- 1Immunology and Infectious Disease Biology, INtegrative GENomics of HOst-PathogEn (INGEN-HOPE) laboratory, CSIR-Institute of Genomics and Integrative Biology (CSIR-IGIB), Delhi, India
- 2Academy of Scientific and Innovative Research (AcSIR), Ghaziabad, India
During an infectious disease progression, it is crucial to understand the cellular heterogeneity underlying the differential immune response landscape that will augment the precise information of the disease severity modulators, leading to differential clinical outcome. Patients with COVID-19 display a complex yet regulated immune profile with a heterogeneous array of clinical manifestation that delineates disease severity sub-phenotypes and worst clinical outcomes. Therefore, it is necessary to elucidate/understand/enumerate the role of cellular heterogeneity during COVID-19 disease to understand the underlying immunological mechanisms regulating the disease severity. This article aims to comprehend the current findings regarding dysregulation and impairment of immune response in COVID-19 disease severity sub-phenotypes and relate them to a wide array of heterogeneous populations of immune cells. On the basis of the findings, it suggests a possible functional correlation between cellular heterogeneity and the COVID-19 disease severity. It highlights the plausible modulators of age, gender, comorbidities, and hosts’ genetics that may be considered relevant in regulating the host response and subsequently the COVID-19 disease severity. Finally, it aims to highlight challenges in COVID-19 disease that can be achieved by the application of single-cell genomics, which may aid in delineating the heterogeneity with more granular understanding. This will augment our future pandemic preparedness with possibility to identify the subset of patients with increased diseased severity.
Introduction
Cellular heterogeneity is defined as an inherent attribute of biological systems that contribute to genetic diversity. Nearly all the cellular systems within an organism are heterogeneous (1). However, there may be differences in the hierarchy of heterogeneity at different levels of expression or regulation. Multicellular organisms undergo specialization, allowing cells to carry out distinct physiological activities. Despite having similar or nearly identical genomic architecture, these cells differentiate in function by retaining diverse (in nature) but specific (in function) gene expression profiles throughout developmental and disease conditions. Hence, it is necessary to unravel the cellular heterogeneity occurring originally via a specific infection toward differentiating healthy and disease states. Therefore, understanding the diversity of disease severity and clinical outcomes, requires uncovering the functional diversity of cells. The outcome modulation by this cellular heterogeneity can imply distinct functionality necessary during a diseased state for survival conditions (2).
There is a diverse array of factors that regulate cellular heterogeneity. Variable biochemical processes, such as stochastic gene and protein expression, functional variances in cell development or cell cycle status, and tissue micro-environmental alterations, are the fundamental sources of cellular heterogeneity (3, 4). Cellular heterogeneity can also be triggered by intrinsic factors such as genetic mutations during transcription and translation, genotype mediated cell switching, or extrinsic factors such as environmental induced adaptive transformation (5, 6). This leads to cell–cell variations that promote functional heterogeneity within the cell population. The competence to extensively characterize cellular heterogeneity is critical for furthering our understanding of cell activity and disease causes. However, because of the complexity in cell diversity, it becomes a cumbersome task to identify significant and specific cellular subgroups that may be responsible for several infectious diseases.
In recent times, several high-throughput sequencing approaches have enabled study of cellular heterogeneity across infectious diseases. Next-generation sequencing (NGS) platforms have been critical toward this as they generate huge amounts of data (7). With bulk NGS analysis, millions of cells can be sequenced at once, but the understanding of distinct cell and tissue type is compromised vis-à-vis extent and functional role of cell heterogeneity (8). During the last few years, single-cell NGS (scNGS) has potential to alleviate limitations associated with the bulk NGS by allowing sequences to be linked to a single cell at the proteomic, epigenomic, transcriptomic, and genetic levels (9).
This article aims at highlighting the role of cellular heterogeneity across infectious disease, particularly the COVID-19. This includes the different levels of immune response defining the host’s response with disease phenotype and correlation of the heterogeneous population of immune cells with COVID-19 disease severity and clinical outcome. It provides a compendium of insights on the possible clinical outcome of a disease from the cellular heterogeneity perspective, based on the clinical phenotypes.
Cellular heterogeneity across infectious diseases
COVID-19 disease severity sub-phenotype
While understanding the infectious diseases, COVID-19 pandemic has surpassed the previous known global infectivity and is one of the major infectious diseases of the era. The single-stranded RNA virus, severe acute respiratory syndrome coronavirus 2 (SARS-CoV-2), causes COVID-19, which is a heterogeneous disease with a variable range of severity symptoms. The clinical presentation of COVID-19 varies from predominantly asymptomatic and mild-to-moderate episodes to more severe and critical, where 10%–20% of patients develop acute respiratory distress syndrome (ARDS) and pneumonia (10, 11). Although the viral genetic diversity, genetic evolution, variable infectivity, or co-pathogenesis contribute to infectivity and fatality, there are missing links to explain the observed diversity of disease heterogeneity for COVID-19. It seems that an important contribution toward the disease heterogeneity modulation is by the human host immune response itself (12).
The COVID-19 disease severity sub-phenotype is categorized by World Health Organization (WHO) into asymptomatic, mild, moderate, severe, and critical, where there is a clear distinction based on clinical symptoms that they exhibit (Table 1) (Clinical management of COVID-19: interim guidance, WHO). Most of the SARS-CoV-2–infected individuals display a mild form of disease that is generally asymptomatic, whereas a few people progress toward a severe or critical phase that necessitates intensive care unit admissions. In addition to the diverse clinical symptoms, the SARS-CoV-2–infected individuals also manifest differential immune responses. Studies have reported that most patients with severe COVID-19 have elevated plasma levels of pro-inflammatory cytokines, interleukin-6 (IL-6), and IL-1β, along with monocyte chemoattractant protein 1 (MCP-1), interferon gamma (IFN-γ)–induced protein 10 (IP-10), and granulocyte colony-stimulating factor (G-CSF) (10). Severe patients have reported elevated levels of inflammatory neutrophils and monocytes, a dramatic decrease in lymphocytes, and an inflammatory environment including IL-1, IL-6, and tumor necrosis factor (TNF) (previously known as TNFα) (13–16). Pro-inflammatory cytokines such TNF, MCP-1 (CCL2), and macrophage inflammatory protein 1a (CCL3) were found to be present at higher levels in severe cases, indicating a robust inflammatory response (10). Further research demonstrated a unique cytokine response with chemokine-enriched signature and activated IL-1 and IL-6 pathways (17, 18). Patients with severe COVID-19 compared with mild patients and healthy individuals revealed a reduced frequency of T cells, accompanied by an increased frequency of monocytes (19). The presence of a high concentration of pro-inflammatory cytokines over the course of disease in severe patients, whereas its lower concentration in patients with mild symptoms, suggests an innate signature shift between the early and late stages of the disease, leading to a divergence of patients into mild and severe COVID-19 over the disease course (19).
Although there is an increasing amount of knowledge on the host immune response to SARS-CoV-2 infection and the pathogenesis of COVID-19, it is still not apparent why some patients progress to severe illness, whereas others present mild symptoms or are asymptomatic. Therefore, it is crucial to define the immunological and inflammatory components of SARS-CoV-2 infection in great detail. The rationale behind different clinical outcomes based on immune profiles of patients infected with similar viral strain could be possibly due to cellular heterogeneity (Figure 1).
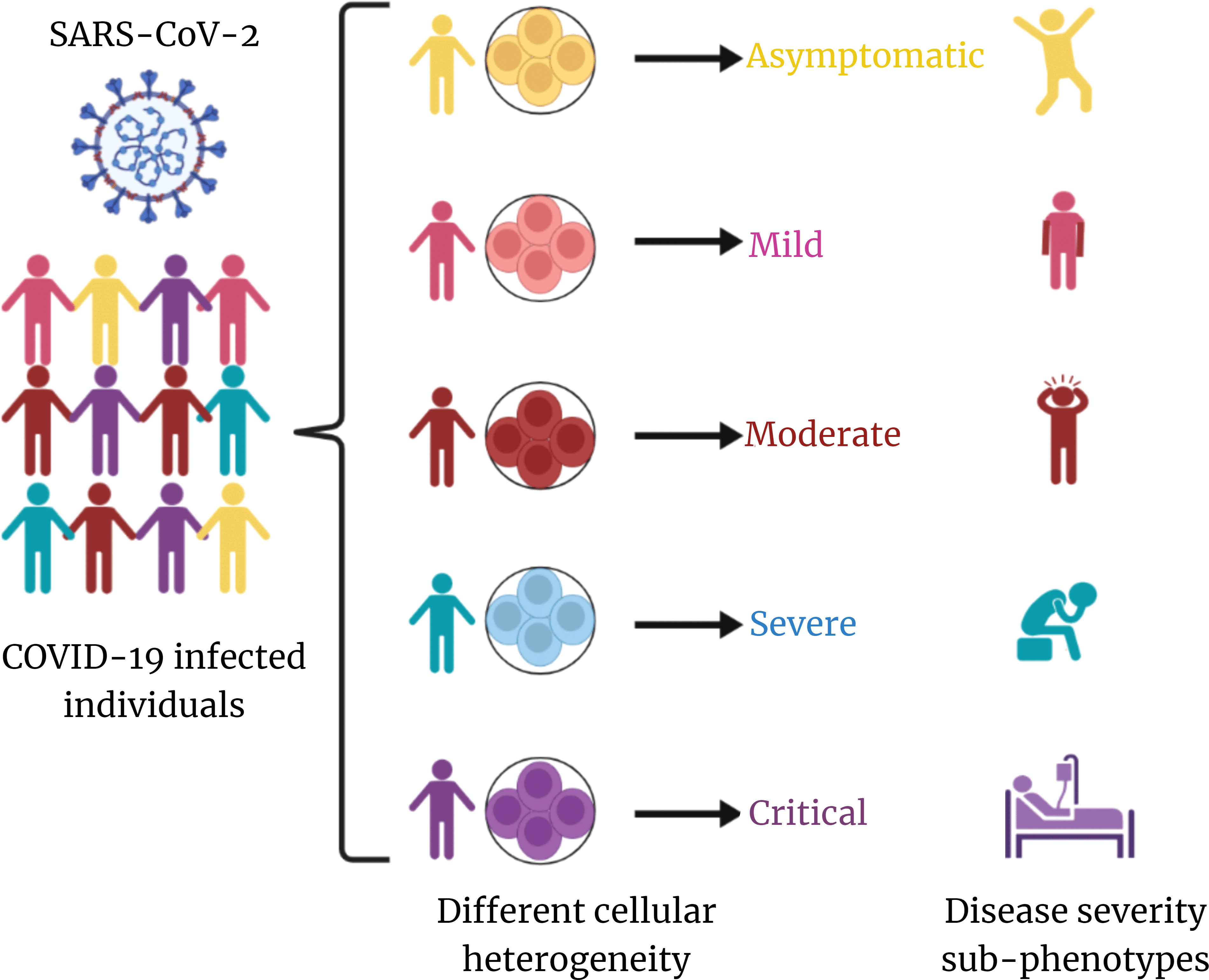
Figure 1 Overview of cellular heterogeneity across COVID-19 disease. SARS-CoV-2–infected individuals exhibit distinct cellular heterogeneity, which is contributing toward the diversity in COVID-19 disease severity sub-phenotypes. This is at the core of differential clinical outcome of recovered and mortality albeit infected by same/similar clade/lineage of the virus.
Different layers of cellular heterogeneity in COVID-19
The role of cellular heterogeneity is essential to understand the mechanism by which a complex network of billions of specialized immune cells functions in harmony and produces a coordinated host response against COVID-19. During a viral infection, there are two different layers of the immune system, i.e., innate and adaptive, which functions one after the other to restrict the entry of virus or prevent it from bypassing the host’s immune system (20, 21). The innate immune system provides the first line of defense during the initial phase of viral infection, which is followed by the activation of adaptive immune response. The similar response has been observed during COVID-19 as well, where the two defense systems attempt to diminish the deleterious effects caused during and after the SARS-CoV-2 infection.
Innate immune response
Innate immune cells carry pathogen recognition receptors (PRRs) on their surface that, upon viral entry, trigger inflammatory signals through recognition and binding of harmful viral particles that behave as pathogen-associated molecular patterns. On the other hand, intrinsic compounds, such as heat shock proteins, are released from viral-infected or damaged cells that act as damage-associated molecular patterns and activate innate immune and inflammatory responses by interacting with PRRs. Nucleotide-binding oligomerization domain, Toll-like receptors (TLRs), C-type lectin receptors, a retinoic acid–inducible gene I–like receptors (RLRs), and absent in melanoma 2–like receptors are the most prevalent PRRs in the host (22). Among these receptors, RLRs and TLRs are two crucial receptors for detecting RNA viral infection. These receptors are known to activate signaling-related pathways, such as IFN signaling, cytokine production, and cell death in response to the SARS-CoV-2 infection (23). However, SARS-CoV-2 counteracts host’s innate immune defenses by encoding proteins and mechanisms that ultimately leads to hyperactivation of the innate immune response, which is a hallmark of COVID-19 severity (24). Recent studies have revealed several mechanisms employed by SARS-CoV-2 to restrict the overall cellular antiviral state, simultaneously leading to hyperactivation of the innate immune response. This involves the following: prevention of PRRs’ sensing of viral RNA by encoding non-structural proteins; inhibition of PRR-mediated signaling pathways by encoding structural proteins (M, membrane; N, Nuclear); and viral protease-mediated cleavage by encoding two proteases (PLpro and 3Clpro), which negatively impacts the innate immune system (25–28).
The innate immune cells are the primary target for successful SARS-CoV-2 infection (29). Macrophages, monocytes, neutrophils, and natural killer (NK) cells are the major types of innate immune cells that are known to activate the downstream signaling of inflammatory response during COVID-19 (23). However, the outcome among COVID-19 disease sub-phenotypes is unique, which indicates the presence of a heterogeneous population of immune cells functioning in response to the SARS-CoV-2 infection. According to previous studies, patients with severe COVID-19 have decreased abundance of monocytes dendritic cells (DCs) and NK cells together with increased proportions of neutrophils, macrophages, and monocyte-derived suppressor cells when compared with patients with mild COVID-19 (30–35). Increase in myeloid-derived suppressor cell population is known to suppress the T-cell functions, leading to the dampening of excessive immune response and simultaneously the inflammatory phenomenon (36). This brings out the diversity within a major class of innate immune cells responding toward sub-phenotypes of COVID-19.
Furthermore, a heterogeneous understanding of innate immune cells can help in functional understanding of the clinical outcome with a dysregulated immune profile of individuals affected with SARS-CoV-2. Xu et al. observed a significant difference among the myeloid cells (monocytes and DCs) composition between patients with severe and mild COVID-19. This was supported by an increased CD14+ monocyte population and decreased CD16+ non-classical monocyte, CD14+CD16+ monocytes, and DC2 in severe patients compared with mild patients and healthy individuals (37). Whereas classical CD14+ monocyte is critical for initiating initial inflammatory response, non-classical monocytes are majorly involved in immune surveillance (38, 39). In a study, inflammatory HLA-DRhiCD11chiCD14+ monocytes were abundant in individuals with mild COVID-19 whereas the severe patients exhibited decreased HLA-DR expression (40). The expression of HLA-DR is a pro-inflammatory sign, where an increased population of inflammatory HLA-DRhiCD11chiCD14+ monocytes in patients with mild COVID-19 suggests the activation of the innate immune response, whereas its reduced expression may lead to immune suppression in patients with severe COVID-19. This decrease in monocyte subpopulations indicates a monocyte dysfunction that may be caused due to the presence of increased levels of IL-6 in patients with severe COVID-19 (41). The macrophages also revealed heterogeneity, where monocyte-derived macrophages were abundant in severe patients, thereby exhibiting hyper-inflammatory conditions (34, 42). Monocyte-derived macrophages have also been linked as a possible source of pro-inflammatory cytokines, TNF, CXCL-8, IL-1, and IL-8 during severe COVID-19, therefore suggesting a strong association between monocyte-derived macrophages and COVID-19 disease severity (37, 43). The upregulation of pro-inflammatory genes in innate immune cells of severe patients mainly belongs to the Nuclear factor kappa-light-chain-enhancer of activated B cells (NF-kB) pathway (44). Therefore, an excess of pathogenic inflammatory neutrophils and macrophages may result from the buildup of NF-kB–dependent pro-inflammatory mediators, and these cells may continue to release pro-inflammatory cytokines and chemokines, including CCL2, CCL3, CCL5, CCL8, TNF, CXCL2, CXCL8, CXCL9, CXCL16, IL-1, IL-17, IL-1RA, IFN-γ, IP-10, MCP-1, G-CSF, and GM-CSF (10, 18, 45–47). A significant increase in the immature neutrophil population, which show evidence of recent activation including increased surface expression of CD64, RANK, RANKL, PD-L1, and reduced CD62L expression, suggests a suppressive character of neutrophils in severe COVID-19 (40). NK-cell subpopulation consists of two subsets based on their relative surface expression of CD56 and CD16 receptors. The CD56brightCD16neg NK-cell subset majorly produces cytokines, and the CD56dimCD16pos NK-cell subset is characterized by strong cytotoxicity and high expression of inhibitory receptors such as killer cell immunoglobulin-like receptors. (48). Among these NK-cell subsets, the frequency of CD56brightCD16neg NK cells has been reported to be depleted in severe patients, suggesting their involvement in mediating the COVID-19 disease severity (49). In contrast, reduced NK-cell subpopulation within severe revealed a reduced CD16 expression (33), which indicates increased NK effector activity and overproduction of cytokines like IFN-γ in response to IL-12, IL-15, and IL-18 stimulation (50). Zheng et al. reported decreased NK-cell subpopulation due to an increased expression of NKG2A, an inhibitory molecule, leading to functional exhaustion of NK cells and elevated cytotoxicity in patients with COVID-19 as compared with healthy (51). It can be suggested that inhibitory checkpoint receptors play a crucial role in reducing the activity of NK cells in patients with COVID-19. In summary, the innate immune response in patients with COVID-19 is divergent, where heterogeneous population of cells behaves differently across the disease sub-phenotypes, thereby contributing toward the diversity of clinical outcomes.
Adaptive immune response
In contrast to the innate mechanism of host defense, the adaptive immune system exhibits specificity for its target antigen. Humoral and cellular immunity forms the adaptive or acquired immune response, where B and T lymphocytes, respectively, provide antigen-specific responses (52). The ability of B cells to mature into plasma cells, which produce a large antibody repertoire to defend against a viral pathogen, as well as the development of immunological memory to prevent recurring infections with the characterization of lymphocytes’ roles, occurs during a viral infection (53). Naïve B cells, mature lymphocytes, memory B cells, transitional B cells, and antibody-secreting plasmablasts/plasma cells are among the several circulating human B cells, each having its own phenotypic and functional subgroups. On the other hand, T lymphocytes, upon viral entry, release mainly CD4+ T cells (helper T cells) and CD8+ T cells (cytotoxic T cells), leading to a combined antiviral immune response (54, 55).
Su et al. reported that, during COVID-19, several B-cell subsets, including naive B cells and antibody secreting cells, were elevated in severe patients, whereas memory B cells were increased in mild patients, suggesting a heterogeneous B-cell population across COVID-19 disease sub-phenotypes (55). Patients with severe COVID-19 exhibited expansion of plasmablasts along with elevated levels of SARS-CoV-2 spike receptor binding domain (RBD)–specific IgM and IgG compared with the healthy individuals, indicating an altered B-cell subset with a strong SARS-CoV-2–specific humoral response (33). Among memory B cells, the frequency of both class-switched and non–class-switched memory B cells is found to be significantly reduced in severe patients, whereas elevated expression of plasmablasts suggests that the decline in the memory B-cell population might be due to the activation of pre-existing memory cells (coronavirus other than SARS-CoV-2), further differentiating into “atypical” cells (56, 57). An increase of memory B cells in patients with mild COVID-19 demonstrates an effective and protective antibody response against SARS-CoV-2 (58). We can say that the memory B-cell subsets are negatively correlated with COVID-19 disease severity. Similarly, transitional B cells were also observed to decrease with disease severity and, therefore, display a loss of immune-suppressive regulatory B cells with an expansion of effector B-cell subsets (59). Another study also reported elevated plasmablasts, enriched T-bet+ B-cell subset, and decreased memory B-cell subsets in severe patients (60). An immunopathologic function for circulating antibody-secreting cells in severe COVID-19 is suggested by the fact that severe patients, compared with mild patients, showed increased levels of antibody-secreting cells (61). Together, these findings indicate that the dynamic B-cell heterogeneity might be modulating COVID-19 disease toward severity.
T lymphopenia has been reported in patients with COVID-19 through multiple studies. Both CD4+ T-cell and CD8+ T-cell populations have been shown to be reduced in severe patients compared with moderate or mild patients (62). There is a negative correlation between the CD4+ T cells and COVID-19 severity, where the extended absence of SARS-CoV-2–specific CD4+ T cells is associated with severe or critical COVID-19 (63). CD4+ T-helper cell subsets also display heterogeneity across COVID-19 disease severity sub-phenotypes, where critical patients report decreased proportion of T-helper 1 (Th1) and T-helper 17 (Th17) lymphocytes, whereas T-helper 2 (Th2) cells’ percentage increased in comparison with less severe individuals (64). It leads to a lower Th1/Th2 ratio that indicates a dysregulated balance of T-helper lymphocytes, wherein decreased Th1/Th2 cell ratio and Th17 cells suggest a reduced humoral response and poor outcomes in COVID-19 disease (65). As Th1 response is essential for viral clearance, lowering of this response and increase of Th2 plausibly indicates an abnormal cellular immune response in patients with severe COVID-19. CD4+ T-cell populations are also associated with the elevated expression of exhaustive markers such as Tim-3, PD-1, and LAG-3, which aids in the progression of disease severity (66). This indicates a link toward discordant CD4+ T-cell responses, which is a likely key element in the development of severe COVID-19. An altered T-cell differentiation and cytotoxicity has been identified by Cervantes et al., wherein circulating cytotoxic CD8+ T cells have been found in higher percentage in severe patients compared with the mild patients (33). Although the absolute number of CD8+ T cells decreases in patients with severe COVID-19, the increased proportion of granzyme B and perforin produced via effector CD8+ T cells provides evidence of elevated cytotoxicity induced in severe patients (67, 68). Furthermore, a pronounced expression of inhibitory markers such as PD-1, Tim-3, and CD39 on CD8+ T cells in severe patients demonstrates an exhausted CD8+ T-cell population that may lead to uncontrolled cytotoxicity response, reduced cellular immunity, and tissue damage with COVID-19 disease severity (69–71). On the other hand, patients in their initial stage show a robust cellular immune response, where lower cytotoxicity and reduced exhaustive marker expression are observed, thereby suggesting a crucial role of overexpressed exhausted T-cell subpopulation in worsening COVID-19 disease severity (72). An association between hyperactivated antigen-specific T cells and COVID-19 disease pathogenesis is supported by the presence of SARS-CoV-2–specific CD8+ T cells in severe patients exhibiting elevated expression of cytotoxic and inflammatory genes, as well as greater levels of TCR clonal expansion (73). In contrast, a reduced cytokine production via CD8+ T-cell population in severe patients is also known. Similar to CD4+ T cells, there is evidence of functional disbalance due to increased expression of exhaustive markers by CD8+ T-cell population in patients with COVID-19 (71). CD8+ T cells from patients with severe COVID-19 produced less cytokine when stimulated (51). The study also suggests that CD8+ T cells exhibit a hyperactivation profile with enhanced cytotoxicity (33). These findings provide evidence for heterogeneous and diverse patterns of CD8+ T-cell responses across patients with COVID-19. Therefore, it can be concluded that, even in patients with severe COVID-19, a significant heterogeneity occurs due to differences in the expression of the T-cell population, leading to diverse clinical outcomes. Together, COVID-19 disease demonstrates a major impact on the innate and adaptive immune system (Table 2), where the response is a variable, thus reflecting a diversified outcome across the infected population (Figure 2).
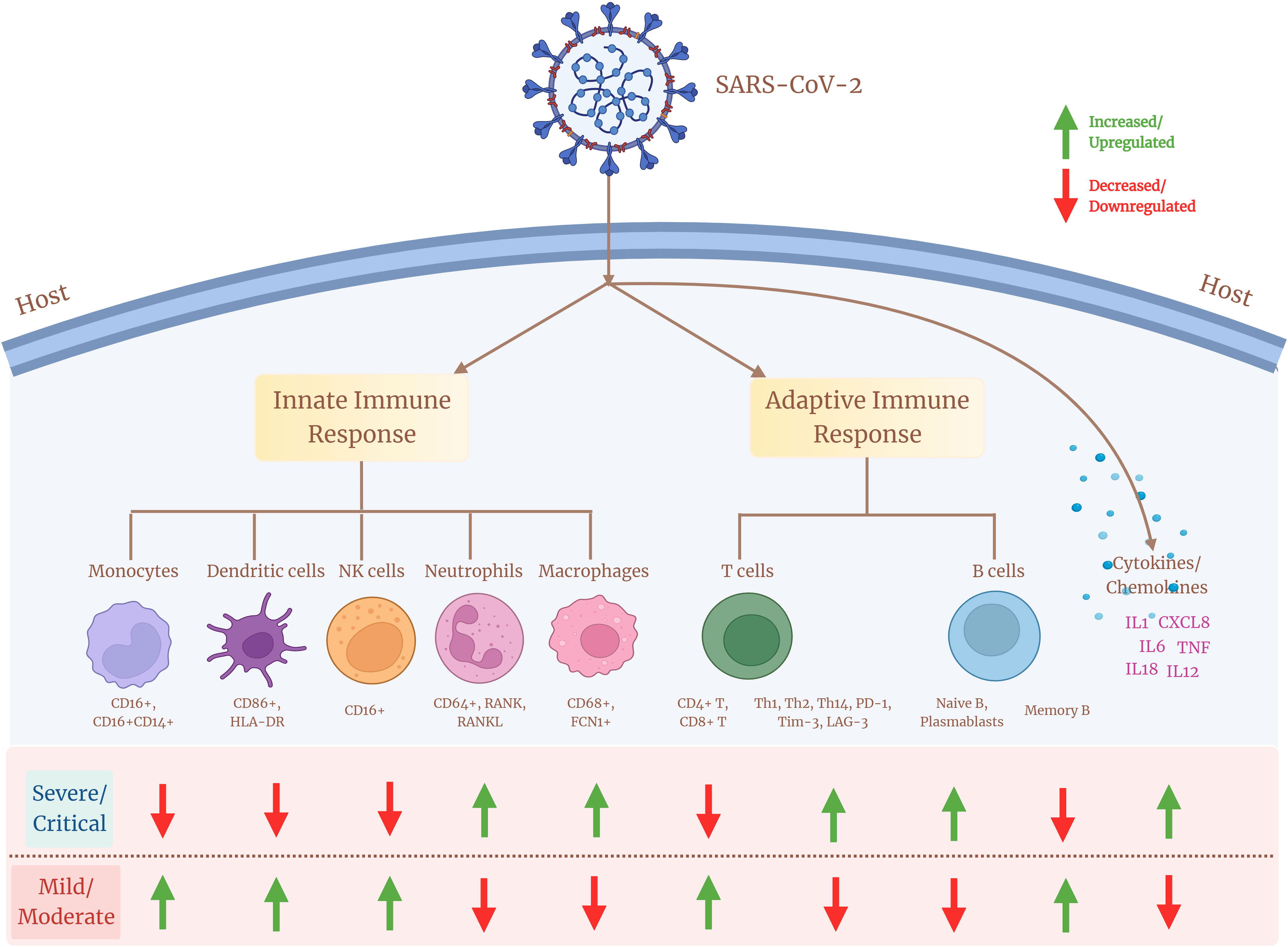
Figure 2 Cellular heterogeneity across the immune landscape of COVID-19 sub-disease severity phenotypes. Different cell subsets of the innate and adaptive immune system and their associated marker expression (upregulation/downregulation) showing diverse heterogeneity across patients with mild/moderate and severe/critical COVID-19.
Correlation between disease severity and immune cell heterogeneity
SARS-CoV-2 has been associated with dynamic immune response, which seems to be at the root of the differential disease severity and clinical outcomes in the infected individuals globally. The presence of immune cell heterogeneity, both innate and adaptive response, during COVID-19 indicates a strong link with the disease severity, which is observed extensively. The COVID-19 severity has been correlated with several granular parameters of immature neutrophils, dysregulated myeloid cell compartments, and increased proliferative CD4+ and CD8+ T cells with heightened cytokine storm (77, 78). With increase in COVID-19 severity, the CD8+ T effector and central memory cells are compromised for their clonotype expansion (79). This suggests an overall decrease in the lymphocyte population with increasing disease severity. However, higher expression of CD8+ T cells is supported by activation of exhaustive markers, which is elevated with the progression of the disease, indicating a SARS-CoV-2–driven T-cell exhaustion in severe patients (69).
Apart from the cell mediated immune response, the correlation between COVID-19 and humoral immunity is also diverse, where higher antibody titers are associated with severe clinical manifestations. Elevated levels of anti-N IgG and IgM antibodies contribute toward the disease severity among patients with fatal COVID-19 (80). Tan et al. reported higher viral clearance rate by weak responders of IgG, whereas strong responders of IgG had lower viral clearance, thereby suggesting that a stronger antibody response supports delayed viral clearance and severity (81). It is also shown that patients with mild to moderate COVID-19 exhibit a rapid decline in the SARS-CoV-2–specific antibody titer and thus indicate a correlation between longevity of the antibody responses with disease severity (82, 83). The presence of increased anti-spike and anti-RBD antibody levels with elevated expression of pro-inflammatory markers in severe patients indicates a correlation between elevated antibody response and inflammation (84). Although increased expression levels of several cytokines and chemokines mediated via monocytes, macrophages, DCs, NK cells, or neutrophils have been observed within the severe patients (13, 18), only a few among them such as CRP, CXCL8, CCL20, IL-6, IL-8, IL-10, PTX3, MCP-3, and IP-10 (85–88) serve as “biomarkers” and indicate a “cytokine storm” underlying COVID-19 severity. Hence, the wide range of impact of COVID-19 on the immune cell population explains the heterogeneous complexity underlying the seriousness of infectious disease and its correlation with disease severity.
Possible modulators of COVID-19 severity
Effect of age
Aging generates significant biochemical changes in the immune system, which have been linked to age-related disorders and infectious disease vulnerability (89–91). The impact of COVID-19 is associated with age, where the severity is observed in elderly patients compared with young adults (92, 93). Studies show that older patients with COVID-19 had higher rates of lymphopenia and the elevation of inflammatory markers such as IL-6 and CRP, which are significantly correlated with disease severity (94). Age-related imbalances in immune response and cellular activity tend to reduce innate activation of a previously weakened adaptive immune system. This chronic long-term stimulation of the innate with a reduced adaptive immune system accentuates aged people toward infection/s. Therefore, the older population has been more susceptible toward COVID-19 and exhibits a diverse immune profile (Table 3). On the other hand, children are less susceptible to becoming SARS-CoV-2–infected, which might be due to cross-immune protection from other coronaviruses or non-specific protection induced by other respiratory viruses (101–103).
Effect of gender
Worldwide, greater COVID-19 mortality rates have been reported in men than in women (104), suggesting that men may be more susceptible to COVID-19 and progress toward severity. This can be correlated with the presence of stronger innate immune responses in women than in men, which possibly allows for faster viral detection and production of interferon and inflammatory cytokines, leading to faster viral clearance (105). X-chromosome harbors many PRRs genes (TLR-7 and 8), ACE2, and interleukins that confer its association with innate and adaptive immunity. A study highlighted that, compared with women, men lack the extra X-chromosome and are therefore more susceptible toward COVID-19 severity (106). TLRs are also different across both genders, where men have higher TLR-2 and TLR-4 expression, whereas women have higher expression of TLR-3, TLR-7, and TLR-9 (107). Because TLR-4 has a higher binding affinity for S-protein of SARS-CoV-2 (108) and induces cytokine production, it causes severe infection in men. On the other hand, TLR-7 stimulates B cells to increase antibodies and also the production of type I IFN during viral infection, which suggests a better initial response and viral attenuation in women upon SARS-CoV-2 infection (109, 110). Macrophages, DCs, T cells, B cells, and NK cells are among the immune-related cells that express estrogen receptors (ER-alpha and ER-beta), suggesting that the female sex steroid hormone, estrogen, regulates immune-related cells to a certain extent. According to Zafari Zangeneh et al., estrogen or estradiol may play a role in regulating the pro-inflammatory immune response against SARS-CoV-2 infection and thereby increasing the anti-inflammatory and antiviral response (110).
In contrast to estrogen, testosterone has a complex role that makes men more susceptible to COVID-19. Testosterone induces ACE2 expression that further increases TMPRSS2 expression (111). Therefore, higher testosterone levels might be associated with increased SARS-CoV-2 entry and disease severity. High levels of testosterone may also lead to COVID-19 severity in men by increasing neutrophil counts and cytokine production (IL-1, IL-10, and IL-2), changing Transforming growth factor beta (TGF-β) production by immune cells, and lowering antibody production that can possibly induce cytokine storm (112). Contrarily, low testosterone levels are correlated with worse disease outcome and increased production of inflammatory markers in men during COVID-19 (113). Testosterone levels are also linked to T-cell immunological activation and have a significant correlation with CD28 expression (114), therefore indicating that low testosterone levels may hamper the activation of the immune system during SARS-CoV-2 infection. This indicates that testosterone acts as a dual edge sword in modulating the COVID-19 severity and further elucidation would help infer the association between testosterone and COVID-19.
Effect of comorbidities
COVID-19 is also associated with several comorbidities that play crucial roles in modulating the disease severity. According to Zhou et al., almost 50% of patients with COVID-19 in their study had either hypertension, diabetes, or coronary heart disease (115). Another study also reported that hypertension, cardiovascular disease, and diabetes are frequently associated comorbidities in severe patients, which correlates with poor clinical outcomes (116). However, other comorbidities such as cerebrovascular disease, chronic kidney disease (CKD), and other renal diseases have also been associated with severity and mortality in patients with COVID-19 (117). This indicates an overall systemic disruption induced by SARS-CoV-2 infection, where multiple organs apart from the site of infection are affected and responsible for COVID-19 severity. Patients with comorbid COVID-19 were also reported to have an altered immune profile compared with the non-comorbid. Del Valle et al. observed the presence of elevated levels of TNF-α and IL-8 in patients with diabetes, hypertension, and CKD-associated COVID-19, suggesting an elevated pro-inflammatory cytokine in patients having comorbidities (118). Higher expression of IL-6 and CRP with increased oxidative stress has also been observed in patients having diabetes as an underlying comorbidity in COVID-19 (119). Together, these findings suggest a hyperactivation and elevated immune and inflammatory response during COVID-19, having at least one comorbidity that often leads to disease severity.
Effect of genetic factors
Host genetic factors are essential determinant of an infectious disease’s susceptibility and severity. During COVID-19, compared with SARS-CoV-2 virus, the host’s genetic variants have an important contribution toward the progression of the disease severity. Multiple genetic variants, together with candidate causal genes, are known to be associated with SARS-CoV-2 infection susceptibility and COVID-19 severity (Table 4). SLC6A20, one of the genes among the cluster of six genes (SLC6A20, CCR9, CXCR6, FYCO1, and LZTFL1) is present on the 3p21.31 locus and encodes the sodium–imino acid (proline) transporter 1 (SIT1), which is known to interact with the ACE2 receptor (128) and therefore may facilitate the entry of SARS-CoV-2. In particular, the Single nucleotide polymorphism (SNP) rs11385942 is present on the chromosome 3 at 3p21.31 locus and spans the six gene-containing clusters and has been associated with respiratory failure in patients with COVID-19 (120). Hospitalization of patients with COVID-19 and the severity of the disease are associated with a different mutation, rs1886814, in the transcription factor FOXP4 (129). A candidate causal variant rs10774671, present in the splice region of OAS1, has been linked with COVID-19 severity (130). DPP9 is a serine protease encoding gene that is involved in antigen presentation, inflammasome activation, and antiviral signaling (131). Its variant rs2109069 present at 19p13.3 locus is related to pulmonary fibrosis along with COVID-19 severity (132). Blood group A individuals are observed at increased risk, whereas O blood group persons have a protective phenotype. The ABO blood group locus contains an overlapping locus 9q34.2 and identified as susceptibility loci for severe COVID-19 (133).
IL-12, IL-23, and IFN signal transduction, as well as Th1/Th17 cell–dependent immune responses, are determined by the Janus kinase encoding gene TYK2 (134). TYK2 variant rs74956615 has been correlated with severity of COVID-19, where high TYK2 expression is associated with critically ill and hospitalized patients (132). TLR7 is a potent innate immune sensor capable of recognizing viral antigen and immediately inducing interferon and other pro-inflammatory cytokines as an antiviral immune response (135). An X-linked deleterious variant of TLR7 is reported to cause loss of function in male patients with COVID-19, which resulted in low levels of type I IFN by plasmacytoid DCs, leading to an impairment of type I interferon in response to SARS-CoV-2 infection, demonstrating the importance of functional TLR7 in regulating the progression and severity of COVID-19 (136). HLA genes (both HLA-I and HLA-II) are one of the major players involved in antigen presentation to the lymphocytes, further activating the host immune response (137). There have been multiple variants of HLA associated with the COVID-19, among which class I HLA, including HLA-A*11:01, -B*51:01, and -C*14:02, is the significantly prevalent one associated with the worst outcome in patients with COVID-19, indicating the immuno-protective role of HLA in regulating the disease severity (122). In direct consequence toward SARS-CoV-2 infection, two major gene loci are necessary: ACE2 and TMPRSS2. Huo et al. reported an association between ACE2 and TMPRSS2 with genetic susceptibility and COVID-19 disease severity, respectively (138). In SARS-CoV-2–infected individuals, both the alternate allele of rs2285666 for ACE2 gene and the SNP (rs12329760) of TMPRSS2 polymorphisms may serve as predictive model for COVID-19 severity (139). Therefore, we can say that, during COVID-19, the host’s genetic factors and the associated polymorphisms do play a significant role in determining the state of infection and disease severity (Figure 3).
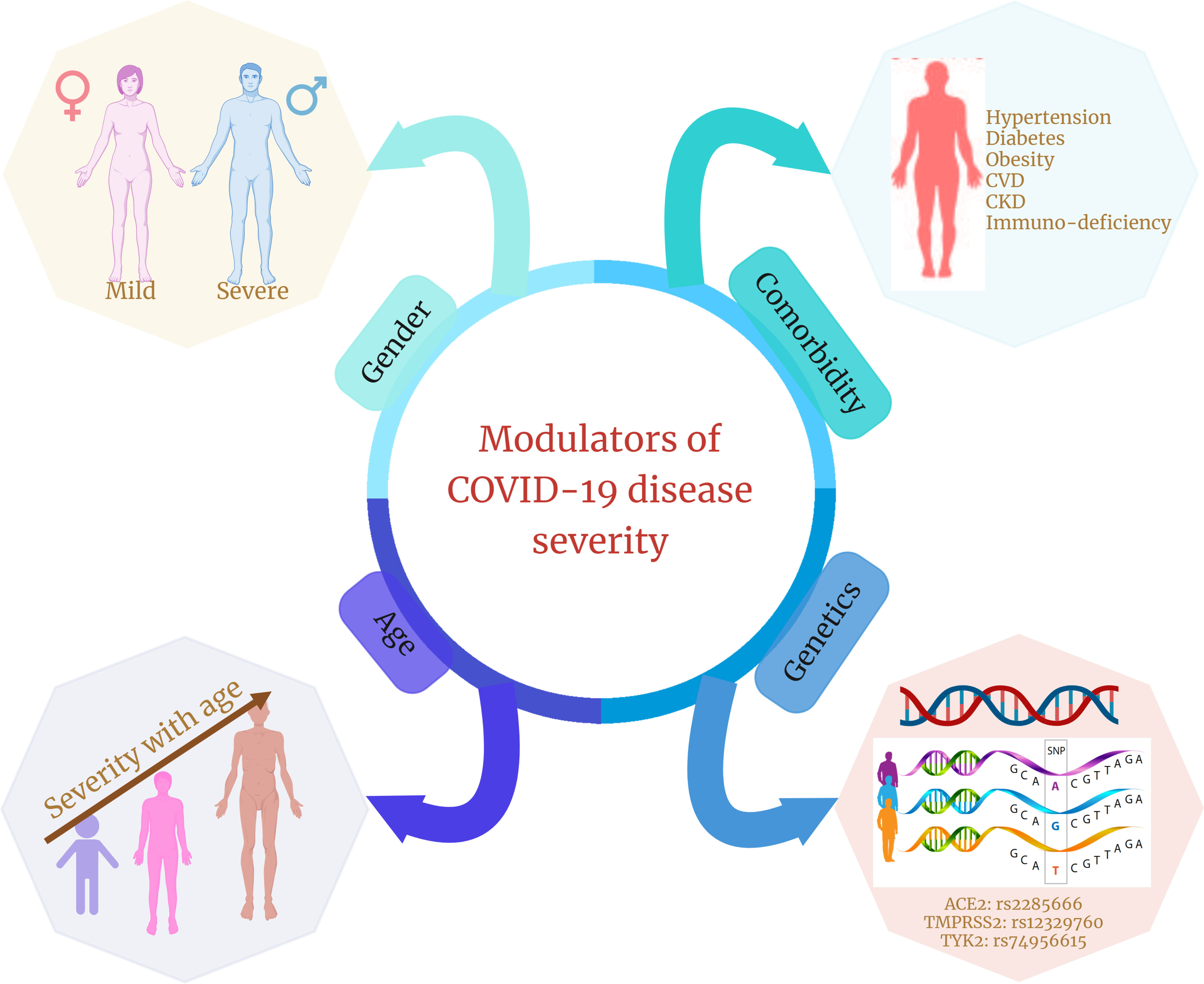
Figure 3 Different modulators of COVID-19 disease severity. Age, gender, presence of comorbidities, and genetic factors are four significant factors that are shown in multiple studies to modulate or are associated with COVID-19 disease severity and clinical outcomes.
Discussion
Cellular heterogeneity has always been at the core for defining spectrum of infectious diseases that can be harnessed to understand the complexity underlying the disease progression. COVID-19 has been a disease of concern that has alarmed the human population globally. This heterogeneous infectious disease has affected a wide range of populations experiencing a diverse array of symptoms. The disparities between the clinical presentation and disease pattern across patients with COVID-19 are possibly due to the presence of immune cell heterogeneity (60). Severe patients reveal a completely different immune profile compared with the ones with mild clinical features. For example, severe patients compared with mild patients exhibit lymphopenia and increased monocyte population with elevated pro-inflammatory cytokines and chemokines (140). This indicates that, during COVID-19, infected individuals experience a major difference in their immune profile that regulates disease progression and affects the host’s survival.
Two levels of the defense program are activated in response to SARS-CoV-2 entry, where each endeavors to avoid any further damage to the host (141). The viral infection triggers the innate and adaptive immune responses, where different cells are programmed to perform their specific function/s in time and space. As the initial line of defense, innate immune cells, such as macrophages, DCs, monocytes, neutrophils, and NK cells, are followed by T and B lymphocytes in the adaptive immune response. Normally, the immune cells are affected during the initial stages of the disease that, with time, increases to limit any further infection. However, this trend is not always followed linearly during COVID-19. The severe patients have decreased abundance of monocytes, NK cells, and DCs, compared with mild patients (142, 143). This indicates a dysregulated myeloid cell compartment during severe COVID-19, which correlates with disease severity and ARDS development (144). However, elevated macrophage and neutrophil cell populations have been associated with overproduction of pro-inflammatory markers—CXCL8, CCL20, IFN-γ, IL-6, IL-1β, and TNF-α—across severe patients (145). These differences within innate immune cells across infected individuals indicate their abnormal expression triggered due to COVID-19, with expected major impact on the cellular landscape. These findings provide crucial information about the presence/absence of specific cell types across infected individuals based on specific markers expressed by these cells. Although there are multiple reports highlighting the upregulation/downregulation of these cell-specific markers, the exact mechanism of these heterogeneous cell populations modulating the immune response during COVID-19 is yet to be known.
Lymphopenia is a hallmark of severe COVID-19, where T- and B-cell populations are decreased with disease severity (146, 147). However, patients reveal cellular heterogeneity, especially within T-cell subsets, where the cell-mediated response is highly diverse within infected individuals. Although severe patients exhibit reduced T-cell population, they also express elevated expression of exhausted markers within T-cell subsets (both CD8+ and CD4+ T cells), suggesting a possible abnormal T-cell response throughout COVID-19 (69, 71). These heterogeneous populations of cells with an abnormal expression within patients with COVID-19 reveal a strong impact of the disease on the immune profile that provides inter-individual differences. We can say that, with disease severity, the heterogeneous immune-cell population is also altered and so is necessary to be taken forward for their functional role in the future studies.
Cellular heterogeneity also defines specific factors that can be considered as possible modulators for COVID-19 disease severity. Aging has always been a concerning issue where individuals fall into the immunocompromised category. These individuals’ immune profile is suboptimal for maintaining a healthy state among elderly, which becomes a major accelerator in COVID-19 severity. Compared with adults, the immune landscape of older individuals exhibits dysregulated innate and adaptive immune cell compartments during COVID-19. This can be correlated with inflammaging and immunoscenesce that are the defining features of infected elderly individuals and therefore indicate the observed COVID-19 severity (148–150). In addition to age, gender is also known to be associated with COVID-19 disease severity. Men, according to studies, are more vulnerable to SARS-CoV-2 infection than women (151). The presence of an extra X chromosome in women and its association with innate and adaptive immune response makes them more protective against COVID-19. Moreover, the immune cell heterogeneity is more pronounced in men as highlighted by increased neutrophil counts, heightened inflammatory response, and reduced antibody production (151, 152). Further understanding of gender-specific immune profiles is necessary to strengthen our understanding of COVID-19 with cellular heterogeneity, where gender may have a major impact on the modulation of disease severity. Specific comorbidities such as hypertension, diabetes, CKD, and cardiovascular disease correlate with higher morbidity and mortality rates in patients with COVID-19 (153). Elevated cytokines and chemokines are observed in comorbid patients compared with non-comorbid, suggesting a major alteration of the immune landscape in patients with comorbid COVID-19 (60, 119). Host’s genetic factors are of immense importance in modulating the COVID-19 disease severity. Numerous genetic variants have been studied to be associated with COVID-19 susceptibility, severity, and clinical outcomes. Among all, the specific variants associated with HLA, ACE2, and TMPRSS2 have significant contribution in modulating the COVID-19 severity (154). A possible explanation for the effect of an HLA allele on disease severity might be abnormal binding affinity with SARS-CoV-2 peptides (155). The HLA-restricted T-cell response mechanism, in which viral epitopes are delivered by DCs to CD8+ T lymphocytes through contact with HLA class I alleles and CD4+ T lymphocytes through interactions with HLA class II alleles, is critical to the human protection mechanism. This interaction is affected in SARS-CoV-2 infection, where HLA variants have lower binding sites for SARS-CoV-2 peptides, leading to a decreased immune response and increased disease severity (156). Moreover, TLR7-, DPP9-, ABO-, OAS1-, and TYK2-associated genetic variants have been correlated with reduced antigen presentation, delayed type I IFN, and heightened cell-mediated immune response in severe patients (18, 157, 158). The respective evidence highlights the relevance of cytokine storm in COVID-19 severity and several complications, including a fatal outcome. Therefore, we can say that identifying genetic factors could aid in explaining the uncontrolled inflammatory response and, if possible, plausible biomarkers defining the COVID-19 severity that can be harnessed for therapeutic strategies.
Challenges and future prospects
An insufficient understanding of cellular heterogeneity within patients with COVID-19 can have considerable implications for prognosis and therapeutic interventions. The disparities in clinical outcomes among COVID-19 individuals have been treated based on the supportive care and close monitoring with treatment intensification for the individuals with worsened symptoms. Hence, more stratified strategies should be opted to identify the patients with the future worse symptoms. It is necessary to dig deeper to understand patient heterogeneity that assists clinicians in utilizing intensive therapy for stratified patients and aiding potentially beneficial treatments required by an individual patient.
Immunological investigations are emerging to reveal insights into patient heterogeneity and clinical variability in patients with COVID-19 that may help understanding the disease trajectories and progression. Both B- and T-cell responses are known to be associated with the COVID-19 disease spectrum. However, the T-cell immune response has widely been shown to have a diversity among infected individuals, highlighting its significance in understanding the disease progression and patient stratification. Vital information on the involvement of T cells for COVID-19 protection is considered necessary and presently awaiting functional elucidation. Longitudinal studies of patients with COVID-19 may help to overcome this limitation by providing a precise understanding of heterogeneity within T cells and T-cell–mediated immune response across patients with COVID-19. It would be crucial to characterize the protective T-cell responses following infection and vaccination. In doing this, the single-cell genomics approach can be helpful in delineating the complex immune profile of infected individuals. Single-cell RNA sequencing (scRNA-seq) is of paramount importance in providing relevant information at single-cell resolution with the highest granularity that can aid in a comprehensive understanding of cellular immune response during COVID-19. Rather than using composites or averages from multiple cells, single-cell approaches offer a high-resolution perspective into these subsets and individual cells. Furthermore, multi-omics profiling of concurrent readouts from the same cell, including gene expression, cell surface proteins, and receptor sequences, enables more accurate and reliable characterization of these heterogeneous cell populations. From evidence within patients with COVID-19, where T-cell population is immensely variable, the single-cell profiling of T-cell subsets can be performed on a clinical cohort to understand insights of T-cell–mediated response and T-cell receptor repertoire dynamics. Detailed characterization of both CD4+ T cells and CD8+ memory T cells using single-cell omics can be helpful in patient categorization and plausible future protection. Understanding from the perspective of genes involved in defining the particular cell states during severe COVID-19 can also help us to elucidate the role of cellular heterogeneity in disease severity. Single-cell omics approach will help address the unresolved query concerning cellular heterogeneity that may assist us in preparing for the future as the SARS-CoV-2 is continuously evolving with acquired immune escape capabilities.
Understanding cellular heterogeneity in COVID-19 can be a cumbersome task as it comes with many challenges (Figure 4). The data generated from scRNA-seq are quite complex and have big volume, and multiple levels of quality check should be taken care of while arriving at the precise result. Often, the cell type identified can be misleading due to its probability of being a false result or noise. Hence, it is crucial to eliminate the noise in the data because each cell type and its expression contribute enormously to delineating patient heterogeneity. Even a low number of cells as input data can also cause a reduction in the discovery of rare cell types that usually are present in a low proportion. To avoid this, cell count as input should be above a threshold to capture even the low percentage of cell types. Generally, annotation of cell clusters is performed manually, which is labor-intensive that reduces the chances of achieving high accuracy in the results. With the help of multiple automated platforms and tools such as SCSA (a cell type annotation tool for single-cell RNA-seq data) and MACA (marker-based automatic cell-type annotation), heterogeneous cell populations may be captured efficiently within a patient (159, 160). Sometimes, the location of a particular cell type in a tissue can also be mistaken, which may lead to wrong interpretation of the spatial and temporal characteristics of the cell. Spatial transcriptomics, together with the scRNA-seq technique, can be performed, which is a high-throughput approach that can assist in providing the exact position with the expression of the cell from the tissue of interest. Gene expression may not provide enough clarity in detecting the granularity of heterogeneous cell populations. Therefore, it can be united with surface marker expression, further aiding elucidation of cellular heterogeneity. Altogether, the solutions toward resolving these challenges associated with single-cell omics technology in the future may help to develop a better understanding of the intricacies associated with cellular heterogeneity and provide substantial insights into the complexity behind COVID-19 disease.
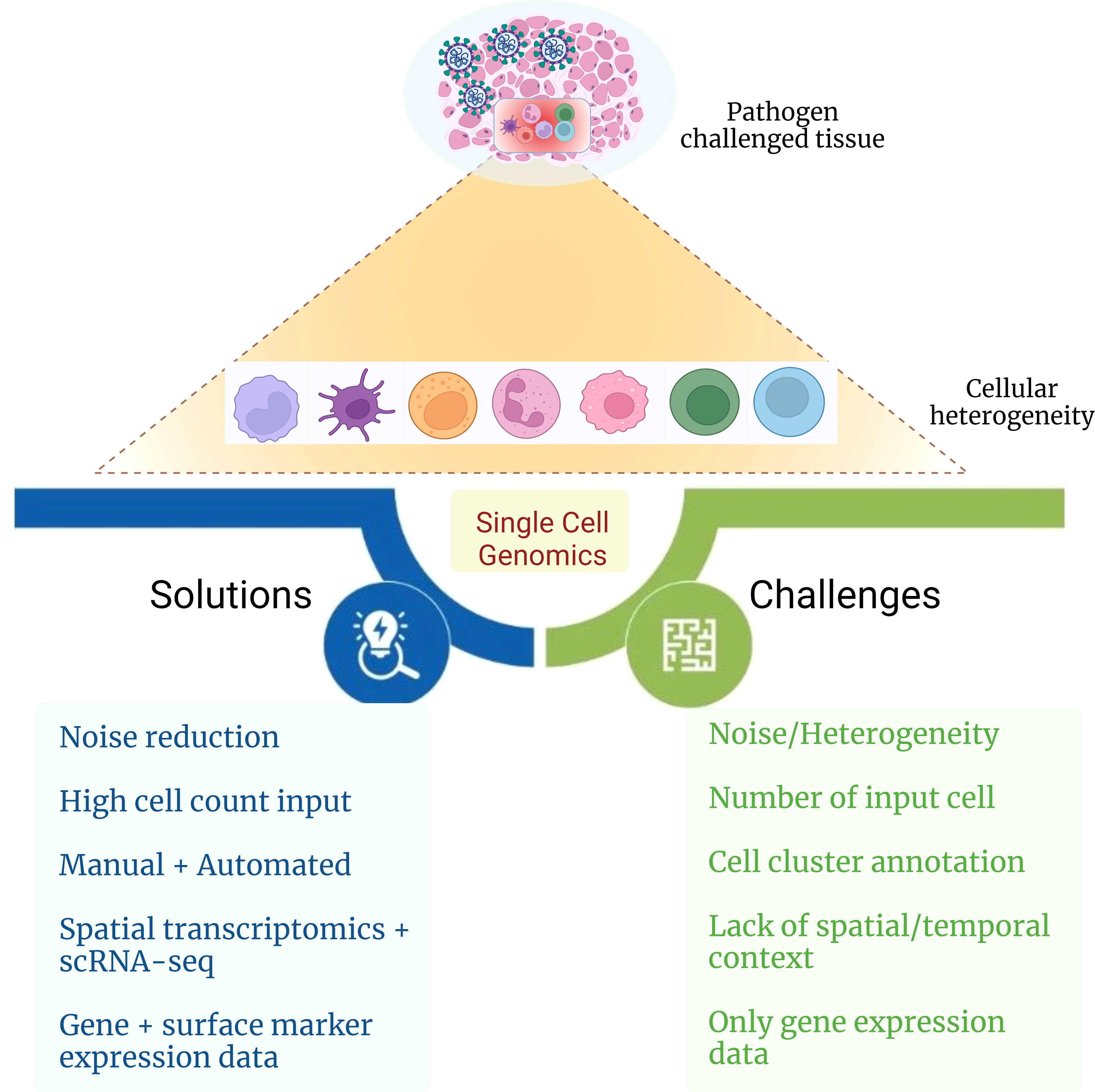
Figure 4 Challenges and possible solutions for delineating cellular heterogeneity. The figure captures challenges and possible solutions toward elucidating the role of cellular heterogeneity during infectious disease with the aid of single-cell genomics approach.
Author contributions
Author contribution KK has read the relevant literature, synthesized the findings, wrote the manuscript and made the figures. RP planned the study, wrote the manuscript, coordinated the manuscript outline, figures, conclusions and future challenges/opportunities. Both the authors contributed to the article, have read and approved the final submission.
Funding
The study was supported by Bill and Melinda Gates Foundation (BMGF), INV-033578, Foundation for Innovative New Diagnostics (FIND), project code – GAP-0249, and AIDS Healthcare Foundation (AHF), project code CLP0043.
Acknowledgments
KK acknowledges the fellowship from CSIR toward her salary support.
Conflict of interest
The authors declare that the article was written in the absence of any commercial or financial relationships that could be construed as a potential conflict of interest.
Publisher’s note
All claims expressed in this article are solely those of the authors and do not necessarily represent those of their affiliated organizations, or those of the publisher, the editors and the reviewers. Any product that may be evaluated in this article, or claim that may be made by its manufacturer, is not guaranteed or endorsed by the publisher.
References
1. Altschuler SJ, Wu LF. Cellular heterogeneity: Do differences make a difference? Cell (2010) 141(4):559–63. doi: 10.1016/j.cell.2010.04.033
2. Gupta RK, Kuznicki J. Biological and Medical Importance of Cellular Heterogeneity Deciphered by Single-Cell RNA Sequencing. Cells (2020) 9(8). doi: 10.3390/cells9081751
3. Wang D, Bodovitz S. Single cell analysis: The new frontier in “omics. Trends Biotechnol (2010) 28(6):281–90. doi: 10.1016/j.tibtech.2010.03.002
4. McClellan J, King M-C. Genetic heterogeneity in human disease. Cell (2010) 141(2):210–7. doi: 10.1016/j.cell.2010.03.032
5. Rosenfeld N, Young JW, Alon U, Swain PS, Elowitz MB. Gene regulation at the single-cell level. Science (2005) 307(5717):1962–5. doi: 10.1126/science.1106914
6. Warrick JW, Timm A, Swick A, Yin J. Tools for Single-Cell Kinetic Analysis of Virus-Host Interactions. PloS One (2016) 11(1):e0145081. doi: 10.1371/journal.pone.0145081
7. Mason CE, Porter SG, Smith TM. Characterizing multi-omic data in systems biology. Adv Exp Med Biol (2014) 799:15–38. doi: 10.1007/978-1-4614-8778-4_2
8. Goldman SL, MacKay M, Afshinnekoo E, Melnick AM, Wu S, Mason CE. The Impact of Heterogeneity on Single-Cell Sequencing. Front Genet (2019) 10:8. doi: 10.3389/fgene.2019.00008
9. Heath JR, Ribas A, Mischel PS. Single-cell analysis tools for drug discovery and development. Nat Rev Drug Discovery (2016) 15(3):204–16. doi: 10.1038/nrd.2015.16
10. Huang C, Wang Y, Li X, Ren L, Zhao J, Hu Y, et al. Clinical features of patients infected with 2019 novel coronavirus in Wuhan, China. Lancet (2020) 395(10223):497–506. doi: 10.1016/S0140-6736(20)30183-5
11. Gupta A, Madhavan MV, Sehgal K, Nair N, Mahajan S, Sehrawat TS, et al. Extrapulmonary manifestations of COVID-19. Nat Med (2020) 26(7):1017–32. doi: 10.1038/s41591-020-0968-3
12. Vabret N, Britton GJ, Gruber C, Hegde S, Kim J, Kuksin M, et al. Immunology of COVID-19: Current State of the Science. Immunity (2020) 52(6):910–41. doi: 10.1016/j.immuni.2020.05.002
13. Chen G, Wu D, Guo W, Cao Y, Huang Da, Wang H, et al. Clinical and immunological features of severe and moderate coronavirus disease 2019. J Clin Invest (2020) 130(5):2620–9. doi: 10.1172/JCI137244
14. Chen N, Zhou M, Dong X, Qu J, Gong F, Han Y, et al. Epidemiological and clinical characteristics of 99 cases of 2019 novel coronavirus pneumonia in Wuhan, China: a descriptive study. Lancet (2020) 395(10223):507–13. doi: 10.1016/S0140-6736(20)30211-7
15. Giamarellos-Bourboulis EJ, Netea MG, Rovina N, Akinosoglou K, Antoniadou A, Antonakos N, et al. Complex Immune Dysregulation in COVID-19 Patients with Severe Respiratory Failure. Cell Host Microbe (2020) 27(6):992–1000.e3. doi: 10.1016/j.chom.2020.04.009
16. Zhou Z, Ren L, Zhang L, Zhong J, Xiao Y, Jia Z, et al. Heightened Innate Immune Responses in the Respiratory Tract of COVID-19 Patients. Cell Host Microbe (2020) 27(6):883–890.e2. doi: 10.1016/j.chom.2020.04.017
17. van de Veerdonk FL, Janssen NAF, Grondman I, de Nooijer AH, Koeken VACM, Matzaraki V, et al. A systems approach to inflammation identifies therapeutic targets in SARS-CoV-2 infection. medRxiv (2020). doi: 10.1101/2020.05.23.20110916
18. Blanco-Melo D, Nilsson-Payant BE, Liu W-C, Uhl S, Hoagland D, Møller R, et al. Imbalanced Host Response to SARS-CoV-2 Drives Development of COVID-19. Cell (2020) 181(5):1036–1045.e9. doi: 10.1016/j.cell.2020.04.026
19. Chevrier S, Zurbuchen Y, Cervia C, Adamo S, Raeber ME, de Souza N, et al. A distinct innate immune signature marks progression from mild to severe COVID-19. Cell Rep Med (2021) 2(1):100166. doi: 10.1016/j.xcrm.2020.100166
20. Aoshi T, Koyama S, Kobiyama K, Akira S, Ishii KJ. Innate and adaptive immune responses to viral infection and vaccination. Curr Opin Virol (2011) 1(4):226–32. doi: 10.1016/j.coviro.2011.07.002
21. Stambas J, Lu C, Tripp RA. Innate and adaptive immune responses in respiratory virus infection: Implications for the clinic. Expert Rev Respir Med (2020) 14(11):1141–7. doi: 10.1080/17476348.2020.1807945
22. Kanneganti T-D. Intracellular innate immune receptors: Life inside the cell. Immunol Rev (2020) 297(1):5–12. doi: 10.1111/imr.12912
23. Diamond MS, Kanneganti T-D. Innate immunity: The first line of defense against SARS-CoV-2. Nat Immunol (2022) 23(2):165–76. doi: 10.1038/s41590-021-01091-0
24. Mazzoni A, Salvati L, Maggi L, Annunziato F, Cosmi L. Hallmarks of immune response in COVID-19: Exploring dysregulation and exhaustion. Semin Immunol (2021) 55:101508. doi: 10.1016/j.smim.2021.101508
25. Ogando NS, Zevenhoven-Dobbe JC, van der Meer Y, Bredenbeek PJ, Posthuma CC, Snijder EJ. The Enzymatic Activity of the nsp14 Exoribonuclease Is Critical for Replication of MERS-CoV and SARS-CoV-2. J Virol (2020) 94(23):e01246–20. doi: 10.1128/JVI.01246-20
26. Fu Y-Z, Wang S-Y, Zheng Z-Q, Huang Yi, Li W-W, Xu Z-S, et al. SARS-CoV-2 membrane glycoprotein M antagonizes the MAVS-mediated innate antiviral response. Cell Mol Immunol (2020) 18:613–20. doi: 10.1038/s41423-020-00571-x
27. Mu J, Fang Y, Yang Q, Shu T, Wang A, Huang M, et al. SARS-CoV-2 N protein antagonizes type I interferon signaling by suppressing phosphorylation and nuclear translocation of STAT1 and STAT2. Cell Discovery (2020) 6:65. doi: 10.1038/s41421-020-00208-3
28. Moustaqil M, Ollivier E, Chiu H-P, Van Tol S, Rudolffi-Soto P, Stevens C, et al. SARS-CoV-2 proteases PLpro and 3CLpro cleave IRF3 and critical modulators of inflammatory pathways (NLRP12 and TAB1): Implications for disease presentation across species. Emerg Microbes Infect (2020) 10(1):178–95. doi: 10.1080/22221751.2020.1870414
29. Schultze JL, Aschenbrenner AC. COVID-19 and the human innate immune system. Cell (2021) 184(7):1671–92. doi: 10.1016/j.cell.2021.02.029
30. Mudd PA, Crawford JC, Turner JS, Souquette A, Reynolds D, Bender D, et al. Distinct inflammatory profiles distinguish COVID-19 from influenza with limited contributions from cytokine storm. Sci Adv (2020) 6(50):eabe3024. doi: 10.1126/sciadv.abe3024
31. Zhou R, To KK-W, Wong Y-C, Liu L, Zhou B, Li X, et al. Acute SARS-CoV-2 Infection Impairs Dendritic Cell and T Cell Responses. Immunity (2020) 53(4):864–877.e5. doi: 10.1016/j.immuni.2020.07.026
32. Maucourant C, Filipovic I, Ponzetta A, Aleman S, Cornillet M, Hertwig L, et al. Natural killer cell immunotypes related to COVID-19 disease severity. Sci Immunol (2020) 5(50):eabd6832. doi: 10.1126/sciimmunol.abd6832
33. Kuri-Cervantes L, Pampena MB, Meng W, Rosenfeld AM, Ittner CAG, Weisman AR, et al. Comprehensive mapping of immune perturbations associated with severe COVID-19. Sci Immunol (2020) 5(49):eabd7114. doi: 10.1126/sciimmunol.abd7114
34. Liao M, Liu Y, Yuan J, Wen Y, Xu G, Zhao J, et al. Single-cell landscape of bronchoalveolar immune cells in patients with COVID-19. Nat Med (2020) 26(6):842–4. doi: 10.1038/s41591-020-0901-9
35. Silvin A, Chapuis N, Dunsmore G, Goubet A-G, Dubuisson A, Derosa L, et al. Elevated Calprotectin and Abnormal Myeloid Cell Subsets Discriminate Severe from Mild COVID-19. Cell (2020) 182(6):1401–1418.e18. doi: 10.1016/j.cell.2020.08.002
36. Veglia F, Sanseviero E, Gabrilovich DI. Myeloid-derived suppressor cells in the era of increasing myeloid cell diversity. Nat Rev Immunol (2021) 21(8):485–98. doi: 10.1038/s41577-020-00490-y
37. Xu G, Qi F, Li H, Yang Q, Wang H, Wang X, et al. The differential immune responses to COVID-19 in peripheral and lung revealed by single-cell RNA sequencing. Cell Discovery (2020) 6:73. doi: 10.1038/s41421-020-00225-2
38. Narasimhan PB, Marcovecchio P, Hamers AAJ, Hedrick CC. Nonclassical monocytes in health and disease. Annu Rev Immunol (2019) 37:439–56. doi: 10.1146/annurev-immunol-042617-053119
39. Rajamanickam A, Kumar NP, Pandiarajan AN, Selvaraj N, Munisankar S, Renji RM, et al. Dynamic alterations in monocyte numbers, subset frequencies and activation markers in acute and convalescent COVID-19 individuals. Sci Rep (2021) 11(1):20254. doi: 10.1038/s41598-021-99705-y
40. Schulte-Schrepping J, Reusch N, Paclik D, Baßler K, Schlickeiser S, Zhang B, et al. Severe COVID-19 Is Marked by a Dysregulated Myeloid Cell Compartment. Cell (2020) 182(6):1419–1440.e23. doi: 10.1016/j.cell.2020.08.001
41. Mazzoni A, Salvati L, Maggi L, Capone M, Vanni A, Spinicci M, et al. Impaired immune cell cytotoxicity in severe COVID-19 is IL-6 dependent. J Clin Invest (2020) 130(9):4694–703. doi: 10.1172/jci138554
42. Grant RA, Morales-Nebreda L, Markov NS, Swaminathan S, Querrey M, Guzman ER, et al. Circuits between infected macrophages and T cells in SARS-CoV-2 pneumonia. Nature (2021) 590(7847):635–41. doi: 10.1038/s41586-020-03148-w
43. Wang J, Jiang M, Chen X, Montaner LJ. Cytokine storm and leukocyte changes in mild versus severe SARS-CoV-2 infection: Review of 3939 COVID-19 patients in China and emerging pathogenesis and therapy concepts. J Leukoc Biol (2020) 108(1):17–41. doi: 10.1002/JLB.3COVR0520-272R
44. Hadjadj J, Yatim N, Barnabei L, Corneau A, Boussier J, Smith N, et al. Impaired type I interferon activity and inflammatory responses in severe COVID-19 patients. Science (2020) 369(6504):718–24. doi: 10.1126/science.abc6027
45. Wang N, Zhan Y, Zhu L, Hou Z, Liu F, Song P, et al. Retrospective Multicenter Cohort Study Shows Early Interferon Therapy Is Associated with Favorable Clinical Responses in COVID-19 Patients. Cell Host Microbe (2020) 28(3):455–464.e2. doi: 10.1016/j.chom.2020.07.005
46. Yang D, Chu H, Hou Y, Chai Y, Shuai H, Lee AC-Y, et al. Attenuated Interferon and Proinflammatory Response in SARS-CoV-2-Infected Human Dendritic Cells Is Associated With Viral Antagonism of STAT1 Phosphorylation. J Infect Dis (2020) 222(5):734–45. doi: 10.1093/infdis/jiaa356
47. Wu D, Yang XO. TH17 responses in cytokine storm of COVID-19: An emerging target of JAK2 inhibitor Fedratinib. J Microbiol Immunol Infect (2020) 53(3):368–70. doi: 10.1016/j.jmii.2020.03.005
48. Fan Y, Yang B, Wu C. Phenotypically and functionally distinct subsets of natural killer cells in human PBMCs. Cell Biol Int (2008) 32(2):188–97. doi: 10.1016/j.cellbi.2007.08.025
49. Varchetta S, Mele D, Oliviero B, Mantovani S, Ludovisi S, Cerino A, et al. Unique immunological profile in patients with COVID-19. Cell Mol Immunol (2021) 18(3):604–12. doi: 10.1038/s41423-020-00557-9
50. van Eeden C, Khan L, Osman MS, Cohen Tervaert JW. Natural Killer Cell Dysfunction and Its Role in COVID-19. Int J Mol Sci (2020) 21(17):6351. doi: 10.3390/ijms21176351
51. Zheng M, Gao Y, Wang G, Song G, Liu S, Sun D, et al. Functional exhaustion of antiviral lymphocytes in COVID-19 patients. Cell Mol Immunol (2020) 17(5):533–5. doi: 10.1038/s41423-020-0402-2
52. Chaplin DD. Overview of the immune response. J Allergy Clin Immunol (2010) 125:S3–S23. doi: 10.1016/j.jaci.2009.12.980
53. Abbott RK, Crotty S. Factors in B cell competition and immunodominance. Immunol Rev (2020) 296(1):120–31. doi: 10.1111/imr.12861
54. Jansen JM, Gerlach T, Elbahesh H, Rimmelzwaan GF, Saletti G. Influenza virus-specific CD4+ and CD8+ T cell-mediated immunity induced by infection and vaccination. J Clin Virol (2019) 119:44–52. doi: 10.1016/j.jcv.2019.08.009
55. Su Y, Chen D, Yuan D, Lausted C, Choi J, Dai CL, et al. Multi-Omics Resolves a Sharp Disease-State Shift between Mild and Moderate COVID-19. Cell (2020) 183(6):1479–1495.e20. doi: 10.1016/j.cell.2020.10.037
56. De Biasi S, Lo Tartaro D, Meschiari M, Gibellini L, Bellinazzi C, Borella R, et al. Expansion of plasmablasts and loss of memory B cells in peripheral blood from COVID-19 patients with pneumonia. Eur J Immunol (2020) 50(9):1283–94. doi: 10.1002/eji.202048838
57. Mann ER, Menon M, Knight SB, Konkel JE, Jagger C, Shaw TN, et al. Longitudinal immune profiling reveals key myeloid signatures associated with COVID-19. Sci Immunol (2020) 5(51). doi: 10.1126/sciimmunol.abd6197
58. Sosa-Hernández VA, Torres-Ruíz J, Cervantes-Díaz R, Romero-Ramírez S, Páez-Franco JC, Meza-Sánchez DE, et al. B Cell Subsets as Severity-Associated Signatures in COVID-19 Patients. Front Immunol (2020) 11:611004. doi: 10.3389/fimmu.2020.611004
59. Shuwa HA, Shaw TN, Knight SB, Wemyss K, McClure FA, Pearmain L, et al. Alterations in T and B cell function persist in convalescent COVID-19 patients. Med (N Y) (2021) 2(6):720–735.e4. doi: 10.1016/j.medj.2021.03.013
60. Mathew D, Giles JR, Baxter AE, Oldridge DA, Greenplate AR, Wu JE, et al. Deep immune profiling of COVID-19 patients reveals distinct immunotypes with therapeutic implications. Science (2020) 369(6508):eabc8511. doi: 10.1126/science.abc8511
61. Hasan A, Al-Ozairi E, Al-Baqsumi Z, Ahmad R, Al-Mulla F. Cellular and Humoral Immune Responses in Covid-19 and Immunotherapeutic Approaches. Immunotargets Ther (2021) 10:63–85. doi: 10.2147/ITT.S280706
62. Wang F, Nie J, Wang H, Zhao Q, Xiong Y, Deng L, et al. Characteristics of Peripheral Lymphocyte Subset Alteration in COVID-19 Pneumonia. J Infect Dis (2020) 221(11):1762–9. doi: 10.1093/infdis/jiaa150
63. Sette A, Crotty S. Adaptive immunity to SARS-CoV-2 and COVID-19. Cell (2021) 184(4):861–80. doi: 10.1016/j.cell.2021.01.007
64. Shahbazi M, Jafari M, Moulana Z, Sepidarkish M, Bagherzadeh M, Rezanejad M, et al. Reduced frequency of T helper 17 and T helper 1 cells and their association with critical coronavirus disease 2019. APMIS (2021) 129(5):271–9. doi: 10.1111/apm.13129
65. Gil-Etayo FJ, Suàrez-Fernández P, Cabrera-Marante O, Arroyo D, Garcinuño S, Naranjo L, et al. T-Helper Cell Subset Response Is a Determining Factor in COVID-19 Progression. Front Cell Infect Microbiol (2021) 11:624483. doi: 10.3389/fcimb.2021.624483
66. Herrmann M, Schulte S, Wildner NH, Wittner M, Brehm TT, Ramharter M, et al. Analysis of Co-inhibitory Receptor Expression in COVID-19 Infection Compared to Acute Plasmodium falciparum Malaria: LAG-3 and TIM-3 Correlate With T Cell Activation and Course of Disease. Front Immunol (2020) 11:1870. doi: 10.3389/fimmu.2020.01870
67. Westmeier J, Paniskaki K, Karaköse Z, Werner T, Sutter K, Dolff S, et al. Impaired Cytotoxic CD8+ T Cell Response in Elderly COVID-19 Patients. MBio (2020) 11(5). doi: 10.1128/mBio.02243-20
68. Kang CK, Han G-C, Kim M, Kim G, Shin HM, Song K-H, et al. Aberrant hyperactivation of cytotoxic T-cell as a potential determinant of COVID-19 severity. Int J Infect Dis (2020) 97:313–21. doi: 10.1016/j.ijid.2020.05.106
69. Diao B, Wang C, Tan Y, Chen X, Liu Y, Ning L, et al. Reduction and Functional Exhaustion of T Cells in Patients With Coronavirus Disease 2019 (COVID-19). Front Immunol (2020) 11:827. doi: 10.3389/fimmu.2020.00827
70. Shahbazi M, Moulana Z, Sepidarkish M, Bagherzadeh M, Rezanejad M, Mirzakhani M, et al. Pronounce expression of Tim-3 and CD39 but not PD1 defines CD8 T cells in critical Covid-19 patients. Microb Pathog (2021) 153:104779. doi: 10.1016/j.micpath.2021.104779
71. Zheng H-Y, Zhang M, Yang C-X, Zhang N, Wang X-C, Yang X-P, et al. Elevated exhaustion levels and reduced functional diversity of T cells in peripheral blood may predict severe progression in COVID-19 patients. Cell Mol Immunol (2020) 17(5):541–3. doi: 10.1038/s41423-020-0401-3
72. Wang F, Hou H, Luo Y, Tang G, Wu S, Huang M, et al. The laboratory tests and host immunity of COVID-19 patients with different severity of illness. JCI Insight (2020) 5(10):e137799. doi: 10.1172/jci.insight.137799
73. Kusnadi A, Ramírez-Suástegui C, Fajardo V, Chee SJ, Meckiff BJ, Simon H, et al. Severely ill COVID-19 patients display impaired exhaustion features in SARS-CoV-2-reactive CD8+ T cells. Sci Immunol (2021) 6(55):eabe4782. doi: 10.1126/sciimmunol.abe4782
74. Winheim E, Rinke L, Lutz K, Reischer A, Leutbecher A, Wolfram L, et al. Impaired function and delayed regeneration of dendritic cells in COVID-19. PloS Pathog (2021) 17(10):e1009742. doi: 10.1371/journal.ppat.1009742
75. Bi J. NK cell dysfunction in patients with COVID-19. Cell Mol Immunol (2022) 19(2):127–9. doi: 10.1038/s41423-021-00825-2
76. Chen Z, John Wherry E. T cell responses in patients with COVID-19. Nat Rev Immunol (2020) 20(9):529–36. doi: 10.1038/s41577-020-0402-6
77. Bernardes JP, Mishra N, Tran F, Bahmer T, Best L, Blase JI, et al. Longitudinal Multi-omics Analyses Identify Responses of Megakaryocytes, Erythroid Cells, and Plasmablasts as Hallmarks of Severe COVID-19. Immunity (2020) 53(6):1296–1314.e9. doi: 10.1016/j.immuni.2020.11.017
78. Stephenson E, Reynolds G, Botting RA, Calero-Nieto FJ, Morgan M, Tuong ZK, et al. The cellular immune response to COVID-19 deciphered by single cell multi-omics across three UK centres. medRxiv (2021). doi: 10.1101/2021.01.13.21249725
79. COvid-19 Multi-omics Blood ATlas (COMBAT) Consortium. A blood atlas of COVID-19 defines hallmarks of disease severity and specificity. Cell (2022) 185(5):916–938.e58. doi: 10.1016/j.cell.2022.01.012
80. Hashem AM, Algaissi A, Almahboub SA, Alfaleh MA, Abujamel TS, Alamri SS, et al. Early Humoral Response Correlates with Disease Severity and Outcomes in COVID-19 Patients. Viruses (2020) 12(12):1390. doi: 10.3390/v12121390
81. Tan W, Lu Y, Zhang J, Wang J, Dan Y, Tan Z, et al. Viral Kinetics and Antibody Responses in Patients with COVID-19. medRxiv (2020). doi: 10.1101/2020.03.24.20042382
82. Röltgen K, Powell AE, Wirz OF, Stevens BA, Hogan CA, Najeeb J, et al. Defining the features and duration of antibody responses to SARS-CoV-2 infection associated with disease severity and outcome. Sci Immunol (2020) 5(54):eabe0240. doi: 10.1126/sciimmunol.abe0240
83. Seow J, Graham C, Merrick B, Acors S, Pickering S, Steel KJA, et al. Longitudinal observation and decline of neutralizing antibody responses in the three months following SARS-CoV-2 infection in humans. Nat Microbiol (2020) 5(12):1598–607. doi: 10.1038/s41564-020-00813-8
84. Garcia-Beltran WF, Lam EC, Astudillo MG, Yang D, Miller TE, Feldman J, et al. COVID-19-neutralizing antibodies predict disease severity and survival. Cell (2021) 184(2):476–488.e11. doi: 10.1016/j.cell.2020.12.015
85. Yang Y, Shen C, Li J, Yuan J, Wei J, Huang F, et al. Plasma IP-10 and MCP-3 levels are highly associated with disease severity and predict the progression of COVID-19. J Allergy Clin Immunol (2020) 146(1):119–127.e4. doi: 10.1016/j.jaci.2020.04.027
86. Zeng F, Huang Y, Guo Y, Yin M, Chen X, Xiao L, et al. Association of inflammatory markers with the severity of COVID-19: A meta-analysis. Int J Infect Dis (2020) 96:467–74. doi: 10.1016/j.ijid.2020.05.055
87. Han H, Ma Q, Li C, Liu R, Zhao L, Wang W, et al. Profiling serum cytokines in COVID-19 patients reveals IL-6 and IL-10 are disease severity predictors. Emerg Microbes Infect (2020) 9(1):1123–30. doi: 10.1080/22221751.2020.1770129
88. Brunetta E, Folci M, Bottazzi B, De Santis M, Gritti G, Protti A, et al. Macrophage expression and prognostic significance of the long pentraxin PTX3 in COVID-19. Nat Immunol (2021) 22(1):19–24. doi: 10.1038/s41590-020-00832-x
89. Fuentes E, Fuentes M, Alarcón M, Palomo I. Immune system dysfunction in the elderly. Acad Bras Cienc (2017) 89(1):285–99. doi: 10.1590/0001-3765201720160487
90. Weyand CM, Goronzy JJ. Aging of the immune system. mechanisms and therapeutic targets. Ann Am Thorac Soc (2016) 13 Suppl 5:S422–8. doi: 10.1513/AnnalsATS.201602-095AW
91. Shaw AC, Goldstein DR, Montgomery RR. Age-dependent dysregulation of innate immunity. Nat Rev Immunol (2013) 13(12):875–87. doi: 10.1038/nri3547
92. Liu K, Chen Y, Lin R, Han K. Clinical features of COVID-19 in elderly patients: A comparison with young and middle-aged patients. J Infect (2020) 80(6):e14–8. doi: 10.1016/j.jinf.2020.03.005
93. Davies NG, Klepac P, Liu Y, Prem K, Jit M, CMMID COVID-19 working group, et al. Age-dependent effects in the transmission and control of COVID-19 epidemics. Nat Med (2020) 26(8):1205–11. doi: 10.1038/s41591-020-0962-9
94. Zhao M, Wang M, Zhang J, Gu J, Zhang P, Xu Y, et al. Comparison of clinical characteristics and outcomes of patients with coronavirus disease 2019 at different ages. Aging (Albany NY) (2020) 12(11):10070–86. doi: 10.18632/aging.103298
95. Pence BD. Severe COVID-19 and aging: are monocytes the key? Geroscience (2020) 42(4):1051–61. doi: 10.1007/s11357-020-00213-0
96. Bajaj V, Gadi N, Spihlman AP, Wu SC, Choi CH, Moulton VR. Aging, Immunity, and COVID-19: How Age Influences the Host Immune Response to Coronavirus Infections? Front Physiol (2020) 11:571416. doi: 10.3389/fphys.2020.571416
97. Cham LB, Pahus MH, Grønhøj K, Olesen R, Ngo H, Monrad I, et al. Effect of Age on Innate and Adaptive Immunity in Hospitalized COVID-19 Patients. J Clin Med (2021) 10(20):4798. doi: 10.3390/jcm10204798
98. Bartleson JM, Radenkovic D, Covarrubias AJ, Furman D, Winer DA, Verdin E. SARS-CoV-2, COVID-19 and the Ageing Immune System. Nat Aging (2021) 1(9):769–82. doi: 10.1038/s43587-021-00114-7
99. Junqueira C, Crespo Â, Ranjbar S, Ingber J, Parry B, Ravid S, et al. SARS-CoV-2 infects blood monocytes to activate NLRP3 and AIM2 inflammasomes, pyroptosis and cytokine release. medRxiv (2021). doi: 10.1101/2021.03.06.21252796
100. Pietrobon AJ, Teixeira FME, Sato MN. I mmunosenescence and Inflammaging: Risk Factors of Severe COVID-19 in Older People. Front Immunol (2020) 11:579220. doi: 10.3389/fimmu.2020.579220
101. Cowling BJ, Fang VJ, Nishiura H, Chan K-H, Ng S, Ip DKM, et al. Increased risk of noninfluenza respiratory virus infections associated with receipt of inactivated influenza vaccine. Clin Infect Dis (2012) 54(12):1778–83. doi: 10.1093/cid/cis307
102. Huang AT, Garcia-Carreras B, Hitchings MDT, Yang B, Katzelnick LC, Rattigan SM, et al. A systematic review of antibody mediated immunity to coronaviruses: kinetics, correlates of protection, and association with severity. Nat Commun (2020) 11(1):4704. doi: 10.1038/s41467-020-18450-4
103. Nickbakhsh S, Ho A, Marques DFP, McMenamin J, Gunson RN, Murcia PR. Epidemiology of seasonal coronaviruses: Establishing the context for the emergence of coronavirus disease 2019. J Infect Dis (2020) 222(1):17–25. doi: 10.1093/infdis/jiaa185
104. Bienvenu LA, Noonan J, Wang X, Peter K. Higher mortality of COVID-19 in males: Sex differences in immune response and cardiovascular comorbidities. Cardiovasc Res (2020) 116(14):2197–206. doi: 10.1093/cvr/cvaa284
105. Klein SL. Sex influences immune responses to viruses, and efficacy of prophylaxis and treatments for viral diseases. Bioessays (2012) 34(12):1050–9. doi: 10.1002/bies.201200099
106. Li Y, Jerkic M, Slutsky AS, Zhang H. Molecular mechanisms of sex bias differences in COVID-19 mortality. Crit Care (2020) 24(1):405. doi: 10.1186/s13054-020-03118-8
107. Taneja V. Sex hormones determine immune response. Front Immunol (2018) 9:1931. doi: 10.3389/fimmu.2018.01931
108. Aboudounya MM, Heads RJ. COVID-19 and Toll-Like Receptor 4 (TLR4): SARS-CoV-2 May Bind and Activate TLR4 to Increase ACE2 Expression, Facilitating Entry and Causing Hyperinflammation. Mediators Inflammation (2021) 2021:8874339. doi: 10.1155/2021/8874339
109. Salvati L, Biagioni B, Vivarelli E, Parronchi P. A gendered magnifying glass on COVID-19. Clin Mol Allergy (2020) 18:14. doi: 10.1186/s12948-020-00129-2
110. Zafari Zangeneh F, Sarmast Shoushtari M. Estradiol and COVID-19: Does 17-Estradiol Have an Immune-Protective Function in Women Against Coronavirus? J Family Reprod Health (2021) 15(3):150–9. doi: 10.18502/jfrh.v15i3.7132
111. Hoffmann M, Kleine-Weber H, Schroeder S, Krüger N, Herrler T, Erichsen S, et al. SARS-CoV-2 Cell Entry Depends on ACE2 and TMPRSS2 and Is Blocked by a Clinically Proven Protease Inhibitor. Cell (2020) 181(2):271–280.e8. doi: 10.1016/j.cell.2020.02.052
112. Rehman S, Ravinayagam V, Nahvi I, Aldossary H, Al-Shammari M, Amiri MSA, et al. Immunity, Sex Hormones, and Environmental Factors as Determinants of COVID-19 Disparity in Women. Front Immunol (2021) 12:680845. doi: 10.3389/fimmu.2021.680845
113. Schroeder M, Schaumburg B, Mueller Z, Parplys A, Jarczak D, Roedl K, et al. High estradiol and low testosterone levels are associated with critical illness in male but not in female COVID-19 patients: A retrospective cohort study. Emerg Microbes Infect (2021) 10(1):1807–18. doi: 10.1080/22221751.2021.1969869
114. Zheng S, Zou Q, Zhang D, Yu F, Bao J, Lou B, et al. Serum level of testosterone predicts disease severity of male COVID-19 patients and is related to T-cell immune modulation by transcriptome analysis. Clin Chim Acta (2022) 524:132–8. doi: 10.1016/j.cca.2021.11.006
115. Zhou F, Yu T, Du R, Fan G, Liu Y, Liu Z, et al. Clinical course and risk factors for mortality of adult inpatients with COVID-19 in Wuhan, China: a retrospective cohort study. Lancet (2020) 395(10229):1054–62. doi: 10.1016/S0140-6736(20)30566-3
116. Guan W-J, Liang W-H, Zhao Y, Liang H-R, Chen Z-S, Li Y-M, et al. Comorbidity and its impact on 1590 patients with COVID-19 in China: a nationwide analysis. Eur Respir J (2020) 55(5):2000547. doi: 10.1183/13993003.00547-2020
117. Thakur B, Dubey P, Benitez J, Torres JP, Reddy S, Shokar N, et al. A systematic review and meta-analysis of geographic differences in comorbidities and associated severity and mortality among individuals with COVID-19. Sci Rep (2021) 11:8562. doi: 10.1038/s41598-021-88130-w
118. Del Valle DM, Kim-Schulze S, Huang H-H, Beckmann ND, Nirenberg S, Wang B, et al. An inflammatory cytokine signature predicts COVID-19 severity and survival. Nat Med (2020) 26(10):1636–43. doi: 10.1038/s41591-020-1051-9
119. Guo W, Li M, Dong Y, Zhou H, Zhang Z, Tian C, et al. Diabetes is a risk factor for the progression and prognosis of COVID-19. Diabetes Metab Res Rev (2020) 36(7):e3319. doi: 10.1002/dmrr.3319
120. Severe Covid-19 GWAS Group, Ellinghaus D, Degenhardt F, Bujanda L, Buti M, Albillos A, et al. Genomewide Association Study of Severe Covid-19 with Respiratory Failure. N Engl J Med (2020) 383(16):1522–34. doi: 10.1056/NEJMoa2020283
121. D’Antonio M, Nguyen JP, Arthur TD, Matsui H, COVID-19 Host Genetics Initiative, D’Antonio-Chronowska A, et al. SARS-CoV-2 susceptibility and COVID-19 disease severity are associated with genetic variants affecting gene expression in a variety of tissues. Cell Rep (2021) 37(7):110020. doi: 10.1016/j.celrep.2021.110020
122. Wang F, Huang S, Gao R, Zhou Y, Lai C, Li Z, et al. Initial whole-genome sequencing and analysis of the host genetic contribution to COVID-19 severity and susceptibility. Cell Discovery (2020) 6(1):83. doi: 10.1038/s41421-020-00231-4
123. Martínez-Gómez LE, Herrera-López B, Martinez-Armenta C, Ortega-Peña S, Camacho-Rea MDC, Suarez-Ahedo C, et al. ACE and ACE2 Gene Variants Are Associated With Severe Outcomes of COVID-19 in Men. Front Immunol (2022) 13:812940. doi: 10.3389/fimmu.2022.812940
124. Rokni M, Heidari Nia M, Sarhadi M, Mirinejad S, Sargazi S, Moudi M, et al. Association of TMPRSS2 Gene Polymorphisms with COVID-19 Severity and Mortality: a Case-Control Study with Computational Analyses. Appl Biochem Biotechnol (2022) 194(8):3507–26. doi: 10.1007/s12010-022-03885-w
125. Fallerini C, Daga S, Mantovani S, Benetti E, Picchiotti N, Francisci D, et al. Association of Toll-like receptor 7 variants with life-threatening COVID-19 disease in males: findings from a nested case-control study. eLife (2021) 10:e67569. doi: 10.7554/eLife.67569
126. Muñiz-Diaz E, Llopis J, Parra R, Roig I, Ferrer G, Grifols J, et al. Relationship between the ABO blood group and COVID-19 susceptibility, severity and mortality in two cohorts of patients. Blood Transfus (2021) 19(1):54–63. doi: 10.2450/2020.0256-20
127. COVID-19 Host Genetics Initiative. Mapping the human genetic architecture of COVID-19. (2021) 600(7889):472–7. doi: 10.1038/s41586-021-03767-x
128. Vuille-dit-Bille RN, Camargo SM, Emmenegger L, Sasse T, Kummer E, Jando J, et al. Human intestine luminal ACE2 and amino acid transporter expression increased by ACE-inhibitors. Amino Acids (2015) 47(4):693–705. doi: 10.1007/s00726-014-1889-6
129. D’Antonio M, COVID-19 Host Genetics Initiative, Arthur TD, Nguyen JP, Matsui H, D’Antonio-Chronowska A, et al. Insights into genetic factors contributing to variability in SARS-CoV-2 susceptibility and COVID-19 disease severity. medRxiv (2021) 21(25):6423. doi: 10.1101/2021.05.10.21256423
130. Huffman JE, Butler-Laporte G, Khan A, Pairo-Castineira E, Drivas TG, Peloso GM, et al. Multi-ancestry fine mapping implicates OAS1 splicing in risk of severe COVID-19. Nat Genet (2022) 54(2):125–7. doi: 10.1038/s41588-021-00996-8
131. Zhang H, Maqsudi S, Rainczuk A, Duffield N, Lawrence J, Keane FM, et al. Identification of novel dipeptidyl peptidase 9 substrates by two-dimensional differential in-gel electrophoresis. FEBS J (2015) 282(19):3737–57. doi: 10.1111/febs.13371
132. Pairo-Castineira E, Clohisey S, Klaric L, Bretherick AD, Rawlik K, Pasko D, et al. Genetic mechanisms of critical illness in COVID-19. Nature (2021) 591(7848):92–8. doi: 10.1038/s41586-020-03065-y
133. Wu Y, Feng Z, Li P, Yu Q. Relationship between ABO blood group distribution and clinical characteristics in patients with COVID-19. Clin Chim Acta (2020) 509:220–3. doi: 10.1016/j.cca.2020.06.026
134. Muromoto R, Oritani K, Matsuda T. Current understanding of the role of tyrosine kinase 2 signaling in immune responses. World J Biol Chem (2022) 13(1):1–14. doi: 10.4331/wjbc.v13.i1.1
135. Petes C, Odoardi N, Gee K. The Toll for Trafficking: Toll-Like Receptor 7 Delivery to the Endosome. Front Immunol (2017) 8:1075. doi: 10.3389/fimmu.2017.01075
136. Asano T, Boisson B, Onodi F, Matuozzo D, Moncada-Velez M, Maglorius Renkilaraj MRL, et al. X-linked recessive TLR7 deficiency in ~1% of men under 60 years old with life-threatening COVID-19. Sci Immunol (2021) 6(62):eabl4348. doi: 10.1126/sciimmunol.abl4348
137. Mosaad YM. Clinical role of human leukocyte antigen in health and disease. Scand J Immunol (2015) 82(4):283–306. doi: 10.1111/sji.12329
138. Hou Y, Zhao J, Martin W, Kallianpur A, Chung MK, Jehi L, et al. New insights into genetic susceptibility of COVID-19: an ACE2 and TMPRSS2 polymorphism analysis. BMC Med (2020) 18:216. doi: 10.1186/s12916-020-01673-z
139. Saengsiwaritt W, Jittikoon J, Chaikledkaew U, Udomsinprasert W. Genetic polymorphisms of ACE1, ACE2, and TMPRSS2 associated with COVID-19 severity: A systematic review with meta-analysis. Rev Med Virol (2022) 32(4):e2323. doi: 10.1002/rmv.2323
140. Costela-Ruiz VJ, Illescas-Montes R, Puerta-Puerta JM, Ruiz C, Melguizo-Rodríguez L. SARS-CoV-2 infection: The role of cytokines in COVID-19 disease. Cytokine Growth Factor Rev (2020) 54:62–75. doi: 10.1016/j.cytogfr.2020.06.001
141. Hosseini A, Hashemi V, Shomali N, Asghari F, Gharibi T, Akbari M, et al. Innate and adaptive immune responses against coronavirus. BioMed Pharmacother (2020) 132:110859. doi: 10.1016/j.biopha.2020.110859
142. Laing AG, Lorenc A, Del Molino Del Barrio I, Das A, Fish M, Monin L, et al. A dynamic COVID-19 immune signature includes associations with poor prognosis. Nat Med (2020) 26(10):1623–35. doi: 10.1038/s41591-020-1038-6
143. Zhou Y, Fu B, Zheng X, Wang D, Zhao C, Qi Y, et al. Pathogenic T-cells and inflammatory monocytes incite inflammatory storms in severe COVID-19 patients. Natl Sci Rev (2020) 7(6):998–1002. doi: 10.1093/nsr/nwaa041
144. Middleton EA, He X-Y, Denorme F, Campbell RA, Ng D, Salvatore SP, et al. Neutrophil extracellular traps contribute to immunothrombosis in COVID-19 acute respiratory distress syndrome. Blood (2020) 136(10):1169–79. doi: 10.1182/blood.2020007008
145. Darif D, Hammi I, Kihel A, El Idrissi Saik I, Guessous F, Akarid K. The pro-inflammatory cytokines in COVID-19 pathogenesis: What goes wrong? Microb Pathog (2021) 153:104799. doi: 10.1016/j.micpath.2021.104799
146. Song J-W, Zhang C, Fan X, Meng F-P, Xu Z, Xia P, et al. Immunological and inflammatory profiles in mild and severe cases of COVID-19. Nat Commun (2020) 11(1):3410. doi: 10.1038/s41467-020-17240-2
147. Tavakolpour S, Rakhshandehroo T, Wei EX, Rashidian M. Lymphopenia during the COVID-19 infection: What it shows and what can be learned. Immunol Lett (2020) 225:31–2. doi: 10.1016/j.imlet.2020.06.013
148. Rydyznski Moderbacher C, Ramirez SI, Dan JM, Grifoni A, Hastie KM, Weiskopf D, et al. Antigen-Specific Adaptive Immunity to SARS-CoV-2 in Acute COVID-19 and Associations with Age and Disease Severity. Cell (2020) 183(4):996–1012.e19. doi: 10.1016/j.cell.2020.09.038
149. Rodrigues TS, de Sá KSG, Ishimoto AY, Becerra A, Oliveira S, Almeida L, et al. Inflammasomes are activated in response to SARS-CoV-2 infection and are associated with COVID-19 severity in patients. J Exp Med (2021) 218(3). doi: 10.1084/jem.20201707
150. Zheng Y, Liu X, Le W, Xie L, Li H, Wen W, et al. A human circulating immune cell landscape in aging and COVID-19. Protein Cell (2020) 11(10):740–70. doi: 10.1007/s13238-020-00762-2
151. Jin J-M, Bai P, He W, Wu F, Liu X-F, Han D-M, et al. Gender Differences in Patients With COVID-19: Focus on Severity and Mortality. Front Public Health (2020) 8:152. doi: 10.3389/fpubh.2020.00152
152. Ciarambino T, Para O, Giordano M. Immune system and COVID-19 by sex differences and age. Womens Health (Lond Engl) (2021) 17:17455065211022262. doi: 10.1177/17455065211022262
153. Singh AK, Misra A. Impact of COVID-19 and comorbidities on health and economics: Focus on developing countries and India. Diabetes Metab Syndr (2020) 14(6):1625–30. doi: 10.1016/j.dsx.2020.08.032
154. Secolin R, de Araujo TK, Gonsales MC, Rocha CS, Naslavsky M, Marco LD, et al. Genetic variability in COVID-19-related genes in the Brazilian population. Hum Gen Variation (2021) 8:15. doi: 10.1038/s41439-021-00146-w
155. Khor S-S, Omae Y, Nishida N, Sugiyama M, Kinoshita N, Suzuki T, et al. HLA-A*11:01:01:01, HLA-C*12:02:02:01-HLA-B*52:01:02:02, Age and Sex Are Associated With Severity of Japanese COVID-19 With Respiratory Failure. Front Immunol (2021) 12:658570. doi: 10.3389/fimmu.2021.658570
156. Langton DJ, Bourke SC, Lie BA, Reiff G, Natu S, Darlay R, et al. The influence of HLA genotype on the severity of COVID-19 infection. HLA (2021) 98(1):14–22. doi: 10.1111/tan.14284
157. Velavan TP, Pallerla SR, Rüter J, Augustin Y, Kremsner PG, Krishna S, et al. Host genetic factors determining COVID-19 susceptibility and severity. EBioMedicine (2021) 72:103629. doi: 10.1016/j.ebiom.2021.103629
158. Guihot A, Litvinova E, Autran B, Debré P, Vieillard V. Cell-Mediated Immune Responses to COVID-19 Infection. Front Immunol (2020) 11:1662. doi: 10.3389/fimmu.2020.01662
159. Cao Y, Wang X, Peng G. SCSA: A Cell Type Annotation Tool for Single-Cell RNA-seq Data. Front Genet (2020) 11:490. doi: 10.3389/fgene.2020.00490
Keywords: cellular heterogeneity, infectious disease, immune response, COVID-19, RNA-seq, single-cell genomics
Citation: Khare K and Pandey R (2022) Cellular heterogeneity in disease severity and clinical outcome: Granular understanding of immune response is key. Front. Immunol. 13:973070. doi: 10.3389/fimmu.2022.973070
Received: 19 June 2022; Accepted: 26 July 2022;
Published: 22 August 2022.
Edited by:
Antonio Serrano, Tissue Engineering and Autoimmunity Group, SpainReviewed by:
Mohammad Mirzakhani, Mashhad University of Medical Sciences, IranFrancisco Javier Gil, University Hospital of Salamanca, Spain
Copyright © 2022 Khare and Pandey. This is an open-access article distributed under the terms of the Creative Commons Attribution License (CC BY). The use, distribution or reproduction in other forums is permitted, provided the original author(s) and the copyright owner(s) are credited and that the original publication in this journal is cited, in accordance with accepted academic practice. No use, distribution or reproduction is permitted which does not comply with these terms.
*Correspondence: Rajesh Pandey, cmFqZXNocEBpZ2liLmlu; cmFqZXNoLnBAaWdpYi5yZXMuaW4=