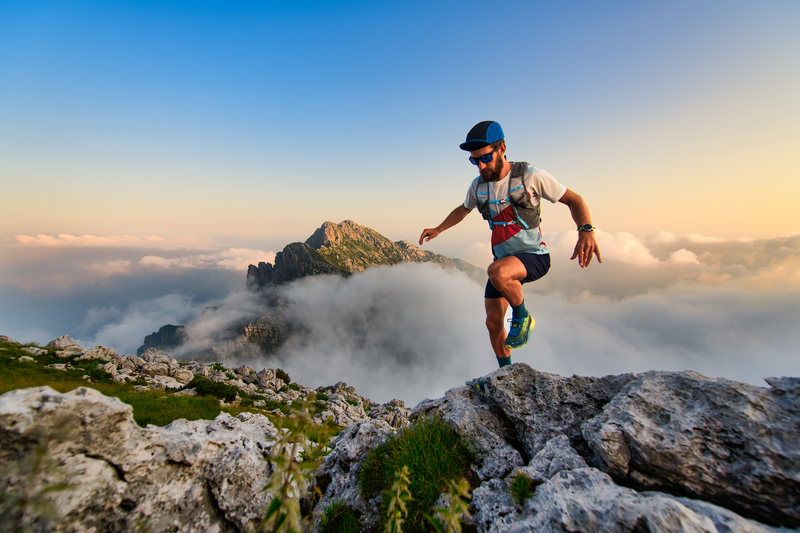
95% of researchers rate our articles as excellent or good
Learn more about the work of our research integrity team to safeguard the quality of each article we publish.
Find out more
REVIEW article
Front. Immunol. , 25 January 2023
Sec. Immunological Tolerance and Regulation
Volume 14 - 2023 | https://doi.org/10.3389/fimmu.2023.1083069
Liver cancer is one of the most common malignant tumors globally. Not only is it difficult to diagnose, but treatments are scarce and the prognosis is generally poor. Hepatocellular carcinoma (HCC) is the most common type of liver cancer. Aggressive cancer cells, such as those found in HCC, undergo extensive metabolic rewiring as tumorigenesis, the unique feature, ultimately causes adaptation to the neoplastic microenvironment. Intratumoral heterogeneity (ITH) is defined as the presence of distinct genetic features and different phenotypes in the same tumoral region. ITH, a property unique to malignant cancers, results in differences in many different features of tumors, including, but not limited to, tumor growth and resistance to chemotherapy, which in turn is partly responsible for metabolic reprogramming. Moreover, the different metabolic phenotypes might also activate the immune response to varying degrees and help tumor cells escape detection by the immune system. In this review, we summarize the reprogramming of glucose metabolism and tumoral heterogeneity and their associations that occur in HCC, to obtain a better understanding of the mechanisms of HCC oncogenesis.
Each year, the American Cancer Society investigates and publishes the number of new cancer cases in the United States. In 2021, the society projected that 1,898,160 new cancer cases of cancer would be reported in the United States, and that 608,570 cancer patients would die (1). Primary liver cancer is the most frequent cause of cancer death worldwide: its incidence is increasing year on year, and it is the currently the fifth most common type of cancer in the United States. Hepatocellular carcinoma (HCC), the predominant histologic type, accounts for 75%–85% of all liver cancer cases and is the most malignant (2). Intrahepatic cholangiocarcinoma (ICC) is another histologic type of liver cancer, accounting for 10%–15% of cases (3). The risk factors for HCC include chronic hepatitis B virus (HBV) and hepatitis C virus (HCV) infection, obesity, inappropriate aflatoxin intake, smoking, type 2 diabetes mellitus, and alcohol consumption (4). Of note, alcohol consumption is considered the most significant factor for its variables with levels of subjectivity, and the study shows that, even in quantities associated with social drinking, alcohol can potentially increase the risk of HCC development in patients with non-alcoholic steatohepatitis (NASH) or HCV cirrhosis when compared with subjects who do not drink (5). Moreover, early-stage HCC patients are often asymptomatic, but patients with advanced HCC usually miss the best opportunity for treatment, such as surgery or liver transplantation (6). Typical treatments for HCC patients include surgery, radiotherapy, chemotherapy, and targeted molecular therapy (7). For example, sorafenib, a multi-target tyrosine kinase inhibitor (TKI), was first approved by the Food and Drug Administration (FDA) for HCC treatment, and now lenvatinib, sorafenib, and other TKIs are also used in the treatment of HCC (8).
HCC originates from and develops in response to a series of crises, including metabolic, immunological, genetic, and microenvironmental pressures. The effects of these pressures vary over time and space in different states and regions of HCC, promoting the initial progress of HCC as a neoplastic microenvironment exhibiting enormous genetic and phenotypic intratumoral heterogeneity (ITH). Under other growing crises, various tumor cells and stromal components in the tumor microenvironment (TME) develop comparable degrees of ITH (9). Interestingly, genome sequencing has revealed another type of ITH, heterogeneity not in different tumor regions, which is known as spatial ITH, but heterogeneity over time in the same neoplasm, which is known as temporal ITH (10). Significantly, ITH affects both the genetic and epigenetic components of HCC; however, in HCC, these events can be closely connected (active genetic and epigenetic aberrations) or quite separate (stable genome with variable epigenetic modification) (11). In addition, ITH results in extensive metabolic rewiring in HCC throughout oncogenesis and development; this unique feature enables tumor cells to adapt to the neoplastic microenvironment. In addition, ITH leads to the same changes in non-malignant cellular compartments of the TME, such as hepatic stellate cells (HSCs), cancer-associated fibroblasts (CAFs), tumor-infiltrating lymphocytes (TILs), tumor-associated macrophages (TAMs), and dendritic cells (DCs) (12–14). Intriguingly, ITH affects the cellular compartments of TME for HCC malignancy. Conversely, cellular compartments undergoing such spatiotemporal transformation also impact the ITH of HCC (15). For example, although genetic and epigenetic aberrations of cancer cells affect TILs, TILs also influences the proliferation and progress of malignant tumors (16). Classically, ITH was considered a simple binomial state with two extremes (i.e., “on” or “off”) that determine the progression of an aggressive tumor (17). Recently, this description of ITH has been refined, and it is now thought that cellular components with a plastic phenotypic or metabolic state can continuously vary in response to disturbance of the microenvironment (18, 19). It is also thought that ITH can only be tolerated under certain thresholds. Otherwise, the phenotype and metabolic burden of malignant cells and the whole tumoral structure would be impaired (20, 21).
In the 1920s, Otto Warburg was the first to suggest that cancer cells undergo aerobic glycolysis, converting glucose into lactate in the cytoplasm, rather than oxidative phosphorylation (OXPHOS), even under conditions of normoxia (22). This led to the novel idea that tumor cells respond to emerging pressures in their microenvironment by undergoing metabolic rearrangements that promote their survival. This process, which is unique to cancer cells, resembles the Darwinian process of survival of the fittest. Aerobic glycolysis confers a growth advantage on HCC cells, by enhancing glucose uptake, speeding up adenosine triphosphate (ATP) generation, and producing abundant metabolic intermediates and an acidic environment (23). Moreover, increase of aerobic glycolysis in HCC leads to lactate acceleration, which causes a steady reduction in extracellular and intracellular pH. (24) The acidosis induces apoptosis and autophagy in healthy cells; modifies the stromal structure in such a way as to facilitate invasion and migration of HCC cells and metastasis; and also acts as a selection for HCC cells (25). However, OXPHOS also occurs in the mitochondria of HCC cells. According to the reverse Warburg effect, stromal cells such as CAFs support metabolism in cancer cells by releasing glucose-derived metabolites, such as lactate and pyruvate. CAFs utilize glucose in glycolysis and then transport intermediates for OXPHOS into HCC cells (26); OXPHOS is not entirely suppressed in HCC cells. It has been found that ubiquinol–cytochrome c reductase complex assembly factor 3 (UQCC3) is essential in maintaining mitochondrial homeostasis in structure and function, and in regulating OXPHOS activity under hypoxia in HCC cells (27). In an acidic microenvironment, glycolysis is inhibited and therefore, in glycolysis-dominant cells, such as HCC cells, pyruvate is transferred to the mitochondria for OXPHOS (28). The distinct metabolic preference is decided by genetic ITH, which confers plasticity on the metabolic demands of HCC. This transformation of HCC cells is mediated by epigenetic ITH, enablingeasier transition more easily between different cell states (11).
Metabolic reprogramming has been significantly linked with poor prognosis in HCC patients (29). It has consistently been found that genetic and epigenomic ITH affects metabolic rewiring in HCC and predicts poorer outcomes (21). A better comprehension of aerobic glycolysis and ITH and their association with HCC would shed light on the pathogenesis and evolution of HCC. This article aims to summarize the correlation between ITH and metabolic rearrangements (predominantly aerobic glycolysis) and the comprehensive characteristics of the respective biological properties of HCC.
ITH was first proposed in 1833 by Johannes Muller, 300 years after the microscope was invented (30). This German physiologist utilized microscopy to analyze human tumor samples, imitating methods in phytology, and described tumors as agglomerations of emerging cells, which showed distinct variations among different regions and also differences between tumor cells and adjacent stromal cells (31). ITH has now been unequivocally proven to be present in many cancers. It encompasses dynamic aspects, such as genetic and epigenetic heterogeneity, which can be further classified as transcriptomic, proteomic, and phenotypic heterogeneity, as well as behavioral and immunological heterogeneity, which includes temporal heterogeneity, spatial heterogeneity, and heterogeneity of the TME (metabolic and immunological components), and leads to heterogeneous behavior of tumor cells and changes in the immune responses of immune cells (32).
Analysis of genetic ITH, involving next-generation sequencing (NGS) studies of the tumoral genetic landscape, have demonstrated that spatiotemporal aberration is caused by genetic instability (20, 33, 34). Genetic instability is reflected in point mutations, short insertions or deletions, copy number variation, and chromosomal alterations, including translocation, deletion, amplification, and aneuploidy (30, 35–37). During the initial development of HCC, only the fittest clones survive clonal pressure and immunological selection and accumulate in cancer cells (38, 39). The diversity of surviving cells, which vary in terms of genetic instability, immune escape, and treatment resistance, is considered the reason for gene heterogeneity in HCC and confers a growth and evolutionary advantage on HCC cells (40–42). High genetic ITH is normally associated with tumor aggressiveness, immune escape, and resistance to treatment, leading to poor outcomes and a worse prognosis for HCC patients (12, 15). Understanding genetic ITH could enable more tailored tumor treatment and could also constitute a negative prognostic marker for HCC patients (43, 44). Epigenetic heterogeneity is regarded as an essential regulator for tumor evolution and development. Generally, epigenetic alterations that induce non-heritable changes in cell clones are reversible, but it is possible that they could be acquired by cell progeny, leading to heritable expression and the progression of cancers (30, 45). One of the most common heritable epigenetic alterations is DNA methylation (46). However, there is no direct evidence that DNA methylation drives cancer initiation in human patients. To date, in vivo experiments have shown that targeting the p16Ink4a epimutation drives tumor origination and aggressive development, and attenuates survival time, in mice (47). Furthermore, demethylation of satellite 2 has been found to correlate with chromosome aneuploidy induced by 5-azacytidine (48) (Figure 1). DNA methylation has also been shown to be temporally altered in advanced lung cancer (12).
Figure 1 Genetic and epigenetic ITH in HCC. During the initial and subsequent development of HCC, only the fittest clones survive clonal pressure and immunological selection and accumulate in cancer cells. The diversity of surviving cells, which vary in genetic instability, immune escape, and degree of treatment resistance, is considered one reason for the genetic heterogeneity of HCC, which confers on HCC an evolutionary growth advantage. Enhanced genetic ITH normally leads to aggressiveness, immune escape, and resistance to treatment, which is associated poor outcomes and worse prognosis in HCC patients.
As mentioned before, genetic and epigenetic heterogeneity, mainly transcriptomic and proteomic ITH, result in functional heterogeneity, and, together with spatiotemporal remodeling of the TME and changes in metabolic and immunological function, promote behavioral and immunological heterogeneity (49–52) (Figure 2). The tumoral ecosystem resembles Darwinian evolution in cancers, which drives HCC behavioral and immunological heterogeneity. Research on lung cancer shows that non-heritable ITH, in contrast to heritable ITH, indicates phenotypic heterogeneity, and predicts ongoing process dynamics (53). Under the comparative topologies of dendrograms at the genomic and transcriptomic levels, functional ITH has been found to affect proliferative properties, epithelial or mesenchymal features, and clinical and histologic subtypes (53). Similarly, behavioral ITH affects the proliferation, invasiveness, and metabolic phenotypes of HCC. Immunological ITH, in contrast, refers to differences in immunogenicity, adjuvanticity, and immune escape, which determine whether or not tumor cells belong to heterobiotics, activate the immune response, and escape detection by the immune system. (54) Interestingly, the research applies multiregional genomic and immunological landscapes on a single HCC and displays a significant degree of immunological ITH in HCC, which is associated with tumoral transcriptomic ITH, especially malignancy and development. Mechanistically, immune stroma with augmented immunological ITH exhibits attenuated immune selective pressure, which confers on HCC cells the capacity to evade or suppress the immune system (55). Coincidentally, another publication has reported that multipoint biopsy sampling can completely restore ITH at both inheritable and non-heritable levels, helping us to understand the interactions between immunological ITH and tumor evolution (56). Histologic analysis of the different regions of HCC revealed immune infiltration in the abnormal tumor regions. In particular, HCC regions exhibiting transcriptomic ITH reveal the heterogeneity of tumor-infiltrating lymphocytes (TILs), mainly T-cell infiltrating via B- and T-cell receptor (B/TCR) RNA sequencing reads mapping to VDJ loci to assess the degree and characteristics of TIL burden (54). Moreover, the article concludes that passenger mutation, which offers no survival advantage, gathers more TILs than driver mutation, which confers a survival advantage (40, 54). Single-cell RNA sequencing (scRNA seq) and cytometry by time of flight (CyTOF) have been used to examine stromal TILs, especially some types of T cells, and their locations relative to cancer cells (57). T cells can be crudely classified as CD4+ or CD8+ T cells based on the type of glycoprotein found on the cell surface. Subsets of CD4+ cells comprise conventional T cells (Tconv cells), T follicular helper cells (TFH cells), regulatory T cells (Treg cells), dysfunctional T cells, naive T cells, effector T cells (TE cells), and memory T cells (TM cells) (Table 1). CD8+ T cells include naive T cells, TE cells, TM cells, and dysfunctional T cells, subdivided according to their level of differentiation (58, 59, 63–67). Dysfunctional T cells are described as dysfunctional or exhausted because they express a higher than usual number of inhibitory receptors such as the programmed cell death protein 1 (PD1) receptor, T-cell immunoglobulin mucin receptor 3 (TIM3), and receptors for lymphocyte activation gene 3 protein (LAG3), cytotoxic T lymphocyte-associated antigen 4 (CTLA4), CD200, and 2B4, and secrete lower than normal levels of cytokines such as tumor necrosis factor (TNF), interleukin 2 (IL-2), and interferon γ (IFNγ) (60–62). Even exhausted T cells demonstrate heterogeneity, but they are not found in all cancer types (68). However, studies of lung cancer and HCC cells have confirmed the presence of exhausted T cells with augmented expression of inhibitory receptors, including receptors for PD1 (encoded by the PDCD1 gene), CTLA4, and LAG3 (65, 67).
Figure 2 Behavioral and immunological ITH in HCC. Spatiotemporal remodeling of the TME, and especially of its metabolic and immunological components, promotes behavioral and immunological heterogeneity.
Intriguingly, scRNA seq has shown that, in contrast to CD8+ T cells, CD4+ T cells are not found in the stroma of all tumors. Unlike CD8+ T cells, which have a distinct role in cancers, which is to drive carcinomatosis, CD4+ T cells play varying roles in the process of tumor promotion (either oncogenesis or carcinomatosis) (69). It has been reported that CD4+ T-cell apoptosis, which can be induced by linoleic acid, which enhances the expression of carnitine palmitoyl transferase (CPT), accelerates the development of HCC (70). Other reports also confirm the inhibitory significance of CD4+ T cells in carcinogenesis and suggest that the presence of these cells is an independent prognostic marker in HCC (71, 72). These multiple features of T cells and their secretions significantly contribute to immunological ITH in HCC. Remarkably, immunological ITH is not a binary state; TILs in the tumoral stroma represent gradual, continuous, and highly plastic phenotypes for the varying TMEs. Traditionally, experts categorize TAMs found in stromal compartments as proinflammatory (M1-like) TAMs or anti-inflammatory (M2-like) TAMs, which is a relatively simple classification obscuring the complexity and plasticity of TAMs (18). The relative proportions of different TAM subpopulations and their cellular states, surface protein expression, and individual secretions account in large part for immunological ITH in HCC stroma (63, 73).
A well-known classification divides tumors into two categories based on their sensitivity to immunological therapy: “hot” tumors are generally sensitive to immunological therapy and contain abundant CD8+ cytotoxic T lymphocytes (CTLs), whereas “cold” tumors are significantly resistant to immunological treatment and contain limited numbers of CTLs (74). The progression and clinical stage of tumors are closely linked to the presence of CTLs, and the Immunoscore, a standardized method of quantification of CTLs in the TME, as a reflection of a tumor’s immunological state, has been validated as a measure of tumor aggressiveness and prognosis (74–77). Subsequently, a third immune profile, so-called “altered” states, was identified in colorectal cancer (CRC) in 2009 (78). The “altered” profile has since been split into two phenotypes: the “excluded” phenotype (reflecting the fact that CTLs are excluded at the edge of the invasive margin by the dense stroma) and the “immunosuppressed” phenotype (reflecting the fact that the tumor contains a small number of CTLs, which represent not a stromal barrier but an immunosuppressive TME) (79, 80).
The previous study on HCC, which integrated data from RNA-seq, TCR-seq, single-nucleotide polymorphism (SNP) array, and DNA-seq analyses across several regions in the same HCC specimen, illustrates a significant immunological ITH signature (54). The same patient (P02) exhibited diverse tumor states, including immune “hot” phenotypes with poor differentiation and immunogenicity (H2.a), immune “cold” phenotypes with good differentiation (H2.b, H2.c, and H2.d), and an intermediate phenotype (H2.e). The results agree with the previous concept that transcriptomic ITH is strongly associated with immunological ITH in HCC. Moreover, regional ITH in the same patient represents different clinical phenotypes and evolutional stages, reflecting spatiotemporal evolution. A better understanding of behavioral ITH and immunological ITH in HCC, and how ITH manipulates phenotypic arrangements under different TMEs, would contribute to the immunotherapy revolution and provide new directions in molecular treatment.
Aerobic glycolysis was first proposed in rat liver cancer as the Warburg effect in the 1920s; researchers observed that cancer tissue consumes less oxygen (O2) than normal tissue. In other words, HCC converts glucose flux product (pyruvate) into lactate in the cytoplasm rather than transporting pyruvate into mitochondria for use in the Krebs cycle (Figure 3). This distinct feature of HCC is probably related to metabolic reprogramming and metabolic ITH. Of note, metabolic plasticity ensures that HCC has strong adaptability in nutrient-deprived TMEs through increasing scavenging pathways, including autophagy, apoptosis, and reverse Warburg effects associated with CAFs, generating intermediate products for metabolic utilization (81, 82). In general, aerobic glycolysis is essential during inception, growth, proliferation, invasion, and immune evasion. This paper promotes a better understanding of glycolysis and ITH in HCC, in particular its pathogenesis, detection, and molecular treatment.
Figure 3 The heterogeneity of glycolysis in HCC. Metabolic ITH is exemplified by glycolysis in HCC. PHI can enhance the ability of HCC cell lines to penetrate Matrigel by activating β1 integrins lines. Overexpression of PFKFB3 results in sorafenib resistance, and elevated PFKFB3 is generally associated with poor outcomes and worse clinical manifestations in HCC. Among PKs, PKM2 is considerably up-regulated in HCC patients, and this is associated with a poor prognosis. Overexpression of PKM2 results in an increase in IFNγ-positive CD8+ T cells in the HCC mouse model by activating the immune checkpoint blockade.
Aggressive cells (such as HCC and pancreatic cancer cells) undergo extensive metabolic rewiring throughout their development. Metabolic reprogramming of glucose, lipid, and amino acids is found in both in situ and metastatic HCC; metabolites and Taylorism produced from reprogramming processes are necessary for energy production and anabolism, including cellular membranes, nucleotides, extracellular matrix (ECM), and cell cytoskeleton, which are fundamental for proliferation, invasion, and metastasis (83–86) (Table 2). Because glucose uptake is enhanced in HCC, the expression of glucose transporter 1 (GLUT-1), as a membrane channel for glucose, also increases. This unique feature, i.e., augmented glucose uptake, can be exploited to enable the early detection of HCC and its systemic metastases by positron emission tomography/computed tomography, by replacing glucose with fluorodeoxyglucose F 18 (18F-FDG), a glucose derivative used as a radiotracer. (96) The expression of GLUT-1 has been shown to be augmented in the stained membranes of HCC cells, and thus the level of expression of GLUT-1 could be used to evaluate liver lesions (87). In addition, miR-505 (micro-RNA-505) down-regulation of GLUT-1 expression attenuates glucose uptake and lactate generation in HCC cells. Together, miR-505 impairs HCC growth by inhibiting the expression of insulin-like growth factor 1 receptor (IGF-1R) and damaging glycolysis in HCC cells (88).
Hexokinase 2 (HK2) is an essential enzyme in aerobic glycolysis, catalyzing the conversion of glucose to glucose 6-phosphate (G-6-P). Silencing HK2 reduces glucose flux to pyruvate and lactate (glycolysis), but has no effect on the tricarboxylic acid (TCA) cycle (OXPHOS). Depletion of HK2, along with sorafenib, sensitizes HCC cells to cell death and inhibits the mammalian target of rapamycin (mTORC1), together with metformin (89). G-6-P is then converted to fructose 6-phosphate (F-6-P), a reaction that is catalyzed by phosphohexose isomerase (PHI), also known as autocrine motility factor (AMF), phosphoglucose isomerase (PGI), or glucose-6-phosphate isomerase (GPI). PHI (AMF) not only catalyzes reactions during glycolysis, but also acts as a cytokine, inducing invasion and metastasis. PHI binds to its receptor, Mr 78,000 glycoprotein (gp78), and promotes matrix metalloproteinase 2 (MMP-2) secretion, adhesion, and motility, thus enhancing the invasive ability of HCC cell lines, enabling them to penetrate Matrigel® (Corning Life Sciences, Corning, NY, USA) by activating β1 integrins (90). Phosphofructokinase (PFK) converts F-6-P to fructose 1,6-biphosphate (F-1,6-BP), which is the second rate-limiting step in glycolysis. PFK is activated by fructose-2,6-biphosphate (F-2,6-BP), the most allosteric activator for PFK. Moreover, F-2,6-BP is catalyzed by phosphofructo-2-kinase/fructose-2,6-biphosphatase (PFK-2/PFKFB), which has two separate catalytic centers. Specifically, PFKFB3, having the strongest phosphofructo-2-kinase activity, is typically up-regulated in cancers, including HCC (91, 92). Overexpression of PFKFB3 and the high flux of glycolysis contribute to sorafenib resistance, and could be targeted by aspirin, as aspirin, in combination with sorafenib, induces apoptosis (91). Elevated PFKFB3 is generally associated with poor outcomes and worse clinical manifestations; therefore, regulating PFKFB3 could not merely inhibit the activity of PFK to target glycolysis, more than arrest cell cycle and cell death in HCC. F-1,6-BP subsequently decomposes into 3-phosphoglyceraldehyde (GA3P) and dihydroxyacetone phosphate (DHAP). Subsequently, a series of reactions catalyzed by enzymes, such as triose phosphate isomerase (TPI), glyceraldehyde 3-phosphate dehydrogenase (GAPDH), phosphoglycerate mutase (PGAM), and enolase, convert intermediates to produce phosphoenolpyruvate (PEP). Interestingly, GAPDH is generally used as a reference in quantitative reverse transcription–polymerase chain reaction (qRT-PCR) and Western blotting because of its stable expression during different states. However, immunohistochemical staining of HCC tissue shows that GAPDH in the nucleus is positively associated with the hypoxia-inducible factor (HIF-1α). Despite this, patients with overexpressed HIF-1α or low levels of GAPDH have a poor prognosis and low overall survival (OS) (93). Following this, PEP is catalyzed to pyruvate by pyruvate kinase (PK) and produces ATP, which is the last committed step in glycolysis. Among PKs, PKM2 is notably up-regulated in HCC patients and a high level of PKM2 is associated with a poor prognosis. Silencing PKM2 inhibits HCC proliferation, migration, and invasion whereas overexpression of PKM2 increases interferon gamma (IFNγ)-positive CD8+ T cells in the HCC mouse model by activating an immune checkpoint blockade (94). Intermediate F-1,6-BP is an allosteric activator of PKM2, whereas ATPacetyl-coenzyme A (CoA), and L-cysteine are allosteric inhibitors of PKM2 (97, 98). Shikonin is a specific inhibitor of PKM2, demonstrating carcinomatosis in HCC. In addition, shikonin In addition, shikonin promotes nuclear localization of PKM2 to recruit Nrf2, then activates BAG3 downstream of Nrf2 to provide a protective effect for cell survival (99). The final step in glycolysis is the conversion of pyruvate to L-lactate, catalyzed by lactate dehydrogenase (LDH). It has been suggested that the measurement of LDH could predict clinical outcomes such as progression-free survival (PFS) and OS in HCC patients treated with sorafenib (95).
A better understanding of glycolysis could not only shed light on the metabolic frame in HCC, but also uncover the relationship between metabolic ITH and immunological ITH, and its evolution in HCC. Indeed, metabolic ITH in aggressive cancers seems to regulate immunological ITH and promote phenotypic transformation. Notably, increased lactate dehydrogenase A (LDHA) is related to poor outcomes in HCC patients and is negatively associated with markers of CTLs. Moreover, lactate dehydrogenase A (LDHA)-related lactate accumulating in the TME creates an acidic stroma favoring the transport of substrates such as glucose and their uptake by HCC cells (100). It has consistently been found that lactate, together with an acidic microenvironment, suppresses some functions of immune cells and changes their morphology, thus reducing their survival. More precisely, lactic acid increases inhibitors’ immunosurveillance by attenuating the survival and function of T and NK cells, leading to the immune escape of tumor cells and a more malignant phenotype (101). Increasing evidence suggests that malignant cancers undergo metabolic adjustment in different TMEs to satisfy growth demands at every stage of the metastatic cascade. According to this view, metabolic flexibility (the use of the same metabolites at different stages of metastasis) and metabolic plasticity (the use of other metabolites that can satisfy identical demands during the metastatic cascade), which are notable properties of aggressive cancers, account for metabolic ITH in variable microenvironments and confer a growth advantage on aggressive cells when the TME is changeable (102). The metabolic profile reflects both the glycolytic phenotype (related to chemosensitivity and rapid proliferation) and the oxidative phenotype (associated with chemoresistance and late proliferation) coexist in glioblastoma, which definitively shows that metabolic ITH exists and is connected to the progression of aggressive cancers.
To summarize, we have described the epidemiology of HCC and reported risk factors for HCC and worldwide morbidity. We then summarized ITH in HCC, including genetic ITH, epigenetic ITH, behavioral ITH, and immunological ITH. Of these, we mainly discussed metabolic ITH and immunological ITH and their role in the progression of ITH. Of note, metabolic ITH, particularly the glycolytic and oxidative phenotypes, is common in HCC. We hope that our findings will enable heterogeneity of the tumoral ecosystem and the resulting metabolic adaptation of tumor cells to be exploited in order to develop novel therapeutic approaches for HCC patients.
F-QX and M-MD contributed equally to this work. F-QX and M-MD contributed to the conception and design of the study. F-QX and M-MD wrote the first draft of the manuscript. Z-FW and L-DC wrote sections of the manuscript and provided critical revisions. All authors contributed to the article and approved the submitted version.
The authors declare that the research was conducted in the absence of any commercial or financial relationships that could be construed as a potential conflict of interest.
All claims expressed in this article are solely those of the authors and do not necessarily represent those of their affiliated organizations, or those of the publisher, the editors and the reviewers. Any product that may be evaluated in this article, or claim that may be made by its manufacturer, is not guaranteed or endorsed by the publisher.
1. Siegel RL, Miller KD, Fuchs HE, Jemal A. Cancer statistics, 2021. CA Cancer J Clin (2021) 71(1):7–33. doi: 10.3322/caac.21654
2. Bray F, Ferlay J, Soerjomataram I, Siegel RL, Torre LA, Jemal A. Global cancer statistics 2018: GLOBOCAN estimates of incidence and mortality worldwide for 36 cancers in 185 countries. CA Cancer J Clin (2018) 68(6):394–424. doi: 10.3322/caac.21492
3. Stavraka C, Rush H, Ross P. Combined hepatocellular cholangiocarcinoma (cHCC-CC): an update of genetics, molecular biology, and therapeutic interventions. J Hepatocell Carcinoma (2019) 6:11–21. doi: 10.2147/JHC.S159805
4. Yu MC, Yuan JM. Environmental factors and risk for hepatocellular carcinoma. Gastroenterology (2004) 127(5 Suppl 1):S72–8. doi: 10.1016/j.gastro.2004.09.018
5. Ascha MS, Hanouneh IA, Lopez R, Tamimi TA, Feldstein AF, Zein NN. The incidence and risk factors of hepatocellular carcinoma in patients with nonalcoholic steatohepatitis. Hepatology (2010) 51(6):1972–8. doi: 10.1002/hep.23527
6. Lee M, Ko H, Yun M. Cancer metabolism as a mechanism of treatment resistance and potential therapeutic target in hepatocellular carcinoma. Yonsei Med J (2018) 59(10):1143–9. doi: 10.3349/ymj.2018.59.10.1143
7. Sia D, Villanueva A, Friedman SL, Llovet JM. Liver cancer cell of origin, molecular class, and effects on patient prognosis. Gastroenterology (2017) 152(4):745–61. doi: 10.1053/j.gastro.2016.11.048
8. Connell LC, Harding JJ, Abou-Alfa GK. Advanced hepatocellular cancer: the current state of future research. Curr Treat Opt Oncol (2016) 17(8):43. doi: 10.1007/s11864-016-0415-3
9. Vitale I, Sistigu A, Manic G, Rudqvist NP, Trajanoski Z, Galluzzi L. Mutational and antigenic landscape in tumor progression and cancer immunotherapy. Trends Cell Biol (2019) 29(5):396–416. doi: 10.1016/j.tcb.2019.01.003
10. Wang J, Cazzato E, Ladewig E, Frattini V, Rosenbloom DI, Zairis S, et al. Clonal evolution of glioblastoma under therapy. Nat Genet (2016) 48(7):768–76. doi: 10.1038/ng.3590
11. Flavahan WA, Gaskell E, Bernstein BE. Epigenetic plasticity and the hallmarks of cancer. Science (2017) 357(6348). doi: 10.1126/science.aal2380
12. Vitale I, Shema E, Loi S, Galluzzi L. Intratumoral heterogeneity in cancer progression and response to immunotherapy. Nat Med (2021) 27(2):212–24. doi: 10.1038/s41591-021-01233-9
13. Costa A, Kieffer Y, Scholer-Dahirel A, Pelon F, Bourachot B, Cardon M, et al. Fibroblast heterogeneity and immunosuppressive environment in human breast cancer. Cancer Cell (2018) 33(3):463–79.e10. doi: 10.1016/j.ccell.2018.01.011
14. Aoki T, Chong LC, Takata K, Milne K, Hav M, Colombo A, et al. Single-cell transcriptome analysis reveals disease-defining T-cell subsets in the tumor microenvironment of classic Hodgkin lymphoma. Cancer Discovery (2020) 10(3):406–21. doi: 10.1158/2159-8290.CD-19-0680
15. Rosenthal R, Cadieux EL, Salgado R, Bakir MA, Moore DA, Hiley CT, et al. Neoantigen-directed immune escape in lung cancer evolution. Nature (2019) 567(7749):479–85. doi: 10.1038/s41586-019-1032-7
16. Zhang AW, McPherson A, Milne K, Kroeger DR, Hamilton PT, Miranda A, et al. Interfaces of malignant and immunologic clonal dynamics in ovarian cancer. Cell (2018) 173(7):1755–69.e22. doi: 10.1016/j.cell.2018.03.073
17. Cao L, Wu J, Qu X, Sheng J, Cui M, Liu S, et al. Glycometabolic rearrangements–aerobic glycolysis in pancreatic cancer: causes, characteristics and clinical applications. J Exp Clin Cancer Res (2020) 39(1):267. doi: 10.1186/s13046-020-01765-x
18. Lambrechts D, Wauters E, Boeckx B, Aibar S, Nittner D, Burton O, et al. Phenotype molding of stromal cells in the lung tumor microenvironment. Nat Med (2018) 24(8):1277–89. doi: 10.1038/s41591-018-0096-5
19. Neftel C, Laffy J, Filbin MG, Hara T, Shore ME, Rahme GJ, et al. An integrative model of cellular states, plasticity, and genetics for glioblastoma. Cell (2019) 178(4):835–49.e21. doi: 10.1016/j.cell.2019.06.024
20. Andor N, Maley CC, Ji HP. Genomic instability in cancer: Teetering on the limit of tolerance. Cancer Res (2017) 77(9):2179–85. doi: 10.1158/0008-5472.CAN-16-1553
21. Lin DC, Mayakonda A, Dinh HQ, Huang P, Lin L, Liu X, et al. Genomic and epigenomic heterogeneity of hepatocellular carcinoma. Cancer Res (2017) 77(9):2255–65. doi: 10.1158/0008-5472.CAN-16-2822
22. Warburg O, Minami S. Versuche an Überlebendem carcinom-gewebe. Klinische Wochenschrift (1923) 2(17):776–7. doi: 10.1007/BF01712130
23. Gatenby RA, Gawlinski ET. The glycolytic phenotype in carcinogenesis and tumor invasion: Insights through mathematical models. Cancer Res (2003) 63(14):3847–54.
24. Gatenby RA, Gillies RJ. Why do cancers have high aerobic glycolysis? Nat Rev Cancer (2004) 4(11):891–9. doi: 10.1038/nrc1478
25. Bose S, Le A. Glucose metabolism in cancer. Adv Exp Med Biol (2018) 1063:3–12. doi: 10.1007/978-3-319-77736-8_1
26. Pavlides S, Tsirigos A, Vera I, Flomenberg N, Frank PG, Casimiro MC, et al. Transcriptional evidence for the "Reverse warburg effect" in human breast cancer tumor stroma and metastasis: Similarities with oxidative stress, inflammation, alzheimer's disease, and "Neuron-glia metabolic coupling". Aging (Albany NY). (2010) 2(4):185–99. doi: 10.18632/aging.100134
27. Yang Y, Zhang G, Guo F, Li Q, Luo H, Shu Y, et al. Mitochondrial UQCC3 modulates hypoxia adaptation by orchestrating OXPHOS and glycolysis in hepatocellular carcinoma. Cell Rep (2020) 33(5):108340. doi: 10.1016/j.celrep.2020.108340
28. Bencze G, Bencze S, Rivera KD, Watson JD, Hidvegi M, Orfi L, et al. Mito-oncology agent: fermented extract suppresses the warburg effect, restores oxidative mitochondrial activity, and inhibits in vivo tumor growth. Sci Rep (2020) 10(1):14174. doi: 10.1038/s41598-020-71118-3
29. Satriano L, Lewinska M, Rodrigues PM, Banales JM, Andersen JB. Metabolic rearrangements in primary liver cancers: Cause and consequences. Nat Rev Gastroenterol Hepatol (2019) 16(12):748–66. doi: 10.1038/s41575-019-0217-8
30. Zellmer VR, Zhang S. Evolving concepts of tumor heterogeneity. Cell Biosci (2014) 4:69. doi: 10.1186/2045-3701-4-69
31. Hajdu SI. A note from history: The first tumor pathologist. Ann Clin Lab Sci (2004) 34(3):355–6.
32. Gaiti F, Chaligne R, Gu H, Brand RM, Kothen-Hill S, Schulman RC, et al. Epigenetic evolution and lineage histories of chronic lymphocytic leukaemia. Nature (2019) 569(7757):576–80. doi: 10.1038/s41586-019-1198-z
33. McGranahan N, Swanton C. Clonal heterogeneity and tumor evolution: Past, present, and the future. Cell (2017) 168(4):613–28. doi: 10.1016/j.cell.2017.01.018
34. Hanahan D, Weinberg RA. Hallmarks of cancer: the next generation. Cell (2011) 144(5):646–74. doi: 10.1016/j.cell.2011.02.013
35. Flintoft L. Explaining aneuploidy patterns. Nat Rev Genet (2013) 14(12):825–. doi: 10.1038/nrg3634
36. Jamal-Hanjani M, Wilson GA, McGranahan N, Birkbak NJ, Watkins TBK, Veeriah S, et al. Tracking the evolution of non-Small-Cell lung cancer. N Engl J Med (2017) 376(22):2109–21. doi: 10.1056/NEJMoa1616288
37. Raynaud F, Mina M, Tavernari D, Ciriello G. Pan-cancer inference of intra-tumor heterogeneity reveals associations with different forms of genomic instability. PloS Genet (2018) 14(9):e1007669. doi: 10.1371/journal.pgen.1007669
38. Salmon H, Remark R, Gnjatic S, Merad M. Host tissue determinants of tumour immunity. Nat Rev Cancer (2019) 19(4):215–27. doi: 10.1038/s41568-019-0125-9
39. Teixeira VH, Pipinikas CP, Pennycuick A, Lee-Six H, Chandrasekharan D, Beane J, et al. Deciphering the genomic, epigenomic, and transcriptomic landscapes of pre-invasive lung cancer lesions. Nat Med (2019) 25(3):517–25. doi: 10.1038/s41591-018-0323-0
40. Hu Z, Li Z, Ma Z, Curtis C. Multi-cancer analysis of clonality and the timing of systemic spread in paired primary tumors and metastases. Nat Genet (2020) 52(7):701–8. doi: 10.1038/s41588-020-0628-z
41. Keenan TE, Burke KP, Van Allen EM. Genomic correlates of response to immune checkpoint blockade. Nat Med (2019) 25(3):389–402. doi: 10.1038/s41591-019-0382-x
42. Juric D, Castel P, Griffith M, Griffith OL, Won HH, Ellis H, et al. Convergent loss of PTEN leads to clinical resistance to a PI(3)Kα inhibitor. Nature (2015) 518(7538):240–4. doi: 10.1038/nature13948
43. Andor N, Graham TA, Jansen M, Xia LC, Aktipis CA, Petritsch C, et al. Pan-cancer analysis of the extent and consequences of intratumor heterogeneity. Nat Med (2016) 22(1):105–13. doi: 10.1038/nm.3984
44. Morris LG, Riaz N, Desrichard A, Şenbabaoğlu Y, Hakimi AA, Makarov V, et al. Pan-cancer analysis of intratumor heterogeneity as a prognostic determinant of survival. Oncotarget (2016) 7(9):10051–63. doi: 10.18632/oncotarget.7067
45. Patten DK, Corleone G, Győrffy B, Perone Y, Slaven N, Barozzi I, et al. Enhancer mapping uncovers phenotypic heterogeneity and evolution in patients with luminal breast cancer. Nat Med (2018) 24(9):1469–80. doi: 10.1038/s41591-018-0091-x
46. Esteller M. Cancer epigenomics: DNA methylomes and histone-modification maps. Nat Rev Genet (2007) 8(4):286–98. doi: 10.1038/nrg2005
47. Yu DH, Waterland RA, Zhang P, Schady D, Chen MH, Guan Y, et al. Targeted p16(Ink4a) epimutation causes tumorigenesis and reduces survival in mice. J Clin Invest (2014) 124(9):3708–12. doi: 10.1172/JCI76507
48. Prada D, González R, Sánchez L, Castro C, Fabián E, Herrera LA. Satellite 2 demethylation induced by 5-azacytidine is associated with missegregation of chromosomes 1 and 16 in human somatic cells. Mutat Res (2012) 729(1-2):100–5. doi: 10.1016/j.mrfmmm.2011.10.007
49. Bolouri H. Network dynamics in the tumor microenvironment. Semin Cancer Biol (2015) 30:52–9. doi: 10.1016/j.semcancer.2014.02.007
50. Buczak K, Ori A, Kirkpatrick JM, Holzer K, Dauch D, Roessler S, et al. Spatial tissue proteomics quantifies inter- and intratumor heterogeneity in hepatocellular carcinoma (HCC). Mol Cell Proteomics (2018) 17(4):810–25. doi: 10.1074/mcp.RA117.000189
51. Furuta M, Ueno M, Fujimoto A, Hayami S, Yasukawa S, Kojima F, et al. Whole genome sequencing discriminates hepatocellular carcinoma with intrahepatic metastasis from multi-centric tumors. J Hepatol (2017) 66(2):363–73. doi: 10.1016/j.jhep.2016.09.021
52. Zhang Q, Lou Y, Yang J, Wang J, Feng J, Zhao Y, et al. Integrated multiomic analysis reveals comprehensive tumour heterogeneity and novel immunophenotypic classification in hepatocellular carcinomas. Gut (2019) 68(11):2019–31. doi: 10.1136/gutjnl-2019-318912
53. Sharma A, Merritt E, Hu X, Cruz A, Jiang C, Sarkodie H, et al. Non-genetic intra-tumor heterogeneity is a major predictor of phenotypic heterogeneity and ongoing evolutionary dynamics in lung tumors. Cell Rep (2019) 29(8):2164–74.e5. doi: 10.1016/j.celrep.2019.10.045
54. Losic B, Craig AJ, Villacorta-Martin C, Martins-Filho SN, Akers N, Chen X, et al. Intratumoral heterogeneity and clonal evolution in liver cancer. Nat Commun (2020) 11(1):291. doi: 10.1038/s41467-019-14050-z
55. Nguyen PHD, Ma S, Phua CZJ, Kaya NA, Lai HLH, Lim CJ, et al. Intratumoural immune heterogeneity as a hallmark of tumour evolution and progression in hepatocellular carcinoma. Nat Commun (2021) 12(1):227. doi: 10.1038/s41467-020-20171-7
56. McGranahan N, Swanton C. Cancer evolution constrained by the immune microenvironment. Cell (2017) 170(5):825–7. doi: 10.1016/j.cell.2017.08.012
57. Zhang L, Yu X, Zheng L, Zhang Y, Li Y, Fang Q, et al. Lineage tracking reveals dynamic relationships of T cells in colorectal cancer. Nature (2018) 564(7735):268–72. doi: 10.1038/s41586-018-0694-x
58. Ahrends T, Borst J. The opposing roles of CD4(+) T cells in anti-tumour immunity. Immunology (2018) 154(4):582–92. doi: 10.1111/imm.12941
59. Qin L, Waseem TC, Sahoo A, Bieerkehazhi S, Zhou H, Galkina EV, et al. Insights into the molecular mechanisms of T follicular helper-mediated immunity and pathology. Front Immunol (2018) 9:1884. doi: 10.3389/fimmu.2018.01884
60. Wherry EJ, Blattman JN, Murali-Krishna K, van der Most R, Ahmed R. Viral persistence alters CD8 T-cell immunodominance and tissue distribution and results in distinct stages of functional impairment. J Virol (2003) 77(8):4911–27. doi: 10.1128/JVI.77.8.4911-4927.2003
61. Baitsch L, Baumgaertner P, Devêvre E, Raghav SK, Legat A, Barba L, et al. Exhaustion of tumor-specific CD8+ T cells in metastases from melanoma patients. J Clin Invest (2011) 121(6):2350–60. doi: 10.1172/JCI46102
62. Thommen DS, Koelzer VH, Herzig P, Roller A, Trefny M, Dimeloe S, et al. A transcriptionally and functionally distinct PD-1(+) CD8(+) T cell pool with predictive potential in non-small-cell lung cancer treated with PD-1 blockade. Nat Med (2018) 24(7):994–1004. doi: 10.1038/s41591-018-0057-z
63. van der Leun AM, Thommen DS, Schumacher TN. CD8(+) T cell states in human cancer: insights from single-cell analysis. Nat Rev Cancer (2020) 20(4):218–32. doi: 10.1038/s41568-019-0235-4
64. Sade-Feldman M, Yizhak K, Bjorgaard SL, Ray JP, de Boer CG, Jenkins RW, et al. Defining T cell states associated with response to checkpoint immunotherapy in melanoma. Cell (2018) 175(4):998–1013.e20. doi: 10.1016/j.cell.2018.10.038
65. Guo X, Zhang Y, Zheng L, Zheng C, Song J, Zhang Q, et al. Global characterization of T cells in non-small-cell lung cancer by single-cell sequencing. Nat Med (2018) 24(7):978–85. doi: 10.1038/s41591-018-0045-3
66. Tirosh I, Izar B, Prakadan SM, Wadsworth MH 2nd, Treacy D, Trombetta JJ, et al. Dissecting the multicellular ecosystem of metastatic melanoma by single-cell RNA-seq. Science (2016) 352(6282):189–96. doi: 10.1126/science.aad0501
67. Zheng C, Zheng L, Yoo JK, Guo H, Zhang Y, Guo X, et al. Landscape of Infiltrating T Cells in Liver Cancer Revealed by Single-Cell Sequencing. Cell. (2017) 169(7):1342–56.e16.
68. Li H, van der Leun AM, Yofe I, Lubling Y, Gelbard-Solodkin D, van Akkooi ACJ, et al. Dysfunctional CD8 T cells form a proliferative, dynamically regulated compartment within human melanoma. Cell (2019) 176(4):775–89.e18. doi: 10.1016/j.cell.2018.11.043
69. Kim HJ, Cantor H. CD4 T-cell subsets and tumor immunity: The helpful and the not-so-helpful. Cancer Immunol Res (2014) 2(2):91–8. doi: 10.1158/2326-6066.CIR-13-0216
70. Brown ZJ, Fu Q, Ma C, Kruhlak M, Zhang H, Luo J, et al. Carnitine palmitoyltransferase gene upregulation by linoleic acid induces CD4(+) T cell apoptosis promoting HCC development. Cell Death Dis (2018) 9(6):620. doi: 10.1038/s41419-018-0687-6
71. Li J, Zhou J, Kai S, Wang C, Wang D, Jiang J. Functional and clinical characterization of tumor-infiltrating T cell subpopulations in hepatocellular carcinoma. Front Genet (2020) 11:586415. doi: 10.3389/fgene.2020.586415
72. Ma C, Kesarwala AH, Eggert T, Medina-Echeverz J, Kleiner DE, Jin P, et al. NAFLD causes selective CD4(+) T lymphocyte loss and promotes hepatocarcinogenesis. Nature (2016) 531(7593):253–7. doi: 10.1038/nature16969
73. Mantovani A, Marchesi F, Malesci A, Laghi L, Allavena P. Tumour-associated macrophages as treatment targets in oncology. Nat Rev Clin Oncol (2017) 14(7):399–416. doi: 10.1038/nrclinonc.2016.217
74. Galon J, Costes A, Sanchez-Cabo F, Kirilovsky A, Mlecnik B, Lagorce-Pagès C, et al. Type, density, and location of immune cells within human colorectal tumors predict clinical outcome. Science (2006) 313(5795):1960–4. doi: 10.1126/science.1129139
75. Mlecnik B, Tosolini M, Kirilovsky A, Berger A, Bindea G, Meatchi T, et al. Histopathologic-based prognostic factors of colorectal cancers are associated with the state of the local immune reaction. J Clin Oncol (2011) 29(6):610–8. doi: 10.1200/JCO.2010.30.5425
76. Pagès F, Mlecnik B, Marliot F, Bindea G, Ou FS, Bifulco C, et al. International validation of the consensus immunoscore for the classification of colon cancer: a prognostic and accuracy study. Lancet (2018) 391(10135):2128–39. doi: 10.1016/S0140-6736(18)30789-X
77. Galon J, Angell HK, Bedognetti D, Marincola FM. The continuum of cancer immunosurveillance: prognostic, predictive, and mechanistic signatures. Immunity (2013) 39(1):11–26. doi: 10.1016/j.immuni.2013.07.008
78. Camus M, Tosolini M, Mlecnik B, Pagès F, Kirilovsky A, Berger A, et al. Coordination of intratumoral immune reaction and human colorectal cancer recurrence. Cancer Res (2009) 69(6):2685–93. doi: 10.1158/0008-5472.CAN-08-2654
79. Mlecnik B, Bindea G, Angell HK, Sasso MS, Obenauf AC, Fredriksen T, et al. Functional network pipeline reveals genetic determinants associated with in situ lymphocyte proliferation and survival of cancer patients. Sci Transl Med (2014) 6(228):228ra37. doi: 10.1126/scitranslmed.3007240
80. Galon J, Bruni D. Approaches to treat immune hot, altered and cold tumours with combination immunotherapies. Nat Rev Drug Discovery (2019) 18(3):197–218. doi: 10.1038/s41573-018-0007-y
81. Duda P, Janczara J, McCubrey JA, Gizak A, Rakus D. The reverse warburg effect is associated with Fbp2-dependent Hif1α regulation in cancer cells stimulated by fibroblasts. Cells (2020) 9(1). doi: 10.3390/cells9010205
82. Wei R, Cao J, Yao S. Matrine promotes liver cancer cell apoptosis by inhibiting mitophagy and PINK1/Parkin pathways. Cell Stress Chaperones (2018) 23(6):1295–309. doi: 10.1007/s12192-018-0937-7
83. Zheng YL, Li L, Jia YX, Zhang BZ, Li JC, Zhu YH, et al. LINC01554-mediated glucose metabolism reprogramming suppresses tumorigenicity in hepatocellular carcinoma via downregulating PKM2 expression and inhibiting Akt/mTOR signaling pathway. Theranostics (2019) 9(3):796–810. doi: 10.7150/thno.28992
84. Sengupta D, Cassel T, Teng KY, Aljuhani M, Chowdhary VK, Hu P, et al. Regulation of hepatic glutamine metabolism by miR-122. Mol Metab (2020) 34:174–86. doi: 10.1016/j.molmet.2020.01.003
85. Cai LY, Chen SJ, Xiao SH, Sun QJ, Ding CH, Zheng BN, et al. Targeting p300/CBP attenuates hepatocellular carcinoma progression through epigenetic regulation of metabolism. Cancer Res (2021) 81(4):860–72. doi: 10.1158/0008-5472.CAN-20-1323
86. Berndt N, Eckstein J, Heucke N, Gajowski R, Stockmann M, Meierhofer D, et al. Characterization of lipid and lipid droplet metabolism in human HCC. Cells (2019) 8(5). doi: 10.3390/cells8050512
87. Zimmerman RL, Fogt F, Burke M, Murakata LA. Assessment of glut-1 expression in cholangiocarcinoma, benign biliary lesions and hepatocellular carcinoma. Oncol Rep (2002) 9(4):689–92. doi: 10.3892/or.9.4.689
88. Ren L, Yao Y, Wang Y, Wang S. MiR-505 suppressed the growth of hepatocellular carcinoma cells via targeting IGF-1R. Biosci Rep (2019) 39(7). doi: 10.1042/BSR20182442
89. DeWaal D, Nogueira V, Terry AR, Patra KC, Jeon SM, Guzman G, et al. Hexokinase-2 depletion inhibits glycolysis and induces oxidative phosphorylation in hepatocellular carcinoma and sensitizes to metformin. Nat Commun (2018) 9(1):446.
90. Torimura T, Ueno T, Kin M, Harada R, Nakamura T, Kawaguchi T, et al. Autocrine motility factor enhances hepatoma cell invasion across the basement membrane through activation of beta1 integrins. Hepatology (2001) 34(1):62–71. doi: 10.1053/jhep.2001.25546
91. Li S, Dai W, Mo W, Li J, Feng J, Wu L, et al. By inhibiting PFKFB3, aspirin overcomes sorafenib resistance in hepatocellular carcinoma. Int J Cancer (2017) 141(12):2571–84. doi: 10.1002/ijc.31022
92. Wu Z, Wu J, Zhao Q, Fu S, Jin J. Emerging roles of aerobic glycolysis in breast cancer. Clin Transl Oncol (2020) 22(5):631–46. doi: 10.1007/s12094-019-02187-8
93. Gong Y, Zou B, Peng S, Li P, Zhu G, Chen J, et al. Nuclear GAPDH is vital for hypoxia-induced hepatic stellate cell apoptosis and is indicative of aggressive hepatocellular carcinoma behavior. Cancer Manag Res (2019) 11:4947–56. doi: 10.2147/CMAR.S202268
94. Li TE, Wang S, Shen XT, Zhang Z, Chen M, Wang H, et al. PKM2 drives hepatocellular carcinoma progression by inducing immunosuppressive microenvironment. Front Immunol (2020) 11:589997. doi: 10.3389/fimmu.2020.589997
95. Faloppi L, Scartozzi M, Bianconi M, Svegliati Baroni G, Toniutto P, Giampieri R, et al. The role of LDH serum levels in predicting global outcome in HCC patients treated with sorafenib: implications for clinical management. BMC Cancer (2014) 14:110. doi: 10.1186/1471-2407-14-110
96. Shi X, Xing H, Yang X, Li F, Yao S, Congwei J, et al. Comparison of PET imaging of activated fibroblasts and (18)F-FDG for diagnosis of primary hepatic tumours: a prospective pilot study. Eur J Nucl Med Mol Imaging (2021) 48(5):1593–603. doi: 10.1007/s00259-020-05070-9
97. Park SH, Ozden O, Liu G, Song HY, Zhu Y, Yan Y, et al. SIRT2-mediated deacetylation and tetramerization of pyruvate kinase directs glycolysis and tumor growth. Cancer Res (2016) 76(13):3802–12. doi: 10.1158/0008-5472.CAN-15-2498
98. Nakatsu D, Horiuchi Y, Kano F, Noguchi Y, Sugawara T, Takamoto I, et al. L-cysteine reversibly inhibits glucose-induced biphasic insulin secretion and ATP production by inactivating PKM2. Proc Natl Acad Sci U S A (2015) 112(10):E1067–76. doi: 10.1073/pnas.1417197112
99. Lu J, Liu SY, Zhang J, Yang GM, Gao GB, Yu NN, et al. Inhibition of BAG3 enhances the anticancer effect of shikonin in hepatocellular carcinoma. Am J Cancer Res (2021) 11(7):3575–93.
100. Shiratori R, Furuichi K, Yamaguchi M, Miyazaki N, Aoki H, Chibana H, et al. Glycolytic suppression dramatically changes the intracellular metabolic profile of multiple cancer cell lines in a mitochondrial metabolism-dependent manner. Sci Rep (2019) 9(1):18699. doi: 10.1038/s41598-019-55296-3
101. Brand A, Singer K, Koehl GE, Kolitzus M, Schoenhammer G, Thiel A, et al. LDHA-associated lactic acid production blunts tumor immunosurveillance by T and NK cells. Cell Metab (2016) 24(5):657–71. doi: 10.1016/j.cmet.2016.08.011
Keywords: metabolic rearrangements, intratumoral heterogeneity, immune response, hepatocellular carcinoma, tumor microenvironment
Citation: Xu F-Q, Dong M-M, Wang Z-F and Cao L-D (2023) Metabolic rearrangements and intratumoral heterogeneity for immune response in hepatocellular carcinoma. Front. Immunol. 14:1083069. doi: 10.3389/fimmu.2023.1083069
Received: 28 October 2022; Accepted: 09 January 2023;
Published: 25 January 2023.
Edited by:
Leonardo M. R. Ferreira, Medical University of South Carolina, United StatesReviewed by:
Albert Li, Stanford University, United StatesCopyright © 2023 Xu, Dong, Wang and Cao. This is an open-access article distributed under the terms of the Creative Commons Attribution License (CC BY). The use, distribution or reproduction in other forums is permitted, provided the original author(s) and the copyright owner(s) are credited and that the original publication in this journal is cited, in accordance with accepted academic practice. No use, distribution or reproduction is permitted which does not comply with these terms.
*Correspondence: Li-Dong Cao, aWxrd2VueHUyMDE5QHNpbmEuY29t; Zhi-Fei Wang, emhpZmVpMTk3M0AxMjYuY29t
†These authors have contributed equally to this work
Disclaimer: All claims expressed in this article are solely those of the authors and do not necessarily represent those of their affiliated organizations, or those of the publisher, the editors and the reviewers. Any product that may be evaluated in this article or claim that may be made by its manufacturer is not guaranteed or endorsed by the publisher.
Research integrity at Frontiers
Learn more about the work of our research integrity team to safeguard the quality of each article we publish.