- 1Department of Dermatology, Xiangya Hospital, Central South University, Changsha, Hunan, China
- 2Hunan Key Laboratory of Skin Cancer and Psoriasis, Xiangya Hospital, Changsha, Hunan, China
- 3Hunan Engineering Research Center of Skin Health and Disease, Xiangya Hospital, Changsha, Hunan, China
- 4Department of Research and Development, Beijing GAP Biotechnology Co., Ltd, Beijing, China
- 5Department of Health Management Center, Xiangya Hospital, Central South University, Changsha, Hunan, China
- 6National Clinical Research Center for Geriatric Disorders, Xiangya Hospital, Central South University, Changsha, Hunan, China
- 7Department of Social Medicine and Health Management, Xiangya School of Public Health, Central South University, Changsha, Hunan, China
Introduction: Psoriasis is a chronic inflammatory disease of the skin. A few studies have shown that psoriasis is an immune-mediated disease in which multiple immune cells play crucial roles. However, the association between circulating immune cells and psoriasis remains elusive.
Methods: To explore the role of circulating immune cells in psoriasis, 361,322 individuals from the UK Biobank (UKB) and 3,971 patients with psoriasis from China were included to investigate the association between white blood cells and psoriasis via an observational study. Genome-wide association studies (GWAS) and Mendelian randomization (MR) were used to evaluate the causal relationship between circulating leukocytes and psoriasis.
Results: The risk of psoriasis increased with high levels of monocytes, neutrophils, and eosinophils (relative risks and 95% confidence intervals, respectively: 1.430 (1.291–1.584) for monocytes, 1.527 (1.379–1.692) for neutrophils, and 1.417 (1.294–1.551) for eosinophils). Upon further MR analysis, eosinophils showed a definite causal relationship with psoriasis (odds ratio of inverse-variance weighted: 1.386, 95% confidence intervals: 1.092–1.759) and a positive correlation with the psoriasis area and severity index (PASI) score (P = 6.6 × 10-5). The roles of the neutrophil-lymphocyte ratio (NLR), platelet-lymphocyte ratio (PLR), and lymphocyte-monocyte ratio (LMR) in psoriasis were also assessed. More than 20,000 genetic variations associated with NLR, PLR, and LMR were discovered in a GWAS analysis using the UKB data. Following adjustment for covariates in the observational study, NLR and PLR were shown to be risk factors for psoriasis, whereas LMR was a protective factor. MR results indicated that there was no causal relationship between these three indicators and psoriasis; however, NLR, PLR, and LMR correlated with the PASI score (NLR: rho = 0.244, P = 2.1 × 10-21; PLR: rho = 0.113, P = 1.4 × 10-5; LMR: rho = -0.242, P = 3.5×10-21).
Discussion: Our findings revealed an important association between circulating leukocytes and psoriasis, which is instructive for the clinical practice of psoriasis treatment.
1 Introduction
As a common chronic inflammatory skin disease, psoriasis affects more than 60 million adults and children, considerably impairs the quality of life of patients, and places a heavy burden on individuals and society (1); however, the pathogenesis of psoriasis has not yet been fully elucidated (2). Immune cells play a crucial role in the pathogenesis of psoriasis (1, 3, 4), and previous studies have indicated the importance of systemic immunity in psoriasis, such as excessive interleukin 17 (IL-17) and interleukin 36, in circulating immune cells (5, 6). Meanwhile, patients with psoriasis have shown a unique profile of circulating leukocytes (7, 8), and increased neutrophil counts have been discovered in multiple studies (9, 10). However, more studies have focused on immunocytes from the skin lesions of psoriasis (11–13), and the role of circulating white blood cells in psoriasis remains unclear. Lymphocytes, especially T cells, have been recognized as disease-causing cells in psoriasis (14); however, some studies have shown decreased circulating lymphocytes in patients with psoriasis (15). The relationship between psoriasis and other circulating cells, such as eosinophils and basophils, has been rarely reported. Therefore, it is necessary to explore this association further.
Although some studies have shown the important role of circulating immune cells in psoriasis, these results were based on observational studies that were limited by sample size and bias. More appropriate methods are required to improve these studies. Mendelian randomization (MR) studies use instrumental variables associated with exposure to assess possible causal relationships with outcomes. This method can reduce the potential confounding bias (16, 17). The causal relationships between various phenotypes and diseases have been revealed using MR analysis (18, 19). Thus, it is feasible to use MR to explore the causal relationship between circulating immune cells and psoriasis.
To further understand the role of circulating immune cells in psoriasis, we explored the association between five main types of white blood cells and psoriasis using data from the UK Biobank (UKB) and a Chinese population and evaluated the potential causal relationship between psoriasis and white blood cells. We further explored the roles of the neutrophil-lymphocyte ratio (NLR), platelet-lymphocyte ratio (PLR), and lymphocyte-monocyte ratio (LMR) in psoriasis. Our results provide a comprehensive overview of the association between circulating white blood cells and psoriasis, suggesting that eosinophil count is a potential factor related to the incidence and severity of psoriasis.
2 Methods
2.1 Study population
In total, 361,322 individuals from the UKB and 3,971 individuals from a Chinese population were included in our study (Figure 1). The UKB is a prospective cohort study with a large amount of genetic and phenotypic data collected from approximately 500,000 individuals across the United Kingdom from 2006 to 2010 (20–22). Patients with psoriasis were included from primary care, hospital admission, self-reports, and other sources in the UKB (Table S1). Participants were excluded from the UKB dataset based on the following criteria: it did not pass quality control of genotypic data (missing information on individual data > 0.02, sex discrepancy, and deviates of more than ±3 standard deviations (SD) from the heterozygosity rate mean of the samples); kinship >first-degree relationship; genetic ethnic grouping showed non-Caucasian (defined by data-field: 22,006 from UKB based on a principal components analysis of the genotypes) (23); suffering from diseases of the blood and hematopoietic system including leukemia, lymphoma, multiple myeloma, aplastic anemias, and agranulocytosis, among others (Table S1); and a white blood cell count >200 × 109 cells/L (24). The Chinese population included patients with psoriasis admitted to Xiangya Hospital, Central South University between 2019 and 2020. Patients with diseases of the blood and hematopoietic system and abnormal white blood cell count (>200 × 109 cells/L) were excluded. The diagnosis of psoriasis in the Chinese population was confirmed by two or more dermatologists. The UKB received ethical approval from the Northwest Multi-Center Research Ethics Committee (11/NW/03820). All procedures involving study participants in the Chinese population were approved by the institutional research ethics board of Xiangya Hospital (2018121106). Written informed consent was obtained from all participants prior to the investigation.
2.2 Exposure and outcome variables
We selected white blood cells, lymphocytes, monocyte, neutrophil, eosinophil, and basophil counts as indicators of circulating leukocytes (19). All measured data of white blood cells were sourced from the baseline in the UKB. To obtain the white blood cell count, the Beckman Coulter LH750 instruments were used to analyze blood samples collected in EDTA (Ethylenediaminetetraacetic acid) vacutainers from participants in the UKB and Chinese populations. Regarding data distribution, eosinophils, and basophils showed a skewed distribution in the UKB (Figure S1). Moreover, the five main white blood cell types correlated with each other (Figure S2, all P <2.2 × 10-16). We simultaneously calculated the NLR, PLR, and LMR via neutrophil count, lymphocyte count, monocyte count, and platelet count excluding individuals whose denominator was zero.
The phenotype of psoriasis was identified using the codes of the International Classification of Disease (ICD-9 and ICD-10) for participants in the UKB and Chinese population. We evaluated the severity of psoriasis using three indices: psoriasis area and severity index (PASI) score, dermatology life quality index (DLQI), and body surface area (BSA) (25–27). The PASI score was calculated based on the intensity of three clinical signs (redness, thickness, and scaling) in four sections of the body (head, arms, trunk, and legs) (more details are available at https://dermnetnz.org/topics/pasi-score). The BSA was calculated based on the proportion of skin affected by psoriasis, denoted as a percentage of the total body area. The DLQI was determined via questionnaires to assess the extent to which the skin problem has affected the patients’ lives over the last week (questionnaires are available at https://www.nhsfife.org/media/32589/dermatology-life-quality-index-dlqi.pdf). Dermatologists evaluated the PASI score and BSA according to the area and severity of lesions, and the DLQI was obtained from a questionnaire.
2.3 Observational study
Patients with psoriasis at baseline were excluded from the UKB, and 353,418 participants were included in the cohort study. The mean follow-up time was 12.88 years. Z-score standardization was adopted to process the data to eliminate the interference of different dimensions among the types of white blood cells. White blood cell count and the count of the five main types of white blood cells were divided into four categories in the UKB based on quartiles. Relative risks (RRs) and 95% confidence intervals (95% CIs) were calculated for each category, using the first category as a reference. We also analyzed the relationship between different types of white blood cells and psoriasis in continuously defined white blood cell counts using Cox regression models with age as the time scale and logistic regression models. Sex, age, smoking status (never, previous, and current), alcohol drinking status (never, previous, and current), and body mass index (BMI) were adjusted as covariates (28). A sensitivity analysis excluding individuals with a reported incidence of psoriasis in the first two years after baseline was performed to confirm the findings. Owing to the correlations among the main types of white blood cells (Figure S2), the lasso regression model, including five white blood cell types and covariates, was used to select important variables and avoid potential multicollinearity (29). For the NLR, PLR, and LMR, z-score standardization was used to remove the impact of dimension, and the association with psoriasis was evaluated using the Cox regression model. In the Chinese population, Spearman’s correlation was used to determine the correlation between indices associated with white blood cells and severity in a total of 3,971 patients with psoriasis. The PASI score, DLQI, and BSA were all included in the correlation study.
2.4 One-sample MR analysis
To test the causal relationship between white blood cells and psoriasis, a one-sample MR analysis was performed in the UKB. First, 482, 457, 512, 372, 464, and 144 single nucleotide polymorphisms (SNPs) associated with white blood cell, lymphocyte, monocyte, neutrophil, eosinophil, and basophil counts, respectively, were selected from a meta-analysis (GWAS) study (Table S2) (30). These SNPs were used as instrumental variables after deleting ambiguous SNPs and controlling for linkage disequilibrium (LD, reference panel: European from the 1000 genomes reference panel, r2 <0.01, window: 10,000 kb): 178 SNPs for white blood cell count, 140 SNPs for lymphocyte count, 167 SNPs for monocyte count, 128 SNPs for the neutrophil count, 166 SNPs for eosinophil count, and 51 SNPs for basophil count (23). The genetic risk score (GRS) of each sample in the UKB was calculated using the selected instrumental variables (31). Allele scores were weighted based on the effect size in the meta-GWAS analysis (30). Thereafter, we evaluated the degree of variation explanation for GRSs and performed z-score standardization for GRSs. White blood cell, lymphocyte, monocyte, neutrophil, eosinophil, and basophil counts were explained by 2.66%, 1.76%, 3.00%, 1.94%, 3.34%, and 0.39%, respectively. The GRSs of white blood cells were divided into four categories according to quartiles. The calculated GRSs were separately associated with circulating immune cell counts (Figure S3). Finally, one-sample MR analysis was performed by two-stage least-squares regression (2SLS) to explore the causal relationship between white blood cells and psoriasis after adjusting for age, sex, BMI, and the top 10 genetic principal components (32). To remove the effect of potential pleiotropy, the association between possible confounding factors (sex, age, BMI, smoking status, and alcohol drinking status) and GRSs was further examined. For categorical variables (sex, smoking status, and alcohol drinking status), logistic regression models were constructed, and categorical variables were viewed as dependent variables to test the association with the count and GRSs of white blood cells. For continuous variables (age and BMI), the count and GRSs of white blood cells were divided into four categories according to quartiles, and the difference in continuous variables was compared among all categories using the Kruskal–Wallis test (32). Thereafter, we performed MR analysis again after excluding SNPs related to confounding factors using PhenoScanner v2 (Table S3) (33).
2.5 Two-sample MR analysis
Two-sample MR analysis was conducted to further verify the results from one-sample MR. Two GWAS summary statistic datasets from MRC-IEU (34, 35) (https://gwas.mrcieu.ac.uk/) and the FinnGen biobank (https://www.finngen.fi/en) were used to select SNPs as instrumental variables. The dataset related to white blood cells was obtained from the study by Astle et al. (18), and the other dataset related to psoriasis was obtained from the FinnGen biobank (36) (release: R2, finn-a-L12_PSORIASIS, and the phenotype of psoriasis were identified by ICD-8, ICD-9, and ICD-10). Final analyses included significant genome-wide (P <8.31 × 10-9) and uncorrelated SNPs (r2 <0.01) available in GWAS summary data of exposure variables. Seven methods of MR analysis were used to perform two-sample MR, including inverse-variance weighted (IVW) (37), MR-Egger (38), weighted median (39), simple mode (40), weighted mode (40), MR-PRESSO (41), and multivariable MR (42). The heterogeneity of all instrumental variables was determined using Cochran’s Q test. Steiger filtering tests were used to filter SNPs related to psoriasis, and Egger intercept tests were performed to check the pleiotropy of instrumental variables. Leave-one-out sensitivity analyses were performed after two-sample MR (43). To further explore causal relationships between relative indicators (NLR, PLR, and LMR) and psoriasis, we performed GWAS analysis for these three indicators in UKB. By setting the threshold of the P value, we discovered 5973 SNPs associated with NLR, 15473 associated with PLR, and 4606 associated with LMR (P adjusted by false discovery rate <8.31 × 10-9, Figure S4). Thereafter, SNPs associated with these three indicators (reference panel: European from the 1000 genomes reference panel, r2 <0.01, window: 10,000 kb) in our GWAS results and summary data associated with psoriasis from the FinnGen biobank were used to perform a two-sample MR analysis.
2.6 GWAS analysis
Genotype data in the UKB were obtained from imputation using HRC and UK10K as reference samples (21, 44). NLR, PLR, and LMR were used as phenotypic data to perform the GWAS. Quality control was completed before association analysis (see Section 2.1; missing information on SNPs < 0.02; minor allele frequency <0.05 and Hardy–Weinberg equilibrium <1 × 10-6 were used to filter SNPs to avoid the rarity and instability of SNPs) (45). The GWAS analysis was adjusted for age, sex, BMI, and the top 10 principal genetic components (24, 30). To distinguish confounding factors from polygenicity in our GWAS study, we used LD score regression to calculate the LD score regression intercepts (46). The intercepts of NLR, PLR, and LMR were 1.09, 1.12, and 1.08, respectively, which showed low confounding biases such as cryptic relatedness and population stratification. The source of the LD score was from Europe in the 1000 Genomes Project, and LD score regression was performed using ldsc (https://github.com/bulik/ldsc) (46).
2.7 Statistical analysis
We used R language (version 4.0.5) to analyze the data. GWAS was performed using PLINK v1.9. The “AER” package in R was used in one-sample MR. GRSs were calculated using PRSice-2 (47). The “TwoSampleMR” and “MRPRESSO” packages were used to perform two-sample MR and sensitivity analyses (34).
3 Results
A total of 361,322 individuals from the UKB and 3,971 individuals from the Chinese population were included in our study. The characteristics of all included individuals are shown in Table 1.
3.1 Association of white blood cells with psoriasis
Using the UKB data, a cohort study was performed after excluding patients with baseline psoriasis. The RRs of psoriasis incidence increased with the increase in monocytes (RR1st vs. 4th: 1.430, 95% CI: 1.291–1.584), neutrophils (RR1st vs. 4th: 1.527, 95% CI: 1.379–1.692), and eosinophils (RR1st vs. 4th: 1.417, 95% CI: 1.294–1.551) (Figure 2A). This was further verified by the analysis of continuously defined white blood cell counts in Cox regression models. Hazard ratios (HRs) were calculated after introducing the time of psoriasis onset. Monocytes, neutrophils, and eosinophils were found to be risk factors for psoriasis (Figures 2B, C). HRs were calculated again after excluding individuals with a reported incidence of psoriasis in the first two years after baseline. Similar results were observed after adjusting for covariates (HR of monocytes: 1.033 (1.022, 1.044), HR of neutrophils: 1.147 (1.110, 1.184); HR of eosinophils: 1.076 (1.054, 1.097)) (Table S4). Furthermore, logistic regression models without consideration for time of incidence also showed consistent results (Table S5). Owing to the correlation among the five major white blood cell types (Figure S2), the lasso regression model was used for the five major white blood cell types and confounding factors. Lymphocytes, monocytes, neutrophils, and eosinophils were retained, and it was found that BMI and smoking status played an important role in psoriasis, which is consistent with the results of previous studies (28, 48) (Figure S5).
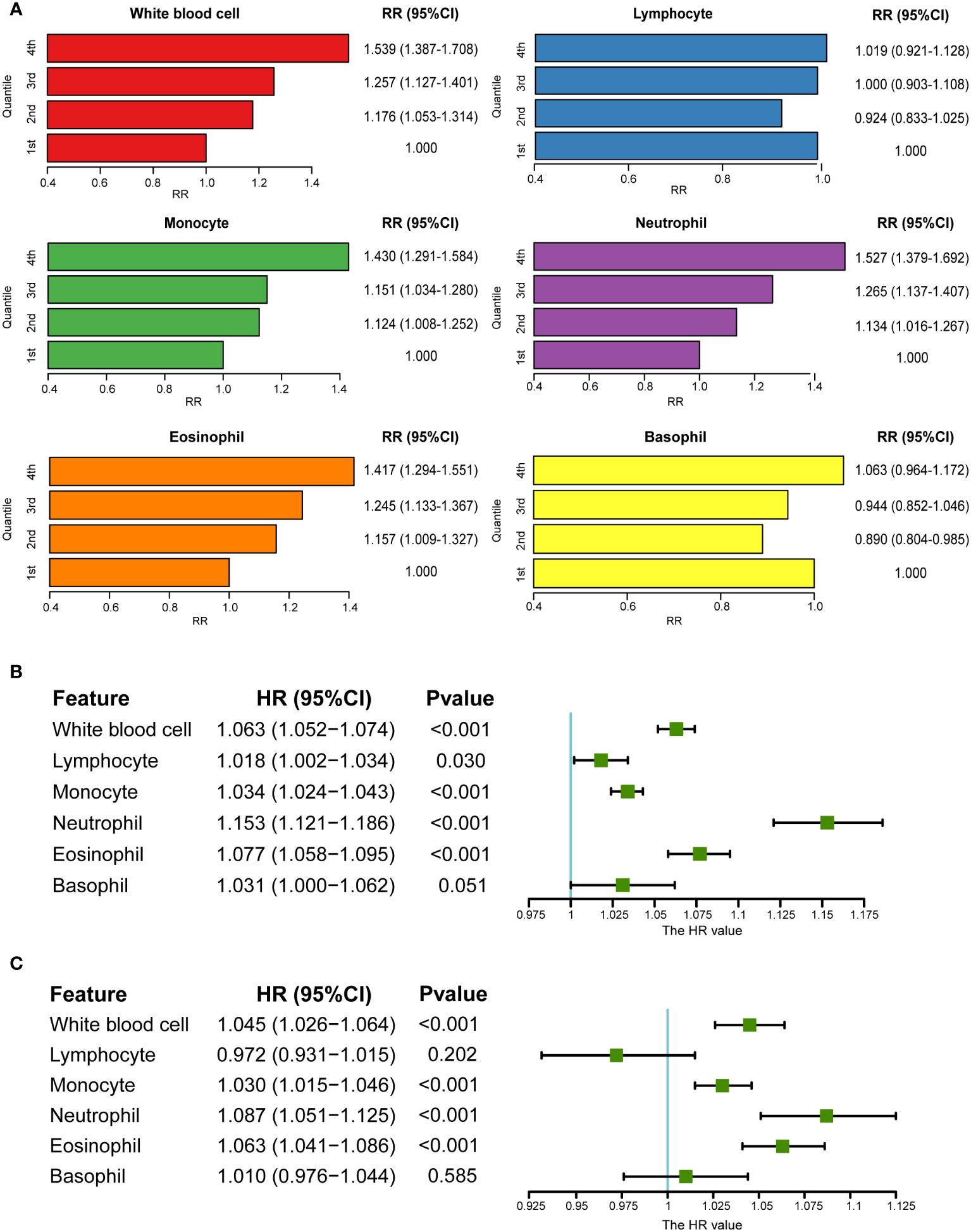
Figure 2 Association between circulating white blood cells and psoriasis in UKB. (A) RRs of psoriasis from categories of white blood cells (the first category was viewed as a reference). (B) Association between white blood cells and psoriasis in univariate Cox regression. (C) Association between white blood cells and psoriasis after adjusting for covariates in multivariate Cox regression (sex, age, BMI, smoking status, and alcohol drinking status).
3.2 Causal effect of white blood cells on psoriasis
The above results show that there is an association between white blood cells and psoriasis. However, whether a causal relationship between them exists needed to be further explored. One-sample MR analysis was performed to explore the potential causal relationship by 2SLS in the UKB. The GRSs of white blood cells were selected as instrumental variables. The result of one-sample MR showed that eosinophils (OR: 1.032, P = 7.37 × 10-3) and basophils (OR: 1.545, P = 2.87 × 10-6) had a positive causal relationship with psoriasis after adjusting for covariates (Table 2).
To determine potential pleiotropy, we tested whether confounding factors were associated with the GRSs of white blood cells. The results indicated that sex, age, smoking status, and alcohol drinking status were associated with the count of the five main types of white blood cells, and not with the calculated GRSs. Only BMI showed a correlation with the GRSs of total white blood cells and basophils (P <0.05), which might be a confounding factor causing pleiotropy in the MR analysis (Figure S6, S7, and S8). Considering the significant causal relationship between basophils and psoriasis (P = 2.87 × 10-6), SNPs associated with BMI were excluded from instrumental variables associated with basophils using PhenoScanner v2 (33), and one-sample MR analysis was performed again. The results showed that the causal relationship was still significant (OR: 1.520, 95% CI: 1.266–1.826).
3.3 Validation of causal relationship using two-sample MR
According to the one-sample MR results, eosinophils and basophils showed a causal relationship with psoriasis. However, because of the existence of “winners’ curse,” the conclusion required more data sources to be verified (49). To further test this result, two-sample MR analysis was performed, and the results revealed that the total white blood cell count and eosinophil count had positive causal relationships with psoriasis (Figures 3A-C and Table S6). In particular, both IVW and weighted median showed a positive causal relationship between eosinophil count and psoriasis (ORIVW: 1.386, 95% CI: 1.092–1.759; ORWM: 1.654, 95% CI: 1.129–2.422), which was consistent with the results of the one-sample MR. To eliminate possible pleiotropy, MR-PRESSO was used to exclude outlier SNPs. We used 1,000 elements to form the null distribution for simulation to compute empirical P-values; only one outlier was discovered in monocytes. In addition, results of Egger intercept test also showed low pleiotropy of SNPs. Meanwhile, the heterogeneity test showed potential heterogeneity in lymphocytes and monocytes (P < 0.05) (Table S6). The random effects model further explored the causal effect of lymphocytes and monocytes, and the results indicated that there was still no significant causal relationship between them and psoriasis (lymphocytes: P = 0.6551; monocytes: P = 0.6788). The Steiger filtering test showed that all instrumental variables exerted their primary effect on circulating immune cells rather than psoriasis. To make the results more reliable, leave-one-out sensitivity analyses were performed, which did not identify any SNPs with a marked impact on the results (Table S7) (43).
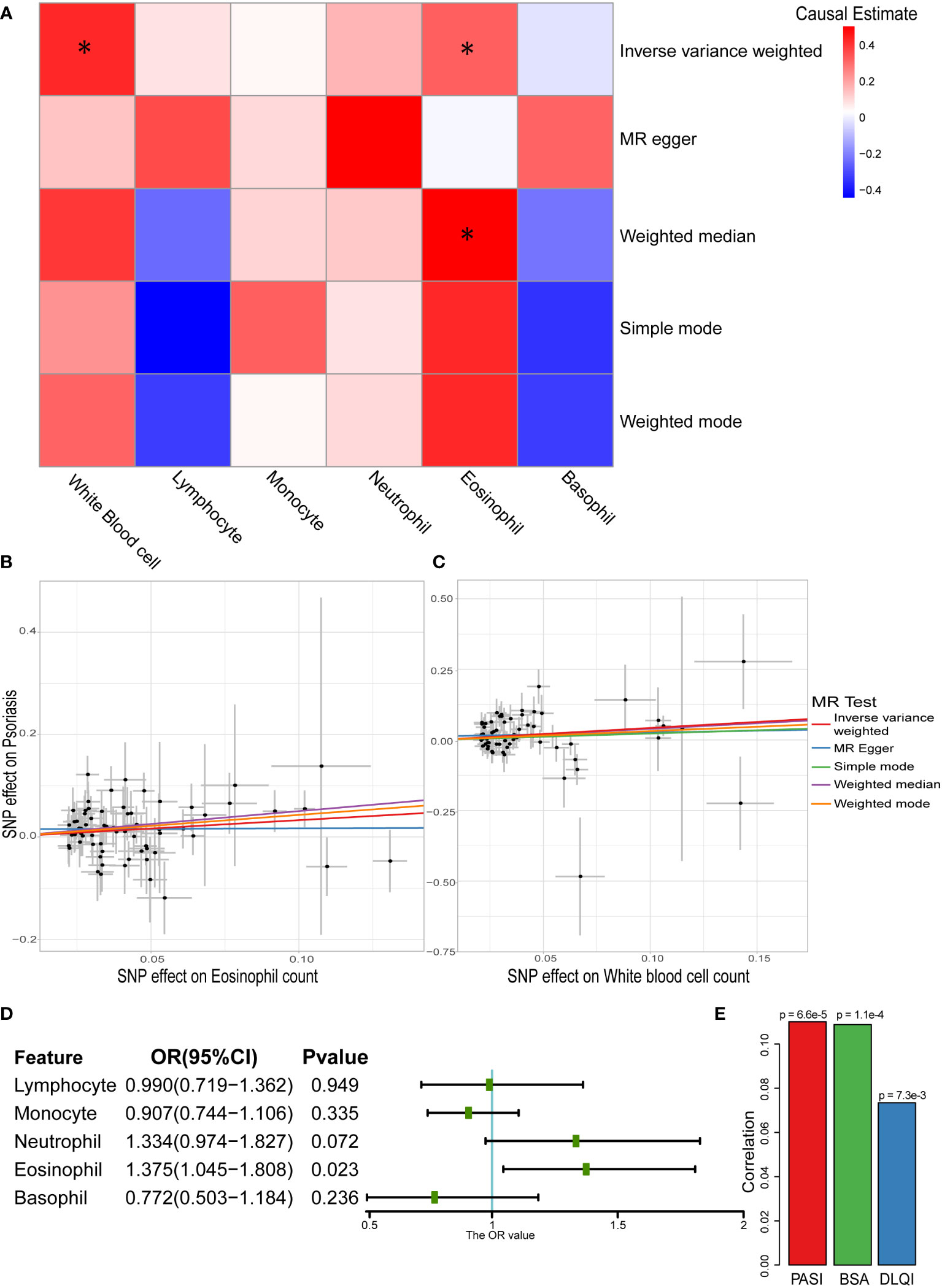
Figure 3 Causal relationship between white blood cells and psoriasis in two-sample MR analysis. (A) Heatmap of two-sample MR results about white blood cells and psoriasis (* represented P < 0.05). (B) Scatter plot of MR analysis related to eosinophil count and psoriasis. (C) Scatter plot of MR analysis related to white blood cell sum count and psoriasis. (D) Result of multivariable MR on different white blood cells and psoriasis. (E) Correlation between eosinophil count and severity of psoriasis (Spearman’s correlation).
Owing to the correlation among the five main types of white blood cells (Figure S2), they were used as exposure variables in multivariable MR analysis. The causal relationship between eosinophil count and psoriasis persisted (OR: 1.375, 95% CI: 1.045–1.808) even considering the association (Figure 3D). We further explored the correlation between eosinophil counts and the severity of psoriasis in the Chinese population. All three assessment methods showed a positive correlation, with the PASI score showing a strongest correlation (P = 6.6×10-5) (Figure 3E). This indicated that eosinophils have a potential impact on the severity of psoriasis.
3.4 The role of NLR, PLR, and LMR in psoriasis
NLR, PLR, and LMR, which are calculated using white blood cell count, have been proven to be important indices in inflammatory diseases (50–52). Whether they can be used to evaluate psoriasis remains unclear. Therefore, the relationship between these three indices and psoriasis was explored further.
Their association with psoriasis was demonstrated in the UKB dataset by constructing a Cox regression model. In the univariate Cox regression model, NLR (HRs: 1.051, 95% CIs: 1.035–1.067) was a potential risk factor, whereas LMR showed a protective function in psoriasis (HRs: 0.912, 95% CIs: 0.862–0.965). To remove the impact of confounding factors, we adjusted for sex, age, BMI, smoking status, and alcohol drinking status in the multivariate Cox regression model, and found that high PLR might increase the risk of psoriasis (HRs: 1.015, 95% CIs: 1.007–1.024) (Figure 4A).
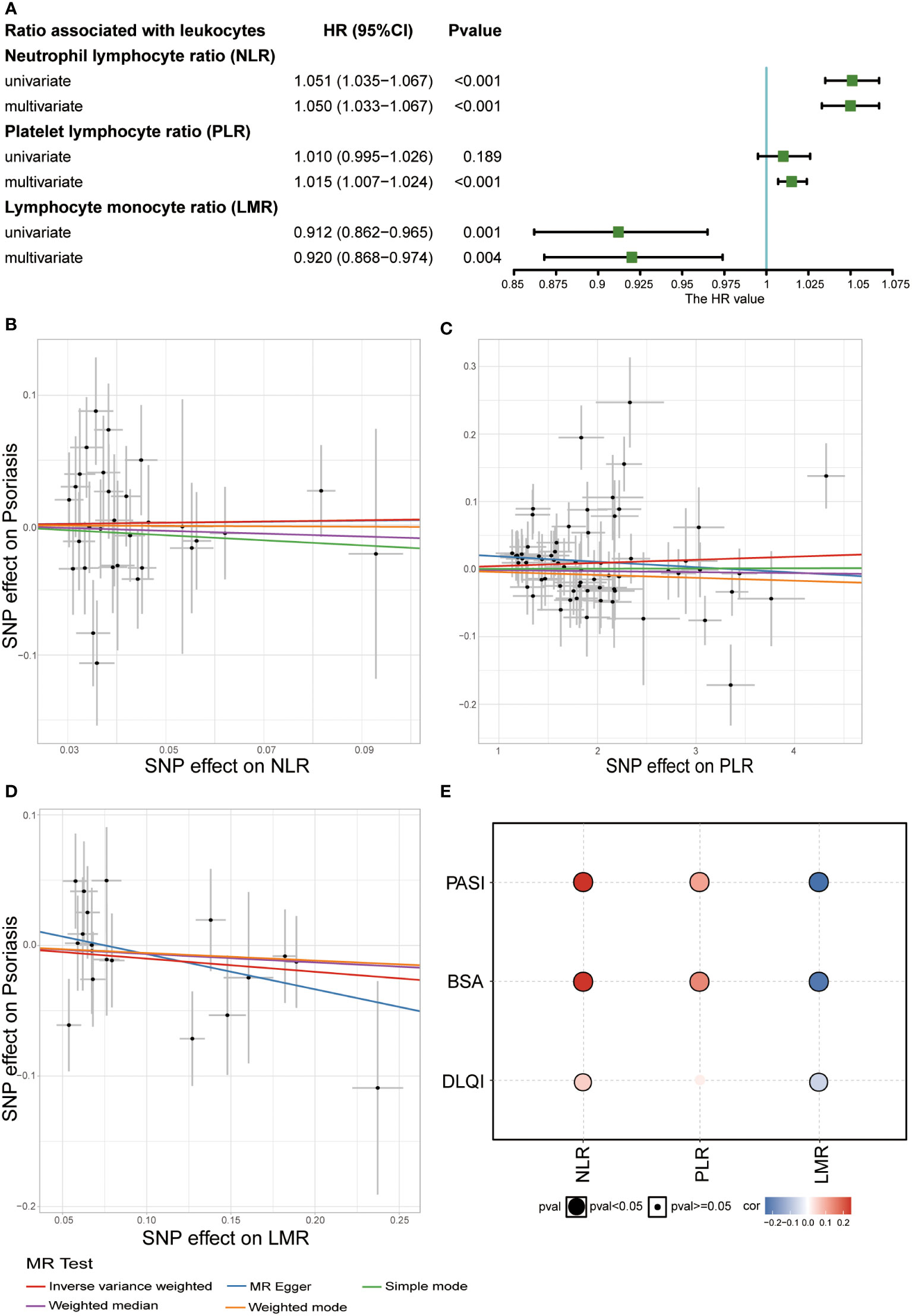
Figure 4 Relationship between white blood cell ratios (NLR, PLR, and LMR) and psoriasis. (A) Result of observational study about white blood cell ratios (NLR, PLR, and LMR) and psoriasis in UKB (Covariates included sex, age, BMI, smoking status, and alcohol drinking status and three ratios in multivariate Cox regression). (B) Scatter plot of MR analysis related to NLR and psoriasis. (C) Scatter plot of MR analysis related to PLR and psoriasis. (D) Scatter plot of MR analysis related to LMR and psoriasis. (E) Correlation between the three ratios and severity of psoriasis (Spearman’s correlation).
To explore the causal relationship between these three ratios and psoriasis, we performed a two-sample MR analysis. Due to the rarity of common large-scale GWAS on these three indices, GWAS analysis was performed in the UKB dataset. By setting the threshold of the P value, we discovered 5973 SNPs associated with NLR, 15473 SNPs associated with PLR, and 4606 SNPs associated with LMR (P adjusted by false discovery rate <8.31 × 10-9) (Figure S4 and Table S8). Our GWAS results, combined with GWAS summary data related to psoriasis from the FinnGen biobank, were used to perform two-sample MR analysis. No causal relationship was observed between the three indices and psoriasis (Figures 4B-D and Table S9). Subsequently, we studied the correlation between these three indices and psoriasis to explore their impact on the severity of psoriasis in the Chinese population. NLR and PLR positively correlated with the severity of psoriasis, particularly PASI score, and BSA, whereas LMR negatively correlated with the severity of psoriasis (Figure 4E). This indicated that the three indices might be potential predictive indices for the severity of psoriasis.
4 Discussion
This study revealed an association between circulating white blood cells and psoriasis in two large-scale populations. One-sample MR combined with two-sample MR analysis was performed in our study, making our results more reliable. Eosinophils showed a definite causal relationship with psoriasis. In addition, the three indices, NLR, PLR, and LMR, were further verified to play important roles in psoriasis. Our study revealed an explicit causal relationship between eosinophils and psoriasis, and provided new insights into the research and clinical practice of psoriasis care.
The observational study and MR analysis revealed that circulating white blood cells are associated with psoriasis. In particular, we found a causal relationship between eosinophils and psoriasis, and eosinophils positively correlated with psoriasis severity. Currently, psoriasis is considered an inflammatory skin disease mediated by T helper 1/17 cells, and the IL-17/IL-23 axis has been regarded as a key driver of psoriasis pathogenesis (53). Eosinophils are involved in type II immune response, which is related to T helper 2 cells and various interleukins (including IL-4, IL-5, IL-9, IL-13, IL-31, and IL-33, among others), differing from the IL-17/23 axis of psoriasis (54). Cytokines related to type II immune response, such as IL-4, could suppress the IL-17/23 axis of psoriasis in lesional skin (55). Furthermore, Ghoreschi et al. reported that IL-4 therapy could improve psoriasis (56). Nevertheless, several studies also observed a significant increase in eosinophils and cytokines, such as IL-4, IL-5, IL-9, IL-31, and IL-33, among others, in the blood of patients with psoriasis (7, 57–60). This contradiction might be caused by the location and environment of these immune cells and cytokines. Herein, a potential association between psoriasis and allergic diseases related to type II immune response, such as asthma and atopic dermatitis, was further verified in the UKB participants (chi-squared test, both P <0.05) (Table S10). Thus, type II immune response may play an important role in psoriasis; however, this role remains unclear.
In addition to type II immune response, eosinophils might have an effect on psoriasis via other mechanisms. In clinical practice, eosinophils are associated with pruritus, and over 85% of psoriasis patients suffer from pruritus (61). Persistent pruritus may lead to more scratching in the lesional skin of psoriasis patients, which may further aggravate psoriasis (62). Moreover, Kim et al. reported that eosinophils provide inflammatory signals that accelerate psoriasis pathogenesis (63). TLR7 is expressed in eosinophils and regulates the secretion of inflammatory mediators, thereby promoting the migration, activation, and survival of neutrophils in psoriasis, which provides a possible mechanism that explains the causal relationship between eosinophils and psoriasis (63). In general, eosinophils play an important role in psoriasis; however, the specific mechanism needs to be further explored.
Besides eosinophils, the other four cell types showed unique profiles in psoriasis. Both neutrophils and monocytes presented strong risk factors for psoriasis, which is consistent with the results of previous studies (9, 64). Neutrophils in psoriatic skin lesions serve as a typical histopathological hallmark of psoriasis, and they could also release IL-17 and are involved in the inflammatory cascade in psoriatic skin lesions (65). The respiratory burst with reactive oxygen species generation, degranulation, and the formation of neutrophil extracellular traps from neutrophils have been discovered to contribute to the immunopathogenesis of psoriasis (10). Monocytes showed a significant increase in chemotactic response in psoriasis (66), and also produce some cytokines of major importance in psoriasis, such as IL-1, IL-6, and tumor necrosis factors, among others (67). Immune cells derived from monocytes, such as dendritic cells and macrophages, play a crucial role in psoriasis-like inflammation (68, 69) These immune cells can secrete drivers of inflammation, such as IL-23, to promote the incidence of psoriasis (70). Meanwhile, biological therapy for psoriasis could decrease the activity of monocytes and neutrophils (71). Although our cohort study also highlighted the importance of these two cell types, no sufficiently causal relationship between them and psoriasis was identified in the MR results. This discrepancy might be explained by epidemiological confounding factors related to the study cohort. In addition, other studies have reported that immune cells derived from the skin might also play a crucial role in the incidence of psoriasis (65, 67); hence, the causal relationship between these two cell types in skin lesions and psoriasis may need to be explored in the future. Although lymphocytes have been proven to be a key factor in the pathogenesis of psoriasis (3, 72), the circulating lymphocyte count tends to decrease in patients with psoriasis, according to a previous study (15). One possible hypothesis is that numerous lymphocytes flow into the skin from the peripheral blood, which causes a decrease in circulating lymphocytes in patients with psoriasis (73). In our study, lymphocytes did not show a significant causal relationship with psoriasis; however, it should be noted that different subtypes of circulating lymphocytes may undergo diverse changes, which requires further study. Basophils, as a type of immune cells associated with allergies, have been reported to be involved in the regulation of skin inflammation (74) and to play an important role in psoriasis-associated pruritus (75). Due to the rarity of basophils in the blood and skin, their function in psoriasis was often ignored in previous studies and is still unclear. Our results showed that basophils may have a potential causal association with psoriasis. However, the statistical significance of the finding could not be substantiated because the count values of basophils in most participants were zero (Figure S1). More data are required to verify the relationship between basophils and psoriasis.
NLR, PLR, and LMR are markers of systemic inflammatory response and showed significant changes in patients with psoriasis (7, 15, 64, 76). However, these studies were limited by their small sample sizes. Moreover, large-scale GWAS data associated with these three indices are unavailable. Our study used large-scale population data to verify this conclusion and provide a potential genetic variation related to these three indices. The MR analysis showed no evidence of a causal relationship between psoriasis and these indices. These changes in psoriasis may be caused by inflammation itself, rather than a direct causal relationship. However, it is certain that these three indices are still potential predictors of the incidence and severity of psoriasis.
Although our study showed an important relationship between circulating white blood cells and psoriasis in a large-scale population, there were some limitations. First, our cohort study and MR analysis were only based on a European population. Thus, the proven causal relationship may only apply to the European population, and further investigation is needed in other races. Second, white blood cells were divided into five main types in our study; however, these subtypes of white blood cells could be further subdivided. For example, lymphocytes can be further divided into B cells and T cells. Identification of key cell subpopulations in psoriasis may be challenging because of their complex cell composition. Third, we identified an association between circulating white blood cells and psoriasis; however, the specific mechanism of circulating white blood cells needs to be further explored. Finally, the LD score regression intercepts of PLR in the GWAS were relatively high, indicating that a more refined population division is needed for the Caucasian population of the UKB.
In summary, our study identified a definite association between circulating white blood cells and psoriasis, which can be helpful in dermato-epidemiology and clinical practice to an extent. The incidence of psoriasis is an important public health concern, and as test results from peripheral blood, white blood cell count is relatively easy to obtain for the prediction of psoriasis risk. Eosinophils, NLR, PLR, and LMR might be indices used to evaluate treatment effects due to their correlation with the severity of psoriasis. Eosinophils might also become a novel target in psoriasis treatment; however, more basic research needs to be performed to elaborate on the specific mechanism of eosinophils in psoriasis.
Data availability statement
The original contributions presented in the study are included in the article/Supplementary Material. Further inquiries can be directed to the corresponding authors.
Ethics statement
The studies involving human participants were reviewed and approved by The North West Multi-Center Research Ethics Committee and the institutional research ethics boards of Xiangya Hospital. The patients/participants provided their written informed consent to participate in this study.
Author contributions
GWZ and XMR designed the experiments, analyzed the data, and prepared the manuscript. HL, MXS, GXZ, XC, and FYM provided supervision and oversaw final manuscript preparation. ZWT, WL, and WQC collected the data and helped with observational study. YH, BLW, and HLZ cleaned the data and helped with MR analysis. All the authors contributed to and approved the final version of this manuscript.
Funding
This work was supported by the National Key Research and Development Program of China (no. 2019YFE0120800 and no. 2019YFA0111600 to HL), the National Natural Science Foundation of China (no. 31800979 to HL, no. 82102472 to XZ, and no.62102455 to GXZ), the Natural Science Foundation of China for outstanding Young Scholars (no. 82022060 to HL), Science Foundation for Youths of Hunan Province (no. 2020JJ5875 to XZ), the Natural Science Foundation of Hunan Province for outstanding Young Scholars (no. 2019JJ30040 to HL), the Natural Science Foundation of Hunan Province of China (no. 2018SK2082 to HL), the Scientific Research Project of Hunan Health and Family Planning Commission (no. B20180855 to HL), China Postdoctoral Science Foundation (no. 2020M682587 to GXZ), Hunan Outstanding Postdoctoral Innovative Talents Program (no.2021RC2035 to GXZ), the Fundamental Research Funds for the Central Universities of Central South University (no. 2022ZZTS0861 to GWZ), and the Project of Intelligent Management Software for Multimodal Medical Big Data for New Generation Information Technology, Ministry of Industry and Information Technology of People’s Republic of China (TC210804V).
Acknowledgments
This study was conducted using the UKB resource under application number 55257. The authors thank the staff and participants of the Chinese population and the support of Xiangya Hospital, Hunan, China. Moreover, we would like to acknowledge the participants and investigators of the FinnGen study.
Conflict of interest
Author HZ was employed by Beijing GAP Biotechnology Co., Ltd.
The remaining authors declare that the research was conducted in the absence of any commercial or financial relationships that could be construed as a potential conflict of interest.
Publisher’s note
All claims expressed in this article are solely those of the authors and do not necessarily represent those of their affiliated organizations, or those of the publisher, the editors and the reviewers. Any product that may be evaluated in this article, or claim that may be made by its manufacturer, is not guaranteed or endorsed by the publisher.
Supplementary material
The Supplementary Material for this article can be found online at: https://www.frontiersin.org/articles/10.3389/fimmu.2023.1043380/full#supplementary-material
References
2. Armstrong AW, Read C. Pathophysiology, clinical presentation, and treatment of psoriasis: A review. Jama (2020) 323:1945–60. doi: 10.1001/jama.2020.4006
3. Ebbo M, Crinier A, Vely F, Vivier E. Innate lymphoid cells: Major players in inflammatory diseases. Nat Rev Immunol (2017) 17:665–78. doi: 10.1038/nri.2017.86
4. Nestle FO, Di Meglio P, Qin JZ, Nickoloff BJ. Skin immune sentinels in health and disease. Nat Rev Immunol (2009) 9:679–91. doi: 10.1038/nri2622
5. Wang C, Suarez-Farinas M, Nograles KE, Mimoso CA, Shrom D, Dow ER, et al. IL-17 induces inflammation-associated gene products in blood monocytes, and treatment with ixekizumab reduces their expression in psoriasis patient blood. J Invest Dermatol (2014) 134:2990–3. doi: 10.1038/jid.2014.268
6. Catapano M, Vergnano M, Romano M, Mahil SK, Choon SE, Burden AD, et al. IL-36 promotes systemic IFN-I responses in severe forms of psoriasis. J Invest Dermatol (2020) 140:816–26. doi: 10.1016/j.jid.2019.08.444
7. Kim DS, Shin D, Lee MS, Kim HJ, Kim DY, Kim SM, et al. Assessments of neutrophil to lymphocyte ratio and platelet to lymphocyte ratio in Korean patients with psoriasis vulgaris and psoriatic arthritis. J Dermatol (2016) 43:305–10. doi: 10.1111/1346-8138.13061
8. Batycka-Baran A, Hattinger E, Zwicker S, Summer B, Howard OMZ, Thomas P, et al. Leukocyte-derived koebnerisin (S100A15) and psoriasin (S100A7) are systemic mediators of inflammation in psoriasis. J Dermatol Sci (2015) 79:214–21. doi: 10.1016/j.jdermsci.2015.05.007
9. Rodriguez-Rosales YA, Langereis JD, Gorris M, van den Reek J, Fasse E, Netea MG, et al. Immunomodulatory aged neutrophils are augmented in blood and skin of psoriasis patients. J Allergy Clin Immunol (2021) 148:1030–40. doi: 10.1016/j.jaci.2021.02.041
10. Chiang CC, Cheng WJ, Korinek M, Lin CY, Hwang TL. Neutrophils in psoriasis. Front Immunol (2019) 10:2376. doi: 10.3389/fimmu.2019.02376
11. Reynolds G, Vegh P, Fletcher J, Poyner E, Stephenson E, Goh I, et al. Developmental cell programs are co-opted in inflammatory skin disease. Science (2021) 371(6527):eaba6500. doi: 10.3390/sci3040037
12. Nakamizo S, Dutertre CA, Khalilnezhad A, Zhang XM, Lim S, Lum J, et al. Single-cell analysis of human skin identifies CD14+ type 3 dendritic cells co-producing IL1B and IL23A in psoriasis. J Exp Med (2021) 218(9):e20202345. doi: 10.1084/jem.20202345
13. Liu J, Chang HW, Huang ZM, Nakamura M, Sekhon S, Ahn R, et al. Single-cell RNA sequencing of psoriatic skin identifies pathogenic Tc17 cell subsets and reveals distinctions between CD8(+) T cells in autoimmunity and cancer. J Allergy Clin Immunol (2021) 147:2370–80. doi: 10.1016/j.jaci.2020.11.028
14. Hawkes JE, Chan TC, Krueger JG. Psoriasis pathogenesis and the development of novel targeted immune therapies. J Allergy Clin Immunol (2017) 140:645–53. doi: 10.1016/j.jaci.2017.07.004
15. Wang WM, Wu C, Gao YM, Li F, Yu XL, Jin HZ. Neutrophil to lymphocyte ratio, platelet to lymphocyte ratio, and other hematological parameters in psoriasis patients. BMC Immunol (2021) 22:64. doi: 10.1186/s12865-021-00454-4
16. Skrivankova VW, Richmond RC, Woolf B, Yarmolinsky J, Davies NM, Swanson SA, et al. Strengthening the reporting of observational studies in epidemiology using mendelian randomization: The STROBE-MR statement. Jama (2021) 326:1614–21. doi: 10.1001/jama.2021.18236
17. Emdin CA, Khera AV, Kathiresan S. Mendelian randomization. Jama (2017) 318:1925–6. doi: 10.1001/jama.2017.17219
18. Astle WJ, Elding H, Jiang T, Allen D, Ruklisa D, Mann AL, et al. The allelic landscape of human blood cell trait variation and links to common complex disease. Cell (2016) 167:1415–29. doi: 10.1016/j.cell.2016.10.042
19. Siedlinski M, Jozefczuk E, Xu X, Teumer A, Evangelou E, Schnabel RB, et al. White blood cells and blood pressure: A mendelian randomization study. Circulation (2020) 141:1307–17. doi: 10.1161/CIRCULATIONAHA.119.045102
20. Van Hout CV, Tachmazidou I, Backman JD, Hoffman JD, Liu D, Pandey AK, et al. Exome sequencing and characterization of 49,960 individuals in the UK biobank. Nature (2020) 586:749–56. doi: 10.1038/s41586-020-2853-0
21. Bycroft C, Freeman C, Petkova D, Band G, Elliott LT, Sharp K, et al. The UK biobank resource with deep phenotyping and genomic data. Nature (2018) 562:203–9. doi: 10.1038/s41586-018-0579-z
22. Manolio TA. UK Biobank debuts as a powerful resource for genomic research. Nat Med (2018) 24:1792–4. doi: 10.1038/s41591-018-0276-3
23. Choi SW, Mak TS, O'Reilly PF. Tutorial: A guide to performing polygenic risk score analyses. Nat Protoc (2020) 15:2759–72. doi: 10.1038/s41596-020-0353-1
24. Chen MH, Raffield LM, Mousas A, Sakaue S, Huffman JE, Moscati A, et al. Trans-ethnic and ancestry-specific blood-cell genetics in 746,667 individuals from 5 global populations. Cell (2020) 182:1198–213. doi: 10.1016/j.cell.2020.06.045
25. Warren RB, Blauvelt A, Bagel J, Papp KA, Yamauchi P, Armstrong A, et al. Bimekizumab versus adalimumab in plaque psoriasis. N Engl J Med (2021) 385:130–41. doi: 10.1056/NEJMoa2102388
26. Augustin M, Lambert J, Zema C, Thompson E, Yang M, Wu EQ, et al. Effect of risankizumab on patient-reported outcomes in moderate to severe psoriasis: The UltIMMa-1 and UltIMMa-2 randomized clinical trials. JAMA Dermatol (2020) 156:1344–53. doi: 10.1001/jamadermatol.2020.3617
27. Bruins FM, Bronckers I, Groenewoud H, van de Kerkhof P, de Jong E, Seyger M. Association between quality of life and improvement in psoriasis severity and extent in pediatric patients. JAMA Dermatol (2020) 156:72–8. doi: 10.1001/jamadermatol.2019.3717
28. Budu-Aggrey A, Brumpton B, Tyrrell J, Watkins S, Modalsli EH, Celis-Morales C, et al. Evidence of a causal relationship between body mass index and psoriasis: A mendelian randomization study. PloS Med (2019) 16:e1002739. doi: 10.1371/journal.pmed.1002739
29. McEligot AJ, Poynor V, Sharma R, Panangadan A. Logistic LASSO regression for dietary intakes and breast cancer. Nutrients (2020) 12(9):2652. doi: 10.3390/nu12092652
30. Vuckovic D, Bao EL, Akbari P, Lareau CA, Mousas A, Jiang T, et al. The polygenic and monogenic basis of blood traits and diseases. Cell (2020) 182:1214–31. doi: 10.1016/j.cell.2020.08.008
31. Davies NM, Holmes MV, Davey SG. Reading mendelian randomisation studies: A guide, glossary, and checklist for clinicians. BMJ (2018) 362:k601. doi: 10.1136/bmj.k601
32. Emanuelsson F, Marott S, Tybjaerg-Hansen A, Nordestgaard BG, Benn M. Impact of glucose level on micro- and macrovascular disease in the general population: A mendelian randomization study. Diabetes Care (2020) 43:894–902. doi: 10.2337/dc19-1850
33. Kamat MA, Blackshaw JA, Young R, Surendran P, Burgess S, Danesh J, et al. PhenoScanner V2: An expanded tool for searching human genotype-phenotype associations. Bioinformatics (2019) 35:4851–3. doi: 10.1093/bioinformatics/btz469
34. Hemani G, Zheng J, Elsworth B, Wade KH, Haberland V, Baird D, et al. The MR-base platform supports systematic causal inference across the human phenome. Elife (2018) 7:e34408. doi: 10.7554/eLife.34408
35. Ben E, Matthew L, Tessa A, Yi L, Peter M, Jon H, et al. The MRC IEU OpenGWAS data infrastructure. bioRxiv (2020) 10:244291v–244293v. doi: 10.1101/2020.08.10.244293
36. Mitja I, Kurki JKPP. FinnGen: Unique genetic insights from combining isolated population and national health register data. medRxiv (2022). doi: 10.1101/2022.03.03.22271360
37. Burgess S, Butterworth A, Thompson SG. Mendelian randomization analysis with multiple genetic variants using summarized data. Genet Epidemiol (2013) 37:658–65. doi: 10.1002/gepi.21758
38. Bowden J, Davey SG, Burgess S. Mendelian randomization with invalid instruments: Effect estimation and bias detection through egger regression. Int J Epidemiol (2015) 44:512–25. doi: 10.1093/ije/dyv080
39. Bowden J, Davey SG, Haycock PC, Burgess S. Consistent estimation in mendelian randomization with some invalid instruments using a weighted median estimator. Genet Epidemiol (2016) 40:304–14. doi: 10.1002/gepi.21965
40. Hwang LD, Lawlor DA, Freathy RM, Evans DM, Warrington NM. Using a two-sample mendelian randomization design to investigate a possible causal effect of maternal lipid concentrations on offspring birth weight. Int J Epidemiol (2019) 48:1457–67. doi: 10.1093/ije/dyz160
41. Verbanck M, Chen CY, Neale B, Do R. Detection of widespread horizontal pleiotropy in causal relationships inferred from mendelian randomization between complex traits and diseases. Nat Genet (2018) 50:693–8. doi: 10.1038/s41588-018-0099-7
42. Sanderson E, Davey SG, Windmeijer F, Bowden J. An examination of multivariable mendelian randomization in the single-sample and two-sample summary data settings. Int J Epidemiol (2019) 48:713–27. doi: 10.1093/ije/dyy262
43. Mazidi M, Katsiki N, Mikhailidis DP, Sattar N, Banach M. Lower carbohydrate diets and all-cause and cause-specific mortality: A population-based cohort study and pooling of prospective studies. Eur Heart J (2019) 40:2870–9. doi: 10.1093/eurheartj/ehz174
44. Yengo L, Sidorenko J, Kemper KE, Zheng Z, Wood AR, Weedon MN, et al. Meta-analysis of genome-wide association studies for height and body mass index in approximately 700000 individuals of European ancestry. Hum Mol Genet (2018) 27:3641–9. doi: 10.1093/hmg/ddy271
45. Marees AT, de Kluiver H, Stringer S, Vorspan F, Curis E, Marie-Claire C, et al. A tutorial on conducting genome-wide association studies: Quality control and statistical analysis. Int J Methods Psychiatr Res (2018) 27:e1608. doi: 10.1002/mpr.1608
46. Bulik-Sullivan BK, Loh PR, Finucane HK, Ripke S, Yang J, Patterson N, et al. LD score regression distinguishes confounding from polygenicity in genome-wide association studies. Nat Genet (2015) 47:291–5. doi: 10.1038/ng.3211
47. Choi SW, O'Reilly PF. PRSice-2: Polygenic risk score software for biobank-scale data. Gigascience (2019) 8(7):giz082. doi: 10.1093/gigascience/giz082
48. Roszkiewicz M, Dopytalska K, Szymanska E, Jakimiuk A, Walecka I. Environmental risk factors and epigenetic alternations in psoriasis. Ann Agric Environ Med (2020) 27:335–42. doi: 10.26444/aaem/112107
49. Burgess S, Davey SG, Davies NM, Dudbridge F, Gill D, Glymour MM, et al. Guidelines for performing mendelian randomization investigations. Wellcome Open Res (2019) 4:186. doi: 10.12688/wellcomeopenres.15555.1
50. Adamstein NH, MacFadyen JG, Rose LM, Glynn RJ, Dey AK, Libby P, et al. The neutrophil-lymphocyte ratio and incident atherosclerotic events: Analyses from five contemporary randomized trials. Eur Heart J (2021) 42:896–903. doi: 10.1093/eurheartj/ehaa1034
51. Terpos E, Ntanasis-Stathopoulos I, Elalamy I, Kastritis E, Sergentanis TN, Politou M, et al. Hematological findings and complications of COVID-19. Am J Hematol (2020) 95:834–47. doi: 10.1002/ajh.25829
52. Tan D, Fu Y, Tong W, Li F. Prognostic significance of lymphocyte to monocyte ratio in colorectal cancer: A meta-analysis. Int J Surg (2018) 55:128–38. doi: 10.1016/j.ijsu.2018.05.030
53. Griffiths C, Armstrong AW, Gudjonsson JE, Barker J. Psoriasis. Lancet (2021) 397:1301–15. doi: 10.1016/S0140-6736(20)32549-6
54. Weller PF, Spencer LA. Functions of tissue-resident eosinophils. Nat Rev Immunol (2017) 17:746–60. doi: 10.1038/nri.2017.95
55. Hahn M, Ghoreschi K. The role of IL-4 in psoriasis. Expert Rev Clin Immunol (2017) 13:171–3. doi: 10.1080/1744666X.2017.1279054
56. Ghoreschi K, Thomas P, Breit S, Dugas M, Mailhammer R, van Eden W, et al. Interleukin-4 therapy of psoriasis induces Th2 responses and improves human autoimmune disease. Nat Med (2003) 9:40–6. doi: 10.1038/nm804
57. Gibbs BF, Patsinakidis N, Raap U. Role of the pruritic cytokine IL-31 in autoimmune skin diseases. Front Immunol (2019) 10:1383. doi: 10.3389/fimmu.2019.01383
58. Cataldi C, Mari NL, Lozovoy M, Martins L, Reiche E, Maes M, et al. Proinflammatory and anti-inflammatory cytokine profiles in psoriasis: Use as laboratory biomarkers and disease predictors. Inflammation Res (2019) 68:557–67. doi: 10.1007/s00011-019-01238-8
59. Dong Y, Hu H, Fu D, Zheng S, Wang Q, Keshav KC, et al. Serum expression of IL-33 and ST2 in patients with psoriasis vulgaris. Arch Iran Med (2021) 24:689–95. doi: 10.34172/aim.2021.99
60. Michalak-Stoma A, Bartosinska J, Raczkiewicz D, Kowal M, Kozak J, Gujski M, et al. Multiple cytokine analysis of Th1/Th2/Th9/Th17/Th22/Treg cytokine pathway for individual immune profile assessment in patients with psoriasis. Med Sci Monit (2022) 28:e938277. doi: 10.12659/MSM.938277
61. Kahremany S, Hofmann L, Harari M, Gruzman A, Cohen G. Pruritus in psoriasis and atopic dermatitis: Current treatments and new perspectives. Pharmacol Rep (2021) 73:443–53. doi: 10.1007/s43440-020-00206-y
62. Malakou LS, Gargalionis AN, Piperi C, Papadavid E, Papavassiliou AG, Basdra EK. Molecular mechanisms of mechanotransduction in psoriasis. Ann Transl Med (2018) 6:245. doi: 10.21037/atm.2018.04.09
63. Kim HJ, Roh JY, Jung Y. Eosinophils accelerate pathogenesis of psoriasis by supporting an inflammatory milieu that promotes neutrophil infiltration. J Invest Dermatol (2018) 138:2185–94. doi: 10.1016/j.jid.2018.03.1509
64. Aktas KE, Demir D, Aksu CA. Evaluation of monocyte to high-density lipoprotein ratio, lymphocytes, monocytes, and platelets in psoriasis. Bras Dermatol (2020) 95:40–5. doi: 10.1016/j.abd.2019.05.002
65. Wang WM, Jin HZ. Role of neutrophils in psoriasis. J Immunol Res (2020) 2020:3709749. doi: 10.1155/2020/3709749
66. Ternowitz T. Monocyte and neutrophil chemotaxis in psoriasis. relation to the clinical status and the type of psoriasis. J Am Acad Dermatol (1986) 15:1191–9. doi: 10.1016/S0190-9622(86)70289-2
67. Costa MC, Rocha BO, Paixao CS, Oliveira M, Mota L, Carvalho LP. Monocyte subpopulations study in patients with plaque psoriasis. Med Hypotheses (2017) 104:101–3. doi: 10.1016/j.mehy.2017.05.031
68. McGinley AM, Sutton CE, Edwards SC, Leane CM, DeCourcey J, Teijeiro A, et al. Interleukin-17A serves a priming role in autoimmunity by recruiting IL-1beta-Producing myeloid cells that promote pathogenic T cells. Immunity (2020) 52:342–56. doi: 10.1016/j.immuni.2020.01.002
69. Singh TP, Zhang HH, Borek I, Wolf P, Hedrick MN, Singh SP, et al. Monocyte-derived inflammatory langerhans cells and dermal dendritic cells mediate psoriasis-like inflammation. Nat Commun (2016) 7:13581. doi: 10.1038/ncomms13581
70. Rendon A, Schakel K. Psoriasis pathogenesis and treatment. Int J Mol Sci (2019) 20(6):1475. doi: 10.3390/ijms20061475
71. Yamanaka K, Umezawa Y, Yamagiwa A, Saeki H, Kondo M, Gabazza EC, et al. Biologic therapy improves psoriasis by decreasing the activity of monocytes and neutrophils. J Dermatol (2014) 41:679–85. doi: 10.1111/1346-8138.12560
72. Baker KF, Isaacs JD. Novel therapies for immune-mediated inflammatory diseases: What can we learn from their use in rheumatoid arthritis, spondyloarthritis, systemic lupus erythematosus, psoriasis, crohn's disease and ulcerative colitis? Ann Rheum Dis (2018) 77:175–87. doi: 10.1136/annrheumdis-2017-211555
73. Langewouters AM, van Erp PE, de Jong EM, van de Kerkhof PC. Lymphocyte subsets in peripheral blood of patients with moderate-to-severe versus mild plaque psoriasis. Arch Dermatol Res (2008) 300:107–13. doi: 10.1007/s00403-007-0819-9
74. Ito Y, Satoh T, Takayama K, Miyagishi C, Walls AF, Yokozeki H. Basophil recruitment and activation in inflammatory skin diseases. Allergy (2011) 66:1107–13. doi: 10.1111/j.1398-9995.2011.02570.x
75. Ruppenstein A, Limberg MM, Loser K, Kremer AE, Homey B, Raap U. Involvement of neuro-immune interactions in pruritus with special focus on receptor expressions. Front Med (Lausanne) (2021) 8:627985. doi: 10.3389/fmed.2021.627985
Keywords: psoriasis, white blood cells, Mendelian randomization, neutrophil-lymphocyte ratio, platelet-lymphocyte ratio, lymphocyte-monocyte ratio
Citation: Zhou G, Ren X, Tang Z, Li W, Chen W, He Y, Wei B, Zhang H, Ma F, Chen X, Zhang G, Shen M and Liu H (2023) Exploring the association and causal effect between white blood cells and psoriasis using large-scale population data. Front. Immunol. 14:1043380. doi: 10.3389/fimmu.2023.1043380
Received: 13 September 2022; Accepted: 31 January 2023;
Published: 14 February 2023.
Edited by:
Antonio Ji-Xu, University of California, Davis, United StatesReviewed by:
Milena Iwaszko, Polish Academy of Sciences, PolandXianyong Yin, Department of Biostatistics, University of Michigan, Ann Arbor, United States
Copyright © 2023 Zhou, Ren, Tang, Li, Chen, He, Wei, Zhang, Ma, Chen, Zhang, Shen and Liu. This is an open-access article distributed under the terms of the Creative Commons Attribution License (CC BY). The use, distribution or reproduction in other forums is permitted, provided the original author(s) and the copyright owner(s) are credited and that the original publication in this journal is cited, in accordance with accepted academic practice. No use, distribution or reproduction is permitted which does not comply with these terms.
*Correspondence: Hong Liu, aG9uZ2xpdTEwMTRAY3N1LmVkdS5jbg==; Minxue Shen, c2hlbm14MTk4OEBjc3UuZWR1LmNu; Guanxiong Zhang, Z3Vhbnhpb25nX3poYW5nQGNzdS5lZHUuY24=; Xiang Chen, Y2hlbnhpYW5nY2tAY3N1LmVkdS5jbg==; Fangyu Ma, d2F0ZXJ4aWFveXVAMTI2LmNvbQ==
†These authors have contributed equally to this work