019) 78(8):1070–8. doi: 10.1136/annrheumdis-2019-215442
150. Ishigaki K, Shoda H, Kochi Y, Yasui T, Kadono Y, Tanaka S, et al. Quantitative and qualitative characterization of expanded CD4+ T cell clones in rheumatoid arthritis patients. Sci Rep (2015) 5:12937. doi: 10.1038/srep12937
151. Sakurai K, Ishigaki K, Shoda H, Nagafuchi Y, Tsuchida Y, Sumitomo S, et al. HLA-DRB1 shared epitope alleles and disease activity are correlated with reduced T cell receptor repertoire diversity in CD4+ T cells in rheumatoid arthritis. J Rheumatol (2018) 45(7):905–14. doi: 10.3899/jrheum.170909
152. Jiang X, Wang S, Zhou C, Wu J, Jiao Y, Lin L, et al. Comprehensive TCR repertoire analysis of CD4+ T-cell subsets in rheumatoid arthritis. J Autoimmun (2020) 109:102432. doi: 10.1016/j.jaut.2020.102432
153. Yohannes DA, Freitag TL, de Kauwe A, Kaukinen K, Kurppa K, Wacklin P, et al. Deep sequencing of blood and gut T-cell receptor β-chains reveals gluten-induced immune signatures in celiac disease. Sci Rep (2017) 7(1):17977. doi: 10.1038/s41598-017-18137-9
154. Risnes LF, Christophersen A, Dahal-Koirala S, Neumann RS, Sandve GK, Sarna VK, et al. Disease-driving CD4+ T cell clonotypes persist for decades in celiac disease. J Clin Invest (2018) 128(6):2642–50. doi: 10.1172/JCI98819
155. Michels AW, Landry LG, McDaniel KA, Yu L, Campbell-Thompson M, Kwok WW, et al. Islet-derived CD4 T cells targeting proinsulin in human autoimmune diabetes. Diabetes (2017) 66(3):722–34. doi: 10.2337/db16-1025
156. Ogura H, Preston-Hurlburt P, Perdigoto AL, Amodio M, Krishnaswamy S, Clark P, et al. Identification and analysis of islet antigen-specific CD8+ T cells with T cell libraries. J Immunol (2018) 201(6):1662–70. doi: 10.4049/jimmunol.1800267
157. Mitchell AM, Kaiser Y, Falta MT, Munson DJ, Landry LG, Eklund A, et al. Shared αβ TCR usage in lungs of sarcoidosis patients with löfgren’s syndrome. J Immunol (2017) 199(7):2279–90. doi: 10.4049/jimmunol.1700570
158. Penkava F, Velasco-Herrera MDC, Young MD, Yager N, Nwosu LN, Pratt AG, et al. Single-cell sequencing reveals clonal expansions of pro-inflammatory synovial CD8 T cells expressing tissue-homing receptors in psoriatic arthritis. Nat Commun (2020) 11(1):4767. doi: 10.1038/s41467-020-18513-6
159. Zhang B, Roesner LM, Traidl S, Koeken VACM, Xu CJ, Werfel T, et al. Single-cell profiles reveal distinctive immune response in atopic dermatitis in contrast to psoriasis. Allergy (2022) 78(2):439–53. doi: 10.1111/all.15486
160. Pappalardo JL, Zhang L, Pecsok MK, Perlman K, Zografou C, Raddassi K, et al. Transcriptomic and clonal characterization of T cells in the human central nervous system. Sci Immunol (2020) 5(51):eabb8786. doi: 10.1126/sciimmunol.abb8786
161. Yang X, Garner LI, Zvyagin IV, Paley MA, Komech EA, Jude KM, et al. Autoimmunity-associated T cell receptors recognize HLA-B*27-bound peptides. Nature. (2022) 612(7941):771–7. doi: 10.1038/s41586-022-05501-7
162. Yi J, Miller AT, Archambault AS, Jones AJ, Bradstreet TR, Bandla S, et al. Antigen-specific depletion of CD4+ T cells by CAR T cells reveals distinct roles of higher- and lower-affinity TCRs during autoimmunity. Sci Immunol (2022) 7(76):eabo0777. doi: 10.1126/sciimmunol.abo0777
163. Benne N, Ter Braake D, Stoppelenburg AJ, Broere F. Nanoparticles for inducing antigen-specific T cell tolerance in autoimmune diseases. Front Immunol (2022) 13:864403. doi: 10.3389/fimmu.2022.864403
164. Mikami N, Kawakami R, Sakaguchi S. New treg cell-based therapies of autoimmune diseases: Towards antigen-specific immune suppression. Curr Opin Immunol (2020) 67:36–41. doi: 10.1016/j.coi.2020.07.004
165. Kircher MF, Haeusler T, Nickel R, Lamb JR, Renz H, Beyer K. Vbeta18.1(+) and V(alpha)2.3(+) T-cell subsets are associated with house dust mite allergy in human subjects. J Allergy Clin Immunol (2002) 109(3):517–23. doi: 10.1067/mai.2002.121945
166. Roesner LM, Farag AK, Pospich R, Traidl S, Werfel T. T-Cell receptor sequencing specifies psoriasis as a systemic and atopic dermatitis as a skin-focused, allergen-driven disease. Allergy (2022) 77(9):2737–47. doi: 10.1111/all.15272
167. Sade K, Kivity S, Levy A, Fireman E. The effect of specific immunotherapy on T-cell receptor repertoire in patients with allergy to house-dust mite. Allergy (2003) 58(5):430–4. doi: 10.1034/j.1398-9995.2003.00055.x
168. Umibe T, Kita Y, Nakao A, Nakajima H, Fukuda T, Yoshida S, et al. Clonal expansion of T cells infiltrating in the airways of non-atopic asthmatics. Clin Exp Immunol (2000) 119(3):390–7. doi: 10.1046/j.1365-2249.2000.01148.x173
169. Wahlström J, Gigliotti D, Roquet A, Wigzell H, Eklund A, Grunewald J. T Cell receptor vbeta expression in patients with allergic asthma before and after repeated low-dose allergen inhalation. Clin Immunol (2001) 100(1):31–9. doi: 10.1006/clim.2001.5045
170. Vandamme C, Rytkönen-Nissinen M, Lönnberg T, Randell J, Harvima RJ, Kinnunen T, et al. Single-cell characterization of dog allergen-specific T cells reveals TH2 heterogeneity in allergic individuals. J Allergy Clin Immunol (2022) 149(5):1732–1743.e15. doi: 10.1016/j.jaci.2021.11.018
171. Monian B, Tu AA, Ruiter B, Morgan DM, Petrossian PM, Smith NP, et al. Peanut oral immunotherapy differentially suppresses clonally distinct subsets of T helper cells. J Clin Invest (2022) 132(2):e150634. doi: 10.1172/JCI150634
172. Smith NP, Ruiter B, Virkud YV, Tu AA, Monian B, Moon JJ, et al. Identification of antigen-specific TCR sequences based on biological and statistical enrichment in unselected individuals. JCI Insight (2021) 6(13):e140028,140028. doi: 10.1172/jci.insight.140028
173. Morgan DM, Ruiter B, Smith NP, Tu AA, Monian B, Stone BE, et al. Clonally expanded, GPR15-expressing pathogenic effector TH2 cells are associated with eosinophilic esophagitis. Sci Immunol (2021) 6(62):eabi5586. doi: 10.1126/sciimmunol.abi5586
174. Greenman C, Stephens P, Smith R, Dalgliesh GL, Hunter C, Bignell G, et al. Patterns of somatic mutation in human cancer genomes. Nature (2007) 446(7132):153–8. doi: 10.1038/nature05610
175. Koşaloğlu-Yalçın Z, Lanka M, Frentzen A, Logandha Ramamoorthy Premlal A, Sidney J, Vaughan K, et al. Predicting T cell recognition of MHC class I restricted neoepitopes. Oncoimmunology (2018) 7(11):e1492508. doi: 10.1080/2162402X.2018.1492508
176. Sahin U, Türeci Ö. Personalized vaccines for cancer immunotherapy. Science. (2018) 359(6382):1355–60. doi: 10.1126/science.aar7112
177. Blankenstein T, Leisegang M, Uckert W, Schreiber H. Targeting cancer-specific mutations by T cell receptor gene therapy. Curr Opin Immunol (2015) 33:112–9. doi: 10.1016/j.coi.2015.02.005
178. Duan F, Duitama J, Al Seesi S, Ayres CM, Corcelli SA, Pawashe AP, et al. Genomic and bioinformatic profiling of mutational neoepitopes reveals new rules to predict anticancer immunogenicity. J Exp Med (2014) 211(11):2231–48. doi: 10.1084/jem.20141308
179. Hundal J, Carreno BM, Petti AA, Linette GP, Griffith OL, Mardis ER, et al. pVAC-seq: a genome-guided in silico approach to identifying tumor neoantigens. Genome Med (2016) 8(1):11. doi: 10.1186/s13073-016-0264-5
180. Saini SK, Rekers N, Hadrup SR. Novel tools to assist neoepitope targeting in personalized cancer immunotherapy. Ann Oncol (2017) 28(suppl_12):xii3–10. doi: 10.1093/annonc/mdx544
181. Schaap-Johansen AL, Vujović M, Borch A, Hadrup SR, Marcatili P. T Cell epitope prediction and its application to immunotherapy. Front Immunol (2021) 12:712488. doi: 10.3389/fimmu.2021.712488
182. Schenck RO, Lakatos E, Gatenbee C, Graham TA, Anderson ARA. NeoPredPipe: high-throughput neoantigen prediction and recognition potential pipeline. BMC Bioinf (2019) 20(1):264. doi: 10.1186/s12859-019-2876-4
183. Pasetto A, Lu YC. Single-cell TCR and transcriptome analysis: An indispensable tool for studying T-cell biology and cancer immunotherapy. Front Immunol (2021) 12:689091. doi: 10.3389/fimmu.2021.689091
184. Reuben A, Zhang J, Chiou SH, Gittelman RM, Li J, Lee WC, et al. Comprehensive T cell repertoire characterization of non-small cell lung cancer. Nat Commun (2020) 11(1):603. doi: 10.1038/s41467-019-14273-0
185. Zhang H, Liu L, Zhang J, Chen J, Ye J, Shukla S, et al. Investigation of antigen-specific T-cell receptor clusters in human cancers. Clin Cancer Res (2020) 26(6):1359–71. doi: 10.1158/1078-0432.CCR-19-3249
186. Li H, van der Leun AM, Yofe I, Lubling Y, Gelbard-Solodkin D, van Akkooi ACJ, et al. Dysfunctional CD8 T cells form a proliferative, dynamically regulated compartment within human melanoma. Cell (2019) 176(4):775–789.e18. doi: 10.1016/j.cell.2018.11.043
187. Lowery FJ, Krishna S, Yossef R, Parikh NB, Chatani PD, Zacharakis N, et al. Molecular signatures of antitumor neoantigen-reactive T cells from metastatic human cancers. Science (2022) 375(6583):877–84. doi: 10.1126/science.abl5447
188. Gros A, Parkhurst MR, Tran E, Pasetto A, Robbins PF, Ilyas S, et al. Prospective identification of neoantigen-specific lymphocytes in the peripheral blood of melanoma patients. Nat Med (2016) 22(4):433–8. doi: 10.1038/nm.4051
189. Zheng C, Fass JN, Shih YP, Gunderson AJ, Sanjuan Silva N, Huang H, et al. Transcriptomic profiles of neoantigen-reactive T cells in human gastrointestinal cancers. Cancer Cell (2022) 40(4):410–423.e7. doi: 10.1016/j.ccell.2022.03.005
190. Pauken KE, Shahid O, Lagattuta KA, Mahuron KM, Luber JM, Lowe MM, et al. Single-cell analyses identify circulating anti-tumor CD8 T cells and markers for their enrichment. J Exp Med (2021) 218(4):e20200920. doi: 10.1084/jem.20200920
191. Burel JG, Singhania A, Dubelko P, Muller J, Tanner R, Parizotto E, et al. Distinct blood transcriptomic signature of treatment in latent tuberculosis infected individuals at risk of developing active disease. Tuberculosis (Edinb) (2021) 131:102127. doi: 10.1016/j.tube.2021.102127
192. Burel JG, Babor M, Pomaznoy M, Lindestam Arlehamn CS, Khan N, Sette A, et al. Host transcriptomics as a tool to identify diagnostic and mechanistic immune signatures of tuberculosis. Front Immunol (2019) 10:221. doi: 10.3389/fimmu.2019.00221
193. Shao MM, Yi FS, Huang ZY, Peng P, Wu FY, Shi HZ, et al. T Cell receptor repertoire analysis reveals signatures of T cell responses to human mycobacterium tuberculosis. Front Microbiol (2022) 13:829694. doi: 10.3389/fmicb.2022.829694
194. Gideon HP, Hughes TK, Tzouanas CN, Wadsworth MH, Tu AA, Gierahn TM, et al. Multimodal profiling of lung granulomas in macaques reveals cellular correlates of tuberculosis control. Immunity (2022) 55(5):827–846.e10. doi: 10.1016/j.immuni.2022.04.004
195. Tippalagama R, Singhania A, Dubelko P, Lindestam Arlehamn CS, Crinklaw A, Pomaznoy M, et al. HLA-DR marks recently divided antigen-specific effector CD4 T cells in active tuberculosis patients. J Immunol (2021) 207(2):523–33. doi: 10.4049/jimmunol.2100011
196. Lammoglia Cobo MF, Welters C, Rosenberger L, Leisegang M, Dietze K, Pircher C, et al. Rapid single-cell identification of Epstein-Barr virus-specific T-cell receptors for cellular therapy. Cytotherapy (2022) 24(8):818–26. doi: 10.1016/j.jcyt.2022.03.005
197. Schneider-Hohendorf T, Gerdes LA, Pignolet B, Gittelman R, Ostkamp P, Rubelt F, et al. Broader Epstein-Barr virus-specific T cell receptor repertoire in patients with multiple sclerosis. J Exp Med (2022) 219(11):e20220650. doi: 10.1084/jem.20220650
198. Grifoni A, Weiskopf D, Ramirez SI, Mateus J, Dan JM, Moderbacher CR, et al. Targets of T cell responses to SARS-CoV-2 coronavirus in humans with COVID-19 disease and unexposed individuals. Cell (2020) 181(7):1489–1501.e15. doi: 10.1016/j.cell.2020.05.015
199. Mateus J, Grifoni A, Tarke A, Sidney J, Ramirez SI, Dan JM, et al. Selective and cross-reactive SARS-CoV-2 T cell epitopes in unexposed humans. Science. (2020) 370(6512):89–94. doi: 10.1126/science.abd3871
200. Sette A, Crotty S. Adaptive immunity to SARS-CoV-2 and COVID-19. Cell. (2021) 184(4):861–80. doi: 10.1016/j.cell.2021.01.007
201. Tarke A, Sidney J, Kidd CK, Dan JM, Ramirez SI, Yu ED, et al. Comprehensive analysis of T cell immunodominance and immunoprevalence of SARS-CoV-2 epitopes in COVID-19 cases. Cell Rep Med (2021) 2(2):100204. doi: 10.1016/j.xcrm.2021.100204
202. Gangaev A, Ketelaars SLC, Isaeva OI, Patiwael S, Dopler A, Hoefakker K, et al. Identification and characterization of a SARS-CoV-2 specific CD8+ T cell response with immunodominant features. Nat Commun (2021) 12(1):2593. doi: 10.1038/s41467-021-22811-y
203. Mayassi T, Barreiro LB, Rossjohn J, Jabri B. A multilayered immune system through the lens of unconventional T cells. Nature (2021) 595:501–10. doi: 10.1038/s41586-021-03578-0
204. Godfrey DI, Uldrich AP, McCluskey J, Rossjohn J, Moody DB. The burgeoning family of unconventional T cells. Nat Immunol (2015) 16(11):1114–23. doi: 10.1038/ni.3298
205. Pellicci DG, Koay HF, Berzins SP. Thymic development of unconventional T cells: How NKT cells, MAIT cells and γδ T cells emerge. Nat Rev Immunol (2020) 20(12):756–70. doi: 10.1038/s41577-020-0345-y
206. Souter MNT, Eckle SBG. Biased MAIT TCR usage poised for limited antigen diversity? Front Immunol (2020) 11:1845. doi: 10.3389/fimmu.2020.01845
207. Corbett AJ, Awad W, Wang H, Chen Z. Antigen recognition by MR1-reactive T cells; MAIT cells, metabolites, and remaining mysteries. Front Immunol (2020) 11:1961. doi: 10.3389/fimmu.2020.01961
208. Gherardin NA, Souter MNT, Koay HF, Mangas KM, Seemann T, Stinear TP, et al. Human blood MAIT cell subsets defined using MR1 tetramers. Immunol Cell Biol (2018) 96(5):507–25. doi: 10.1111/imcb.12021
209. Cameron G, Pellicci DG, Uldrich AP, Besra GS, Illarionov P, Williams SJ, et al. Antigen specificity of type I NKT cells is governed by TCR β-chain diversity. J Immunol (2015) 195(10):4604–14. doi: 10.4049/jimmunol.1501222
210. Mallevaey T, Selvanantham T. Strategy of lipid recognition by invariant natural killer T cells: ‘One for all and all for one’. Immunology (2012) 136:273–82. doi: 10.1111/j.1365-2567.2012.03580.x
211. Exley MA, Tahir SMA, Cheng O, Shaulov A, Joyce R, Avigan D, et al. Cutting edge: a major fraction of human bone marrow lymphocytes are Th2-like CD1d-reactive T cells that can suppress mixed lymphocyte responses. J Immunol (2001) 167(10):5531–4. doi: 10.4049/jimmunol.167.10.5531
212. Kumar V, Delovitch TL. Different subsets of natural killer T cells may vary in their roles in health and disease. Immunology (2014) 142(3):321–36. doi: 10.1111/imm.12247
213. Dasgupta S, Kumar V. Type II NKT cells: A distinct CD1d-restricted immune regulatory NKT cell subset. Immunogenetics (2016) 68(8):665–76. doi: 10.1007/s00251-016-0930-1
214. Jahng A, Maricic I, Aguilera C, Cardell S, Halder RC, Kumar V. Prevention of autoimmunity by targeting a distinct, noninvariant CD1d-reactive T cell population reactive to sulfatide. J Exp Med (2004) 199(7):947–57. doi: 10.1084/jem.20031389
215. Marrero I, Ware R, Kumar V. Type II NKT cells in inflammation, autoimmunity, microbial immunity, and cancer. Front Immunol (2015) 6:316. doi: 10.3389/fimmu.2015.00316
216. Zeissig S, Olszak T, Melum E, Blumberg RS. Analyzing antigen recognition by natural killer T cells. Methods Mol Biol (2013) 960:557–72. doi: 10.1007/978-1-62703-218-6_41
217. Gapin L. INKT cell autoreactivity: What is ‘self’ and how is it recognized? Nat Rev Immunol (2010) 10:272–77. doi: 10.1038/nri2743
218. Singh AK, Tripathi P. Type II NKT cells: An elusive population with immunoregulatory properties. Front Immunol (2018) 9:1969. doi: 10.3389/fimmu.2018.01969
219. Fichtner AS, Ravens S, Prinz I. Human γδ TCR repertoires in health and disease. Cells (2020) 9:800. doi: 10.3390/cells9040800
220. Champagne E. γδ T cell receptor ligands and modes of antigen recognition. Archivum Immunologiae Therapiae Experimentalis (2011) 59:117–37. doi: 10.1007/s00005-011-0118-1
221. Deseke M, Prinz I. Ligand recognition by the γδ TCR and discrimination between homeostasis and stress conditions. Cell Mol Immunol (2020) 17:914–24. doi: 10.1038/s41423-020-0503-y
222. Morita CT, Mariuzza RA, Brenner MB. Antigen recognition by human γδ T cells: Pattern recognition by the adaptive immune system. Springer Semin Immunopathology (2000) 22(3):191–217. doi: 10.1007/s002810000042
223. Morita CT, Jin C, Sarikonda G, Wang H. Nonpeptide antigens, presentation mechanisms, and immunological memory of human Vγ2Vδ2 T cells: Discriminating friend from foe through the recognition of prenyl pyrophosphate antigens. Immunol Rev (2007) 215:59–76. doi: 10.1111/j.1600-065X.2006.00479.x
224. Davey MS, Willcox CR, Hunter S, Kasatskaya SA, Remmerswaal EBM, Salim M, et al. The human Vδ2+ T-cell compartment comprises distinct innate-like Vγ9+ and adaptive Vγ9- subsets. Nat Commun (2018) 9(1):1760. doi: 10.1038/s41467-018-04076-0
225. Vermijlen D, Brouwer M, Donner C, Liesnard C, Tackoen M, Van Rysselberge M, et al. Human cytomegalovirus elicits fetal γδ T cell responses in utero. J Exp Med (2010) 207(4):807–21. doi: 10.1084/jem.20090348
226. Hintz M, Reichenberg A, Altincicek B, Bahr U, Gschwind RM, Kollas AK, et al. Identification of (E)-4-hydroxy-3-methyl-but-2-enyl pyrophosphate as a major activator for human γδ T cells in escherichia coli. FEBS Lett (2001) 509(2):317–22. doi: 10.1016/s0014-5793(01)03191-x
227. Moulin M, Alguacil J, Gu S, Mehtougui A, Adams EJ, Peyrottes S, et al. Vγ9Vδ2 T cell activation by strongly agonistic nucleotidic phosphoantigens. Cell Mol Life Sci (2017) 74(23):4353–67. doi: 10.1007/s00018-017-2583-0
228. Ravens S, Schultze-Florey C, Raha S, Sandrock I, Drenker M, Oberdörfer L, et al. Human γδ T cells are quickly reconstituted after stem-cell transplantation and show adaptive clonal expansion in response to viral infection. Nat Immunol (2017) 18(4):393–401. doi: 10.1038/ni.3686
229. Zheng R, Yang Q. The role of the γδ T cell in allergic diseases. J Immunol Res (2014) 2014:963484. doi: 10.1155/2014/963484
230. Benveniste PM, Roy S, Nakatsugawa M, Chen ELY, Nguyen L, Millar DG, et al. Generation and molecular recognition of melanoma-associated antigen-specific human T cells. Sci Immunol (2018) 3(30):eaav4036. doi: 10.1126/sciimmunol.aav4036
231. Rice MT, von Borstel A, Chevour P, Awad W, Howson LJ, Littler DR, et al. Recognition of the antigen-presenting molecule MR1 by a Vδ3+ γδ T cell receptor. Proc Natl Acad Sci USA (2021) 118(49):e2110288118. doi: 10.1073/pnas.2110288118
232. Marchitto MC, Dillen CA, Liu H, Miller RJ, Archer NK, Ortines RV, et al. Clonal Vγ6+Vδ4+ T cells promote IL-17–mediated immunity against staphylococcus aureus skin infection. Proc Natl Acad Sci USA (2019) 166(22):10917–26. doi: 10.1073/pnas.1818256116
233. Xu L, Weng J, Huang X, Zeng C, Chen S, Geng S, et al. Persistent donor derived Vδ4 T cell clones may improve survival for recurrent T cell acute lymphoblastic leukemia after HSCT and DLI. Oncotarget (2016) 7(28):42943–52. doi: 10.18632/oncotarget.10260
234. Reijneveld JF, Ocampo TA, Shahine A, Gully BS, Vantourout P, Hayday AC, et al. Human γδ T cells recognize CD1b by two distinct mechanisms. Proc Natl Acad Sci USA (2020) 117(37):22944–52. doi: 10.1073/pnas.2010545117
Keywords: T cell, antigen-specificity, TCR, sequencing, adaptive immunity
Citation: Tippalagama R, Chihab LY, Kearns K, Lewis S, Panda S, Willemsen L, Burel JG and Lindestam Arlehamn CS (2023) Antigen-specificity measurements are the key to understanding T cell responses. Front. Immunol. 14:1127470. doi: 10.3389/fimmu.2023.1127470
Received: 19 December 2022; Accepted: 30 March 2023;
Published: 14 April 2023.
Edited by:
Vandana Kalia, School of Medicine, University of Washington, United StatesReviewed by:
Federico Simonetta, Hôpitaux universitaires de Genève (HUG), SwitzerlandCopyright © 2023 Tippalagama, Chihab, Kearns, Lewis, Panda, Willemsen, Burel and Lindestam Arlehamn. This is an open-access article distributed under the terms of the Creative Commons Attribution License (CC BY). The use, distribution or reproduction in other forums is permitted, provided the original author(s) and the copyright owner(s) are credited and that the original publication in this journal is cited, in accordance with accepted academic practice. No use, distribution or reproduction is permitted which does not comply with these terms.
*Correspondence: Cecilia S. Lindestam Arlehamn, Y2VjaWxpYUBsamkub3Jn
Disclaimer: All claims expressed in this article are solely those of the authors and do not necessarily represent those of their affiliated organizations, or those of the publisher, the editors and the reviewers. Any product that may be evaluated in this article or claim that may be made by its manufacturer is not guaranteed or endorsed by the publisher.
Research integrity at Frontiers
Learn more about the work of our research integrity team to safeguard the quality of each article we publish.