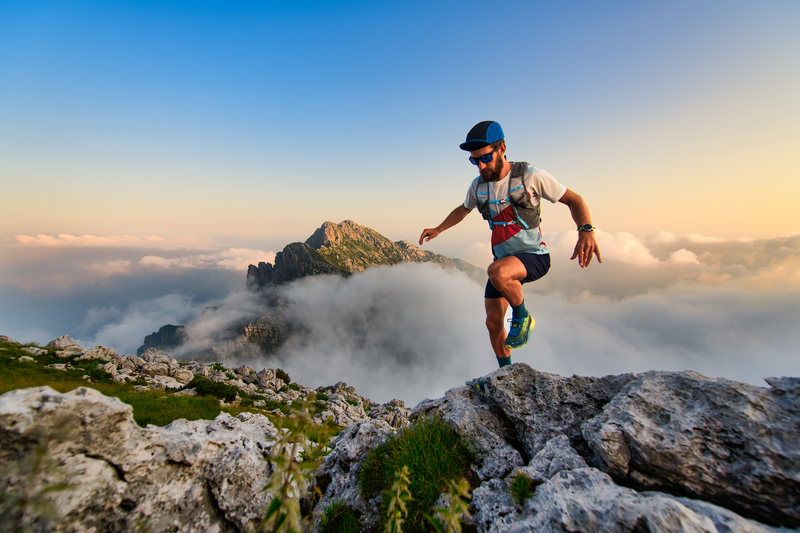
94% of researchers rate our articles as excellent or good
Learn more about the work of our research integrity team to safeguard the quality of each article we publish.
Find out more
REVIEW article
Front. Immunol. , 01 March 2023
Sec. Cancer Immunity and Immunotherapy
Volume 14 - 2023 | https://doi.org/10.3389/fimmu.2023.1088874
This article is part of the Research Topic Mechanism and Application of Synergistic Effect of Radiotherapy and Immunotherapy View all 7 articles
Immunotherapy using immune checkpoint inhibitors (ICIs) is a breakthrough in oncology development and has been applied to multiple solid tumors. However, unlike traditional cancer treatment approaches, immune checkpoint inhibitors (ICIs) initiate indirect cytotoxicity by generating inflammation, which causes enlargement of the lesion in some cases. Therefore, rather than declaring progressive disease (PD) immediately, confirmation upon follow-up radiological evaluation after four–eight weeks is suggested according to immune-related Response Evaluation Criteria in Solid Tumors (ir-RECIST). Given the difficulty for clinicians to immediately distinguish pseudoprogression from true disease progression, we need novel tools to assist in this field. Radiomics, an innovative data analysis technique that quantifies tumor characteristics through high-throughput extraction of quantitative features from images, can enable the detection of additional information from early imaging. This review will summarize the recent advances in radiomics concerning immunotherapy. Notably, we will discuss the potential of applying radiomics to differentiate pseudoprogression from PD to avoid condition exacerbation during confirmatory periods. We also review the applications of radiomics in hyperprogression, immune-related biomarkers, efficacy, and immune-related adverse events (irAEs). We found that radiomics has shown promising results in precision cancer immunotherapy with early detection in noninvasive ways.
Immunotherapy using ICIs has been revolutionary in cancer treatment owing to its significant impact on the reactivation of the immune system (1, 2). Unlike traditional cancer treatment approaches, which kill tumor cells directly, ICIs initiate indirect cytotoxicity by generating inflammation and may cause enlargement of the lesion in some cases. Hence, there may be different interpretations of medical imaging for patients undergoing immunotherapy (3).
Medical images contain many quantitative biomedical features based on intensity, shape, size or volume, and texture, which can offer information on the tumor microenvironment and phenotype. These features are difficult to identify by human vision alone.
Radiomics is an emerging field that extracts quantitative features from medical images and converts digital medical images into mineable, high-dimensional data with new high-throughput approaches.
Features extracted in radiomics can be divided into two categories: “semantic” and “agnostic” (4). Semantic features include shape, location, vascularity, speculation, necrosis, attachments, and lepidics, commonly used in imaging reports. However, radiomics can quantify these features with computer assistance. Agnostic features include histograms (skewness, kurtosis), haralick textures, laws textures, wavelets, Laplacian transforms, Minkowski functionals, and fractal dimensions. These features can provide intratumoral heterogeneity information through quantitative descriptors (4).
The process of radiomics involves the following discrete steps:
1. Image acquisition (i.e., CT, MR, and PET/CT)
2. Volume of interest (VOI) identification and segmentation: identifying tumors and their surroundings as VOIs and delineating the borders of the volume
3. Feature extraction and qualification: extracting and qualifying high-dimensional features from the VOI
4. Modeling: mining extracted features with artificial intelligence to develop classifier models that aid detection, diagnosis, prognosis assessment, and treatment response prediction
This approach could be applied to any aspect of medical imaging analysis, including immunotherapy, thereby providing a novel noninvasive approach to precision cancer treatment.
Genomic and microenvironment heterogeneity within the tumor volume is displayed on the imaging, while these fine distinctions cannot be recognizable by the naked eye, even for experienced radiologists. Nevertheless, these subtle differences can be recognized by radiomics using quantitative assays, allowing for microscopic analyses of medical imaging to establish predictive, diagnostic, and prognostic models (Figure 1).
Figure 1 Radiomics analysis can obtain more information from medical images. (A) There is genomic and microenvironment heterogeneity within the tumor volume displayed on the imaging, while these fine distinctions cannot be recognizable by the naked eye, even for experienced radiologists. (B) These subtle differences can be recognized by radiomics using quantitative assays, allowing microscopic analyses of medical imaging to establish predictive, diagnostic, and prognostic models.
This review summarizes radiomics concerning immunotherapy from a clinical perspective. It discusses its potential to predict outcomes, molecular biomarkers, atypical responses, and immune-related adverse events (irAEs) of immunotherapy.
To date, predictive biomarkers of immune responses are mainly driven by invasive tissue biopsy, while limited biopsy samples may be difficult to provide a holistic picture of the heterogeneity within the tumor and its microenvironment. Radiomics is a powerful auxiliary to conventional invasive biopsies, overcoming the intratumoral heterogeneity within the same patient. The section below describes current progress in immune-related biomarkers using radiomics and how radiomics can overcome these limitations (Table 1).
Table 1 Summary of Key Studies on the Role of radiomics in predicting the expression of immune-related biomarkers.
As a promising treatment for cancer patients, immunotherapy is not effective for all patients (3, 29, 30). Thus, recognizing the appropriate candidate for immunotherapy is of vital importance. PD-L1 expression examined via immunohistochemistry (IHC) is associated with the clinical efficacy of anti-PD-1/PD-L1 therapy. It has been widely applied as a reference to immunotherapy decision-making in most cancer types during clinical practice (29, 31). The expression levels of PD-L1 may change during therapy (32, 33). Due to the existence of intratumoral heterogeneity, the IHC test results of a small number of biopsy samples could not be representative of the whole (34–36).
As the artificial intelligence (AI) field progresses, PD-L1 prediction with radiomics has received increased attention in recent years (Table 1). Giulia Mazzaschi et al. established a noninvasive model with computed tomography (CT)-extracted features to predict the level of PD-L1 expression and tumor infiltrating lymphocytes (TILs). They found that texture, effect, and margins were directly associated with PD-L1 expression and TILs. These features also correlated with the prognosis of patients with non-small cell lung cancer (NSCLC) (5). Similarly, researchers have tried to explore the potential of radiomics biomarkers for predicting the immuno-oncologic characteristics of hepatocellular carcinomas (HCC) based on magnetic resonance imaging (MRI). They found that radiomics features, specifically texture feature variance and enhancement ratios, were strongly associated with PD-L1 expression and were predictive diagnostic biomarkers for assessing early HCC recurrence (6).
PET/CT was also applied to predict PD-L1 expression levels. The researchers extracted imaging histology features from PET/CT images of 399 lung cancer patients, of which 24 features were closely related to PD-L1 expression levels. The researchers further developed prediction models based on these features. PET/CT-based prediction model achieves 88% AUC in predicting patients with >50% PD-L1 expression (8).
Radiomics features, in combination with clinical characteristics, may present a better predictive performance. Yoon et al. built a PD-L1 prediction model using both the Rad-score and clinical variables, which turned out to be more accurate than the clinical-variable-only derived model (7).
To date, radiomics remains insufficient to replace IHC testing for the detection of PD-L1. Despite this, the repeatable and noninvasive nature of radiomics analysis may offer additional information for difficult-to-repeat invasive PD-L1 analyses.
TILs in the tumor microenvironment play an essential part in the immune response against cancer and appear to be associated with the outcome of immunotherapy. Previous studies have indicated that tumor-infiltrating regulatory T cells (Treg) and tumor-associated macrophages (TAMs) induce an immunosuppressive microenvironment that is directly responsible for the failure of immunotherapy, while CD8+ T tumor infiltration is associated with better outcomes to cancer immunotherapy (37).
One of the early attempts to create radiomics signatures aimed at predicting the presence of CD8+ T cells and the clinical efficacy of immunotherapy was conducted by Sun et al. The signature combining eight radiomics features was developed from CT images, genomic data, and ribonucleic acid (RNA) sequencing. The signature was validated on external cohorts to discriminate CD8+ cells and immune phenotypes. Researchers also found that a higher baseline radiomics score is associated with a better response to immunotherapy (12). This study used a radiomics-based biomarker to correlate pathology with prognosis, while the area under the ROC curve (AUC) score for this prediction model was relatively low. In addition, the parameters of image acquisition were not uniform. Therefore, the credibility of the radiomics signature would be affected (12). Another retrospective study revealed that low CT image intensity and high heterogeneity were associated with lower PD-L1 expression and higher CD3 cell infiltration, which was an immune-activated state strongly correlated with favorable overall survival (OS) (13).
Many studies extract radiomics features from pretreatment or posttreatment medical images to predict the TILs associated with the response to immunotherapy (14, 15). “Delta radiomics” can explore changes in the tumor microenvironment before and after immunotherapy. Therefore, it may provide an earlier and more accurate prediction of the efficacy of immunotherapy before visible changes to the naked eye. Khorrami and colleagues explored the potential of radiomics using pretreatment and subsequent CT images from 135 NSCLC patients treated with ICIs. The concordance of the radiomics features with TIL infiltration was confirmed by comparison with TIL infiltration in diagnostic biopsy samples. They reported that delta-radiomics is associated with response and OS in NSCLC patients undergoing ICIs (16).
Previous studies have suggested that TMB is another predictive biomarker for immunotherapy across multiple cancer types, as high TMB is correlated with greater neoantigen and immune infiltration (38).
Researchers have investigated the potential of applying radiomics to predict the TMB status in patients with advanced NSCLC and endometrial and bladder cancers (17, 18, 21, 22) (Table 1). He et al. constructed the TMB radiomics biomarker (TMBRB) to predict the pretreatment TMB status. They observed that TMRRB could accurately divide patients into high TMB and low TMB, thus predicting the OS and progression-free survival (PFS) of NSCLC patients treated with ICIs. The predicted treatment efficacy improves when combined with the Eastern Cooperative Oncology Group (ECOG) performance status (17).
Radiomics has the potential value of being a powerful aid in classifying TMB status. Specifically, combining clinical and pathological features may improve prediction performance (14–16). Radiomics may provide sufficient information despite intratumor heterogeneity to assist clinical decisions on immunotherapy.
Studies have also explored the prediction of other predictive biomarkers of immunotherapy. Recent studies have evaluated whether radiomics can identify mismatch repair (MMR)/microsatellite instability (MSI) status. These explorations focused on gastrointestinal malignancies based on CT, MRI, and positron emission tomography-computed tomography (PET/CT) images (19, 23–25, 39–43) (Table 1).
CAO et al. evaluated whether CT-based radiomics can predict MSI status in 502 patients with colorectal cancer (CRC) based on preoperative contrast-enhanced CT images. They further combined the clinical characteristics with radiomics and then developed a nomogram to predict the MSI status. The predictive performance of the radiomics-clinical nomogram was superior to radiomics only and clinical only (24). Such findings are consistent with another study (25). Combining clinical and pathological features with radiomics may add specificity to the prediction model and contribute to personalized clinical decision-making. The MRI-based MSI prediction model was similarly developed, with an AUC of 0.868 (26). Jiang et al. immunoscored gastric cancer patients based on the immunohistochemical expression of CD3, CD8, CD45 and CD66b and classified the immunoscores using CT-based radiomics model. The radiomics model accurately classified patients with high immunoscore and low immunoscore with an AUC of 0.786 and had the potential to select patients who would benefit from chemotherapy (27).
The efficacy of immunotherapy has been proven in large-scale, randomized clinical trials and clinical practice (29, 44–51). However, immunotherapy is only partially effective, emphasizing the need for finding noninvasive and accurate predictive biomarkers to target immunotherapy to the appropriate patients (52, 53).
Predictive biomarkers of immunotherapy, including PD-L1 and TMB status, are acquired via biopsy, which is invasive, difficult to perform dynamically, and restricted to a small sample of pathological specimens.
Radiomics offers a noninvasive whole-body evaluation of tissue biomarkers. Heterogeneity within the tumor may harbor prognostic information that can be captured and transferred into radiomics features by radiomics analysis. Considerable evidence suggests that radiomics could predict immunotherapy efficacy by recognizing radiomics features associated with response (Table 2).
Table 2 Summary of Key Studies on the Role of radiomics in predicting the response to immunotherapy.
Trebeschi et al. developed a model able to predict the response to immunotherapy. They suggested that greater heterogeneous and nonuniform lesions were associated with a better response in NSCLC, possibly with infiltration and inflammation of the tumor, while the sample size of the melanoma cohort was too small to identify optimal imaging biomarkers (54).
Skewness, representing the heterogeneity of a segmented lesion, was a significant independent predictor of OS and PFS, with a higher skewness value linked with poorer survival (55). Similarly, Velcheti analyzed the CT features of 50 NSCLC patients who underwent nivolumab, and they found that vessel tortuosity was an independent predictor of nivolumab’s efficacy (56).
Radiomics features from 18F-FDG PET/CT scans also showed the ability to predict the response to immunotherapy. Several texture features (PET_SRLGE, KLD_SZE) were associated with durable clinical benefits, demonstrating that patients with more heterogeneous tumors might benefit more from immunotherapy. Notably, the prospective cohort validated the model with an AUC of 0.81 (57). However, these results are somewhat inconsistent with the results from prior studies, which indicated that more heterogeneous tumors with CT textures are associated with worse response rates to chemotherapy or radiation.
Other retrospective studies have explored the potential of radiomics in evaluating survival and responses to immunotherapy in different cancer types, including melanoma (58, 66), gastrointestinal malignancies (59, 67), metastatic renal cell carcinoma (mRCC) (60), treatment-refractory adult solid tumors (61), metastatic urothelial carcinoma (62), and NSCLC (11, 65, 68).
The predictive performance of the radiomics model (consisting of small run emphasis and difference entropy) developed by Valentinuzzi et al. was superior to that of PD-L1 and iRECIST, with an AUC of 0.90. Specifically, small run emphasis has the highest predictive performance to discriminate survival, with higher small run emphasis possibly having OS survival from pembrolizumab treatment (63). These results reflected that patients with more homogeneous tumors might benefit from immunotherapy, consistent with a previous study conducted by Polverari et al., where nonresponders exhibited higher tumor heterogeneity at pretreatment CT images (reflected by higher kurtosis and skewness) than responders (11). A study conducted by Mu et al. showed the opposite result. They found that heterogeneous tumors might be more likely to achieve durable clinical responses (57).
These studies have demonstrated that radiomics has the potential to predict the response to immunotherapy and could facilitate clinical decision-making. Despite its great potential, the application of radiomics in clinical immunotherapy is still in its infancy. Reproducibility and standardization are major problems. Studies have already explored the standardized workflow of radiomics (70–74).
In the widespread use of immunotherapy in cancer treatment, unconventional characteristics of response, so-called atypical response, have been observed through imaging (Figure 2) (75–77). Atypical patterns of response, including pseudoprogression and hyperprogression, have been demonstrated in clinical trials of ICIs and have prompted the development of immune-related response criteria (78–80). In this part, we summarize how radiomics can support clinical decision-making in light of atypical responses (Table 3).
Figure 2 Atypical patterns in patients undergoing immunotherapy. (A) Hyperprogression is an atypical response to ICIs with a paradoxical acceleration of tumor growth soon after the initiation of immunotherapy. (B) Dissociated Response is defined as a reduction at baseline or increase < 20% in target lesions compared with a nadir in the presence of the new lesion. (C) Pseudoprogression is defined as an initial radiographic increase in tumor size or the appearance of new lesions, followed by a response.
Pseudoprogression is defined as an initial radiographic increase in tumor size or the appearance of new lesions, followed by a response (76, 87, 88). This radiologic effect is due to inflammatory cell infiltration around tumor cells, with an apparent increase in size, and can be confused with tumor cell proliferation (79, 87, 89). To discern pseudoprogression, immune-related response criteria were developed, which suggest further radiological evaluation after four to eight weeks rather than declaring PD immediately (90). A switch to next-line treatment might be delayed while waiting for the confirmatory follow-up evaluation. It is crucial to distinguish pseudoprogression from true disease progression in a timely manner, as it is highly relevant in daily clinical decision-making processes. Therefore, early detection of pseudoprogression is of vital importance.
Texture features extracted from radiological images have been identified to distinguish inflammatory infiltration from tumor cell proliferation. Basler et al. evaluated the capability of PET/CT-based radiomics features, lesion volume, and routine blood markers to differentiate pseudoprogression from true progression at the third month. Of the seven models constructed based on blood, volume, and radiomics, the blood-radiomics model has the best predictive performance, achieving the highest AUC (0.82), and it is a promising biomarker for the early differentiation of pseudoprogression in the third month (81). In a single-center retrospective study, Ji et al. used four radiomics models constructed using contrast-enhanced CT of 87 patients with lung cancer treated with ICIs; of these, model three and model four had AUCs of 0.736 and 0.760, respectively, and were both accurate in predicting responses in two of three pseudoprogression patients (59). Similarly, He et al. used a radiomics approach to identify pseudoprogression from true progression. They extracted intratumoral and peritumoral radiomics features from baseline chest CT scans of 135 patients and built a predictive model. The model had an AUC of 0.96 in the validation set (82). These findings suggest that radiomics can predict pseudoprogression in the course of immunotherapy and may supplement immune-related response evaluation criteria.
Hyperprogression is an atypical response to ICIs with paradoxical tumor growth acceleration soon after immunotherapy initiation (91–94). It has been described in numerous cancer types with an incidence of 6%–29% (95, 96). It has been associated with high metastatic burden, significantly shortened survival, and poorer performance status (95, 97–100), thus limiting the potential for administration of other therapies. Identifying high-risk groups is vital, yet there are no predictive biomarkers with apparent effects to identify the risk of hyperprogression (96, 98). As a noninvasive method, radiomics has been explored for the risk stratification of hyperprogression in patients undergoing immunotherapy.
To evaluate the accuracy of a pretreatment CT-based radiomics model in predicting hyperprogression in NSCLC patients treated with ICIs, Vaidya et al. found that peritumoural texture and vasculature patterns in the baseline CT scans positively correlate with hyperprogression. These features had higher expression in hyperprogression than in responders or nonresponders, suggesting that patients with more heterogeneous tumors are more likely to derive durable clinical benefits (83). Consistent with a previous study, a complex model including three radiomics features extracted from the tumor border and several clinical variables was able to predict hyperprogression with an accuracy of 82.28% (84). Other radiomics features, such as the maximum gray value, are intimately linked to the determination of hyperprogression (59). Deep learning models built by Mu et al. have also been reported as possible predictive biomarkers of hyperprogression in NSCLC patients undergoing ICIs. A total of 33.33% of patients with higher EGFR-deep learning scores developed hyperprogression, and deep learning scores were associated with shorter PFS among patients undergoing ICIs (10). Similarly, He et al. developed a prediction model using a CT-based radiomics approach which had an accuracy of 0.933 in identifying hyperprogression from true progression (82). PET/CT-based radiomics has also been attempted for predicting hyperprogression. Gabryś et al. developed a predictive model using PET/CT of patients with metastatic melanoma. CT-based radiological features were shown to be better predictors of hyperprogression than PET-based features (85).
Given the poor prognosis of hyperprogression, it is of great importance for high-risk populations to be screened before initiating immunotherapy. The remarkably accelerated development of radiomics in immunotherapy suggests that radiomics could be used to stratify the risk of hyperprogression. These findings warrant further exploration.
IrAEs associated with immunotherapy, resulting from activating an immune response against healthy tissues, may involve almost every organ and system (101, 102). Timely diagnosis and prompt management depending on its severity, with a proper suspension of ICIs or corticosteroid treatment, is vital. If left untreated, irAEs could develop into life-threatening complications. Therefore, early diagnosis and monitoring of irAEs are crucial for radiologists.
Medical imaging, including CT, ultrasonography, magnetic resonance imaging, X-rays, and PET/CT, was used to detect irAEs. In a retrospective study with a small sample size, Mekki et al. found that 74% (19) of irAE patients (55) showed abnormalities on medical imaging and could be diagnosed by radiologists. The rates of enterocolitis, hypophysitis, thyroiditis, hepatitis, arthralgia or arthritis, lung/mediastinum side effects, and pancreas range from 28% to 100% (103).
The detection of irAEs generally depends on blood test indicators, clinical manifestations, and imaging characteristics. However, radiomics can help in the identification of early invisible signs of irAEs in medical imaging. Colen et al. utilized a radiomics approach to predict the risk for immune-related pneumonitis. They extracted 1860 radiomics features from baseline chest CT scans of 32 patients treated with ICIs, of whom two developed immune-related pneumonitis. Selective radiomics features were utilized to develop the predictive model of the subsequent development of pneumonitis. This model correctly identified the two patients who developed immune-related pneumonitis (86). Despite the noninvasive and impersonal nature of radiomics, studies relevant to the early detection of irAEs are rarely reported (Table 3).
With the rapid development and application of artificial intelligence in medicine, radiomics may become a valuable tool in clinical decision-making. Here, we focus on the exciting and innovative space of radiomics to solve the problems in immunotherapy and discuss how radiomics serves as a means to support the precision design of immunotherapy, especially in pseudoprogression and hyperprogression.
Radiomics represents a potential noninvasive and feasible strategy in clinical decision-making that can ensure timely access to results and minimize the bias caused by localized tissue sampling from heterogeneous tumors. In addition, radiomics can potentially be applied to daily clinical practice to monitor responses and side reactions to ICIs.
These advantages provide convenience for clinical diagnosis and treatment, such as noninvasive biopsy, differentiating pseudoprogression from true progression, risk stratification for hyperprogression, immune-related response assessment, and so on. Current studies have indicated that the potential of radiomics in immunotherapy is substantial.
While the results of recent radiomics research are promising, they remain insufficient for its widespread application in daily clinical practice, and radiomics cannot replace biopsy or iRECIST in clinical application at this stage. Limitations and challenges in terms of practical application are not neglectable, and reproducibility presents the most significant challenge.
The heterogeneity of articles exploring radiomics features is an important issue that limits the generalization of the role of radiomics in daily clinical practice, as different imaging modalities are studied (CT, MRI, PET/CT) in different clinical settings (several different neoplasms at various stages of disease) with different “a priori” expected responsivities to immunotherapy. Due to the complexity of radiomics, few studies can be wholly reproduced, thus inhibiting the widespread use of radiomics in clinical practice (104).
Reproducibility remains a huge obstacle in the pace of clinical application Guidelines were established to standardize protocol and analysis of radiomics research (70, 105). The radiomics quality score (RQS) (106) and Individual Prognosis or Diagnosis (TRIPOD) (107) were developed to bridge this gap. Moreover, ongoing single and multicenter prospective randomized clinical trials are needed to improve and validate reliability and reproducibility (Table 4). Integration and analysis of radiomics features with genomics, proteomics, and other omics data would provide additional information in precision medicine by uncovering microlevel features (105, 108).
Radiomics provides a window of opportunity for precision medicine of immunotherapy by analyzing microscopic medical imaging in a noninvasive, efficient, economical, and rapid fashion. In the foreseeable future, we envision that radiomics will be widely applied to clinical decision-making and will serve as the impetus for the next major breakthroughs in precision medicine. However, at this stage, there are still significant challenges in the process of clinical translation and application, and further refinements are warranted.
HZ, QL, WW, NL, CY and LZ: Literature search, concepts development, manuscript drafts. All authors contributed to the article and approved the submitted version. All figures created with BioRender.com.
The authors declare that the research was conducted in the absence of any commercial or financial relationships that could be construed as a potential conflict of interest.
All claims expressed in this article are solely those of the authors and do not necessarily represent those of their affiliated organizations, or those of the publisher, the editors and the reviewers. Any product that may be evaluated in this article, or claim that may be made by its manufacturer, is not guaranteed or endorsed by the publisher.
1. Waldman AD, Fritz JM, Lenardo MJ. A guide to cancer immunotherapy: From T cell basic science to clinical practice. Nat Rev Immunol (2020) 20(11):651–68. doi: 10.1038/s41577-020-0306-5
2. Pinter M, Scheiner B, Peck-Radosavljevic M. Immunotherapy for advanced hepatocellular carcinoma: A focus on special subgroups. Gut (2021) 70(1):204–14. doi: 10.1136/gutjnl-2020-321702
3. Bagchi S, Yuan R, Engleman EG. Immune checkpoint inhibitors for the treatment of cancer: Clinical impact and mechanisms of response and resistance. Annu Rev Pathol (2021) 16:223–49. doi: 10.1146/annurev-pathol-042020-042741
4. Gillies RJ, Kinahan PE, Hricak H. Radiomics: Images are more than pictures, they are data. Radiology (2016) 278(2):563–77. doi: 10.1148/radiol.2015151169
5. Mazzaschi G, Milanese G, Pagano P, Madeddu D, Gnetti L, Trentini F, et al. Integrated CT imaging and tissue immune features disclose a radio-immune signature with high prognostic impact on surgically resected NSCLC. Lung Cancer (2020) 144:30–9. doi: 10.1016/j.lungcan.2020.04.006
6. Hectors SJ, Lewis S, Besa C, King MJ, Said D, Putra J, et al. MRI Radiomics features predict immuno-oncological characteristics of hepatocellular carcinoma. Eur Radiol (2020) 30(7):3759–69. doi: 10.1007/s00330-020-06675-2
7. Yoon J, Suh YJ, Han K, Cho H, Lee HJ, Hur J, et al. Utility of CT radiomics for prediction of PD-L1 expression in advanced lung adenocarcinomas. Thorac Cancer (2020) 11(4):993–1004. doi: 10.1111/1759-7714.13352
8. Jiang M, Sun D, Guo Y, Guo Y, Xiao J, Wang L, et al. Assessing PD-L1 expression level by radiomic features from PET/CT in nonsmall cell lung cancer patients: An initial result. Acad Radiol (2020) 27(2):171–9. doi: 10.1016/j.acra.2019.04.016
9. Chen RY, Lin YC, Shen WC, Hsieh TC, Yen KY, Chen SW, et al. Associations of tumor PD-1 ligands, immunohistochemical studies, and textural features in (18)F-FDG PET in squamous cell carcinoma of the head and neck. Sci Rep (2018) 8(1):105. doi: 10.1038/s41598-017-18489-2
10. Mu W, Jiang L, Zhang J, Shi Y, Gray JE, Tunali I, et al. Non-invasive decision support for NSCLC treatment using PET/CT radiomics. Nat Commun (2020) 11(1):5228. doi: 10.1038/s41467-020-19116-x
11. Polverari G, Ceci F, Bertaglia V, Reale ML, Rampado O, Gallio E, et al. (18)F-FDG pet parameters and radiomics features analysis in advanced nsclc treated with immunotherapy as predictors of therapy response and survival. Cancers (Basel) (2020) 12(5). doi: 10.3390/cancers12051163
12. Sun R, Limkin EJ, Vakalopoulou M, Dercle L, Champiat S, Han SR, et al. A radiomics approach to assess tumour-infiltrating CD8 cells and response to anti-PD-1 or anti-PD-L1 immunotherapy: An imaging biomarker, retrospective multicohort study. Lancet Oncol (2018) 19(9):1180–91. doi: 10.1016/S1470-2045(18)30413-3
13. Tang C, Hobbs B, Amer A, Li X, Behrens C, Canales JR, et al. Development of an immune-pathology informed radiomics model for non-small cell lung cancer. Sci Rep (2018) 8(1):1922. doi: 10.1038/s41598-018-20471-5
14. Liao H, Zhang Z, Chen J, Liao M, Xu L, Wu Z, et al. Preoperative radiomic approach to evaluate tumor-infiltrating CD8(+) T cells in hepatocellular carcinoma patients using contrast-enhanced computed tomography. Ann Surg Oncol (2019) 26(13):4537–47. doi: 10.1245/s10434-019-07815-9
15. Chen S, Feng S, Wei J, Liu F, Li B, Li X, et al. Pretreatment prediction of immunoscore in hepatocellular cancer: A radiomics-based clinical model based on gd-EOB-DTPA-enhanced MRI imaging. Eur Radiol (2019) 29(8):4177–87. doi: 10.1007/s00330-018-5986-x
16. Khorrami M, Prasanna P, Gupta A, Patil P, Velu PD, Thawani R, et al. Changes in CT radiomic features associated with lymphocyte distribution predict overall survival and response to immunotherapy in non-small cell lung cancer. Cancer Immunol Res (2020) 8(1):108–19. doi: 10.1158/2326-6066.CIR-19-0476
17. He B, Dong D, She Y, Zhou C, Fang M, Zhu Y, et al. Predicting response to immunotherapy in advanced non-small-cell lung cancer using tumor mutational burden radiomic biomarker. J Immunother Cancer (2020) 8(2). doi: 10.1136/jitc-2020-000550
18. Veeraraghavan H, Friedman CF, DeLair DF, Ninčević J, Himoto Y, Bruni SG, et al. Machine learning-based prediction of microsatellite instability and high tumor mutation burden from contrast-enhanced computed tomography in endometrial cancers. Sci Rep (2020) 10(1):17769. doi: 10.1038/s41598-020-72475-9
19. Golia Pernicka JS, Gagniere J, Chakraborty J, Yamashita R, Nardo L, Creasy JM, et al. Radiomics-based prediction of microsatellite instability in colorectal cancer at initial computed tomography evaluation. Abdom Radiol (NY) (2019) 44(11):3755–63. doi: 10.1007/s00261-019-02117-w
20. Kather JN, Pearson AT, Halama N, Jäger D, Krause J, Loosen SH, et al. Deep learning can predict microsatellite instability directly from histology in gastrointestinal cancer. Nat Med (2019) 25:1054–6. doi: 10.1038/s41591-019-0462-y
21. Wang X, Kong C, Xu W, Yang S, Shi D, Zhang J, et al. Decoding tumor mutation burden and driver mutations in early stage lung adenocarcinoma using CT-based radiomics signature. Thorac Cancer (2019) 10(10):1904–12. doi: 10.1111/1759-7714.13163
22. Wen Q, Yang Z, Dai H, Feng A, Li Q. Radiomics study for predicting the expression of PD-L1 and tumor mutation burden in non-small cell lung cancer based on CT images and clinicopathological features. Front Oncol (2021) 11:620246. doi: 10.3389/fonc.2021.620246
23. Li J, Yang Z, Xin B, Hao Y, Lu W, Song S, et al. Quantitative prediction of microsatellite instability in colorectal cancer with preoperative PET/CT-based radiomics. Front Oncol (2021) 11:702055. doi: 10.3389/fonc.2021.702055
24. Cao Y, Zhang G, Zhang J, Yang Y, Ren J, Yan X, et al. Predicting microsatellite instability status in colorectal cancer based on triphasic enhanced computed tomography radiomics signatures: A multicenter study. Front Oncol (2021) 11:687771. doi: 10.3389/fonc.2021.687771
25. Pei Q, Yi X, Chen C, Pang P, Fu Y, Lei G, et al. Pre-treatment CT-based radiomics nomogram for predicting microsatellite instability status in colorectal cancer. Eur Radiol (2022) 32(1):714–24. doi: 10.1007/s00330-021-08167-3
26. Zhang W, Yin H, Huang Z, Zhao J, Zheng H, He D, et al. Development and validation of MRI-based deep learning models for prediction of microsatellite instability in rectal cancer. Cancer Med (2021) 10(12):4164–73. doi: 10.1002/cam4.3957
27. Jiang Y, Wang H, Wu J, Chen C, Yuan Q, Huang W, et al. Noninvasive imaging evaluation of tumor immune microenvironment to predict outcomes in gastric cancer. Ann Oncol (2020) 31(6):760–8. doi: 10.1016/j.annonc.2020.03.295
28. Xue K, Liu L, Liu Y, Guo Y, Zhu Y, Zhang M. Radiomics model based on multi-sequence MR images for predicting preoperative immunoscore in rectal cancer. Radiol Med (2022) 127:702–13. doi: 10.1007/s11547-022-01507-3
29. Reck M, Rodríguez-Abreu D, Robinson AG, Hui R, Csőszi T, Fülöp A, et al. Pembrolizumab versus chemotherapy for PD-L1-Positive non-Small-Cell lung cancer. N Engl J Med (2016) 375(19):1823–33. doi: 10.1056/NEJMoa1606774
30. de Miguel M, Calvo E. Clinical challenges of immune checkpoint inhibitors. Cancer Cell (2020) 38(3):326–33. doi: 10.1016/j.ccell.2020.07.004
31. Daud AI, Wolchok JD, Robert C, Hwu WJ, Weber JS, Ribas A, et al. Programmed death-ligand 1 expression and response to the anti-programmed death 1 antibody pembrolizumab in melanoma. J Clin Oncol (2016) 34(34):4102–9. doi: 10.1200/JCO.2016.67.2477
32. Van Der Kraak L, Goel G, Ramanan K, Kaltenmeier C, Zhang L, Normolle DP, et al. 5-fluorouracil upregulates cell surface B7-H1 (PD-L1) expression in gastrointestinal cancers. J Immunother Cancer (2016) 4:65. doi: 10.1186/s40425-016-0163-8
33. Vranic S, Cyprian FS, Gatalica Z, Palazzo J. PD-L1 status in breast cancer: Current view and perspectives. Semin Cancer Biol (2021) 72:146–54. doi: 10.1016/j.semcancer.2019.12.003
34. Mamlouk S, Childs LH, Aust D, Heim D, Melching F, Oliveira C, et al. DNA Copy number changes define spatial patterns of heterogeneity in colorectal cancer. Nat Commun (2017) 8:14093. doi: 10.1038/ncomms14093
35. Cell lines recapitulate intratumoral heterogeneity observed In vivo. Cancer Discovery (2021) 11(1):11. doi: 10.1158/2159-8290.CD-RW2020-166
36. Kinker GS, Greenwald AC, Tal R, Orlova Z, Cuoco MS, McFarland JM, et al. Pan-cancer single-cell RNA-seq identifies recurring programs of cellular heterogeneity. Nat Genet (2020) 52(11):1208–18. doi: 10.1038/s41588-020-00726-6
37. Pascual-García M, Bonfill-Teixidor E, Planas-Rigol E, Rubio-Perez C, Iurlaro R, Arias A, et al. LIF regulates CXCL9 in tumor-associated macrophages and prevents CD8(+) T cell tumor-infiltration impairing anti-PD1 therapy. Nat Commun (2019) 10(1):2416. doi: 10.1038/s41467-019-10369-9
38. Samstein RM, Lee CH, Shoushtari AN, Hellmann MD, Shen R, Janjigian YY, et al. Tumor mutational load predicts survival after immunotherapy across multiple cancer types. Nat Genet (2019) 51(2):202–6. doi: 10.1038/s41588-018-0312-8
39. Fan S, Li X, Cui X, Zheng L, Ren X, Ma W, et al. Computed tomography-based radiomic features could potentially predict microsatellite instability status in stage II colorectal cancer: A preliminary study. Acad Radiol (2019) 26(12):1633–40. doi: 10.1016/j.acra.2019.02.009
40. Huang Z, Zhang W, He D, Cui X, Tian S, Yin H, et al. Development and validation of a radiomics model based on T2WI images for preoperative prediction of microsatellite instability status in rectal cancer: Study protocol clinical trial (SPIRIT compliant). Med (Baltimore) (2020) 99(10):e19428. doi: 10.1097/MD.0000000000019428
41. Li Z, Dai H, Liu Y, Pan F, Yang Y, Zhang M. Radiomics analysis of multi-sequence MR images for predicting microsatellite instability status preoperatively in rectal cancer. Front Oncol (2021) 11:697497. doi: 10.3389/fonc.2021.697497
42. Li Z, Zhong Q, Zhang L, Wang M, Xiao W, Cui F, et al. Computed tomography-based radiomics model to preoperatively predict microsatellite instability status in colorectal cancer: A multicenter study. Front Oncol (2021) 11:666786. doi: 10.3389/fonc.2021.666786
43. Zhang W, Huang Z, Zhao J, He D, Li M, Yin H, et al. Development and validation of magnetic resonance imaging-based radiomics models for preoperative prediction of microsatellite instability in rectal cancer. Ann Transl Med (2021) 9(2):134. doi: 10.21037/atm-20-7673
44. André T, Shiu KK, Kim TW, Jensen BV, Jensen LH, Punt C, et al. Pembrolizumab in microsatellite-Instability-High advanced colorectal cancer. N Engl J Med (2020) 383(23):2207–18. doi: 10.1056/NEJMoa2017699
45. Horn L, Mansfield AS, Szczęsna A, Havel L, Krzakowski M, Hochmair MJ, et al. First-line atezolizumab plus chemotherapy in extensive-stage small-cell lung cancer. N Engl J Med (2018) 379(23):2220–9. doi: 10.1056/NEJMoa1809064
46. Janjigian YY, Shitara K, Moehler M, Garrido M, Salman P, Shen L, et al. First-line nivolumab plus chemotherapy versus chemotherapy alone for advanced gastric, gastro-oesophageal junction, and oesophageal adenocarcinoma (CheckMate 649): A randomised, open-label, phase 3 trial. Lancet (2021) 398(10294):27–40. doi: 10.1016/S0140-6736(21)00797-2
47. Kato K, Cho BC, Takahashi M, Okada M, Lin CY, Chin K, et al. Nivolumab versus chemotherapy in patients with advanced oesophageal squamous cell carcinoma refractory or intolerant to previous chemotherapy (ATTRACTION-3): A multicentre, randomised, open-label, phase 3 trial. Lancet Oncol (2019) 20(11):1506–17. doi: 10.1016/S1470-2045(19)30626-6
48. Kuruvilla J, Ramchandren R, Santoro A, Paszkiewicz-Kozik E, Gasiorowski R, Johnson NA, et al. Pembrolizumab versus brentuximab vedotin in relapsed or refractory classical Hodgkin lymphoma (KEYNOTE-204): An interim analysis of a multicentre, randomised, open-label, phase 3 study. Lancet Oncol (2021) 22(4):512–24. doi: 10.1016/S1470-2045(21)00005-X
49. Powles T, Csőszi T, Özgüroğlu M, Matsubara N, Géczi L, Cheng SY, et al. Pembrolizumab alone or combined with chemotherapy versus chemotherapy as first-line therapy for advanced urothelial carcinoma (KEYNOTE-361): A randomised, open-label, phase 3 trial. Lancet Oncol (2021) 22(7):931–45. doi: 10.1016/S1470-2045(21)00152-2
50. Reardon DA, Brandes AA, Omuro A, Mulholland P, Lim M, Wick A, et al. Effect of nivolumab vs bevacizumab in patients with recurrent glioblastoma: The CheckMate 143 phase 3 randomized clinical trial. JAMA Oncol (2020) 6(7):1003–10. doi: 10.1001/jamaoncol.2020.1024
51. Rittmeyer A, Barlesi F, Waterkamp D, Park K, Ciardiello F, von Pawel J, et al. Atezolizumab versus docetaxel in patients with previously treated non-small-cell lung cancer (OAK): A phase 3, open-label, multicentre randomised controlled trial. Lancet (2017) 389(10066):255–65. doi: 10.1016/S0140-6736(16)32517-X
52. Manson G, Norwood J, Marabelle A, Kohrt H, Houot R. Biomarkers associated with checkpoint inhibitors. Ann Oncol (2016) 27(7):1199–206. doi: 10.1093/annonc/mdw181
53. Jerby-Arnon L, Shah P, Cuoco MS, Rodman C, Su MJ, Melms JC, et al. A cancer cell program promotes T cell exclusion and resistance to checkpoint blockade. Cell (2018) 175(4):984–97.e24. doi: 10.1016/j.cell.2018.09.006
54. Trebeschi S, Drago SG, Birkbak NJ, Kurilova I, Calin AM, Delli Pizzi A, et al. Predicting response to cancer immunotherapy using noninvasive radiomic biomarkers. Ann Oncol (2019) 30(6):998–1004. doi: 10.1093/annonc/mdz108
55. Durot C, Mulé S, Soyer P, Marchal A, Grange F, Hoeffel C. Metastatic melanoma: Pretreatment contrast-enhanced CT texture parameters as predictive biomarkers of survival in patients treated with pembrolizumab. Eur Radiol (2019) 29(6):3183–91. doi: 10.1007/s00330-018-5933-x
56. Velcheti V, Alilou M, Khunger M, Thawani R, Madabhushi A. Changes in computer extracted features of vessel tortuosity on CT scans post-treatment in responders compared to non-responders for non–small cell lung cancer on immunotherapy. J Thorac Oncol (2017) 12(8, Supplement):S1547. doi: 10.1016/j.jtho.2017.06.067
57. Mu W, Tunali I, Gray JE, Qi J, Schabath MB, Gillies RJ. Radiomics of (18)F-FDG PET/CT images predicts clinical benefit of advanced NSCLC patients to checkpoint blockade immunotherapy. Eur J Nucl Med Mol Imaging (2020) 47(5):1168–82. doi: 10.1007/s00259-019-04625-9
58. Bhatia A, Birger M, Veeraraghavan H, Um H, Tixier F, McKenney AS, et al. MRI Radiomic features are associated with survival in melanoma brain metastases treated with immune checkpoint inhibitors. Neuro Oncol (2019) 21(12):1578–86. doi: 10.1093/neuonc/noz141
59. Ji Z, Cui Y, Peng Z, Gong J, Zhu HT, Zhang X, et al. Use of radiomics to predict response to immunotherapy of malignant tumors of the digestive system. Med Sci Monit (2020) 26:e924671. doi: 10.12659/MSM.924671
60. Khene ZE, Mathieu R, Peyronnet B, Kokorian R, Gasmi A, Khene F, et al. Radiomics can predict tumour response in patients treated with nivolumab for a metastatic renal cell carcinoma: An artificial intelligence concept. World J Urol (2021) 39(9):3707–9. doi: 10.1007/s00345-020-03334-5
61. Korpics MC, Polley MY, Bhave SR, Redler G, Pitroda SP, Luke JJ, et al. A validated T cell radiomics score is associated with clinical outcomes following multisite SBRT and pembrolizumab. Int J Radiat Oncol Biol Phys (2020) 108(1):189–95. doi: 10.1016/j.ijrobp.2020.06.026
62. Park KJ, Lee JL, Yoon SK, Heo C, Park BW, Kim JK. Radiomics-based prediction model for outcomes of PD-1/PD-L1 immunotherapy in metastatic urothelial carcinoma. Eur Radiol (2020) 30(10):5392–403. doi: 10.1007/s00330-020-06847-0
63. Valentinuzzi D, Vrankar M, Boc N, Ahac V, Zupancic Z, Unk M, et al. [18F]FDG PET immunotherapy radiomics signature (iRADIOMICS) predicts response of non-small-cell lung cancer patients treated with pembrolizumab. Radiol Oncol (2020) 54(3):285–94. doi: 10.2478/raon-2020-0042
64. Wang ZL, Mao LL, Zhou ZG, Si L, Zhu HT, Chen X, et al. Pilot study of CT-based radiomics model for early evaluation of response to immunotherapy in patients with metastatic melanoma. Front Oncol (2020) 10:1524. doi: 10.3389/fonc.2020.01524
65. Nardone V, Tini P, Pastina P, Botta C, Reginelli A, Carbone SF, et al. Radiomics predicts survival of patients with advanced non-small cell lung cancer undergoing PD-1 blockade using nivolumab. Oncol Lett (2020) 19(2):1559–66. doi: 10.3892/ol.2019.11220
66. Dercle L, Zhao B, Gönen M, Moskowitz CS, Firas A, Beylergil V, et al. Early readout on overall survival of patients with melanoma treated with immunotherapy using a novel imaging analysis. JAMA Oncol (2022) 8(3):385–92. doi: 10.1001/jamaoncol.2021.6818
67. Liang Z, Huang A, Wang L, Bi J, Kuang B, Xiao Y, et al. A radiomics model predicts the response of patients with advanced gastric cancer to PD-1 inhibitor treatment. Aging (Albany NY) (2022) 14(2):907–22. doi: 10.18632/aging.203850
68. Barabino E, Rossi G, Pamparino S, Fiannacca M, Caprioli S, Fedeli A, et al. Exploring response to immunotherapy in non-small cell lung cancer using delta-radiomics. Cancers (Basel) (2022) 14(2). doi: 10.3390/cancers14020350
69. Yu Y, Bai Y, Zheng P, Wang N, Deng X, Ma H, et al. Radiomics-based prediction of response to immune checkpoint inhibitor treatment for solid cancers using computed tomography: a real-world study of two centers. BMC Cancer (2022) 22:1241. doi: 10.1186/s12885-022-10344-6
70. Zwanenburg A, Vallières M, Abdalah MA, Aerts HJWL, Andrearczyk V, Apte A, et al. The image biomarker standardization initiative: Standardized quantitative radiomics for high-throughput image-based phenotyping. Radiology (2020) 295(2):328–38. doi: 10.1148/radiol.2020191145
71. Vallières M, Zwanenburg A, Badic B, Cheze Le Rest C, Visvikis D, Hatt M. Responsible radiomics research for faster clinical translation. J Nucl Med (2018) 59(2):189–93. doi: 10.2967/jnumed.117.200501
72. Bogowicz M, Vuong D, Huellner MW, Pavic M, Andratschke N, Gabrys HS, et al. CT radiomics and PET radiomics: Ready for clinical implementation? Q J Nucl Med Mol Imaging (2019) 63(4):355–70. doi: 10.23736/S1824-4785.19.03192-3
73. Kumar V, Gu Y, Basu S, Berglund A, Eschrich SA, Schabath MB, et al. Radiomics: The process and the challenges. Magn Reson Imaging (2012) 30(9):1234–48. doi: 10.1016/j.mri.2012.06.010
74. Pfaehler E, Beukinga RJ, de Jong JR, Slart R, Slump CH, Dierckx R, et al. Repeatability of (18) f-FDG PET radiomic features: A phantom study to explore sensitivity to image reconstruction settings, noise, and delineation method. Med Phys (2019) 46(2):665–78. doi: 10.1002/mp.13322
75. Borcoman E, Kanjanapan Y, Champiat S, Kato S, Servois V, Kurzrock R, et al. Novel patterns of response under immunotherapy. Ann Oncol (2019) 30(3):385–96. doi: 10.1093/annonc/mdz003
76. Billan S, Kaidar-Person O, Gil Z. Treatment after progression in the era of immunotherapy. Lancet Oncol (2020) 21(10):e463–e76. doi: 10.1016/S1470-2045(20)30328-4
77. Sheikhbahaei S, Marcus CV, Sadaghiani MS, Rowe SP, Pomper MG, Solnes LB. Imaging of cancer immunotherapy: Response assessment methods, atypical response patterns, and immune-related adverse events, from the AJR special series on imaging of inflammation. AJR Am J Roentgenol (2022) 218(6):940–52. doi: 10.2214/AJR.21.26538
78. Hodi FS, Hwu WJ, Kefford R, Weber JS, Daud A, Hamid O, et al. Evaluation of immune-related response criteria and RECIST v1.1 in patients with advanced melanoma treated with pembrolizumab. J Clin Oncol (2016) 34(13):1510–7. doi: 10.1200/JCO.2015.64.0391
79. Chiou VL, Burotto M. Pseudoprogression and immune-related response in solid tumors. J Clin Oncol (2015) 33(31):3541–3. doi: 10.1200/JCO.2015.61.6870
80. Gerwing M, Herrmann K, Helfen A, Schliemann C, Berdel WE, Eisenblätter M, et al. The beginning of the end for conventional RECIST - novel therapies require novel imaging approaches. Nat Rev Clin Oncol (2019) 16(7):442–58. doi: 10.1038/s41571-019-0169-5
81. Basler L, Gabryś HS, Hogan SA, Pavic M, Bogowicz M, Vuong D, et al. Radiomics, tumor volume, and blood biomarkers for early prediction of pseudoprogression in patients with metastatic melanoma treated with immune checkpoint inhibition. Clin Cancer Res (2020) 26(16):4414–25. doi: 10.1158/1078-0432.CCR-20-0020
82. He S, Feng Y, Lin Q, Wang L, Wei L, Tong J, et al. CT-based peritumoral and intratumoral radiomics as pretreatment predictors of atypical responses to immune checkpoint inhibitor across tumor types: A preliminary multicenter study. Front Oncol (2021) 11:729371. doi: 10.3389/fonc.2021.729371
83. Vaidya P, Bera K, Patil PD, Gupta A, Jain P, Alilou M, et al. Novel, non-invasive imaging approach to identify patients with advanced non-small cell lung cancer at risk of hyperprogressive disease with immune checkpoint blockade. J Immunother Cancer (2020) 8(2). doi: 10.1136/jitc-2020-001343
84. Tunali I, Gray JE, Qi J, Abdalah M, Jeong DK, Guvenis A, et al. Novel clinical and radiomic predictors of rapid disease progression phenotypes among lung cancer patients treated with immunotherapy: An early report. Lung Cancer (2019) 129:75–9. doi: 10.1016/j.lungcan.2019.01.010
85. Gabryś HS, Basler L, Burgermeister S, Hogan S, Ahmadsei M, Pavic M, et al. PET/CT radiomics for prediction of hyperprogression in metastatic melanoma patients treated with immune checkpoint inhibitors. Front Oncol (2022) 12:977822. doi: 10.3389/fonc.2022.977822
86. Colen RR, Fujii T, Bilen MA, Kotrotsou A, Abrol S, Hess KR, et al. Radiomics to predict immunotherapy-induced pneumonitis: Proof of concept. Invest New Drugs (2018) 36(4):601–7. doi: 10.1007/s10637-017-0524-2
87. Curioni-Fontecedro A, Ickenberg C, Franzen D, Rogler G, Burger IA, van den Broek M. Diffuse pseudoprogression in a patient with metastatic non-small-cell lung cancer treated with nivolumab. Ann Oncol (2017) 28(8):2040–1. doi: 10.1093/annonc/mdx233
88. Galldiks N, Kocher M, Ceccon G, Werner JM, Brunn A, Deckert M, et al. Imaging challenges of immunotherapy and targeted therapy in patients with brain metastases: Response, progression, and pseudoprogression. Neuro Oncol (2020) 22(1):17–30. doi: 10.1093/neuonc/noz147
89. Gopalakrishnan D, Jain RK, Herbst L, Sikorski M, Mandava S, Azabdaftari G, et al. Case report: Immune checkpoint inhibitor-induced exuberant tumor inflammation with accelerated clinical deterioration in metastatic renal cell carcinoma. Front Oncol (2021) 11:679177. doi: 10.3389/fonc.2021.679177
90. Hodi FS, Ballinger M, Lyons B, Soria JC, Nishino M, Tabernero J, et al. Immune-modified response evaluation criteria in solid tumors (imRECIST): Refining guidelines to assess the clinical benefit of cancer immunotherapy. J Clin Oncol (2018) 36(9):850–8. doi: 10.1200/JCO.2017.75.1644
91. Kas B, Talbot H, Ferrara R, Richard C, Lamarque JP, Pitre-Champagnat S, et al. Clarification of definitions of hyperprogressive disease during immunotherapy for non-small cell lung cancer. JAMA Oncol (2020) 6(7):1039–46. doi: 10.1001/jamaoncol.2020.1634
92. Ferrara R, Mezquita L, Texier M, Lahmar J, Audigier-Valette C, Tessonnier L, et al. Hyperprogressive disease in patients with advanced non-small cell lung cancer treated with PD-1/PD-L1 inhibitors or with single-agent chemotherapy. JAMA Oncol (2018) 4(11):1543–52. doi: 10.1001/jamaoncol.2018.3676
93. Kim CG, Kim KH, Pyo KH, Xin CF, Hong MH, Ahn BC, et al. Hyperprogressive disease during PD-1/PD-L1 blockade in patients with non-small-cell lung cancer. Ann Oncol (2019) 30(7):1104–13. doi: 10.1093/annonc/mdz123
94. Saâda-Bouzid E, Defaucheux C, Karabajakian A, Coloma VP, Servois V, Paoletti X, et al. Hyperprogression during anti-PD-1/PD-L1 therapy in patients with recurrent and/or metastatic head and neck squamous cell carcinoma. Ann Oncol (2017) 28(7):1605–11. doi: 10.1093/annonc/mdx178
95. Champiat S, Ferrara R, Massard C, Besse B, Marabelle A, Soria JC, et al. Hyperprogressive disease: Recognizing a novel pattern to improve patient management. Nat Rev Clin Oncol (2018) 15(12):748–62. doi: 10.1038/s41571-018-0111-2
96. Champiat S, Besse B, Marabelle A. Hyperprogression during immunotherapy: do we really want to know? Ann Oncol (2019) 30(7):1028–31. doi: 10.1093/annonc/mdz184
97. Kim Y, Kim CH, Lee HY, Lee SH, Kim HS, Lee S, et al. Comprehensive clinical and genetic characterization of hyperprogression based on volumetry in advanced non-small cell lung cancer treated with immune checkpoint inhibitor. J Thorac Oncol (2019) 14(9):1608–18. doi: 10.1016/j.jtho.2019.05.033
98. Petrova MP, Donev IS, Radanova MA, Eneva MI, Dimitrova EG, Valchev GN, et al. Sarcopenia and high NLR are associated with the development of hyperprogressive disease after second-line pembrolizumab in patients with non-small-cell lung cancer. Clin Exp Immunol (2020) 202(3):353–62. doi: 10.1111/cei.13505
99. Kim SR, Chun SH, Kim JR, Kim SY, Seo JY, Jung CK, et al. The implications of clinical risk factors, CAR index, and compositional changes of immune cells on hyperprogressive disease in non-small cell lung cancer patients receiving immunotherapy. BMC Cancer (2021) 21(1):19. doi: 10.1186/s12885-020-07727-y
100. Frelaut M, Le Tourneau C, Borcoman E. Hyperprogression under immunotherapy. Int J Mol Sci (2019) 20(11). doi: 10.3390/ijms20112674
101. Reynolds KL, Arora S, Elayavilli RK, Louv WC, Schaller TH, Khandelwal A, et al. Immune-related adverse events associated with immune checkpoint inhibitors: A call to action for collecting and sharing clinical trial and real-world data. J Immunother Cancer (2021) 9(7). doi: 10.1136/jitc-2021-002896
102. Jing Y, Liu J, Ye Y, Pan L, Deng H, Wang Y, et al. Multi-omics prediction of immune-related adverse events during checkpoint immunotherapy. Nat Commun (2020) 11(1):4946. doi: 10.1038/s41467-020-18742-9
103. Mekki A, Dercle L, Lichtenstein P, Marabelle A, Michot JM, Lambotte O, et al. Detection of immune-related adverse events by medical imaging in patients treated with anti-programmed cell death 1. Eur J Cancer (2018) 96:91–104. doi: 10.1016/j.ejca.2018.03.006
104. Flavell RR, Evans MJ, Villanueva-Meyer JE, Yom SS. Understanding response to immunotherapy using standard of care and experimental imaging approaches. Int J Radiat Oncol Biol Phys (2020) 108(1):242–57. doi: 10.1016/j.ijrobp.2020.06.025
105. Collins GS, Reitsma JB, Altman DG, Moons KG. Transparent reporting of a multivariable prediction model for individual prognosis or diagnosis (TRIPOD): the TRIPOD statement. Bmj (2015) 350:g7594. doi: 10.1161/CIRCULATIONAHA.114.014508
106. Lambin P, Rios-Velazquez E, Leijenaar R, Carvalho S, van Stiphout RG, Granton P, et al. Radiomics: Extracting more information from medical images using advanced feature analysis. Eur J Cancer (2012) 48(4):441–6. doi: 10.1016/j.ejca.2011.11.036
107. Buckler AJ, Bresolin L, Dunnick NR, Sullivan DC. A collaborative enterprise for multi-stakeholder participation in the advancement of quantitative imaging. Radiology (2011) 258(3):906–14. doi: 10.1148/radiol.10100799
Keywords: radiomics, immunotherapy, precision medicine, pseudoprogression, hyperprogression
Citation: Zhou H, Luo Q, Wu W, Li N, Yang C and Zou L (2023) Radiomics-guided checkpoint inhibitor immunotherapy for precision medicine in cancer: A review for clinicians. Front. Immunol. 14:1088874. doi: 10.3389/fimmu.2023.1088874
Received: 03 November 2022; Accepted: 16 February 2023;
Published: 01 March 2023.
Edited by:
Qiang Tong, Renmin Hospital of Wuhan University, ChinaReviewed by:
Guozhu Hou, Chinese Academy of Medical Sciences and Peking Union Medical College, ChinaCopyright © 2023 Zhou, Luo, Wu, Li, Yang and Zou. This is an open-access article distributed under the terms of the Creative Commons Attribution License (CC BY). The use, distribution or reproduction in other forums is permitted, provided the original author(s) and the copyright owner(s) are credited and that the original publication in this journal is cited, in accordance with accepted academic practice. No use, distribution or reproduction is permitted which does not comply with these terms.
*Correspondence: Liqun Zou, em91bGlxdW4xOTcxQDE2My5jb20=
†These authors have contributed equally to this work and share first authorship
Disclaimer: All claims expressed in this article are solely those of the authors and do not necessarily represent those of their affiliated organizations, or those of the publisher, the editors and the reviewers. Any product that may be evaluated in this article or claim that may be made by its manufacturer is not guaranteed or endorsed by the publisher.
Research integrity at Frontiers
Learn more about the work of our research integrity team to safeguard the quality of each article we publish.